The Future of Automatic Speech Recognition Datasets
This is Why Automatic Speech Recognition Datasets Are The Future
Automatic Speech Recognition datasets have come a long way in recent years. Thanks to advancements in machine learning and the availability of vast datasets, automatic speech recognition (ASR) technology has become more accurate, faster, and more affordable. With the increasing demand for speech recognition applications in various industries, including customer service, healthcare, and education, the field of ASR is experiencing significant growth. In this blog post, we will discuss some emerging trends and technologies in automatic speech recognition datasets and how they may impact the field in the future.
Transfer Learning
One of the most promising developments in ASR is transfer learning. Transfer learning is a machine learning technique where a model trained on one task is re-purposed to another related task. In the context of ASR, transfer learning can be used to train models on a small amount of data and then fine-tune the model on a larger dataset. This approach can significantly reduce the amount of data required to train a model, making it more cost-effective and time-efficient.
One example of a transfer learning approach is the use of pre-trained models like BERT (Bidirectional Encoder Representations from Transformers) and GPT (Generative Pre-trained Transformer). These models are pre-trained on large text datasets and can be fine-tuned on speech datasets to improve ASR accuracy. By leveraging pre-trained models, ASR developers can reduce the amount of time and resources required to develop a new ASR system significantly.
Unsupervised Learning
Another emerging trend in ASR is the use of unsupervised learning. Unsupervised learning is a machine learning technique where a model learns patterns in data without explicit supervision. In the context of ASR, unsupervised learning can be used to train models on large amounts of unlabelled speech data, which can then be used to improve the accuracy of existing ASR systems.
One example of unsupervised learning in ASR is the use of contrastive predictive coding (CPC). CPC is a technique that trains a model to predict the next audio frame in a sequence. By doing so, the model learns to represent the audio sequence in a way that captures its underlying structure. CPC has shown promising results in improving the accuracy of ASR systems on low-resource languages, where labelled speech datasets are scarce.
