How Speech Data Annotation Enhances Speech Recognition for Linguistic Diversity
Improving ASR Models with Speech Data Annotation
When it comes to creating accurate automated speech recognition (ASR) models, speech data annotation plays a crucial role in enhancing natural language processing (NLP) and machine learning (ML) models. To put it simply, data annotation involves the labelling of a large volume of speech data by human annotators, which helps these models accurately understand and interpret human spoken language. Leveraging call centre data, specifically human-labelled speech data, can significantly enhance the performance of NLP and ML models, and in this case recognising the diverse dialects and accents found in the United Kingdom.
The Challenge of Linguistic Diversity
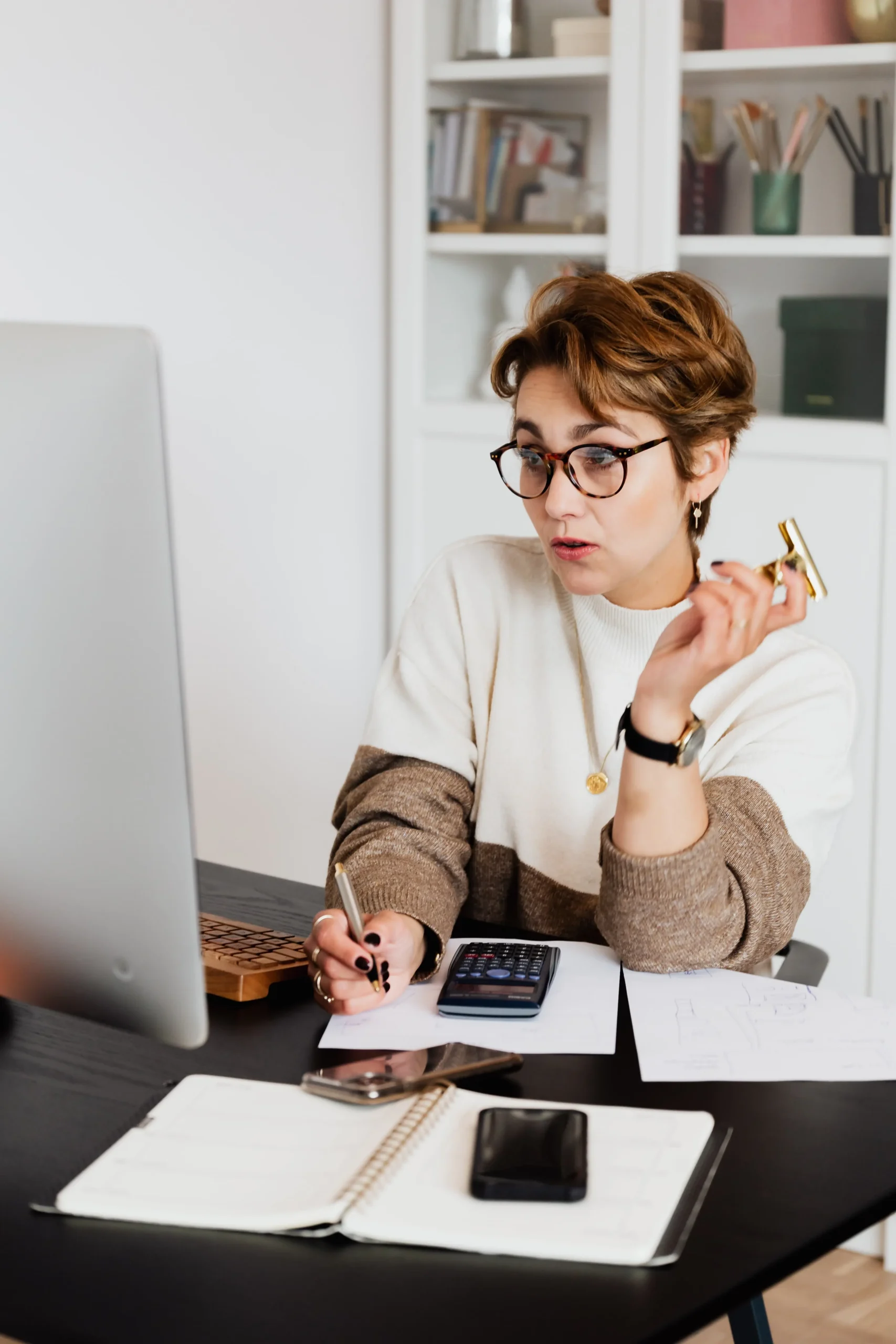
The Benefits of Using Annotated Speech Data for UK English
1. Improved Accuracy: ML models trained on annotated speech data become more accurate in understanding and interpreting various UK dialects and accents. By learning from this data, models can recognise and adapt to regional variations, leading to more precise and context-aware responses.
2. Enhanced User Experience: NLP models can better understand user queries and respond appropriately, regardless of the dialect or accent used. This personalised approach leads to a more satisfactory user experience and ultimately increases customer satisfaction.
