How is Machine Learning Used in Healthcare
How is Machine Learning Used in Healthcare and What Role Does it Play in The Advancement of Healthcare
With the fast paced growth of machine learning, it is important to highlight how is machine learning used in healthcare. Machine learning, a subset of artificial intelligence, is revolutionising the healthcare industry. With its ability to analyse massive amounts of data and identify patterns, machine learning has become an invaluable tool for healthcare professionals in diagnostics, treatment, patient monitoring, and data analysis. This blog post aims to provide a comprehensive overview of the role of machine learning in healthcare, highlighting its benefits and potential impact on the industry.
Diagnostics
Accurate and timely diagnostics are critical for effective treatment and patient outcomes. Machine learning algorithms excel at analysing complex medical data, enabling healthcare providers to make faster and more accurate diagnoses. Machine learning models can process various types of medical data, including medical images, genomic data, electronic health records (EHRs), and patient-reported symptoms.
In medical imaging, machine learning algorithms can analyse radiological images such as X-rays, CT scans, and MRIs to detect anomalies, classify diseases, and aid in early detection. These algorithms can quickly analyse large datasets, assisting radiologists in identifying patterns and making more accurate diagnoses.
Genomic data analysis is another area where machine learning plays a vital role. By analysing genetic information, machine learning algorithms can identify patterns and associations that help predict the likelihood of developing certain diseases. This information can lead to personalised treatment plans and proactive interventions.
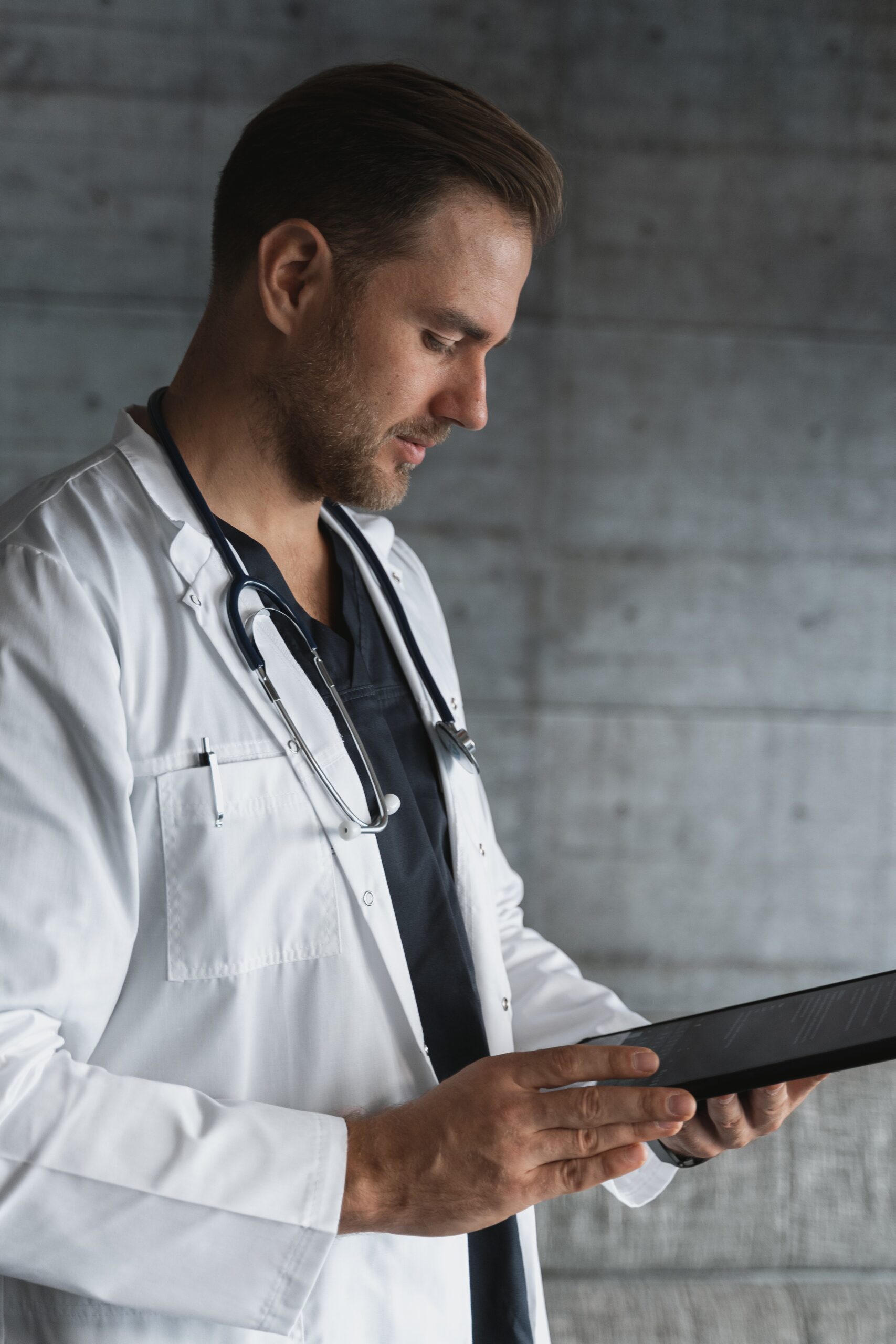
Treatment
Machine learning is transforming treatment methods by enabling personalised medicine and improving treatment outcomes. By analysing patient data, including medical records, lab results, and genomic information, machine learning algorithms can identify personalised treatment options and predict the effectiveness of different interventions.