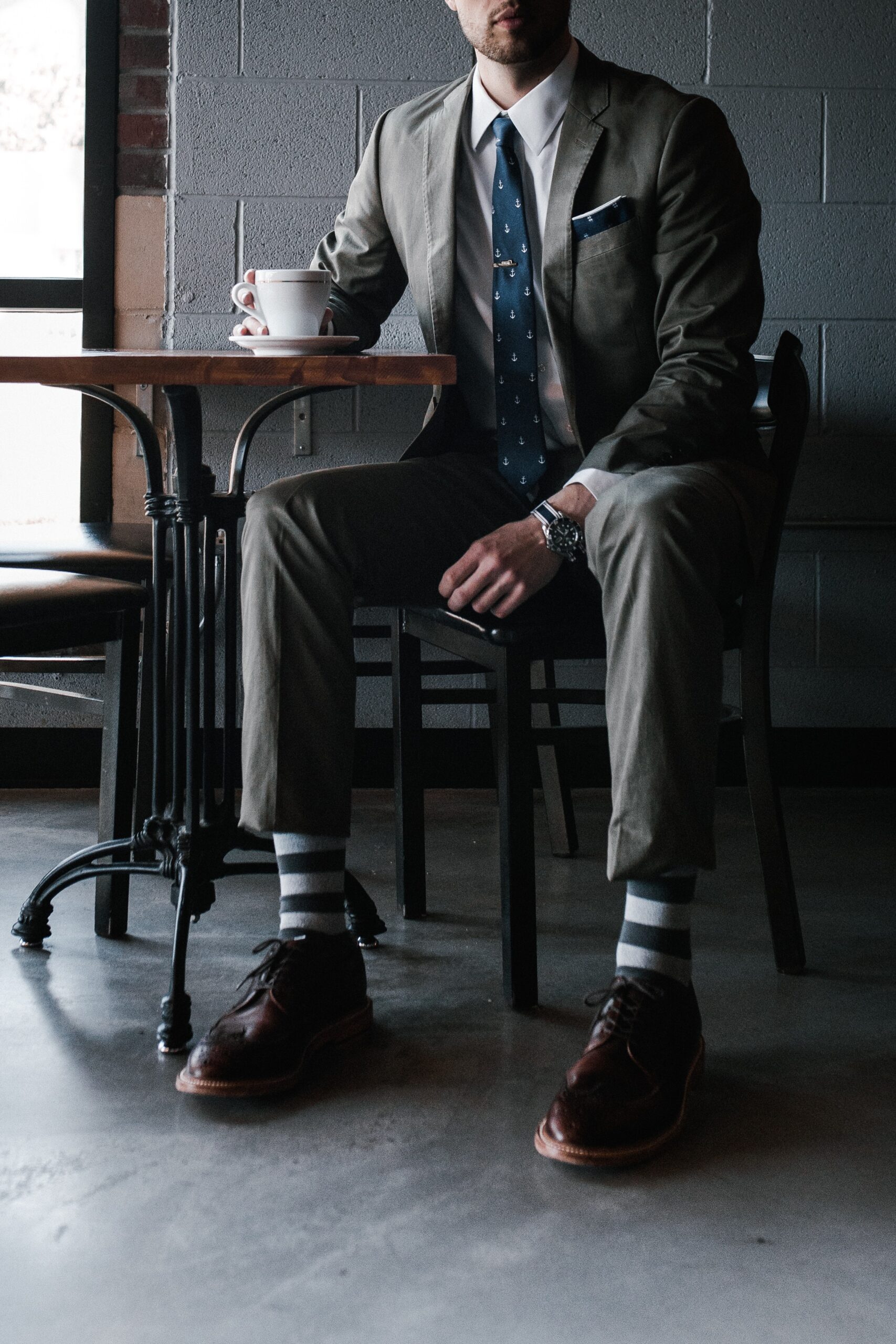
Use of Synthetic Data
The use of synthetic data is another emerging trend in ASR. Synthetic data refers to data that is generated by a computer program rather than collected from the real world. In the context of ASR, synthetic data can be used to augment existing speech datasets or to create new datasets for specific tasks.
One example of synthetic data in ASR is the Way With Words speech collection dataset. This dataset contains 200 hours of speech data generated using real-world scenarios. The dataset was designed to provide high-quality speech data for ASR training and evaluation. By using synthetic data, ASR developers can create large, diverse datasets that are tailored to their specific needs, without the cost and time required to collect real-world data.
Impact on the Future of ASR
The emerging trends and technologies in ASR datasets have significant implications for the future of the field. Transfer learning, unsupervised learning, and the use of synthetic data can all contribute to improving the accuracy and efficiency of ASR systems, making them more accessible to a wider range of industries and applications.
For example, transfer learning can enable the development of ASR systems for low-resource languages, where labelled speech datasets are scarce. By leveraging pre-trained models and fine-tuning on smaller datasets, ASR developers can create more accurate and affordable systems for these languages.
Similarly, unsupervised learning can help improve the accuracy of ASR systems on a broader range of speech data, including non-native accents and dialects. By training models on large amounts of unlabelled speech data, ASR systems can learn to recognise patterns in speech that are not present in labelled datasets. This can improve the robustness and accuracy of ASR systems, making them more useful in real-world scenarios.
Finally, the use of synthetic data can help overcome the limitations of collecting and labelling speech data. In industries where data privacy is a concern, synthetic data can be used to generate realistic speech data without compromising the privacy of individuals. Additionally, synthetic data can be used to create speech datasets for niche applications that would be difficult or expensive to collect in the real world.
