Accuracy in Speech Data Collection: Best Practices
How do Speech Data Collection Services Ensure Data Accuracy?
Accurate and reliable speech data is essential for developing robust AI and machine learning systems. Speech data collection services play a crucial role in this process, ensuring that the data gathered is precise and of high quality. In this short guide, we will explore how these services ensure data accuracy, discussing best practices and standards in the field.
Accuracy is paramount. The success of AI and machine learning models heavily depends on the quality of the data they are trained on. This raises several questions for those involved in data science and technology:
- How can speech data collection services ensure the accuracy of their data?
- What methods are used to verify the quality of speech data?
- What tools and technologies are employed to maintain high standards in speech data collection?
Addressing these questions is vital for data scientists, AI developers, quality assurance specialists, technology firms, and academic researchers. This short guide aims to provide comprehensive insights into the best practices and standards for ensuring accurate speech data collection.
Best Practices and Insights For Speech Data Accuracy
Importance of Data Accuracy in Speech Collection
Accurate speech data is the cornerstone of any successful AI-driven application. Inaccuracies can lead to misinterpretations, errors in decision-making, and ultimately, failure of the system. Here’s why data accuracy is critical:
- Model Performance: High-quality, accurate data improves the performance of speech recognition models, making them more reliable and effective.
- User Experience: Accurate speech data ensures a seamless user experience, as applications respond correctly to voice commands.
- Trust and Credibility: Organisations that utilise accurate data build trust and credibility with their users, stakeholders, and clients.
- Compliance: Ensuring data accuracy helps meet regulatory requirements and standards, which is crucial for legal and ethical reasons.
Ensuring the accuracy of speech data is vital to the success of AI-driven applications, as it directly impacts the performance and reliability of these systems. Inaccurate data can lead to significant errors, misinterpretations, and overall system failures, which can be costly and damaging to an organisation’s reputation. Let’s delve deeper into why data accuracy is so crucial.
Model Performance
High-quality, accurate data is essential for training effective speech recognition models. When the data used to train these models is accurate, the resulting algorithms are more reliable, delivering better performance in real-world applications. Accurate speech data helps the model understand and process various accents, dialects, and speech nuances, which enhances its ability to accurately transcribe or respond to spoken inputs. Poor data quality, on the other hand, can lead to models that are biased, less effective, and more prone to errors, ultimately reducing their usability and effectiveness.
User Experience
The end-user experience is greatly influenced by the accuracy of speech data. Applications that rely on speech recognition, such as virtual assistants, transcription services, and customer service bots, must accurately interpret user commands to provide satisfactory interactions. Accurate speech data ensures these applications can understand and respond correctly to user inputs, leading to a seamless and efficient user experience. Conversely, inaccuracies can frustrate users, reduce trust in the application, and lead to decreased adoption and usage.
Trust and Credibility
Organisations that consistently utilise accurate data build a reputation for reliability and trustworthiness. Stakeholders, including users, clients, and partners, are more likely to trust a system that demonstrates high accuracy in its operations. This trust is critical for the adoption of AI-driven applications, as users need confidence that their interactions with the system will be correctly understood and processed. Maintaining high standards of data accuracy helps organisations establish and retain credibility in a competitive market.
Compliance
Ensuring data accuracy is also crucial for meeting regulatory requirements and standards. Various industries, such as healthcare, finance, and telecommunications, have stringent regulations governing data quality and accuracy. Non-compliance can result in legal repercussions, financial penalties, and damage to an organisation’s reputation. Accurate data helps organisations adhere to these regulations, ensuring that their operations remain lawful and ethically sound.
Methods for Verifying Speech Data Quality
Ensuring data accuracy involves multiple verification methods:
- Manual Review: Human reviewers listen to and analyse speech data samples to identify and correct errors.
- Automated Checks: Algorithms can detect inconsistencies and anomalies in speech data, flagging them for further review.
- Cross-Validation: Comparing multiple datasets or sources to verify the consistency and accuracy of the speech data.
- Pilot Testing: Running initial tests on a small dataset to identify potential issues before full-scale data collection.
