The Importance of A Diverse Speech Dataset for Speech Recognition
A Diverse Speech Dataset for Speech Recognition Technology Can Be What Sets Your Technology Apart From The Rest.
A diverse dataset for speech recognition has become crucial in the development of these technologies. Speech recognition technologies have become increasingly important in today’s world, where people are increasingly relying on them for various tasks. From dictating emails and texts to virtual assistants like Siri and Alexa, speech recognition technologies have revolutionised the way we communicate and interact with devices. However, these technologies are not without their limitations. One of the biggest challenges faced by speech recognition technologies is the accurate recognition and transcription of speech in various accents, languages, and dialects.
Speech recognition technologies work by using machine learning algorithms that are trained on large datasets of speech samples. The more diverse the dataset, the more accurate the algorithms can become. This is where the importance of diverse speech datasets comes in. By including a wide range of speech samples in training models, speech recognition technologies can become more accurate and reduce bias. In this blog post, we will explore the importance of diverse speech datasets in training models for speech recognition technologies and highlight some best practices for creating and using these datasets.
Importance of A Diverse Speech Dataset For Speech Recognition
The importance of diverse speech datasets cannot be overstated. To be effective, speech recognition technologies must be able to recognise and transcribe speech in various accents, languages, and dialects. This is because speech patterns and pronunciations vary significantly across different regions and demographics. By including a wide range of speech samples in training models, speech recognition technologies can become more accurate and reduce bias.
Diverse speech datasets can also help these technologies to better understand different speech patterns and pronunciations, improving overall performance. For example, if a speech recognition technology is trained on a diverse dataset of English language speakers, it will be better equipped to recognise and transcribe speech in different English accents, such as American English, British English, or Australian English.
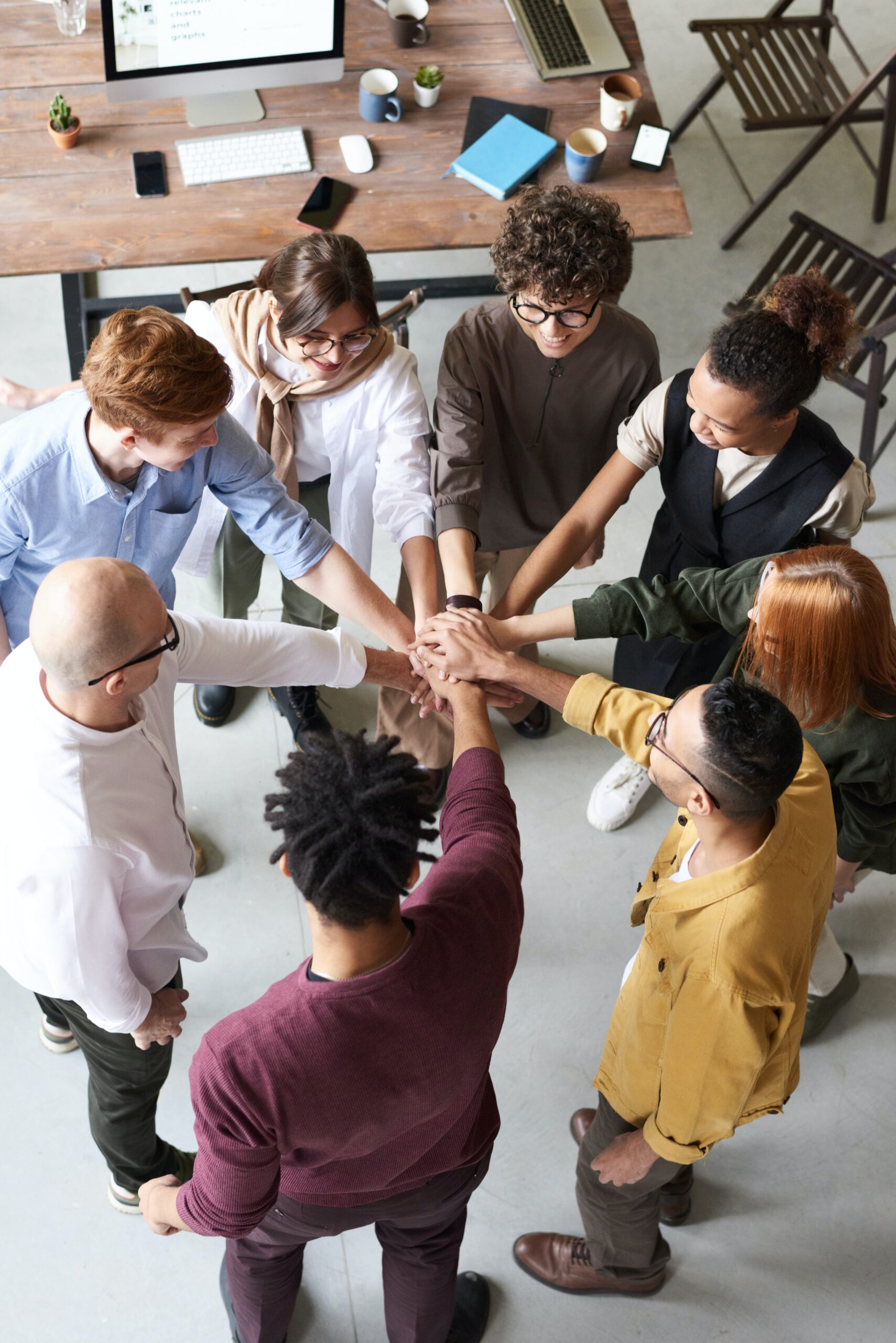
Best Practices for Creating Diverse Speech Datasets
- Include a wide range of accents, languages, and dialects in the dataset to ensure diversity. The first step in creating a diverse speech dataset is to ensure that it includes a wide range of accents, languages, and dialects. This ensures that the speech recognition model is trained to recognize speech in various contexts, which improves its accuracy. It’s essential to include accents and dialects that are less commonly spoken, as these are often underrepresented in existing speech datasets.
