Ethics in Speech Data Collection: Balancing Innovation and Responsibility
What are the Ethical Considerations in Speech Data Collection?
Speech data collection and speech data labelling is essential for advancing artificial intelligence and machine learning technologies. However, the rapid adoption of these technologies raises important ethical considerations. From ensuring privacy to fostering inclusivity, ethical data practices are fundamental to building trust and creating systems that serve society responsibly. This short guide addresses the question: What are the ethical considerations in speech data collection?
Common Questions:
- What are the primary ethical concerns when collecting speech data?
- How can organisations ensure compliance with ethical guidelines in data collection?
- What role do regulations play in safeguarding individuals during the data collection process?
This guide explores these and other questions by delving into the intersection of ethics, technology, and regulatory compliance, offering actionable insights for professionals navigating this critical field.
Key Speech Data Ethics Topics
Importance of Ethical Guidelines in Speech Data Collection
Ethical guidelines provide a foundation for responsible data practices. They ensure that individuals’ rights are respected, data is used fairly, and potential harms are mitigated. Organisations adhering to ethical guidelines can foster trust among stakeholders while minimising reputational risks.
- Transparency: Inform participants about how their data will be used.
- Informed Consent: Ensure all participants clearly understand the purpose of the data collection and its implications.
- Accountability: Establish mechanisms for reviewing data collection practices and addressing concerns.
Ethical guidelines serve as a compass for organisations, guiding how data is gathered, processed, and used responsibly. Beyond mitigating risks, they establish a culture of accountability and fairness that benefits both participants and stakeholders. Guidelines ensure that speech data collection aligns with societal values, legal frameworks, and business integrity.
One critical aspect of ethical guidelines is ensuring fairness in participant recruitment. For instance, selecting participants from diverse demographics prevents biased data collection that could skew the outcomes of AI systems. Ethical oversight ensures that datasets represent a wide range of accents, dialects, and socio-economic backgrounds, creating a foundation for unbiased AI development. This inclusivity fosters broader societal acceptance and trust in AI systems.
Moreover, ethical guidelines often extend to data storage and usage, emphasising the principle of data minimisation. Collecting only the data necessary for a specific purpose reduces risks associated with misuse or unauthorised access. By setting clear limits on data retention, organisations can further demonstrate their commitment to safeguarding participants’ information while complying with privacy laws.
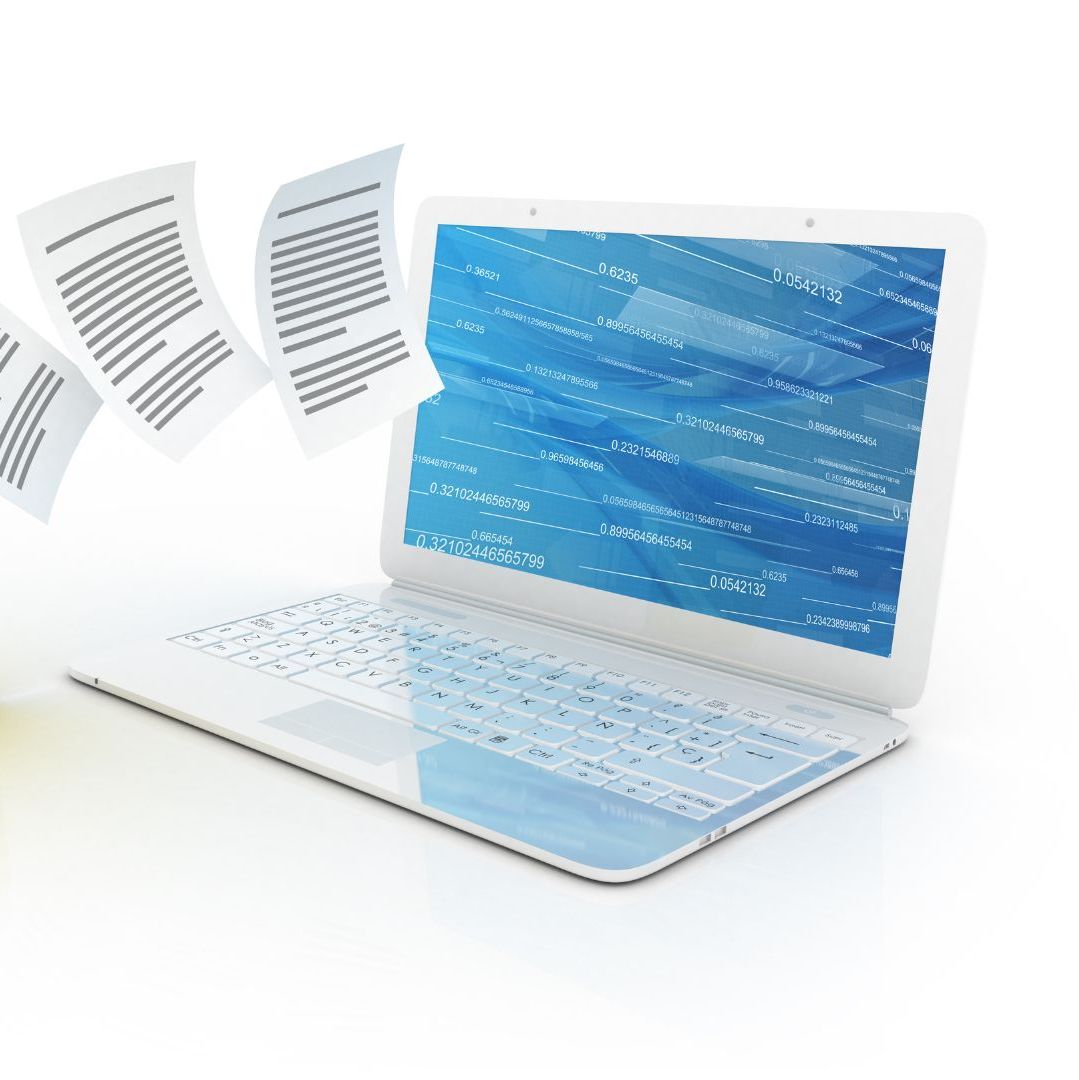
Ethical Challenges in AI and Machine Learning
AI systems trained on speech data can perpetuate biases if datasets are not representative or collected ethically. These biases can lead to discriminatory outcomes in applications like hiring tools, voice assistants, and customer service bots.