In cancer treatment, machine learning algorithms can analyse large datasets to identify specific tumour characteristics, predict treatment responses, and optimise treatment plans. This approach helps oncologists design targeted therapies and select the most effective treatment options for individual patients.
Patient Monitoring
Machine learning algorithms are also enhancing patient monitoring and improving outcomes through real-time data analysis. By continuously analysing patient data, including vital signs, EHRs, and wearable device data, machine learning algorithms can detect early signs of deterioration or complications and alert healthcare providers.
For patients with chronic conditions, machine learning algorithms can analyse continuous monitoring data to identify patterns and predict exacerbations. This information enables healthcare professionals to intervene early, preventing hospital readmissions and improving patient outcomes.
Data Analysis
The vast amount of healthcare data generated every day presents a significant challenge for traditional analysis methods. Machine learning excels in analysing large datasets, extracting valuable insights, and improving data-driven decision-making in healthcare.
Machine learning algorithms can analyse EHRs to identify trends, predict disease outbreaks, and improve resource allocation. By integrating data from various sources, such as hospital records, wearable devices, and public health databases, machine learning can provide a holistic view of patient health and population health trends.
In clinical research, machine learning algorithms can analyse massive datasets to identify patterns, discover new biomarkers, and assist in drug discovery. This approach accelerates the research process and enables scientists to make breakthroughs that would otherwise be challenging to achieve.
Benefits of Machine Learning in Healthcare
The adoption of machine learning in healthcare brings numerous benefits and has the potential to transform the industry. Some of the key benefits include:
Enhanced Accuracy: Machine learning algorithms can process vast amounts of data and identify patterns that human analysis may overlook. This leads to more accurate diagnoses, treatment plans, and predictions.
Personalised Medicine: Machine learning enables personalised medicine by analysing individual patient data and tailoring treatment plans accordingly. This approach improves treatment outcomes and reduces adverse reactions.
Improved Efficiency: Machine learning algorithms automate time-consuming tasks, such as data analysis and documentation, freeing up healthcare professionals to focus on patient care. This improves efficiency and reduces healthcare costs.
Early Detection and Prevention: Machine learning algorithms can detect early signs of diseases or complications, enabling timely interventions and preventive measures. By analysing large datasets and identifying subtle patterns, machine learning algorithms can help healthcare providers identify individuals at high risk for certain diseases, allowing for early intervention and preventive measures. This proactive approach has the potential to significantly reduce the burden of chronic diseases and improve population health outcomes.
Streamlined Workflows: Machine learning algorithms can automate repetitive and mundane tasks, such as data entry and administrative work. This streamlines workflows, reduces the risk of errors, and allows healthcare professionals to focus on delivering quality care to patients.
Real-time Decision Support: Machine learning algorithms can provide real-time decision support to healthcare providers, aiding in clinical decision-making. By analysing patient data and comparing it with vast amounts of existing medical knowledge, machine learning algorithms can offer treatment recommendations, flag potential medication interactions, and suggest optimal dosage adjustments.
Predictive Analytics: Machine learning models can leverage historical data to predict future health outcomes. This capability enables healthcare organisations to anticipate patient needs, allocate resources effectively, and plan for potential healthcare challenges. Predictive analytics can also be utilised for forecasting disease outbreaks and optimising public health strategies.
