Annotating Data Meaning – Why Is It Important?
Annotating Data Meaning And its Importance in The Field of Data Science
The annotating data meaning can be complex and difficult to understand. Data annotation is a crucial process in data science that involves adding relevant information or metadata to raw data to make it easier to understand and analyse. This process is particularly important in the development of machine learning algorithms, which rely heavily on annotated data to learn and make predictions. We will provide an overview of what annotating data means, the different types of annotations, and how to effectively annotate data. We will also explore the challenges and common errors that transcription clients may encounter when annotating data, including ethical considerations that should be addressed.
Overview of Annotating Data
Annotating data involves adding metadata or additional information to raw data to enhance its usefulness. The goal of annotating data is to make it easier to understand and analyse, especially in machine learning applications. The process of data annotation involves a human annotator who reads and interprets data, adding information based on their knowledge and expertise. The added information could be in the form of labels, tags, descriptions, or comments.
Types of Annotations
There are several types of annotations used in data science, depending on the type of data being annotated. Some of the common types of annotations include:
Text Annotation: This type of annotation involves adding metadata to text data, such as natural language text, tweets, and comments. Text annotation is used in applications such as sentiment analysis, named entity recognition, and topic modelling.
Audio Annotation: Audio annotation involves adding metadata to audio data, such as speech, music, and sound effects. Audio annotation is used in applications such as speech recognition, speaker identification, and sound event detection.
Image Annotation: Image annotation involves adding metadata to image data, such as object detection, object recognition, and segmentation. Image annotation is used in applications such as autonomous vehicles, face recognition, and medical image analysis.
Video Annotation: Video annotation involves adding metadata to video data, such as object tracking, action recognition, and scene segmentation. Video annotation is used in applications such as surveillance, video indexing, and augmented reality.
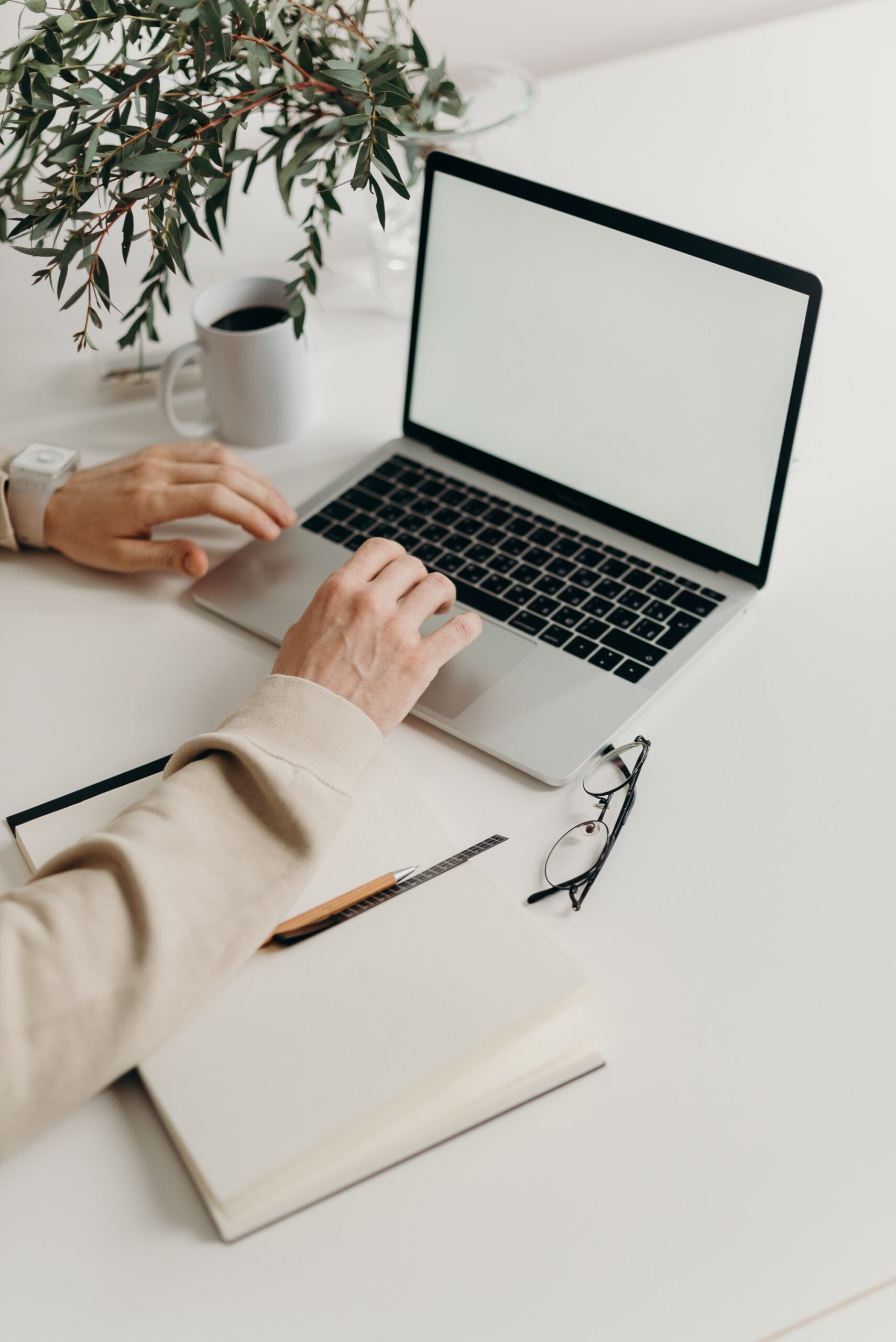
Effective Annotation Techniques
Effective annotation techniques involve a well-defined and systematic process that ensures accurate and consistent annotations. Here are some tips for effective annotation:
Define Clear Guidelines: Before starting the annotation process, it is essential to define clear guidelines that provide instructions on how to annotate the data. The guidelines should include information on what to annotate, how to annotate it, and how to deal with ambiguous cases.
Ensure Consistency: Consistency is crucial in annotation, as it ensures that the annotations are accurate and reliable. To ensure consistency, it is important to have multiple annotators work on the same data, and to have a system in place to resolve disagreements.