- Record Speech Samples in Real-World Scenarios. Speech recognition models need to be trained on speech samples that reflect real-world scenarios. It’s important to record speech samples in various environments and settings, such as in a noisy room, outdoors, or on the phone. This ensures that the speech recognition model is trained to recognize speech in various contexts, which improves its accuracy. Our custome speech collection datasets here at Way With Words ensure that real-world scenarios are the foundation of all recorded calls.
- Use high-quality recording equipment to capture clear audio. High-quality recording equipment is essential for creating a diverse speech dataset. The equipment should capture clear audio and minimize background noise. This ensures that the speech recognition model is trained on high-quality speech samples, which improves its accuracy.
- Consider the demographics of the speakers in the dataset to avoid bias It’s important to consider the demographics of the speakers when creating a diverse speech dataset. Including speech samples from various age groups, genders, and cultural backgrounds ensures that the speech recognition model is trained to recognize speech from a diverse range of speakers. This also helps to reduce bias in the dataset.
- Continuously update and expand the dataset to include new accents and dialects as they emerge. Speech recognition technology is constantly evolving, and new accents, languages, and dialects are emerging all the time. It’s essential to continuously update and expand the speech dataset to include these new speech samples. This ensures that the speech recognition model is trained on the most up-to-date speech samples, which improves its accuracy.
Challenges in Recognising Diverse Speech
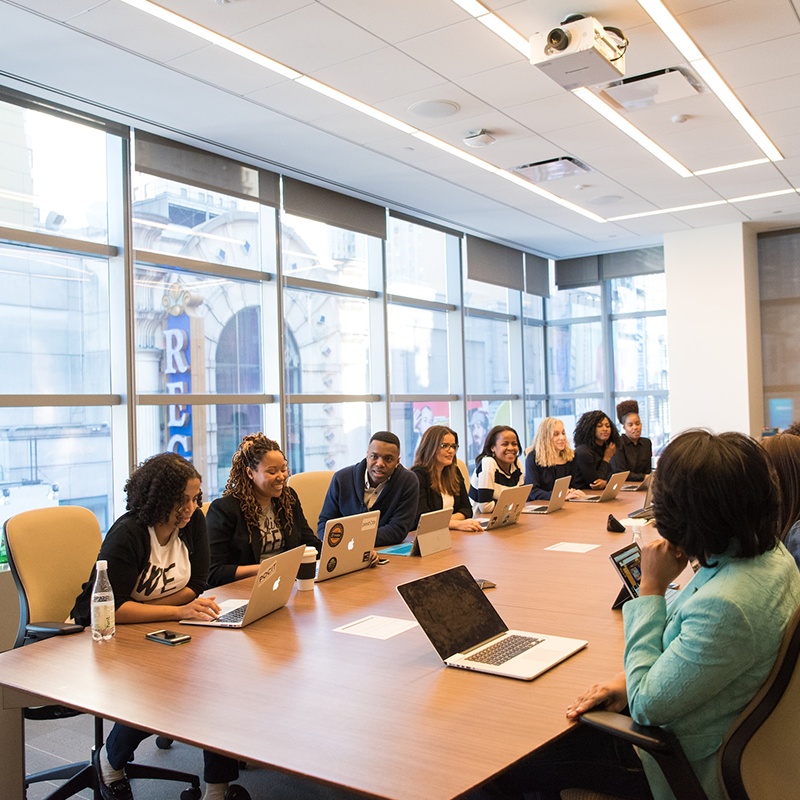
Examples of Successful Use of Diverse Speech Datasets in Speech Recognition Technologies
Google’s speech recognition technology has utilised diverse speech datasets in its training models, leading to significant improvements in accuracy. Google’s speech recognition technology can recognise over 110 languages and dialects, thanks to its diverse speech datasets.
Amazon’s Alexa has also incorporated diverse speech datasets to improve its ability to recognise and respond to different accents and languages. Alexa can recognise and respond to different English accents, as well as various languages, including Spanish, French, and German.
Diverse speech datasets are essential for improving the accuracy and reducing bias in speech recognition technologies. We take great pride in our diverse African speech dataset, contact us today to find out more about it. By including a wide range of accents, languages, and dialects in training models, these technologies can become more effective at recognising and transcribing speech in various contexts. As speech recognition technologies continue to evolve, it is important to prioritise the use of diverse speech datasets to ensure that they can be used effectively by people from all backgrounds and regions. By doing so, we can create more inclusive and effective speech recognition technologies that benefit everyone.
Additional Services
About Captioning
Perfectly synched 99%+ accurate closed captions for broadcast-quality video.
Machine Transcription Polishing
For users of machine transcription that require polished machine transcripts.