- Feedback Loops: Implementing feedback mechanisms where users report errors, which are then used to refine and improve the data.
Maintaining high data accuracy involves implementing robust verification methods. These methods help identify and correct errors, ensuring the data used for training and analysis is of the highest quality.
Manual Review
Manual review involves human reviewers listening to and analysing speech data samples. This method is particularly effective for identifying subtle errors that automated systems might miss. Reviewers can check for accuracy in transcription, proper labelling, and the presence of any anomalies. Although time-consuming, manual review is a critical step in ensuring data quality, especially for complex or nuanced speech data.
Automated Checks
Automated systems can perform initial checks to detect inconsistencies and anomalies in speech data. These algorithms can flag discrepancies in transcription accuracy, identify outliers, and highlight potential errors for further review. Automated checks are efficient for processing large datasets, providing a preliminary layer of quality assurance before more detailed manual reviews.
Cross-Validation
Cross-validation involves comparing multiple datasets or sources to verify the consistency and accuracy of speech data. By cross-referencing data from different sources, discrepancies can be identified and corrected. This method is particularly useful for large-scale projects where data is collected from various environments and conditions, ensuring that the final dataset is comprehensive and accurate.
Pilot Testing
Before full-scale data collection, running initial tests on a small dataset can help identify potential issues. Pilot testing allows organisations to fine-tune their data collection processes, adjust methodologies, and address any problems early on. This proactive approach helps ensure that the final data collection process is more efficient and accurate.
Feedback Loops
Implementing feedback mechanisms where users can report errors and provide input on data accuracy is invaluable. This feedback is used to refine and improve data collection and processing methods continuously. By incorporating user feedback, organisations can address real-world issues more effectively, enhancing the overall accuracy of their speech data.
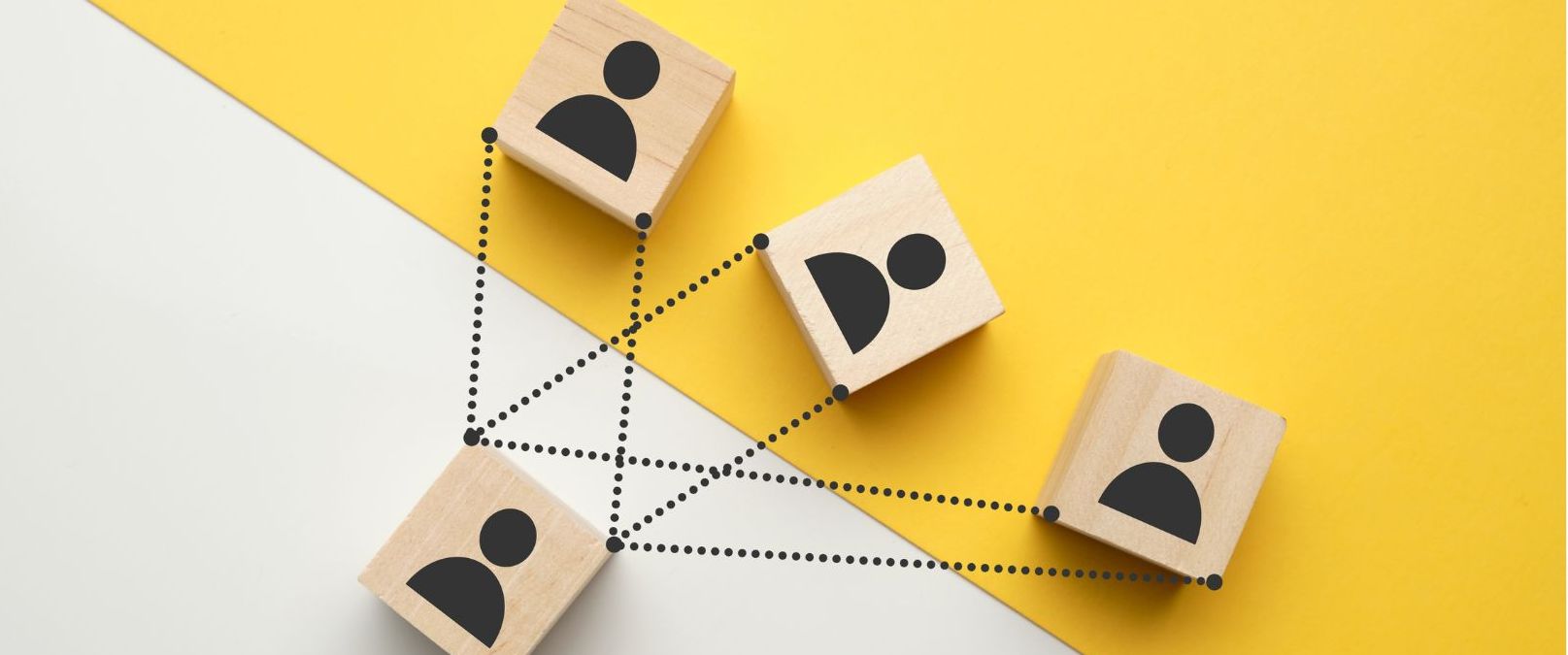
Tools and Technologies for Accurate Speech Data
Several tools and technologies are instrumental in maintaining data accuracy:
- Speech Recognition Software: Advanced software that transcribes speech with high accuracy, reducing manual efforts.
