Timeline of Speech Data Collection: From Start to Finish
How Long Does it Take to Collect a Large Dataset of Speech Data?
The process of collecting a large dataset of speech data is a nuanced endeavour, influenced by numerous variables that determine how long it takes to complete to ensure speech data quality. Project managers, data scientists, AI developers, technology firms, and academic researchers often find themselves asking, “How long will it take to collect a comprehensive speech dataset?” Addressing this question involves exploring the primary factors impacting speech data collection time, planning the project effectively, and understanding how to streamline the process when necessary.
Common questions often include:
- What factors impact the speed of speech data collection?
- How can project timelines for speech data collection be optimised?
- What are realistic timelines for various types of speech data projects?
In this short guide, we’ll examine critical topics to provide insights into the “Speech Data Collection Time,” “Data Collection Timeline,” and effective strategies for “Collecting Large Datasets.” Through this, you’ll gain a clear understanding of the timeline for speech data collection from start to finish.
Key Topics to Explore For Data Collecting
Factors Influencing Data Collection Time
Collecting speech data is affected by factors such as the volume of data needed, language diversity, demographic requirements, and technical requirements. For example, gathering a diverse dataset across multiple accents and dialects takes longer than collecting data in a single language or dialect.
Key factors to consider:
- Volume and Scope: Larger datasets with stringent requirements will take longer to collect.
- Demographic Requirements: If the data must cover a wide range of age groups, genders, and regional accents, collection will be more time-consuming.
- Technical Requirements: Specific technical needs, such as the quality of recording and device consistency, can extend collection timelines.
The timeline for collecting speech data is heavily influenced by several intersecting factors that determine how swiftly and efficiently a project can progress. Volume and Scope are significant considerations; the larger and more detailed the dataset, the longer it will take to gather. For example, a project requiring 1,000 hours of speech in multiple languages with various regional dialects and age groups will naturally extend the timeline compared to a more streamlined dataset in a single language. Larger projects often necessitate additional layers of management, data quality checks, and perhaps a greater pool of participants, all of which contribute to longer timelines.
Demographic Requirements also play a crucial role. Diverse datasets that aim to include multiple age groups, genders, or ethnic backgrounds require more targeted recruitment efforts. Finding a balanced demographic representation can be particularly challenging in multilingual projects, where each language and dialect must meet the same diversity standards. Additional requirements, such as speaker accent diversity or specific vocal characteristics, can also extend the project timeline, as locating the right participants becomes more complex.
Technical Requirements further influence data collection time. For instance, a project that demands high-fidelity audio recordings without background noise might require specialised recording equipment and environments. Additionally, maintaining consistency in recording devices and environments across participants requires meticulous organisation and instruction, especially if participants are dispersed across different regions. Thus, the technical specifications set forth by the project dictate not only the quality of the data but also the speed and feasibility of its collection.
Typical Timelines for Speech Data Projects
Timelines vary depending on the project’s complexity. A straightforward collection project with minimal requirements might take just a few weeks, while projects with complex requirements can span several months.
- Small Projects: 4-8 weeks
- Medium Projects: 2-4 months
- Large Projects: 6-12 months or more
Speech data projects can vary widely in duration, with timelines tailored to the specific needs and scale of the project. Small Projects might range from 4 to 8 weeks, particularly if the data requirements are straightforward and the demographic pool is readily accessible. These projects often involve basic demographic needs and minimal language variation, allowing for quicker recruitment and data gathering. Small projects are also more likely to use automated validation processes, expediting the workflow further.
Medium Projects typically extend to 2-4 months, as they involve more complex requirements, such as additional dialects or broader demographic categories. These projects might require hiring field managers or local supervisors to oversee data collection in different regions. The need for added layers of quality control—especially in ensuring demographic representation and technical standards—requires more intensive management and extends the project timeline.
For Large Projects, timelines can extend from 6 months to over a year. Large-scale projects often involve multilingual datasets with intricate demographic and technical specifications, requiring significant resources in terms of staff, technology, and time. The recruitment, validation, and quality control processes are more robust and frequently involve several iterative cycles. The long timeline reflects the need for continuous monitoring, data validation, and potential participant management across different regions or countries.
