How A Speech To Text Dataset Can Revolutionise Your Speech Recognition Technology
A Speech To Text Dataset Can Be Revolutionary To The Development Of Your Speech Recognition Technology.
A speech to text dataset is critical in the development of speech recognition technology. Speech recognition technology has come a long way over the past decade, thanks in large part to the availability of high-quality speech to text datasets that enable machine learning algorithms to accurately transcribe human speech. These datasets, which contain thousands or even millions of hours of recorded speech, are essential for training speech recognition models and enabling them to accurately transcribe speech in a wide range of languages, accents, and dialects.
Here, we will explore the key features of a popular speech to text dataset and how they can be used to train speech recognition models. We’ll also discuss some of the challenges associated with building and using these datasets and provide insights into best practices for working with them.
Benefits Of Using Speech To Text Datasets
Improved Accuracy
One of the most significant benefits of using speech-to-text datasets is that they can improve the accuracy of speech recognition models. These datasets enable machine learning algorithms to recognise and transcribe speech with greater precision and accuracy, resulting in more reliable and effective speech recognition applications.
Better Training
Speech to text datasets provide a valuable resource for training machine learning models to recognise and transcribe human speech accurately. By using these datasets, researchers can train models on a diverse range of speech samples, including different accents, dialects, and speech patterns. This diversity helps to ensure that speech recognition models are effective across a broad range of contexts and situations, making them more useful for practical applications.
Faster Development
Another benefit of using speech to text datasets is that they can speed up the development process for speech recognition applications. By providing a pre-existing corpus of speech samples, researchers can quickly and easily train machine learning models, reducing the time and resources required for model development. This allows companies to bring speech recognition applications to market more quickly, giving them a competitive advantage in the rapidly evolving speech technology market.
Increased Accessibility
Speech to text datasets can also help to increase accessibility for people with speech disabilities or impairments. By providing accurate and reliable speech recognition, these datasets enable individuals with speech difficulties to communicate more effectively with others, enhancing their quality of life and reducing the impact of their disability.
Enhanced User Experience
