How to Determine Mean Opinion Scores for Audio and Video Quality Evaluation
Determining Mean Opinion Score For Your Audio is Vital
Mean Opinion Score (MOS) is a standardised method of evaluating the quality of audio and video content based on subjective human judgments. MOS is an important metric in the development of speech recognition technology (SRT) and natural language processing (NLP) systems, as accurate evaluation of audio quality is essential for accurate transcription and analysis. In this blog post, we will explore how to calculate MOS for different types of audio commonly encountered in SRT and NLP projects, as well as the importance of MOS in improving the accuracy of NLP and SRT technology. We will also look at examples of how MOS scores have been used to improve SRT and NLP technology in the past, with a focus on the use of MOS scores to train machine learning models.
What is MOS?
MOS is a numerical score that represents the subjective quality of an audio or video file. It is calculated based on the ratings given by a group of human listeners who listen to the file and rate its quality on a scale of 1 to 5, where 1 represents the worst quality and 5 represents the best quality.
Calculating MOS
The MOS is calculated by taking the average of the ratings given by the listeners. For example, if a group of 10 listeners rated an audio file with scores of 4, 4.5, 5, 3.5, 4.5, 3, 4, 3.5, 4, and 5, the MOS would be calculated as follows:
MOS = (4 + 4.5 + 5 + 3.5 + 4.5 + 3 + 4 + 3.5 + 4 + 5) / 10 MOS = 4.15
In this example, the MOS score for the audio file is 4.15.