- Addressing bias requires intentional dataset design.
- Oversight by ethicists or committees can prevent harmful practices.
- Building diverse teams enhances the ethical rigor of projects.
AI and machine learning systems inherit biases from the datasets they are trained on, and speech data collection is no exception. A lack of representation in datasets can result in systems that perform poorly for underrepresented groups, perpetuating inequalities. For example, voice recognition tools often struggle with non-dominant accents, which could exclude users from accessing critical services.
Ethical challenges also arise when balancing commercial interests with fairness. Companies might prioritise efficiency over inclusivity, leading to the exclusion of nuanced speech patterns or languages that require greater resources to process. Addressing these challenges requires collaboration between technical teams, ethicists, and policymakers to ensure that commercial goals do not undermine societal values.
Another layer of complexity involves identifying unintentional biases during model training. Machine learning algorithms often rely on historical data, which may contain implicit societal biases. Developing tools for bias detection and correction during training can significantly improve the fairness and accuracy of AI systems. By proactively identifying and addressing such challenges, organisations can foster trust in AI technologies.
Regulatory Frameworks and Compliance
Global regulations like GDPR (General Data Protection Regulation) and CCPA (California Consumer Privacy Act) highlight the importance of ethical data handling. Compliance not only ensures legal adherence but also signals a commitment to responsible AI practices.
- GDPR Highlights: Data minimisation, purpose limitation, and the right to be forgotten.
- CCPA Highlights: Transparent data usage and opt-out options.
- Compliance frameworks must be integrated into every stage of speech data collection.
Compliance with regulatory frameworks like GDPR and CCPA is not just a legal obligation—it’s an ethical imperative. These laws emphasise transparency, giving individuals control over their data and how it’s used. Compliance frameworks require organisations to integrate ethical considerations into every step of the data lifecycle, from collection to deletion.
GDPR, for example, mandates that individuals must be informed about how their data is being processed and provides them with the right to withdraw consent at any time. This principle of informed consent ensures that participants retain agency over their personal information, fostering trust between organisations and the public. Similarly, GDPR’s data minimisation requirement ensures that only necessary data is collected, reducing the risks of misuse.
CCPA introduces additional layers of protection by granting individuals the right to opt out of data sales and request information on how their data is used. These rights highlight the growing recognition of data as a personal asset that must be safeguarded. Organisations that proactively integrate compliance measures demonstrate their commitment to ethical data practices, ultimately strengthening their reputations and fostering public trust.