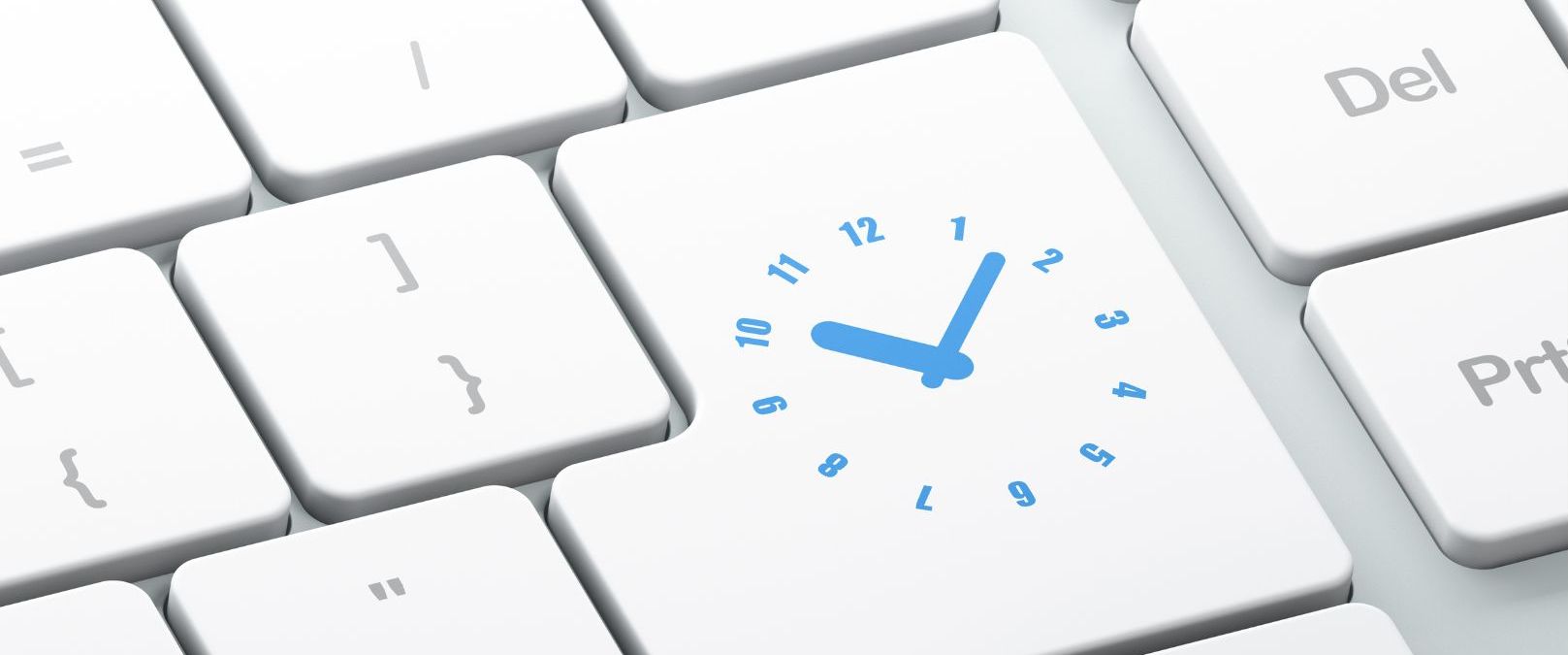
Accelerating the Data Collection Process
To speed up data collection, consider streamlining participant recruitment, employing specialised technology, and using well-planned workflows. Collaborating with established data collection providers can also help fast-track the process.
Strategies for acceleration:
- Use Automated Quality Checks: Automated checks help identify issues early, reducing the time spent on quality control.
- Efficient Participant Management: Organise participant recruitment and data submission to prevent bottlenecks.
Accelerating the data collection process without compromising quality is a priority for many project managers. One of the most effective ways to speed up the process is by streamlining participant recruitment. Leveraging targeted recruitment platforms or working with recruitment agencies experienced in speech data collection can significantly reduce the time spent on finding qualified participants. Additionally, having clear onboarding processes and easily accessible resources for participants ensures they understand the requirements quickly, thus reducing delays caused by participant errors or misunderstandings.
