Collecting Speech Data in Noisy Environments: Challenges & Solutions
The Challenges of Collecting Speech data in Noisy Places
Collecting speech data in noisy environments is a complex but necessary task for advancing speech recognition, AI development, and linguistic research. Whether the setting is a bustling urban street, a factory floor, or a crowded event, noise presents significant hurdles for capturing clear, usable speech samples. Organisations involved in developing automated speech recognition (ASR) systems, voice assistants, or audio analytics must understand these challenges and the technical requirements for effective speech data collection amidst noise.
Several questions frequently arise when considering speech data collection in noisy conditions:
- How can speech data be accurately captured when background noise levels are high?
- What technologies and techniques are essential to reduce noise interference during data collection?
- What are the best practices to ensure data quality and integrity despite environmental noise?
This guide explores the various challenges posed by noisy environments and offers practical solutions and requirements to overcome them. It is intended for AI developers, audio engineers, field researchers, and technology firms tasked with collecting speech data under difficult acoustic conditions. By understanding the requirements and employing appropriate noise reduction strategies, organisations can ensure the quality and reliability of their speech datasets, which in turn leads to better AI and machine learning models.
10 Key Thoughts on The Challenges of Collecting Speech data in Noisy Places
1. Challenges of Collecting Speech Data in Noisy Environments
Collecting speech data in noisy environments presents numerous challenges that can significantly affect the quality and usability of the recordings. One of the most significant obstacles is the signal-to-noise ratio (SNR), which measures the level of the desired speech signal relative to the background noise. When the noise level approaches or surpasses the speaker’s voice level, distinguishing speech becomes difficult for both human listeners and automatic speech recognition (ASR) systems. This can result in distorted or unintelligible recordings, which reduce the reliability of datasets used to train AI models.
Another challenge lies in the diversity and unpredictability of noise sources. Noise in real environments is rarely consistent—it can range from steady, low-frequency sounds such as air conditioning units or factory machinery to sudden, transient noises like sirens, vehicle horns, or crowd chatter. These variable noise types require different noise suppression strategies, making it difficult to develop a one-size-fits-all solution. Reverberation within enclosed spaces further complicates speech clarity, as reflected sound waves cause echoes and blurring of speech signals.
The physical behaviour of speakers during data collection can also affect recording quality. Changes in the speaker’s position relative to the microphone, variations in speaking volume, and movement introduce inconsistencies that can make post-processing more complex. In addition, equipment limitations play a role; not all microphones are designed to filter noise effectively, especially low-cost or generic devices.
Environmental factors such as weather conditions (wind, rain) and crowd density add further complexity, especially in outdoor or public space recordings. The cumulative effect of these challenges means that noisy environment speech data collection requires careful planning, specialised equipment, and well-trained personnel to maximise data integrity.
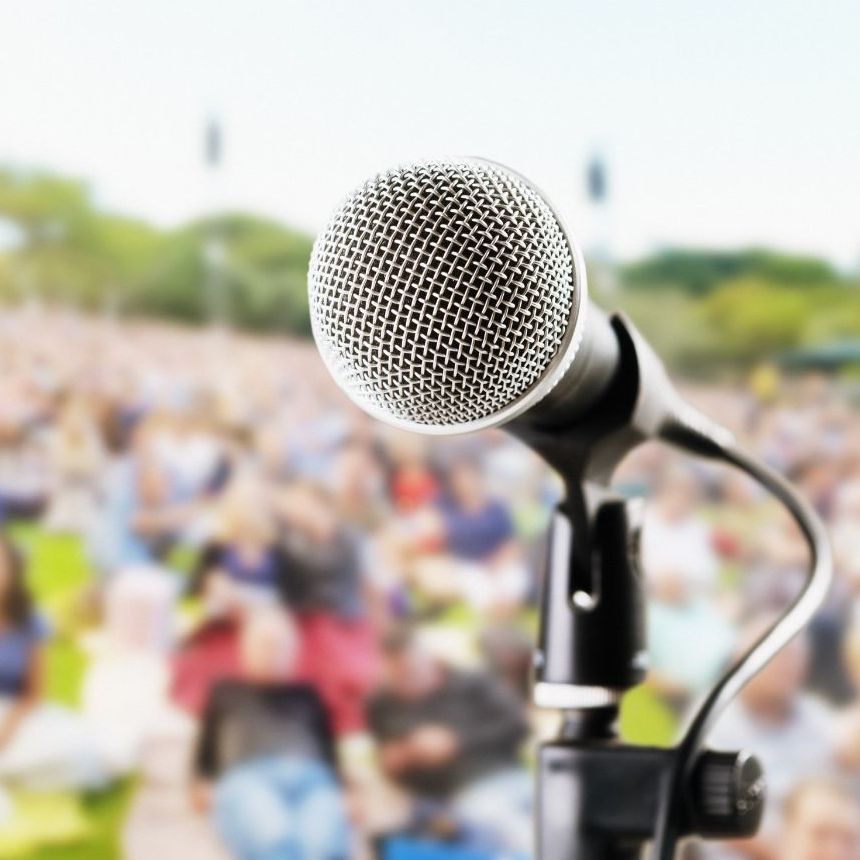
2. Techniques and Technologies for Noise Reduction