Case Studies on Ethical Data Collection Practices
Examining real-world practices can provide valuable insights:
- Case Study 1: A technology firm implemented community consultations to design inclusive speech datasets, incorporating multiple dialects and accents.
- Case Study 2: A nonprofit utilised anonymised and consented data to develop educational tools without compromising privacy.
These examples illustrate the importance of balancing innovation with responsibility.
Real-world examples highlight the importance of ethical considerations in speech data collection. One illustrative case involved a technology firm partnering with local communities to build a dataset inclusive of indigenous languages. By working directly with native speakers and offering fair compensation, the company ensured that the dataset authentically represented these languages while respecting cultural nuances.
Another compelling example is a health-focused nonprofit that prioritised anonymisation in its dataset development. To protect participants’ identities, the organisation implemented advanced encryption techniques and separated personally identifiable information from speech data. This approach not only safeguarded privacy but also set a precedent for ethical practices in sensitive fields like healthcare.
These case studies underscore the value of collaborative and inclusive approaches. Whether through community engagement or stringent privacy safeguards, they highlight that ethical practices can coexist with technological advancement. Organisations can draw lessons from these examples to balance innovation with responsibility effectively.
Future Trends in Ethical AI
As AI technologies evolve, so too must the ethical considerations surrounding their development.
- Explainable AI (XAI): Ensures that systems provide transparent reasoning behind their outputs.
- Decentralised Data Systems: Empower individuals to control their data.
- Ethical AI will likely shift towards proactive policies, preventing harm before it occurs.
The ethical landscape of AI is evolving, with emerging trends pointing toward more proactive approaches. Explainable AI (XAI) is gaining traction as a way to enhance transparency. By designing models that clearly outline how decisions are made, organisations can address public concerns about the opacity of AI systems. This trend is particularly relevant in speech data applications, where outputs like transcriptions or voice commands have direct implications for users.
Decentralised data systems represent another transformative trend. By enabling individuals to control their own data through blockchain technology or decentralised frameworks, these systems reduce the risk of centralised breaches and misuse. They also align with privacy-first principles, empowering individuals to decide how their data is shared or monetised.
Looking ahead, ethical AI will increasingly focus on predictive harm prevention. Organisations are beginning to adopt proactive measures, such as scenario testing and ethical impact assessments, to identify potential risks before they manifest. These forward-thinking strategies ensure that ethical practices evolve alongside technological advancements, creating a more trustworthy AI ecosystem.