Using Specialised Technology is another essential strategy. Tools that support Automated Quality Checks allow teams to identify and rectify issues in real time, such as audio quality problems or demographic mismatches. Automating these checks helps reduce the volume of data that needs to be manually reviewed, freeing up valuable time for other aspects of the project. Likewise, adopting AI-driven tools that manage participant tracking, demographic compliance, and progress updates contributes to faster, more organised data collection.
Efficient Participant Management plays a critical role in maintaining the momentum of the project. Setting up clear communication channels and a responsive support system for participants keeps them engaged and informed, minimising dropouts and re-recording needs. Organising participant recruitment and data submission in a phased approach can also prevent bottlenecks by staggering the flow of submissions, ensuring that each phase of the project receives adequate attention without overwhelming the team.
Case Studies on Efficient Data Collection
Exploring case studies where data collection timelines were effectively managed can provide insights into best practices and innovative approaches. Highlighting specific methods used to overcome common challenges allows teams to apply similar strategies to their projects.
Analysing case studies on successful data collection projects offers practical insights into managing similar projects effectively. In one case, a large-scale multilingual project aimed to gather speech samples in over 20 languages with specific dialectical and demographic requirements. By partnering with local agencies for participant recruitment and setting up mobile recording booths in high-traffic areas, the project team achieved a high-quality dataset within a reduced timeframe. The use of localised recruitment and on-site recording solutions helped overcome recruitment and technical challenges while adhering to strict demographic standards.
In another instance, a smaller, time-sensitive project focused on gathering data from urban youth for an AI training model. The team used an app-based data submission platform, allowing participants to record and submit their speech data from their own devices. By implementing automatic quality checks within the app, the project was able to maintain data integrity while reducing manual review time, completing the project in half the estimated timeline. This approach demonstrates the importance of user-friendly technology and automation in streamlining data collection.
These case studies underscore that flexible strategies, innovative technology, and targeted recruitment can significantly impact data collection efficiency. While each project has unique challenges, adapting best practices from similar cases can enhance project planning and execution.
Planning and Managing Data Collection Projects
Effective planning is critical to successful data collection. A structured timeline with clear milestones and deliverables helps ensure timely project completion.
Elements of a successful plan include:
- Milestone Setup: Break down the project into smaller milestones for progress tracking.
- Risk Management: Identify potential risks, such as participant dropout or technical issues, and develop mitigation strategies.
Effective planning and management are essential for any successful speech data collection project. A structured approach begins with setting clear goals and timelines, aligning the project’s objectives with realistic milestones. Milestone Setup helps ensure that each phase of the project, from recruitment to data validation, progresses in an organised manner. By breaking down large tasks into smaller, manageable milestones, teams can better monitor their progress, make timely adjustments, and ensure resources are allocated efficiently.
Risk Management is also crucial in the planning stage, as data collection projects often encounter unforeseen challenges. Potential risks, such as participant dropouts, device malfunctions, or quality issues, should be anticipated and addressed in the project’s risk management strategy. Creating a contingency plan allows the project to proceed smoothly even when unexpected issues arise, ensuring timelines and data quality standards remain intact.
To enhance coordination across teams and stakeholders, establishing a robust Project Management Framework with defined roles, responsibilities, and communication channels is essential. This framework provides transparency and ensures all team members understand their tasks, helping to avoid bottlenecks and miscommunications. Regular status meetings and progress updates can keep the project on track, fostering accountability and facilitating problem-solving.