Provide Feedback: Providing feedback to annotators is important, as it helps them learn and improve their annotation skills. Feedback could be in the form of quality metrics, such as precision and recall, or by providing annotated examples.
Challenges and Common Errors in Annotating Data
There are several challenges and common errors that transcription clients may encounter when annotating data. Some of these include:
Ambiguity: Data annotation can be challenging when the data is ambiguous, making it difficult to determine the correct annotation. Ambiguity can arise in cases where the data is subjective, such as sentiment analysis or tone of voice.
Bias: Annotators may have their biases, which can affect the quality and accuracy of the annotations. Bias can arise from personal beliefs, culture, or background.
Inconsistency: Inconsistency in annotation can arise from differences in interpretation, lack of guidelines, or lack of proper training. Inconsistent annotation can lead to inaccurate and unreliable results.
Ethical Considerations in Annotating Data
Data annotation can raise ethical concerns, especially when dealing with sensitive or personal data. Some ethical considerations that transcription clients should be aware of include:
Privacy: Annotating data that contains personal information can be a breach of privacy. Annotators should be trained on how to handle personal data, such as anonymising it or deleting it after use.
Consent: Annotating data without consent can also be a breach of ethical principles. Clients should ensure that they have obtained consent from data subjects before annotating their data.
Fairness: Annotators should be aware of potential biases in the data, and take steps to avoid perpetuating them. This is particularly important in applications such as facial recognition, where biased data can lead to unfair outcomes.
Examples of Machine Learning Algorithms that Rely on Annotated Data
There are several machine learning algorithms that rely heavily on annotated data, such as:
Image Recognition: Image recognition algorithms rely on annotated image data to learn and make accurate predictions. For example, an image recognition algorithm used in autonomous vehicles requires annotated data on objects such as pedestrians, cars, and traffic signs.
Speech Recognition: Speech recognition algorithms rely on annotated speech data to learn and accurately transcribe spoken words. Annotated speech data can include information on the speaker, language, and context.
Natural Language Processing: Natural language processing algorithms rely on annotated text data to learn and accurately process natural language. Annotated text data can include information on part of speech, named entities, and sentiment.
Impact of Accurate Annotations on Machine Learning Models
The quality and accuracy of annotations can have a significant impact on the performance of machine learning models. Accurate annotations help machine learning algorithms learn patterns and make accurate predictions. On the other hand, inaccurate or inconsistent annotations can lead to unreliable results and decreased performance.
For example, if an image recognition algorithm is trained on annotated data with incorrect labels, it will make inaccurate predictions when presented with new data. Similarly, if a speech recognition algorithm is trained on annotated data with incorrect transcriptions, it will produce inaccurate transcriptions when presented with new speech data.