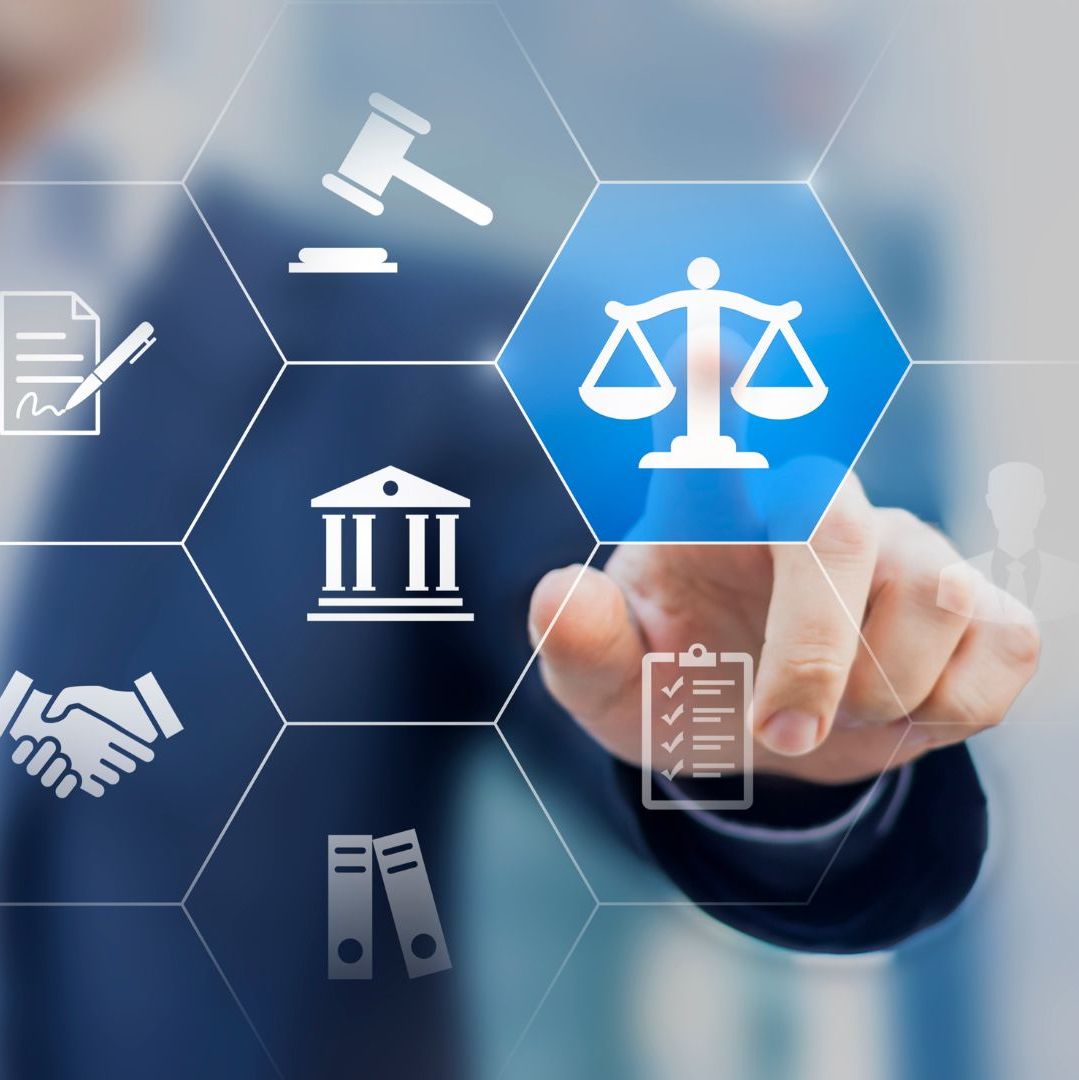
Privacy and Security Concerns in Speech Data
Privacy breaches are a significant risk in speech data collection. Measures to mitigate these risks include:
- End-to-end encryption during data storage and transmission.
- Regular audits to identify vulnerabilities.
- Minimising data retention periods to reduce exposure.
Privacy breaches can undermine public trust in AI systems and result in legal or financial repercussions for organisations. To mitigate these risks, robust security measures must be implemented at every stage of data handling. For example, encrypting data during both storage and transmission can significantly reduce the likelihood of unauthorised access.
Another key consideration is limiting access to sensitive data. By adopting role-based access controls, organisations can ensure that only authorised personnel can view or manipulate speech data. This principle of least privilege reduces the risk of accidental leaks or misuse within organisations.
Finally, organisations must prioritise secure data deletion practices. Retaining data unnecessarily increases the likelihood of breaches, particularly as cyber threats become more sophisticated. Implementing processes for secure data erasure demonstrates a commitment to privacy and aligns with legal mandates like GDPR, which emphasise the right to be forgotten.
Inclusivity and Diversity in Data Collection
Creating speech datasets that reflect the diversity of human language is critical for fair AI systems. This includes capturing underrepresented languages, dialects, and speaking styles.
- Collaborate with local communities to collect authentic data.
- Avoid over-reliance on commonly spoken languages to prevent systemic exclusion.
Inclusivity in speech data collection is not just a moral obligation—it directly impacts the effectiveness of AI systems. When datasets fail to include diverse languages, accents, or speaking styles, the resulting AI models may perform poorly for underrepresented groups. This can perpetuate systemic inequalities, especially in critical applications such as voice-activated healthcare or educational tools.
To ensure inclusivity, organisations must adopt deliberate strategies for dataset diversity. Collaborating with local communities to capture authentic speech samples is one effective approach. For example, partnering with linguistic experts or cultural advisors can help ensure that data collection methods are sensitive to regional nuances and cultural norms. Fair compensation for participants from underrepresented groups further supports equitable practices.
Additionally, maintaining linguistic and demographic balance in datasets is essential for mitigating bias. This requires ongoing evaluation of data collection processes and results. AI developers must regularly audit their datasets for gaps and take corrective action when disparities are identified. By embedding inclusivity into their workflows, organisations can create AI systems that cater to global audiences and reduce barriers to access.