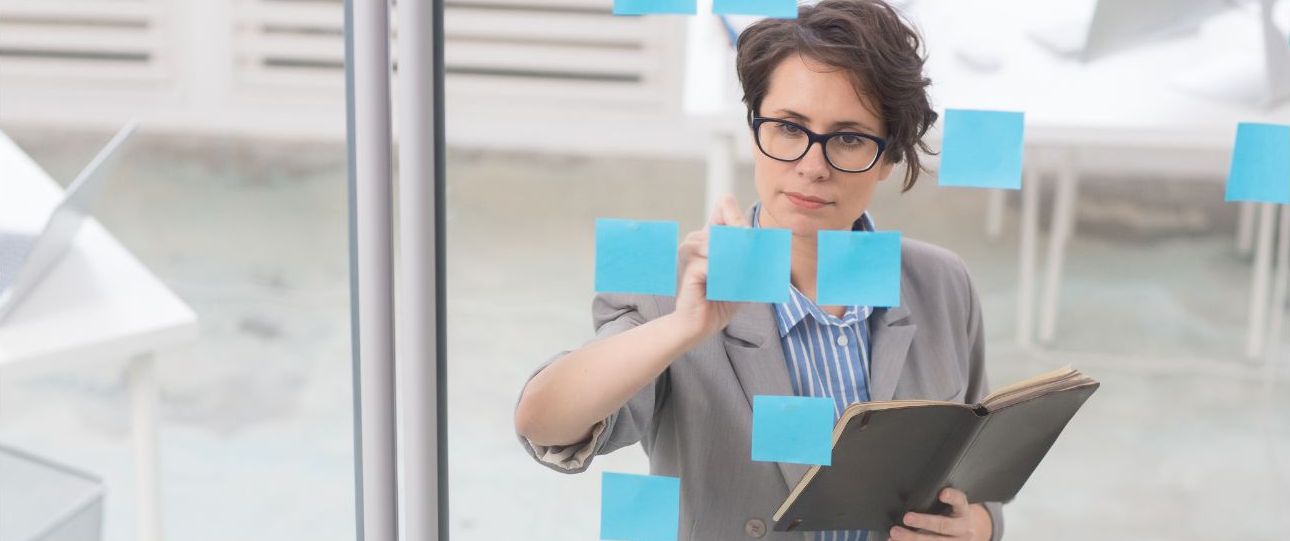
Recruitment and Retention of Participants
Recruiting a diverse set of participants and ensuring their sustained involvement is essential but can be time-consuming. Focusing on engagement, ease of use, and timely compensation helps in participant retention.
Recruitment tips:
- Targeted Outreach: Engage participants fitting specific demographics through targeted recruitment.
- Retention Incentives: Offering timely compensation or incentives can improve participant retention rates.
Recruiting and retaining a suitable participant pool is often one of the most time-intensive parts of speech data collection. Targeted Outreach to recruit participants meeting specific demographic criteria can improve recruitment efficiency. For example, using social media platforms and local community networks helps identify participants who match the age, gender, and language requirements for the project. Additionally, employing specialised recruitment platforms that focus on speech data projects can speed up the recruitment process by connecting with a pre-screened participant pool.
To ensure participants remain engaged throughout the project, Retention Incentives are effective. Compensation, feedback, and appreciation for participants’ contributions foster a sense of involvement, making participants more likely to complete the project. Timely compensation, transparent communication, and an easy-to-use submission platform can significantly improve participant retention, reducing dropout rates and ensuring a steady flow of data submissions.
Offering Ongoing Support is also beneficial. A dedicated support team that answers participant queries, assists with technical issues, and provides encouragement throughout the data collection process helps participants feel valued and motivated to continue. When participants are supported and appreciated, they are more likely to remain engaged, ultimately leading to a more consistent and reliable dataset.
Managing Quality Control and Data Validation
Quality control ensures that the collected data meets the required standards. Implementing checks for recording clarity, accuracy, and demographic compliance will help maintain data quality.
- Automated Validation Tools: Use software tools for preliminary data validation.
- Random Sampling: Conduct random sampling checks to ensure consistency in quality.
Maintaining the quality of the collected data is essential for the success of any speech dataset project. Implementing Automated Validation Tools can help teams maintain high data quality without extensive manual oversight. These tools automatically analyse submitted audio files for clarity, adherence to technical specifications, and demographic accuracy, ensuring only high-quality data enters the final dataset. Automated validation significantly reduces time spent on quality control while helping teams meet their quality standards.
Random Sampling is another effective quality control strategy. By reviewing random samples of the submitted data at regular intervals, the team can assess overall data quality, identify patterns of errors, and make any necessary adjustments. Sampling allows quality control to be conducted quickly and efficiently, particularly in large datasets where manual checking of every submission would be impractical.
Establishing Feedback Loops also enhances quality control. Providing participants with feedback on their recordings allows them to make immediate adjustments, ensuring data quality meets project standards. These feedback loops can be facilitated through automated messages in the data submission platform, reminding participants of specific quality requirements and helping to ensure consistent data quality.
Ensuring Data Privacy and Compliance
Data privacy and compliance regulations affect the speed and handling of data. Adhering to standards such as GDPR may require additional steps, like data anonymisation, to protect participant privacy.