Speech to text datasets can also enhance the user experience for speech recognition applications. By providing accurate and reliable transcriptions of spoken language, these datasets can improve the speed and efficiency of speech-based interactions, making them more convenient and user-friendly. This enhanced user experience can be particularly beneficial in applications such as virtual assistants, where the speed and accuracy of speech recognition are critical.
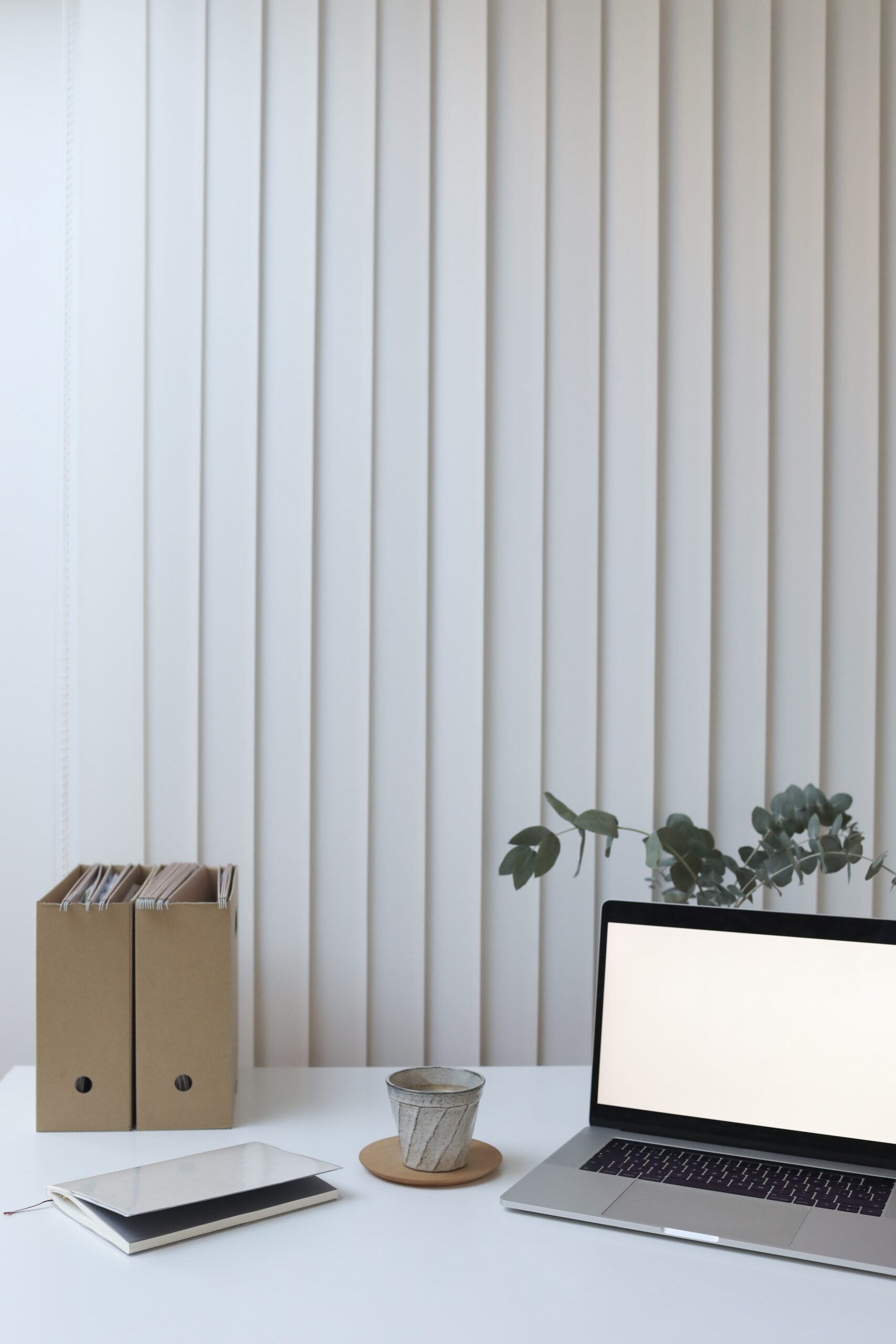
Challenges of Building and Using Speech To Text Datasets
Speech to text (STT) datasets have become an essential resource for developing accurate and reliable speech recognition models. However, building and using these datasets is not without its challenges. In this blog post, we will explore some of the challenges associated with building and using speech to text datasets and discuss how researchers and developers can address these challenges.
- Data Collection
One of the most significant challenges associated with building speech to text datasets is collecting data. Collecting a large and diverse corpus of speech samples can be time-consuming and costly. It can also be challenging to obtain high-quality data that accurately reflects the speech patterns and language usage of the target population. To overcome these challenges, researchers may need to employ a range of data collection methods, including crowdsourcing and data augmentation techniques. At Way With Words we understand the importance of diverse data and have refined the data collection process to collect data suited to any diversity needs.
Creating meaningful data is incredibly important to the development of speech recognition technology (SRT) as well. Using a speech collection dataset that is rich with metadata is advantageous to any business using SRT for customer services.
- Data Processing
Once data has been collected, it must be preprocessed before it can be used to train machine learning models. Preprocessing involves a range of tasks, including data cleaning, normalisation, and feature extraction. This process can be time-consuming and may require specialised skills and expertise. Preparation of the data is a vital step in ensuring that the data collected will have a meaningful impact on SRT development.
- Data Annotation
Data annotation is another significant challenge associated with building and using speech-to-text datasets. Annotation involves manually labelling each speech sample with its corresponding text transcription. This process can be time-consuming and requires significant human resources. Furthermore, the quality of the annotations can have a significant impact on the accuracy and reliability of the resulting speech recognition model.
- Data Bias
Another challenge associated with speech to text datasets is data bias. Data bias can occur when the dataset is not diverse enough or does not accurately represent the language usage and speech patterns of the target population. This can lead to biased and inaccurate speech recognition models, which can have serious consequences for the end-users.