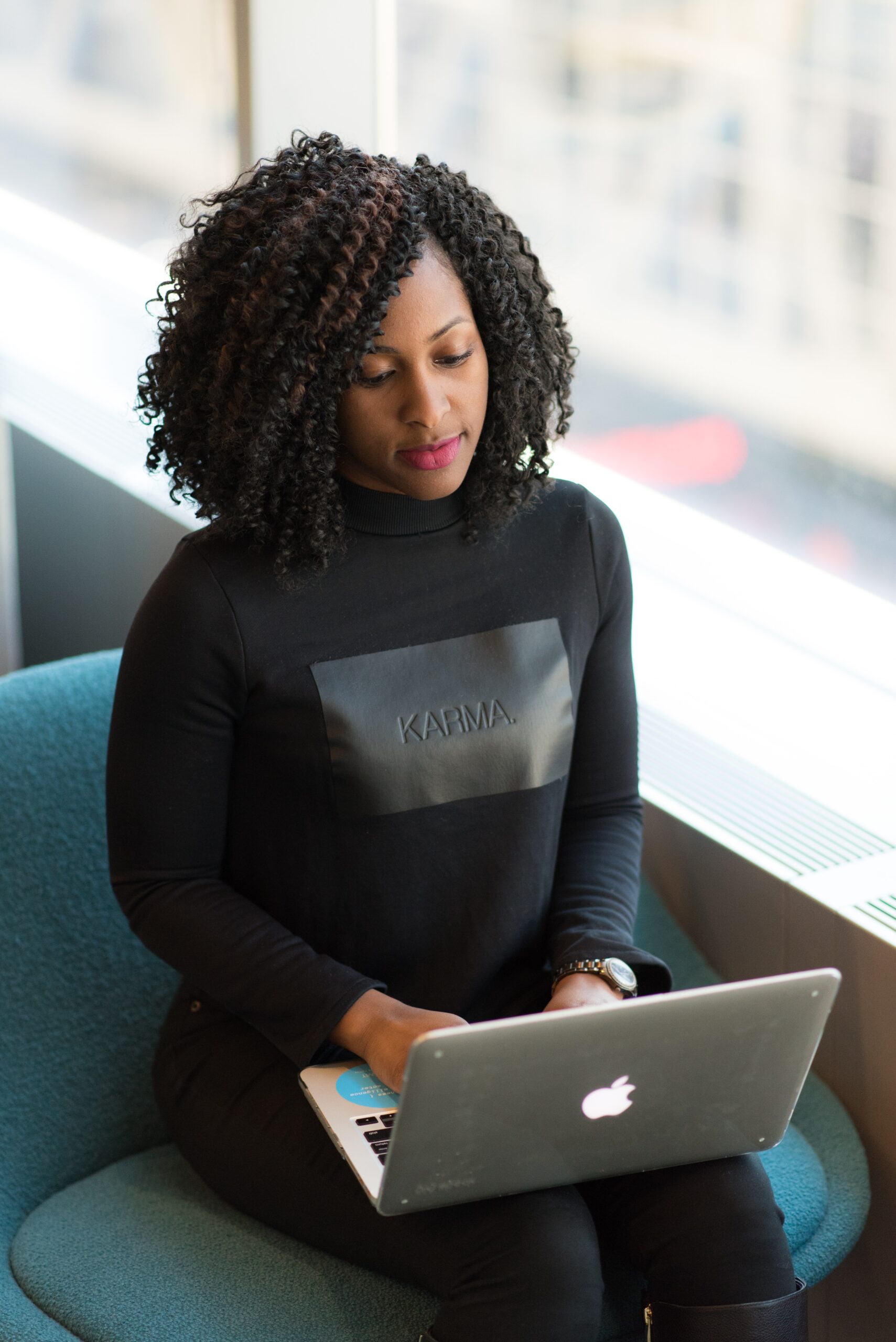
The emerging trends and technologies in ASR datasets are transforming the field and have significant implications for its future. Transfer learning, unsupervised learning, and the use of synthetic data are all promising approaches that can improve the accuracy and efficiency of ASR systems. By leveraging these techniques, ASR developers can create more accurate and affordable systems for a wider range of industries and applications. The Way With Words speech collection dataset is an excellent example of how synthetic data can be used to generate high-quality speech datasets for ASR. As ASR technology continues to evolve, we can expect to see more innovative approaches to data collection and processing that will continue to push the boundaries of what is possible with ASR.
Additional Services
About Captioning
Perfectly synched 99%+ accurate closed captions for broadcast-quality video.
Machine Transcription Polishing
For users of machine transcription that require polished machine transcripts.