Data privacy and compliance are critical considerations in speech data collection, especially when collecting data from diverse demographics across multiple regions. Compliance with data protection regulations, such as GDPR in the European Union, requires rigorous processes to ensure participant privacy. One common approach is Data Anonymisation, where identifiable information is removed from the data to protect participant privacy. This process is essential for regulatory compliance and safeguarding participants’ trust.
Secure Data Storage is another aspect of compliance, ensuring that collected data is stored in encrypted and protected environments. Access control measures, such as limiting access to only authorised team members, further enhance security. Regular audits and monitoring of storage systems are necessary to ensure data privacy remains intact throughout the project.
Providing Transparency and Informed Consent to participants is also vital for ethical and regulatory compliance. By clearly explaining the project’s purpose, data usage, and participants’ rights in the consent forms, teams can establish trust with participants and ensure their consent aligns with legal standards. Transparent communication about privacy measures and data handling also reassures participants, increasing their willingness to contribute.
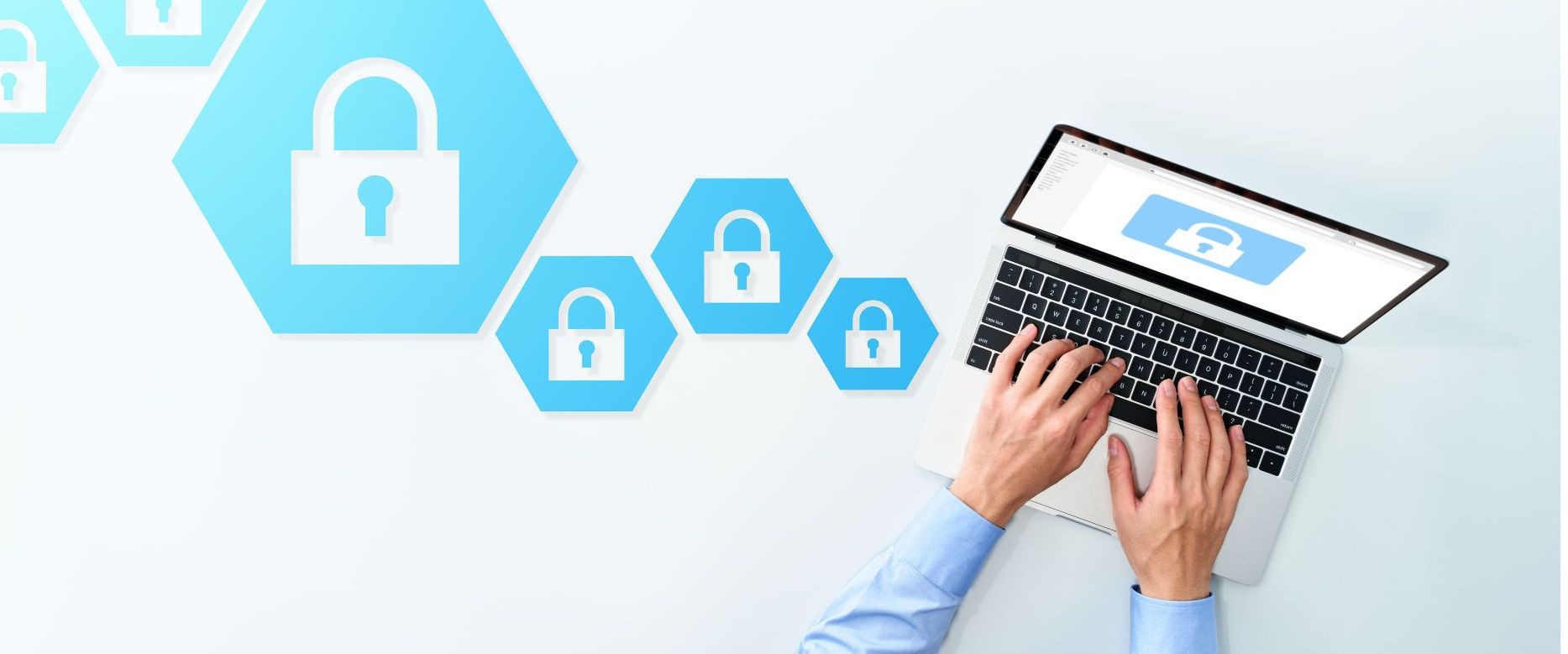
Challenges in Collecting Multilingual Data
Multilingual data projects typically require more time due to the complexity of managing multiple languages and dialects. Additionally, regional and cultural nuances necessitate specific recruitment and validation methods.