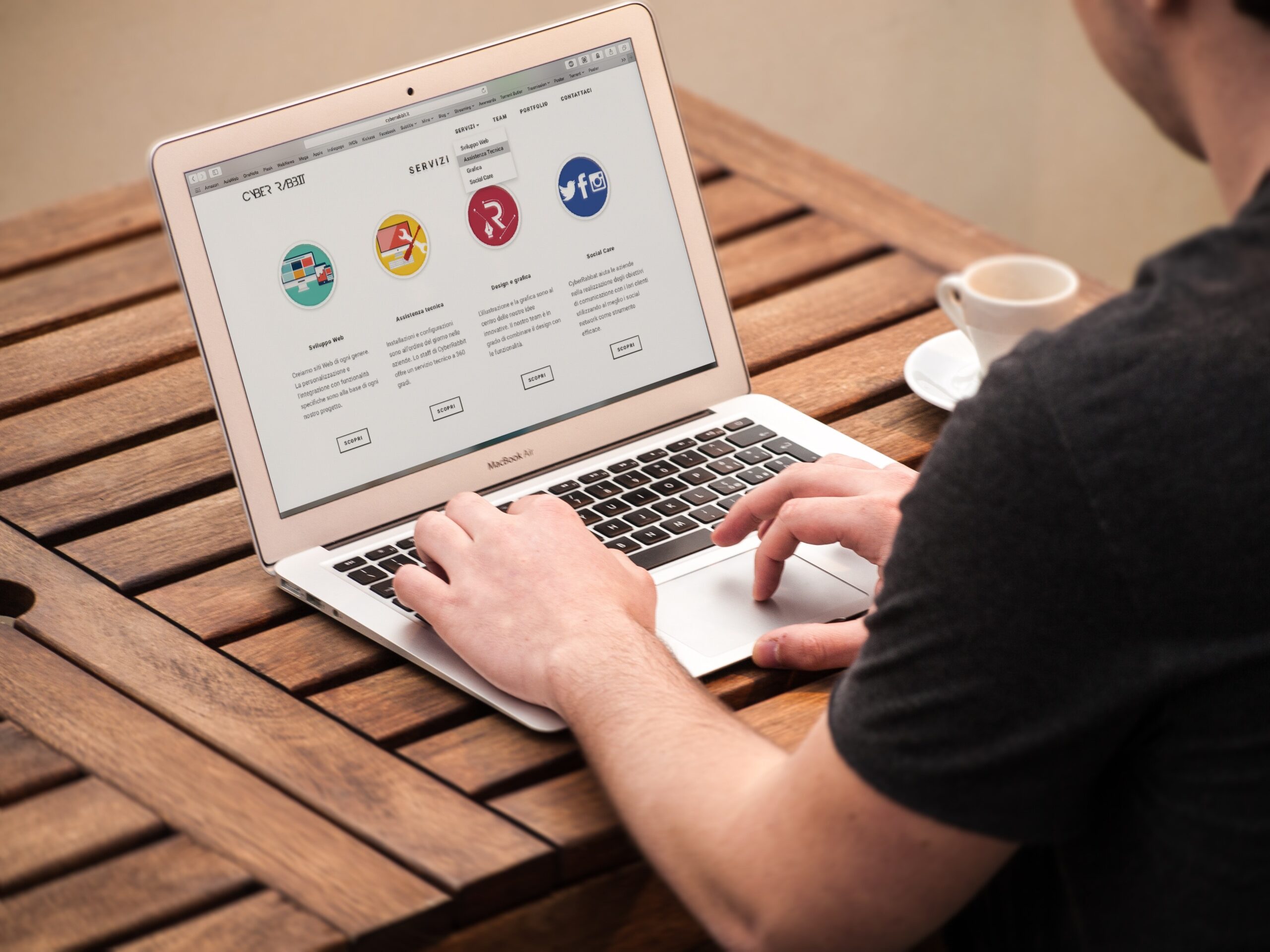
Data annotation is a crucial process in data science that involves adding relevant information or metadata to raw data to make it easier to understand and analyse. Effective annotation techniques involve a well-defined and systematic process that ensures accurate and consistent annotations. There are several challenges and common errors that transcription clients may encounter when annotating data, including ethical considerations that should be addressed. Accurate annotations are crucial for the performance of machine learning models, and clients should take steps to ensure the quality and accuracy of their annotations.
Additional Services
About Captioning
Perfectly synched 99%+ accurate closed captions for broadcast-quality video.
Machine Transcription Polishing
For users of machine transcription that require polished machine transcripts.