Reducing noise interference during speech data collection involves a combination of hardware solutions and software-based techniques. On the hardware side, directional microphones are a fundamental tool. These microphones are designed to pick up sound primarily from a specific direction (usually where the speaker is positioned) while suppressing sounds from other angles. Cardioid and shotgun microphones are common examples that help isolate speech from ambient noise.
Active noise-cancelling microphones take this a step further by using built-in processors to detect ambient sounds and produce an opposing sound wave that cancels out noise before the signal is even recorded. Beamforming microphone arrays combine input from multiple microphones and use algorithms to focus on the desired sound source, significantly improving signal clarity in noisy environments.
Portable acoustic treatments, such as sound shields or foam panels, can be used on-site to reduce reflections and reverberation, especially in semi-controlled indoor environments. These physical barriers prevent sound waves from bouncing around, which often degrades speech quality.
Once audio is captured, post-processing software plays a crucial role. Traditional signal processing techniques like spectral subtraction and Wiener filtering analyse the frequency spectrum of the recording to reduce noise components. More recently, deep learning approaches have been developed that train neural networks to differentiate speech from noise with higher precision, resulting in cleaner audio output.
Choosing the right combination of these tools depends on factors like the noise environment, budget constraints, and the desired data quality. Continuous advancements in AI-driven noise reduction are improving the ability to extract intelligible speech even from highly contaminated audio, opening new possibilities for collecting speech data in previously prohibitive settings.
3. Case Studies on Successful Data Collection in Noise
Real-world examples illustrate how organisations have effectively collected speech data despite noisy conditions. One notable case is the collection of urban voice assistant training data in major cities. Urban environments are characterised by diverse and unpredictable noise sources—traffic, construction, crowds, and weather elements. In these projects, data collection teams employed beamforming microphone arrays mounted on mobile rigs to spatially filter the speaker’s voice from surrounding noise.
Complementing this, manual quality checks ensured that only recordings meeting minimum intelligibility thresholds were used for training. The result was a rich dataset that reflected real-world conditions, improving voice assistant performance in busy public settings.
Industrial settings offer another interesting example. Factories and manufacturing plants generate constant machinery noise at high decibel levels. Here, workers wore noise-cancelling headsets equipped with high-quality microphones positioned close to the mouth, significantly reducing ambient noise pickup. Acoustic panels installed near recording stations helped minimise reverberation, while sessions were scheduled during quieter shifts when possible. Such careful environmental control combined with targeted equipment choice enabled the capture of clear speech samples necessary for worker safety systems and industrial voice commands.
In public event settings, such as conferences or live performances, recording clear speech is particularly challenging due to large crowds and variable acoustics. Multi-microphone setups, sometimes combined with directional lavalier microphones worn by speakers, were used to maximise signal capture. Advanced post-processing techniques helped separate speech from crowd noise, and multiple takes ensured data completeness. These case studies highlight the importance of a tailored approach that integrates technology, environment understanding, and process management to successfully collect speech data in noisy environments.