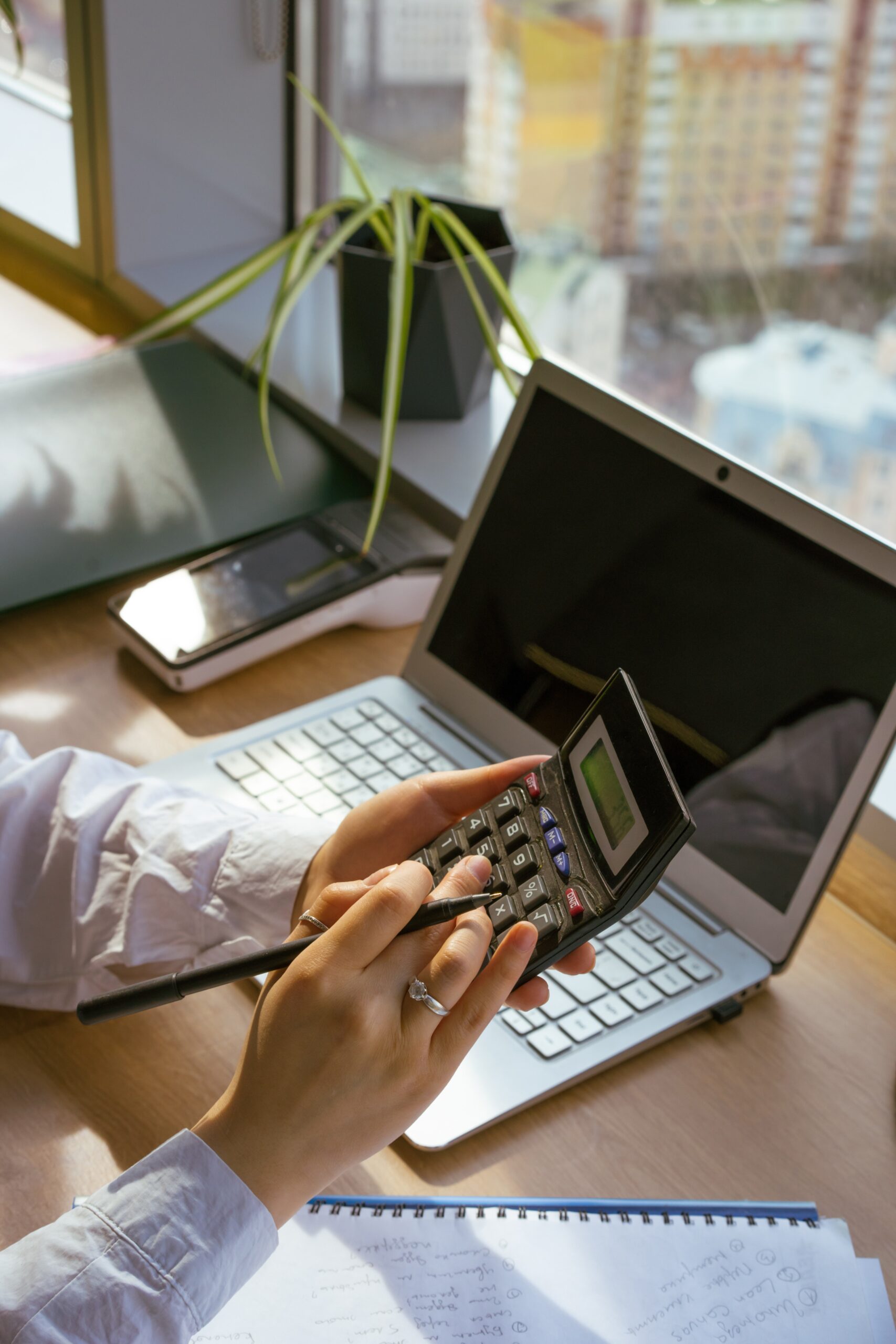
Calculating MOS for Different Types of Audio
When calculating MOS for speech, it is important to consider factors such as intelligibility, clarity, and naturalness. Listeners may rate speech quality based on factors such as the speaker’s accent, speaking rate, and the presence of background noise.
MOS can also be calculated for music. In this case, the focus may be on factors such as tonality, pitch accuracy, and dynamics. Listeners may rate music quality based on factors such as the presence of distortion, the balance between instruments, and the overall emotional impact of the music.
When evaluating noise quality, MOS can be calculated based on factors such as the loudness, tonality, and overall annoyance of the noise.
Importance of MOS in NLP and SRT:
MOS is an essential metric for evaluating the accuracy of NLP and SRT systems. The quality of the audio or video signal can have a significant impact on the accuracy of transcription and analysis. Low-quality audio signals can lead to errors in speech recognition and transcription, resulting in inaccurate results. MOS scores can be used to identify areas where audio quality can be improved, and to optimise the performance of NLP and SRT systems.
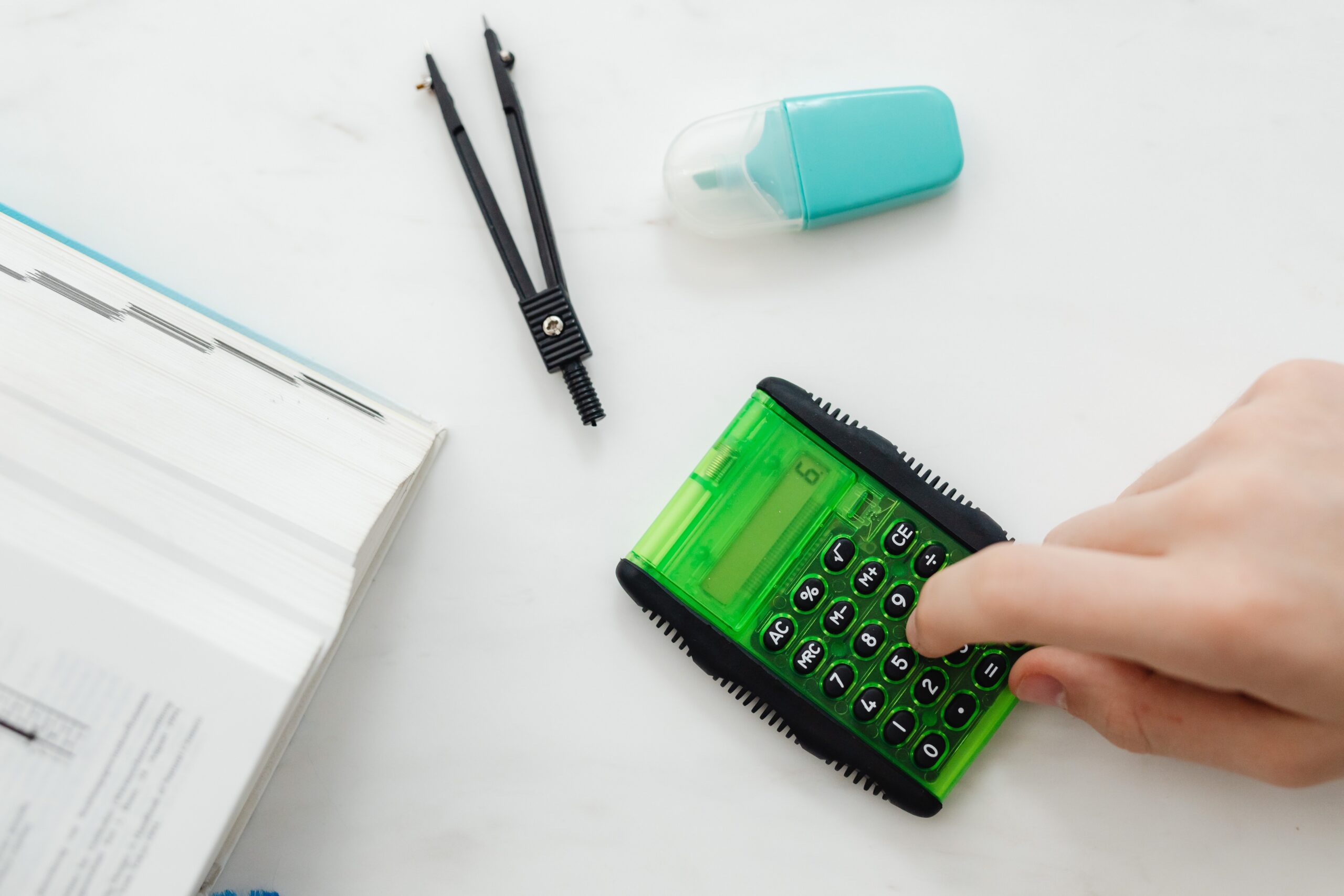
Examples of MOS in Action
MOS scores have been used in a variety of applications to improve the accuracy of NLP and SRT systems. For example, MOS scores have been used to train machine learning models for speech recognition. In one study, researchers used MOS scores to train a machine learning model to recognise accented speech, resulting in a significant improvement in accuracy. MOS scores have also been used to improve the accuracy of speech recognition in noisy environments. By using MOS scores to identify areas of low-quality audio, researchers were able to develop a machine learning model that was better able to recognise speech in noisy environments.
MOS scores have also been used to evaluate the quality of text-to-speech (TTS) systems. In one study, MOS scores were used to evaluate the performance of a TTS system that was designed to read news articles aloud. By using MOS scores to identify areas where the TTS system was producing low-quality audio, the researchers were able to improve the performance of the system.
Conclusion
MOS is a crucial metric for evaluating the quality of audio and video content in the context of NLP and SRT development. By calculating MOS scores for different types of audio commonly encountered in NLP and SRT projects, developers can optimise the performance of their systems and improve the accuracy of transcription and analysis. MOS scores can also be used to train machine learning models, resulting in significant improvements in accuracy. As such, MOS is a vital tool for anyone working in the field of NLP and SRT, and its importance should not be underestimated.
Additional Services
About Captioning
Perfectly synched 99%+ accurate closed captions for broadcast-quality video.
Machine Transcription Polishing
For users of machine transcription that require polished machine transcripts.