- Dialect-Specific Recruitment: Tailor recruitment to regional dialects or variations.
- Cultural Sensitivity: Ensure that prompts and content are culturally appropriate.
Multilingual data collection presents unique challenges, requiring careful planning and customised recruitment strategies. Dialect-Specific Recruitment is critical for projects involving multiple dialects within a single language. Recruiting participants who speak specific dialects and training them on the required prompts can be challenging, as these dialects may have varying linguistic features. Tailoring recruitment to each dialect ensures that the collected data accurately represents linguistic diversity.
Cultural Sensitivity is another vital consideration. The content of prompts and questions must be adapted to cultural norms, especially when working across multiple regions. Ensuring that prompts are neutral and culturally appropriate fosters a better participant experience and enhances data quality. Culturally sensitive recruitment and prompt design are essential for maintaining participant comfort and engagement, especially in sensitive regions or communities.
Multilingual projects also require In-Depth Validation Processes to maintain quality across languages. Quality checks must consider phonetic and linguistic differences across languages, ensuring each language’s unique features are accurately represented. Dedicated linguists and language specialists can play a crucial role in overseeing these checks, helping the project team maintain consistent data quality across multiple languages and dialects.
Use of Technology and Tools in Data Collection
Modern tools and platforms can simplify data collection. AI-driven tools aid in managing workflows, tracking participant progress, and automating quality checks.
- Speech Recognition Tools: Use ASR (automatic speech recognition) to filter and tag data efficiently.
- Project Management Platforms: Track milestones, participant submissions, and data quality.
Incorporating modern technology and tools into the data collection process enhances efficiency and quality. Speech Recognition Tools, such as ASR (automatic speech recognition), can be used for initial filtering and tagging of data. These tools help sort data by linguistic features, making it easier to identify and organise speech samples according to project needs. ASR tools are especially useful in large datasets, where manual sorting would be time-consuming and impractical.
Project Management Platforms are essential for organising data collection workflows. These platforms provide dashboards for tracking milestones, participant progress, and data quality metrics, enabling teams to monitor the project’s progress at a glance. Advanced project management tools also support team collaboration, allowing multiple stakeholders to work cohesively and make real-time updates on project developments.
In addition to ASR and project management platforms, Automated Quality Control Software plays a vital role in ensuring data accuracy. This software uses algorithms to scan audio files for sound quality, volume consistency, and background noise levels, providing instant feedback to participants. Automating these quality checks ensures that only high-quality data reaches the final dataset, minimising the need for time-intensive manual reviews and enhancing the overall efficiency of the project.
Key Tips for Efficient Speech Data Collection
- Set Clear Requirements: Define your project scope and requirements before starting to minimise changes mid-project.
- Select the Right Partner: Choose a data collection provider experienced in speech data projects to leverage their expertise.
- Use Automation: Automate routine checks and validation processes to reduce manual effort.
- Establish Milestones: Break down the project timeline into clear milestones for progress tracking.
- Prioritise Participant Experience: Making participation easy and rewarding encourages retention and consistent data quality.
Collecting a large dataset of speech data is an intricate process shaped by factors like project scope, participant demographics, and technical requirements. By understanding these influences, project managers, data scientists, and AI developers can estimate realistic timelines and navigate common challenges effectively. Whether managing a small dataset or a multilingual dataset spanning diverse demographics, clear planning and optimised workflows can make a substantial difference in efficiency.
Remember, the key to successful speech data collection lies in precise planning, selecting the right tools, and working with skilled partners. A well-structured timeline, backed by proactive risk management, not only shortens the project duration but also ensures the collected data meets quality and compliance standards.
Further Data Collection Resources
Data Collection – Wikipedia: This article discusses the process of data collection, including methods and factors affecting the duration, which are relevant to understanding the timeline for collecting speech data.
Way With Words – Speech Collection: Way With Words offers bespoke speech collection projects tailored to specific needs, ensuring high-quality datasets that complement freely available resources. Their services fill gaps that free data might not cover, providing a comprehensive solution for advanced AI projects.