The Role of Open-Source Data in Ethical Practices
Open-source datasets provide opportunities for collaboration and transparency but require careful ethical management.
- Advantages: Access for smaller organisations, fostering innovation.
- Challenges: Ensuring data quality and compliance with ethical standards.
Open-source datasets are a valuable resource for researchers and developers, but they present unique ethical challenges. While they promote collaboration and innovation, open datasets must be curated carefully to ensure compliance with ethical and legal standards. The absence of oversight in some open-source initiatives can lead to privacy breaches or biased data.
To address these issues, organisations contributing to open-source datasets should prioritise transparency in their processes. Documenting how data is collected, anonymised, and validated allows other stakeholders to assess its ethical soundness. For example, publishing detailed metadata about contributors, including linguistic and demographic characteristics, can help ensure that datasets are representative and useful for a variety of applications.
Another critical consideration is the licensing of open-source datasets. Ethical licenses that restrict the use of data for harmful purposes, such as surveillance or discriminatory practices, are becoming increasingly popular. These licenses empower contributors to protect the integrity of their data while enabling positive advancements in AI research.
The Cost of Ignoring Ethical Practices
Neglecting ethics in speech data collection can have significant repercussions:
- Legal penalties for non-compliance with data protection laws.
- Erosion of public trust and negative press.
- Financial losses from lawsuits or data breaches.
Neglecting ethical practices in speech data collection can have far-reaching consequences for organisations. Beyond immediate legal and financial penalties, the reputational damage from unethical practices can erode stakeholder trust and impact long-term success. For instance, data breaches exposing personal information often result in public backlash and decreased consumer confidence.
Legal non-compliance is a significant risk when ethical guidelines are overlooked. Regulations like GDPR impose hefty fines for violations, and high-profile cases have demonstrated the financial toll of failing to protect user data. Additionally, litigation arising from unethical practices can divert resources and attention from innovation, hindering an organisation’s competitive edge.
The societal implications of ignoring ethics are equally concerning. When speech data is used irresponsibly, it can exacerbate existing inequalities and marginalise vulnerable populations. Organisations that prioritise ethics can mitigate these risks while fostering trust and credibility in their products. Ultimately, the cost of ethical lapses far outweighs the investment required to implement responsible data practices.
The Need for Continuous Ethical Training
Organisations must invest in ongoing training to keep teams informed about emerging ethical challenges.
- Include ethics modules in employee onboarding and continuing education.
- Partner with experts to design effective training programs.
Ethical challenges in speech data collection are not static; they evolve alongside technological advancements. Continuous training is essential for keeping teams informed about emerging risks and best practices. This ensures that ethical considerations remain central to an organisation’s operations, from data collection to model deployment.
Effective training programs go beyond compliance checklists. They incorporate real-world scenarios and case studies to illustrate the complexities of ethical decision-making. For example, workshops on recognising implicit bias in datasets or responding to privacy concerns can equip teams with practical tools for addressing challenges. Interactive sessions encourage collaboration across departments, fostering a shared understanding of ethical principles.
Another vital component of training is the involvement of external experts. Partnering with ethicists, legal advisors, and industry leaders ensures that training materials are grounded in the latest research and regulatory developments. Organisations that invest in continuous learning not only enhance their internal capabilities but also demonstrate a commitment to upholding ethical standards in an ever-changing environment.