4. Best Practices for Handling Noise in Speech Data Collection
To effectively handle noise during speech data collection, several best practices have been established based on experience across diverse projects. First and foremost, conducting a thorough pre-collection environment assessment is essential. This involves measuring ambient noise levels, identifying dominant noise sources, and noting temporal noise variations. Such assessments allow for strategic microphone placement, selection of appropriate equipment, and scheduling to avoid peak noise times.
Using multiple microphones can improve overall data capture by providing redundant audio sources and different perspectives. For example, combining a close-talk microphone with ambient microphones can help isolate speech while capturing contextual sounds. Training speakers on proper microphone technique is equally important; participants should maintain a consistent distance and orientation to the microphone, avoiding sudden movements that introduce variability.
Real-time audio monitoring during recording sessions allows engineers to detect issues promptly and make necessary adjustments. This can be done using headphones and dedicated software that displays signal quality metrics such as SNR. Additionally, implementing rigorous quality control procedures, including both manual listening and automated speech quality algorithms, helps identify unusable recordings early, reducing time spent on post-processing.
Documentation of noise conditions and session notes supports transparency and reproducibility, enabling future users of the dataset to understand its limitations and strengths. Finally, fostering close communication between data collectors, speakers, and technical teams ensures smooth operation and quick resolution of problems.
Adopting these practices enhances data integrity and ensures the final speech dataset meets the quality standards required for effective machine learning and research.
5. Future Innovations in Noise-Cancelling Technologies
The future of speech data collection in noisy environments looks promising, driven by rapid advances in noise-cancelling technologies and artificial intelligence. AI-powered noise suppression techniques are increasingly able to distinguish between speech and complex noise patterns with remarkable accuracy. Unlike traditional filters, these models learn from vast datasets to dynamically adapt to new noise types and acoustic conditions, enabling clearer recordings even in highly challenging environments.
Smart microphones embedded with onboard processing chips can perform real-time noise cancellation and beamforming without relying on external hardware. This reduces latency and allows for portable, scalable solutions suited to diverse field conditions. Wearable recording devices are also evolving to provide consistent microphone placement close to the speaker’s mouth, reducing interference and improving voice capture reliability.