- Natural Language Processing (NLP) Tools: These tools analyse and process speech data, ensuring linguistic accuracy.
- Data Annotation Platforms: Platforms that provide accurate labelling and annotation of speech data.
- Machine Learning Algorithms: Algorithms that continuously learn and improve from data, enhancing accuracy over time.
- Quality Assurance Tools: Tools designed specifically for QA processes, ensuring each data point meets the required standards.
The advancement of technology has introduced a range of tools and technologies that play a crucial role in maintaining data accuracy. These tools streamline data collection, annotation, and validation processes, ensuring high standards are met.
Speech Recognition Software
Advanced speech recognition software transcribes speech with high accuracy, significantly reducing the need for manual transcription efforts. These tools leverage machine learning algorithms to understand and process spoken language, delivering precise transcriptions that form the basis for further analysis and application development.
Natural Language Processing (NLP) Tools
NLP tools are designed to analyse and process speech data, ensuring linguistic accuracy. These tools can handle various aspects of language, including syntax, semantics, and context, providing a deeper understanding of the speech data. NLP tools are essential for applications that require not just transcription but also an understanding of the meaning and intent behind spoken words.
Data Annotation Platforms
Accurate labelling and annotation of speech data are critical for training effective models. Data annotation platforms provide the infrastructure needed for systematic and precise labelling of speech data. These platforms often include tools for tagging, segmenting, and categorising speech data, ensuring that every aspect of the data is correctly annotated.
Machine Learning Algorithms
Machine learning algorithms continuously learn and improve from data, enhancing accuracy over time. These algorithms can adapt to new patterns and variations in speech, becoming more precise with each iteration. Employing machine learning for data validation and correction ensures that the accuracy of speech data improves progressively.
Quality Assurance Tools
Dedicated quality assurance tools are designed to monitor and maintain data quality throughout the collection and processing stages. These tools can automate various QA processes, such as consistency checks, error detection, and compliance verification, ensuring that each data point meets the required standards.
Industry Standards for Speech Data Collection
Adhering to industry standards is essential for maintaining data accuracy:
- ISO Standards: International standards for data quality management.
- IEEE Standards: Guidelines for the quality and interoperability of speech data.
- GDPR Compliance: Ensuring data practices comply with the General Data Protection Regulation for privacy and security.
- NIST Standards: National Institute of Standards and Technology guidelines for speech data accuracy.
- Sector-Specific Regulations: Adhering to standards specific to industries like healthcare, finance, and education.
Adhering to industry standards is essential for ensuring data accuracy. These standards provide guidelines and best practices that help organisations maintain high-quality data.
ISO Standards
International Organisation for Standardisation (ISO) standards provide a framework for data quality management. ISO standards cover various aspects of data accuracy, from collection methodologies to validation processes. Following ISO standards ensures that speech data collection practices meet globally recognised quality benchmarks.