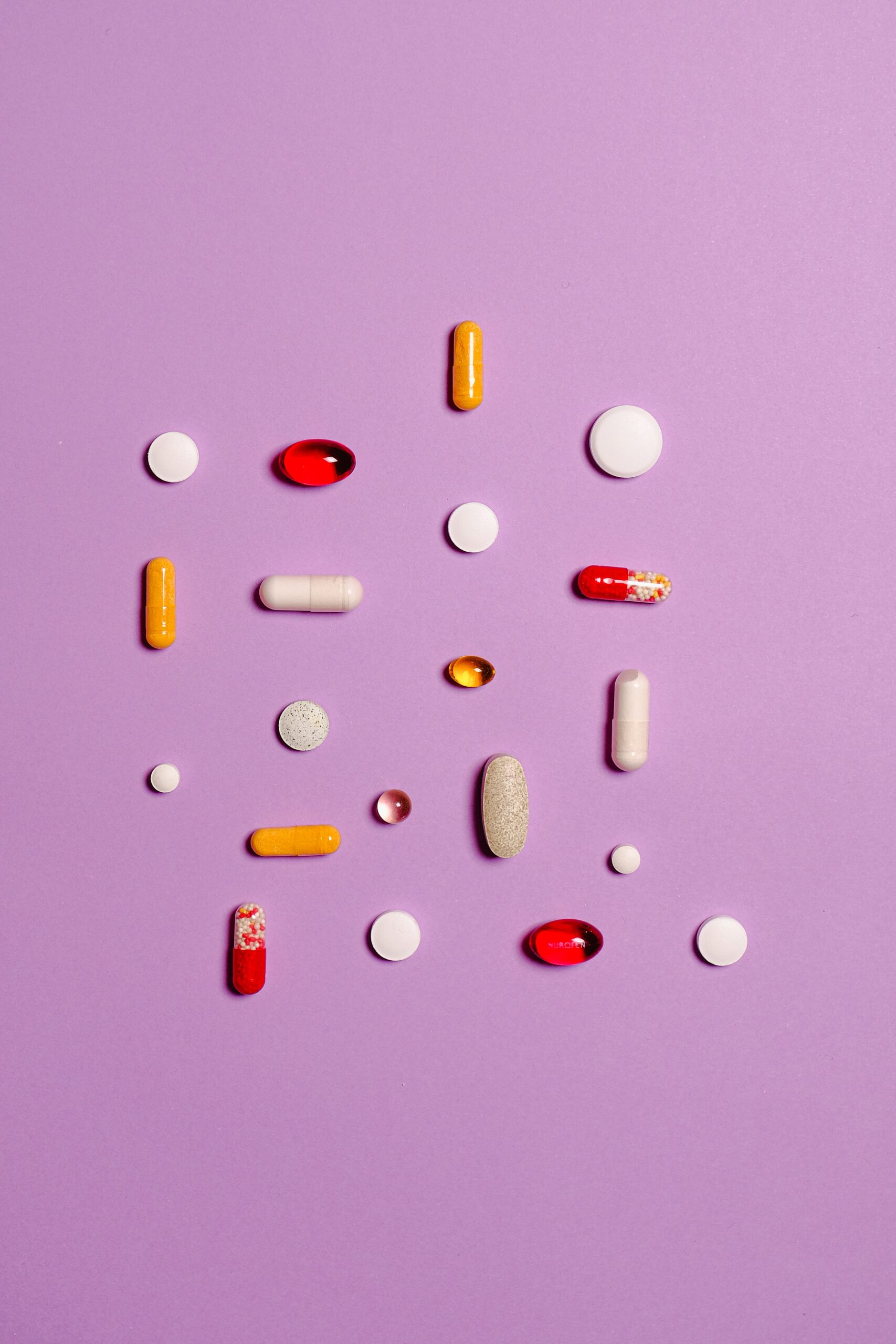
Potential Impact of Machine Learning in Healthcare
The potential impact of machine learning on healthcare is immense. It has the power to revolutionise healthcare delivery, improve patient outcomes, and enhance population health management. Here are some key ways machine learning can shape the future of healthcare:
Precision Medicine: Machine learning algorithms can analyse genomic data, medical history, and other patient-specific factors to develop tailored treatment plans. This approach allows for targeted interventions and more effective treatments, minimising trial and error in healthcare.
Remote Monitoring and Telehealth: Machine learning algorithms can analyse data from wearable devices and remote monitoring systems, enabling remote patient monitoring. This technology facilitates proactive care, reduces hospital visits, and improves patient convenience, particularly for individuals with chronic conditions.
Drug Discovery and Development: Machine learning algorithms can analyse large volumes of biological and chemical data to accelerate drug discovery and development. By identifying potential drug targets, predicting drug interactions, and optimising drug design, machine learning can significantly reduce the time and cost associated with bringing new drugs to market.
Health Data Security: As the healthcare industry embraces digital transformation, ensuring the security and privacy of sensitive patient data becomes paramount. Machine learning algorithms can assist in detecting anomalies and identifying potential security breaches, safeguarding patient information from unauthorised access.
Ethical Considerations: The integration of machine learning in healthcare raises important ethical considerations. Issues such as data privacy, bias in algorithms, and accountability need to be addressed to ensure that machine learning technologies are deployed responsibly and equitably, maintaining trust and fairness in healthcare.
Machine learning is reshaping the healthcare landscape, revolutionising diagnostics, treatment, patient monitoring, and data analysis. With its ability to analyse vast amounts of data and identify patterns, machine learning holds great potential to improve patient outcomes, personalise medicine, and enhance population health management. However, it is important to strike a balance between the benefits of machine learning and ethical considerations to ensure that these technologies are deployed responsibly and equitably. As machine learning continues to advance, it will undoubtedly play a central role in the future of healthcare, driving innovation and transforming the way healthcare is delivered.
With a 21-year track record of excellence, we are considered a trusted partner by many blue-chip companies across a wide range of industries. At this stage of your business, it may be worth your while to invest in a human transcription service that has a Way With Words.
Additional Services
About Captioning
Perfectly synched 99%+ accurate closed captions for broadcast-quality video.
Machine Transcription Polishing
For users of machine transcription that require polished machine transcripts.