Multi-sensor fusion is another area gaining attention. Combining audio inputs with video or other sensor data can help isolate speech signals more effectively. For example, lip-reading algorithms integrated with audio analysis can enhance speech detection in noisy scenes.
Advancements in wireless technology and cloud processing mean that complex noise reduction algorithms can run remotely, offering real-time enhanced audio streams back to data collectors or end-users.
As these innovations mature, the barriers to collecting high-quality speech data in noisy environments will diminish, expanding opportunities for research and commercial applications. Organisations that stay informed and adopt these technologies early will gain a competitive edge in developing robust speech recognition systems capable of operating in a wide range of acoustic conditions.
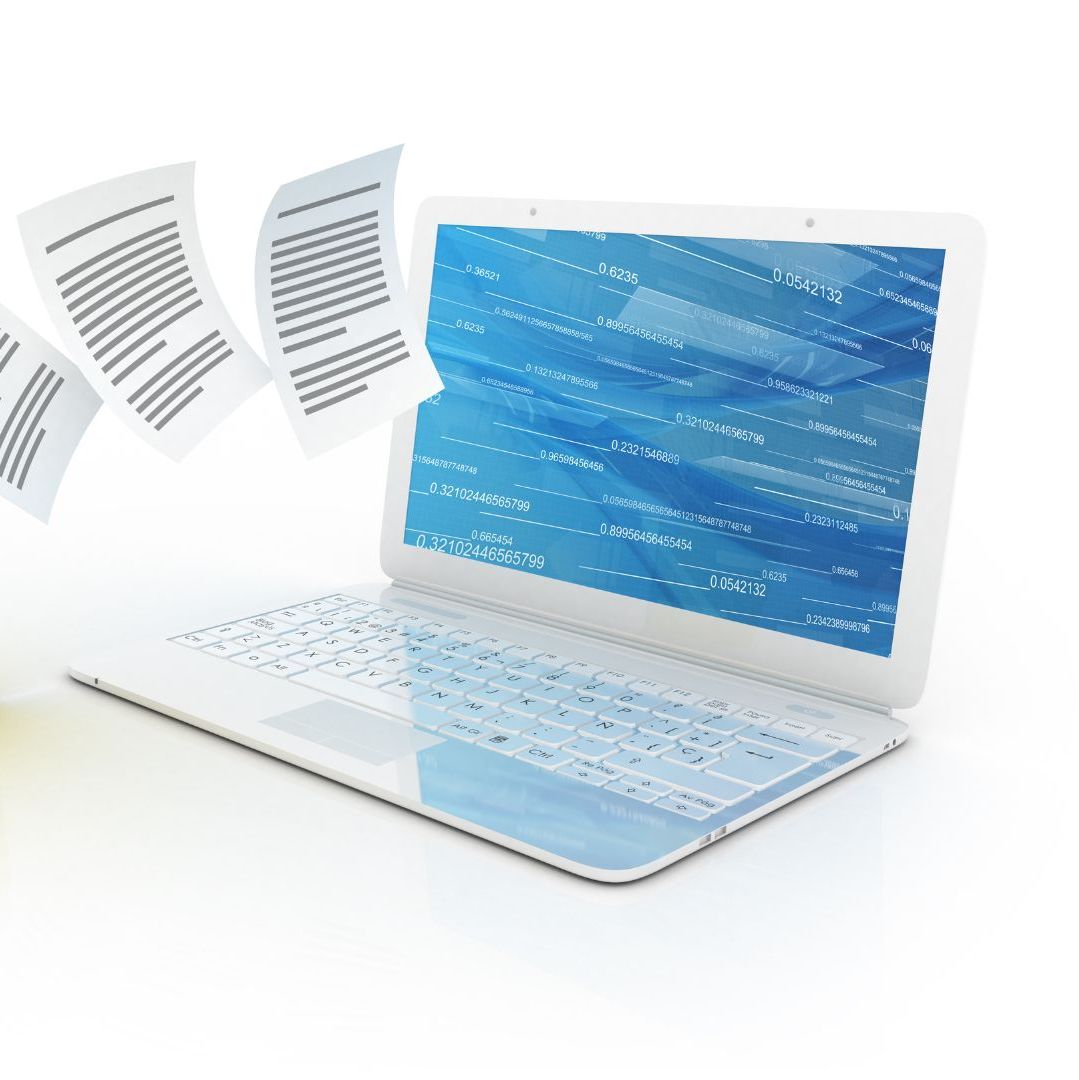
6. Importance of Signal-to-Noise Ratio (SNR) Monitoring
Signal-to-noise ratio (SNR) is a critical metric in the collection of speech data, particularly in noisy environments. It represents the ratio of the power of the speech signal to the power of background noise. Maintaining a high SNR ensures that speech recordings are clear and intelligible, which is fundamental for accurate transcription and subsequent model training.
During data collection, actively monitoring SNR allows engineers to identify when noise levels rise and adjust microphone placement or settings accordingly. For example, moving a microphone closer to the speaker or switching to a directional microphone can improve the SNR. In field research, portable SNR meters or software tools integrated with recording equipment provide real-time feedback on audio quality.
Low SNR recordings often require extensive post-processing to salvage usable speech, which increases time and cost. Additionally, ASR systems trained on low SNR data tend to perform poorly, resulting in higher word error rates and less reliable applications.
Best practice is to establish minimum SNR thresholds for acceptance of speech samples, ensuring that datasets meet quality standards. This proactive approach helps maintain consistency and reduces the burden on annotation teams and machine learning pipelines.
7. Impact of Noise on Speech Recognition Accuracy
Environmental noise has a pronounced effect on the accuracy of speech recognition systems. Studies indicate that ASR word error rates can increase dramatically as background noise intensifies. For instance, error rates can double when SNR falls below 10 decibels, which is common in many noisy real-world environments.
Noise introduces acoustic variability that models must learn to handle, making it harder to correctly interpret phonemes and words. If training data is dominated by clean speech, the system may struggle to generalise to noisy inputs, resulting in poor user experiences.