IEEE Standards
The Institute of Electrical and Electronics Engineers (IEEE) sets guidelines for the quality and interoperability of speech data. IEEE standards focus on ensuring that speech data can be used effectively across different systems and applications, promoting consistency and reliability.
GDPR Compliance
The General Data Protection Regulation (GDPR) imposes strict requirements on data practices, including accuracy and privacy. Ensuring compliance with GDPR is crucial for organisations operating in or serving the European Union. Accurate data practices help meet these regulatory requirements, protecting user privacy and maintaining legal compliance.
NIST Standards
The National Institute of Standards and Technology (NIST) provides guidelines for speech data accuracy. NIST standards are widely adopted in various industries, offering a robust framework for data validation and quality assurance. Following NIST guidelines ensures that speech data collection practices are thorough and reliable.
Sector-Specific Regulations
Different industries have specific regulations governing data quality. For example, the healthcare industry has stringent requirements for data accuracy to ensure patient safety and care quality. Similarly, the finance sector demands high data accuracy to prevent fraud and ensure regulatory compliance. Adhering to these sector-specific regulations is critical for maintaining data accuracy and operational integrity.
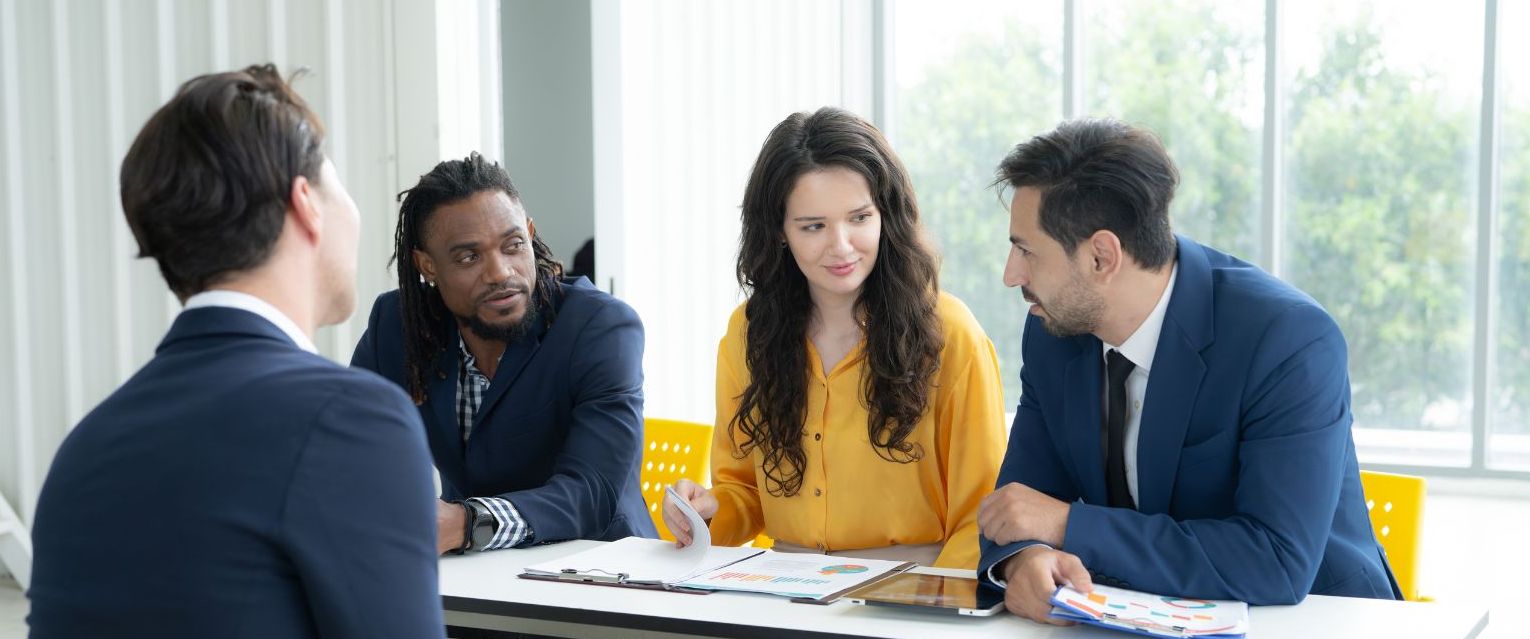
Case Studies of Successful Data Accuracy Implementation
Understanding how organisations have successfully implemented data accuracy practices provides valuable insights:
- Google: Employs extensive data validation and cross-checking methods to ensure the accuracy of its speech recognition models.