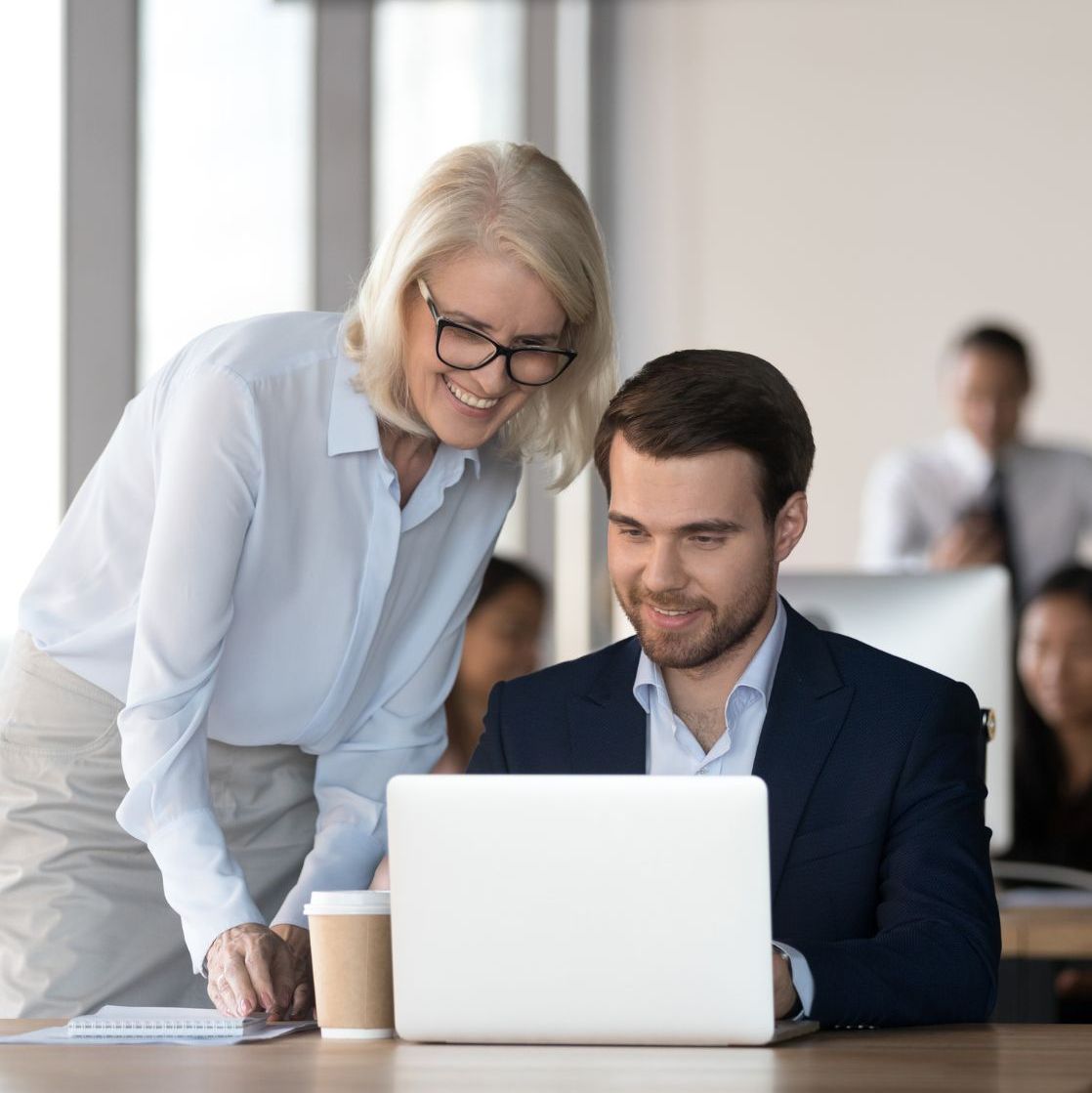
Key Tips for Addressing Ethical Considerations
- Establish Clear Ethical Policies: Define and implement policies aligned with global standards like GDPR.
- Engage Stakeholders: Involve ethicists, legal experts, and community representatives in decision-making.
- Leverage Technology Responsibly: Use encryption, anonymisation, and secure storage systems to protect data.
- Promote Diversity in Datasets: Ensure datasets represent a wide range of languages and demographics.
- Monitor and Review Practices: Regularly audit data collection and use processes to identify and correct issues.
Ethics in speech data collection is a dynamic field requiring constant vigilance, adaptability, and collaboration. By implementing clear guidelines, embracing inclusivity, and adhering to legal frameworks, organisations can foster responsible AI development while protecting individual rights.
The ten topics explored in this guide emphasize the importance of balancing technological advancement with ethical responsibility. The key piece of advice is this: Always prioritise transparency, inclusivity, and privacy in your speech data initiatives to build systems that benefit everyone.
Further Speech Collection Resources
Wikipedia: Ethics of Artificial Intelligence: This article discusses the ethical implications of artificial intelligence, including data collection practices and ethical considerations specific to speech data.
Way With Words: Speech Collection: Way With Words offers bespoke speech collection projects tailored to specific needs, ensuring high-quality datasets that complement freely available resources. Their services fill gaps that free data might not cover, providing a comprehensive solution for advanced AI projects.