To mitigate this, collecting diverse datasets with realistic noise conditions is essential. It allows models to learn robust features that separate speech from noise. However, if noise overwhelms the speech signal, even advanced algorithms can fail.
Therefore, reducing noise at the data collection stage significantly improves recognition accuracy, user satisfaction, and the usability of voice-enabled technologies across different settings, from hands-free vehicle systems to mobile assistants.
8. Role of Data Annotation in Noisy Environments
Accurate data annotation is vital for building high-performance speech recognition systems. In noisy environments, transcription becomes more challenging because background sounds can obscure words or alter their perception. Annotators must often rely on contextual clues or listen multiple times to ensure correct labelling.
Hiring experienced annotators trained to work with noisy audio improves transcript quality. Alternatively, semi-automated transcription tools can assist by providing initial drafts, which human annotators then correct. This hybrid approach balances efficiency with accuracy.
Clear annotation guidelines that define how to handle unclear or overlapping speech help maintain consistency across annotators. Additionally, tagging noise events or marking uncertain transcriptions provides valuable metadata that can be used to train noise-robust models.
Without high-quality annotation, datasets from noisy environments risk propagating errors into the models, undermining their performance and reliability.
9. Ethical Considerations in Noisy Environment Data Collection
Collecting speech data in noisy, often public environments raises ethical questions related to privacy, consent, and data security. Individuals captured in recordings may be unaware that their speech or background conversations are being recorded, especially in busy public spaces.
Organisations must establish clear protocols for obtaining informed consent from participants, including explaining the purpose of the data collection and how recordings will be used. When recording in public, anonymisation techniques or explicit signage may be necessary to comply with local regulations.
Data security measures must be in place to protect recordings from unauthorised access or misuse. Transparency about data handling fosters trust and ensures compliance with legal frameworks such as GDPR in Europe.
Ethical diligence safeguards both participants and organisations, preserving the integrity of research and commercial applications.
10. Balancing Cost and Quality in Noise Handling
Handling noise in speech data collection often involves trade-offs between cost and quality. High-end directional microphones, acoustic treatments, and advanced noise reduction software can significantly increase project expenses. Conversely, skimping on these elements may lead to poor data quality, requiring costly re-collection or extensive post-processing.
A balanced approach requires assessing the intended use of the data, acceptable error rates, and budget constraints. For example, preliminary data for prototyping may tolerate some noise, whereas training data for commercial ASR systems demands high fidelity.
Strategic investment in the right combination of equipment, personnel training, and processing tools can maximise value. Additionally, iterative testing helps identify the minimum requirements to meet quality goals without overspending.
Ultimately, transparent cost-quality analysis supports informed decision-making and efficient resource allocation.
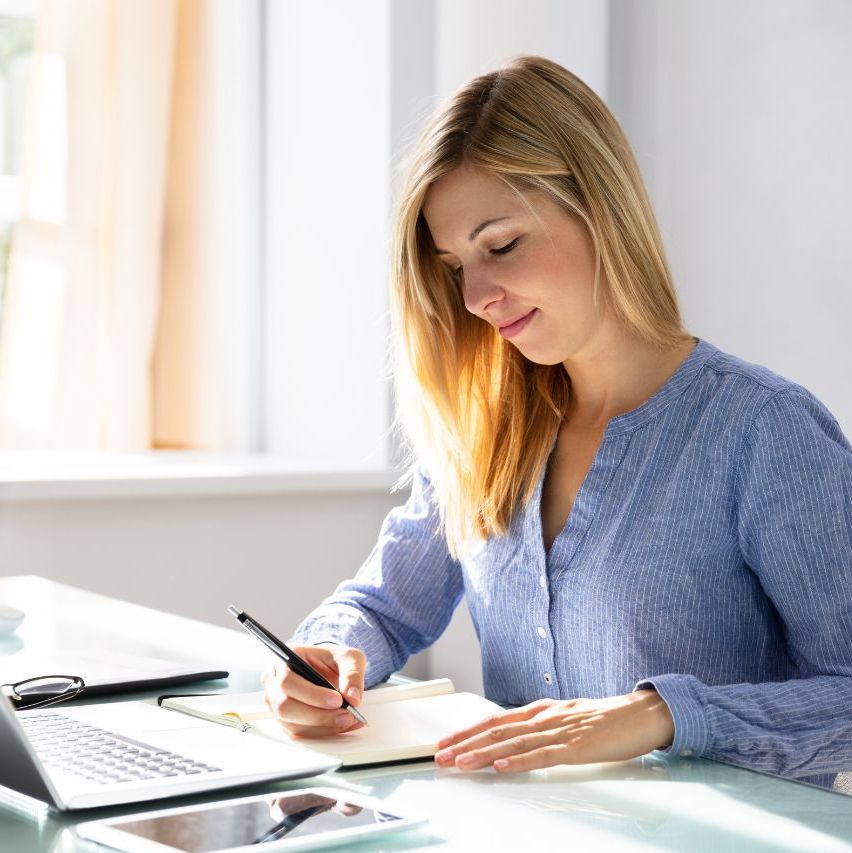
Key Tips for Collecting Speech Data in Noisy Environments
- Select the Right Microphone Type: Use directional or noise-cancelling microphones suited to the noise profile of the environment to enhance speech clarity.