- Privacy and Security
Finally, privacy and security concerns are another challenge associated with building and using speech-to-text datasets. Collecting and storing speech samples from individuals can raise privacy concerns, and companies must ensure that they obtain user consent and follow best practices for data collection, storage, and handling to protect user privacy.
Best Practices for Working with Speech To Text Datasets
To address the challenges associated with building and using speech-to-text datasets, speech recognition companies should follow best practices, including:
Ensuring data quality: Companies should take steps to ensure the quality of their speech-to-text datasets, including implementing quality control measures and using established quality metrics.
Mitigating data bias: Companies should aim to source diverse datasets and use techniques such as data augmentation to address bias in their speech recognition models.
Respecting user privacy: Companies should ensure that they obtain user consent and follow best practices for data collection, storage, and handling to protect user privacy.
Collaboration: Collaboration between different companies and research institutions can help to improve the quality and diversity of speech-to-text datasets, leading to more accurate and effective speech recognition models.
Regular updates: Speech recognition models and speech-to-text datasets should be regularly updated to reflect changes in language usage, accent and dialect shifts, and changes in technology.
Continual monitoring: Continual monitoring of speech-to-text datasets and speech recognition models is essential to identify and address data quality issues and ensure ongoing accuracy.
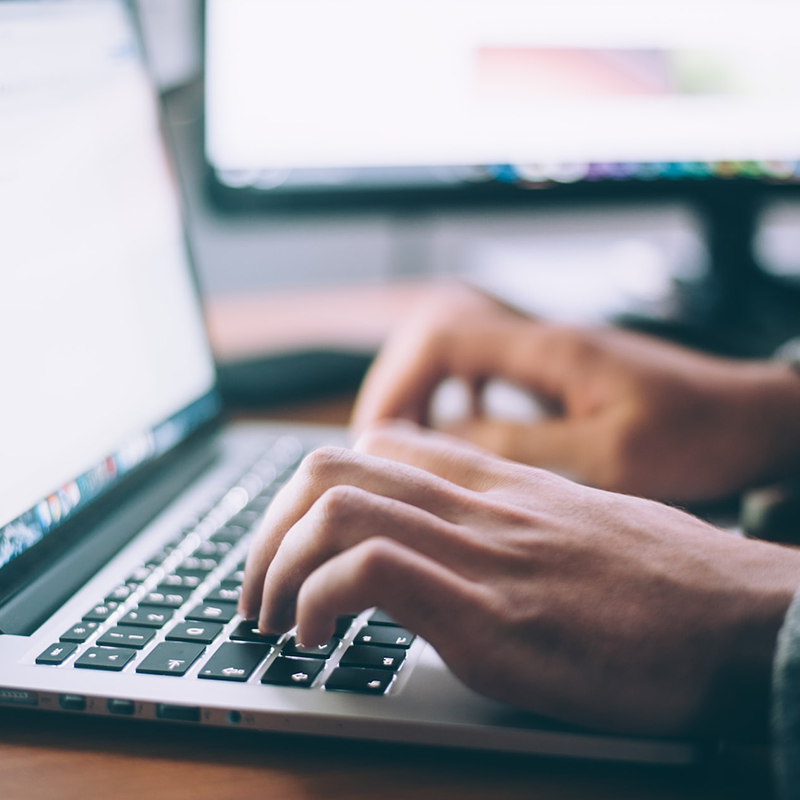
Speech to text datsets are an essential resource for speech recognition companies, enabling the development of accurate and effective speech recognition models. While there are challenges associated with building and using these datasets, following best practices can help to ensure high-quality, diverse datasets that accurately reflect the needs of the population as a whole. These are the pillars to successful speech to text datasets and here at Way With Words we take these pillars very seriously. We are proud to present our own speech collection dataset, contact us today to find out more about how this dataset can set your speech recognition technology apart from the rest.
Additional Services
Machine Transcription and the Role of The Transcriber
About Captioning
Perfectly synched 99%+ accurate closed captions for broadcast-quality video.
Machine Transcription Polishing
For users of machine transcription that require polished machine transcripts.