The Benefits Of A Conversational Speech Dataset
Here Are Some Benefits Of A Conversational Speech Dataset That You Should Know About
A conversational speech dataset is a powerful tool in natural language processing (NLP) that can help in training machine learning algorithms to understand, process and generate human language. They are created by collecting speech data of natural human conversations, transcribing the audio into text and then annotating it with relevant information like speaker identification, language, dialect, gender, and more. The use of conversational speech datasets enables NLP models to be trained on more realistic and diverse speech patterns, and this has a direct impact on their accuracy and efficacy in various applications. In this blog post, we will explore the benefits of conversational speech datasets, their importance in developing NLP models, and their potential for real-world applications. We will also discuss the process of creating and using high-quality conversational speech datasets and provide specific examples and insights.
Benefits of Conversational Speech Datasets
Improved accuracy of NLP models: The use of conversational speech datasets allows NLP models to be trained on more varied and realistic speech patterns. This improves the accuracy of these models in understanding and processing natural language. The use of such datasets has been shown to improve the performance of a wide range of NLP models, including speech recognition, machine translation, and chatbot systems.
Increased scalability: Conversational datasets provide a large amount of training data, which enables NLP models to be trained on a vast number of language patterns. This scalability is essential for developing models that can accurately understand and process language on a global scale. With the increasing demand for multilingual and multicultural communication, the use of conversational speech datasets has become even more important for developing NLP models that can cater to diverse populations.
Better representation of diverse populations: Conversational datasets provide an opportunity to collect speech samples from a diverse range of populations, including different languages, dialects, accents, and speech patterns. This helps to ensure that NLP models are not biased towards a particular population and can accurately represent diverse speech patterns. This is crucial for developing NLP models that can cater to different regions, cultures, and communities.
Real-world applications: Conversational datasets are increasingly being used in real-world applications such as chatbots, speech recognition systems, and virtual assistants. These applications require NLP models that can accurately process natural language, and conversational speech datasets play a crucial role in developing these models. With the growing demand for intelligent virtual assistants and chatbots, the use of conversational speech datasets has become a key factor in their development and deployment.
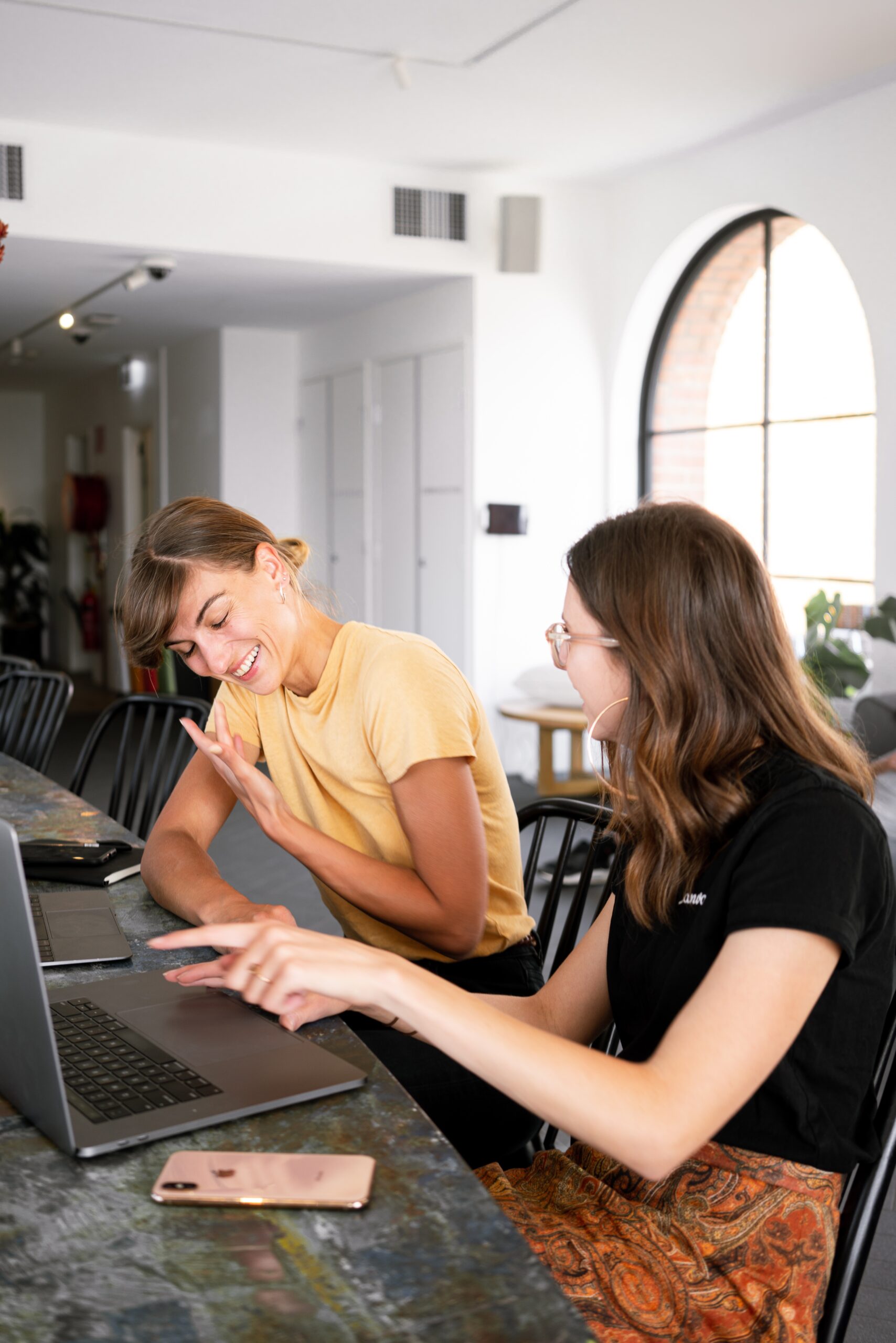
Using Conversational Speech Datasets in NLP Models