- Conduct a Pre-Collection Noise Assessment: Measure noise levels and identify sources to plan equipment placement and timing effectively.
- Train Speakers in Microphone Usage: Ensure consistent distance and angle to microphones to reduce variability in recordings.
- Monitor Audio Quality in Real-Time: Use headphones and software tools to detect issues during recording sessions, enabling immediate adjustments.
- Implement Rigorous Quality Control: Employ both manual listening and automated algorithms post-collection to filter out unusable data and maintain dataset integrity.
Collecting speech data in noisy environments is a challenging but essential undertaking for organisations seeking to develop effective speech recognition systems and other voice technologies that function reliably in real-world conditions. The challenges posed by noisy environments are multifaceted: from low signal-to-noise ratios and diverse, unpredictable noise sources to reverberation and equipment limitations. Addressing these challenges requires a thorough understanding of the acoustic environment and careful planning before data collection begins.
The choice of techniques and technologies plays a critical role in overcoming noise-related issues. Directional and noise-cancelling microphones, beamforming arrays, and acoustic treatments help isolate speech at the hardware level, while post-processing algorithms—especially those powered by AI—further enhance audio clarity. Real-world case studies demonstrate that success hinges on tailoring solutions to specific noise conditions, whether in urban centres, factories, or public venues.
Implementing best practices, including environment assessments, speaker training, real-time monitoring, and rigorous quality control, is vital to maintaining data integrity. The importance of monitoring signal-to-noise ratio throughout the process cannot be overstated, as this metric directly influences transcription accuracy and the performance of trained models.
Looking ahead, exciting innovations in AI-driven noise suppression, smart microphones, and sensor fusion promise to make speech data collection in noisy settings more accessible and reliable. However, practitioners must also carefully consider ethical issues, including consent and privacy, when recording in public or sensitive environments.
A key piece of advice for anyone undertaking speech data collection in noisy environments is to prioritise achieving the clearest possible speech capture at the source. Investing effort and resources into noise reduction at the point of recording reduces the need for costly and complex post-processing and ultimately leads to higher quality datasets that improve machine learning outcomes.
By combining well-informed strategies, appropriate technology, and thorough quality assurance, organisations can confidently gather valuable speech data that accurately reflects the acoustic realities their systems must navigate.
Further Speech Data Resources
Wikipedia: Noise Reduction – This article provides an overview of noise reduction techniques and technologies, essential for understanding requirements for collecting speech data in noisy environments. It covers foundational concepts, hardware solutions, and signal processing methods that form the backbone of effective noise handling strategies.
Featured Transcription Solution: Way With Words: Speech Collection – Way With Words excels in collecting speech data from noisy environments, employing advanced noise-cancelling technologies and expert transcription services to ensure data integrity and quality. Their solutions are tailored to challenging acoustic conditions, providing accurate and reliable speech datasets that support high-performance AI applications.