- Amazon: Utilises a combination of manual review and automated checks to maintain high data accuracy for Alexa.
- Apple: Implements rigorous QA processes and feedback loops for Siri’s speech data.
- IBM Watson: Uses advanced NLP tools and continuous learning algorithms to enhance speech data accuracy.
- Way With Words: A leading speech collection service provider, ensures high accuracy through robust quality control processes and compliance with industry standards.
Examining case studies of successful data accuracy implementation provides valuable insights into effective practices and methodologies.
Google employs extensive data validation and cross-checking methods to ensure the accuracy of its speech recognition models. By leveraging a combination of manual reviews and automated checks, Google maintains high data accuracy. Their approach includes continuous feedback loops and machine learning algorithms that improve accuracy over time. This multi-layered strategy ensures that Google’s speech recognition systems are among the most reliable and effective.
Amazon
Amazon utilises a blend of manual review and automated checks to maintain high data accuracy for Alexa. Their quality assurance processes involve extensive pilot testing and user feedback mechanisms. By continuously refining their data collection methods based on user input, Amazon ensures that Alexa delivers accurate and responsive interactions. This commitment to data accuracy is a key factor in Alexa’s widespread adoption and success.
Apple
Apple implements rigorous QA processes and feedback loops for Siri’s speech data. Their approach includes cross-validation and pilot testing to identify and address potential issues early in the data collection process. Apple also employs advanced NLP tools to ensure linguistic accuracy, enhancing Siri’s ability to understand and respond to user commands accurately.
IBM Watson
IBM Watson uses advanced NLP tools and continuous learning algorithms to enhance speech data accuracy. Their quality assurance processes include automated checks, manual reviews, and feedback loops. By adopting a comprehensive approach to data accuracy, IBM Watson ensures that its AI systems deliver reliable and effective performance in various applications.
Way With Words
Way With Words, a leading speech collection service provider, ensures high accuracy through robust quality control processes and compliance with industry standards. Their approach includes rigorous manual reviews, automated validation checks, and adherence to international standards. By maintaining high data accuracy, Way With Words supports the development of effective AI and machine learning applications.
Key Tips for Ensuring Speech Data Accuracy
- Implement Multi-Layered QA Processes: Combine manual reviews, automated checks, and feedback loops for comprehensive quality assurance.
- Utilise Advanced Tools: Leverage state-of-the-art speech recognition and NLP tools to maintain high data accuracy.
- Adhere to Industry Standards: Follow international and sector-specific standards to ensure compliance and quality.
- Conduct Pilot Tests: Run pilot tests to identify and address potential issues early in the data collection process.
- Engage with Users: Implement feedback mechanisms to continuously improve data accuracy based on user input.
Ensuring the accuracy of speech data collection is a multi-faceted process involving various methods, tools, and industry standards. By prioritising data accuracy, organisations can enhance the performance of their AI models, improve user experience, build trust, and comply with regulatory requirements. Implementing best practices and learning from successful case studies are essential steps towards achieving accurate speech data.
As a key piece of advice, always consider the continuous improvement of data accuracy processes through feedback and the adoption of advanced technologies. The dynamic nature of speech data collection requires constant vigilance and adaptation to maintain the highest standards of quality.
Further Accuracy in Speech Data Resources
Data Quality – This Wikipedia article discusses the principles of data quality, including definitions, dimensions, and methodologies for ensuring high-quality data, which are applicable to speech data collection.
Way With Words Speech Collection – Way With Words ensures high accuracy in speech datasets by employing rigorous quality control processes. Their approach guarantees that the collected data meets the stringent requirements necessary for effective machine learning and AI applications.
By following these best practices and utilising the available resources, you can ensure that your speech data collection efforts yield accurate and reliable data, crucial for the success of any AI and machine learning initiative.