Conversational speech datasets can be used in various NLP models, including speech recognition, machine translation, sentiment analysis, and chatbot systems. These models require large amounts of training data to learn and understand natural language patterns accurately.
One example of the use of conversational datasets is in the development of chatbot systems. Chatbots are virtual assistants that can engage in human-like conversations with users. These systems require a large amount of conversational data to be trained effectively. Conversational speech datasets can be used to train chatbot systems to understand and respond to natural language effectively.
Another example is in speech recognition systems. Speech recognition systems are used to convert spoken language into text. The accuracy of these systems is crucial for their success in various applications, including virtual assistants, transcription services, and dictation software. Conversational datasets can be used to train speech recognition systems to accurately recognise different speech patterns, including accents, dialects, and languages.
Creating High-Quality Conversational Speech Datasets
The process of creating high-quality conversational speech datasets involves several steps, including:Real-World Applications of Conversational Speech Datasets
The use of conversational datasets has many real-world applications, including:
Virtual assistants: Conversational speech datasets are used to train virtual assistants, such as Amazon’s Alexa and Google Assistant. These assistants are designed to understand natural language and respond to users’ queries and commands.
Customer service chatbots: Conversational datasets are used to train chatbots that can provide customer service support through chat. These chatbots can handle simple queries, provide information about products or services, and help customers with their issues.
Language translation: Conversational datasets are used to train machine translation models that can translate speech from one language to another. This is useful for multilingual communication and can be applied in various fields, including business, healthcare, and tourism.
Conversational speech datasets are a powerful tool in developing NLP models that can accurately understand and process natural language. The use of these datasets enables NLP models to be trained on more diverse and realistic speech patterns, improving their accuracy and efficacy. The creation of high-quality conversational speech datasets involves collecting speech recordings, cleaning and annotating the transcriptions, and ensuring quality control. Conversational datasets have many real-world applications, including virtual assistants, customer service chatbots, language translation, and transcription services. With the growing demand for intelligent virtual assistants and chatbots, the use of conversational datasets has become increasingly important for developing and deploying these systems.
Additional Services
About Captioning
Perfectly synched 99%+ accurate closed captions for broadcast-quality video.
Machine Transcription Polishing
For users of machine transcription that require polished machine transcripts.