3. Better Representation: Dialects and accents from across the UK are well-represented in the training datasets. This promotes inclusivity and avoids biased or skewed outcomes that may arise from relying solely on standardised language data.
4. Real-World Applications: ML models trained on call centre data are better equipped to handle real-world scenarios and challenges. By learning from authentic conversational data, models can adapt to the intricacies of UK dialects and accents encountered in everyday interactions.
Challenges and Considerations
While leveraging call centre data for training NLP and ML models holds immense promise, it’s important to address a few challenges and considerations:
1. Data Privacy: Proper anonymisation and adherence to data protection regulations are crucial when handling sensitive call centre data to ensure privacy and security.
2. Representation Bias: It’s essential to include a diverse range of dialects and accents in the dataset, avoiding bias towards any specific region or group. This ensures fair and unbiased results.
3. Continuous Model Improvement: Regular updates and fine-tuning of models are necessary to keep up with evolving language patterns, emerging dialects, and accents.
Success Story
How UK Call Centre Data Improved Regional Linguistic Diversity of Speech Recognition Models
The Client Brief
Evaluate a large volume of call centre speech data of UK and non-UK English and annotate based on regional accent and/or dialect. Knowledge of UK dialects essential.The Solution
Way With Words put together a team of data annotators as per client specification to evaluate all call centre speech samples. Following annotator and inter-annotator agreement, the confidence ratings were recorded and exported in csv format from our data annotation workflow for delivery to the client.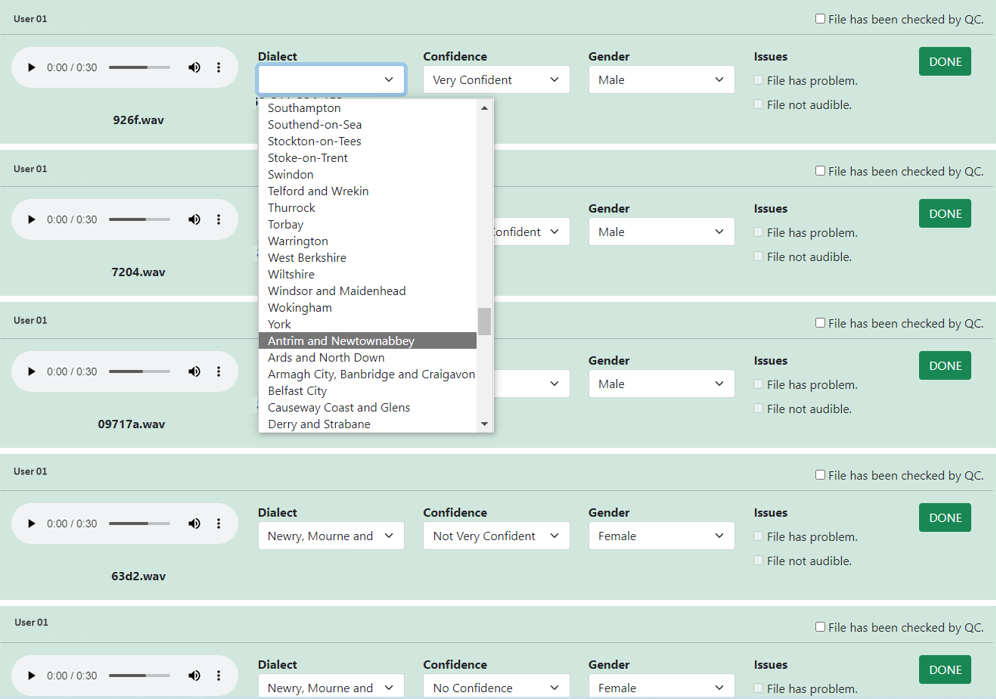
Step 1. Our data annotators identified the UK English dialect for each call centre speech sample from a verified regional list provided by the client.
Step 2. The confidence level rating of the dialect selected for each speech sample was recorded. Files with foreign or non-UK accents, or files with problematic audio, were identified and categorised for exclusion from the final results.
Step 3. Each speech sample underwent a second annotation process to ensure the accuracy and reliability of the data annotation. The level of agreement between the two annotators per speech sample, known as inter-annotator agreement, served as a measure of the consistency and reliability of the annotations.
Final outcome
The client was able to optimise their NLP and ML models to better understand UK dialects and accents. Incorporating the diverse linguistic variations encountered in call centre conversations, models can deliver more accurate, context-aware, and personalised results.
Ready to take your data annotation projects to the next level? With a proven track record of success and the ability to handle large-scale projects, we’re confident that we can help you achieve your goals. Contact us today to get started!
Additional Services
About Captioning
Perfectly synched 99%+ accurate closed captions for broadcast-quality video.
Machine Transcription Polishing
For users of machine transcription that require polished machine transcripts.