Text Annotation: Unlocking the Power of SRT and NLP
What Is Text Annotation? A Comprehensive Guide on All You Need to Know About Text Annotation
In the world of language processing, text annotation plays a pivotal role in harnessing the power of Speech Recognition Technology (SRT) and Natural Language Processing (NLP). From enabling accurate transcription to training sophisticated language models, text annotation provides the crucial bridge between unstructured textual data and machine understanding. In this blog post, we will delve into the concept of text annotation, its significance in SRT and NLP, and explore real-world examples and use cases within these domains.
Understanding Text Annotation
Text annotation involves the process of adding metadata or labels to unstructured text data. It involves identifying and categorising specific components within a given text, such as named entities, parts of speech, sentiment, or relationships between words. Annotation tasks can range from simple tasks like part-of-speech tagging to complex tasks like semantic role labelling or entity recognition.
Role of Text Annotation in SRT
Speech Recognition Technology (SRT) aims to convert spoken language into written text. However, this process can be challenging due to various factors such as accents, background noise, or speech disfluencies. Text annotation plays a vital role in SRT by improving the accuracy and efficiency of transcription.
Acoustic Modelling: Text annotation helps build acoustic models that map speech signals to corresponding textual representations. By annotating speech data with word-level transcriptions, phonetic representations, and contextual information, SRT systems can learn to better recognise and interpret different speech patterns.
Language Modelling: To enhance the performance of SRT systems, language modelling is crucial. Text annotation aids in creating language models by annotating vast amounts of text data with information such as part-of-speech tags, syntactic dependencies, and semantic roles. These annotations enable SRT systems to generate more accurate and coherent transcriptions by understanding the context and relationships between words.
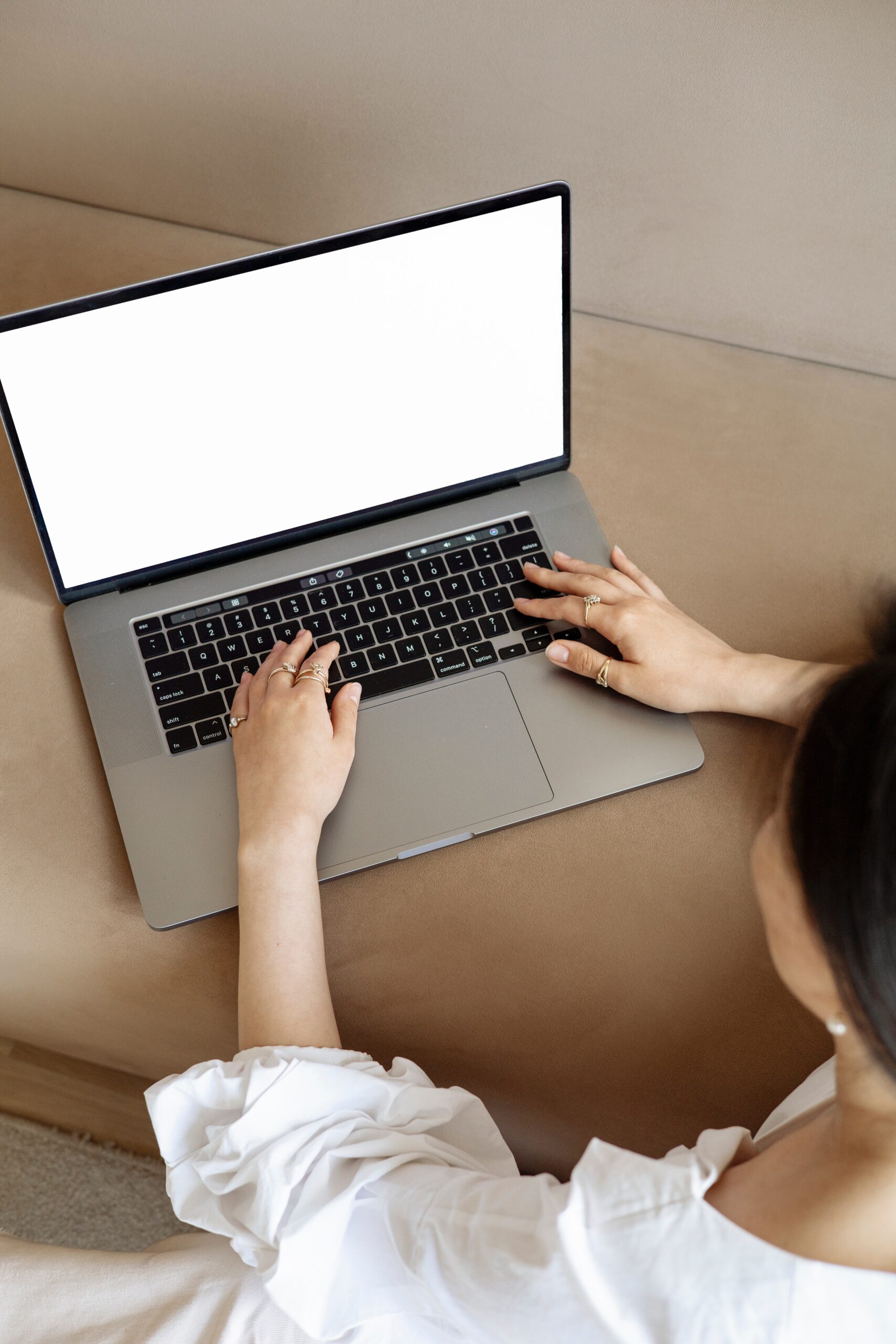
Use Cases of Text Annotation in SRT
Transcription Services: Text annotation plays a pivotal role in professional transcription services, where large volumes of audio data need to be accurately converted into text. Annotating the transcribed text with timestamps, speaker identification, and other relevant information helps in creating highly precise and well-structured transcripts.
Voice Assistants: Voice assistants like Siri, Google Assistant, or Amazon Alexa rely on text annotation to understand and respond to user commands accurately. By annotating training data with intent labels, entities, and dialog acts, these systems can recognise user intent, extract relevant information, and provide appropriate responses.
Role of Text Annotation in NLP:
Text annotation forms the backbone of Natural Language Processing (NLP) by enabling machines to understand and process human language effectively. It facilitates various NLP tasks, ranging from sentiment analysis and named entity recognition to machine translation and question answering.
Sentiment Analysis: Text annotation is instrumental in sentiment analysis, where the sentiment or opinion expressed in a given text is determined. By labelling text data with sentiment scores or categories, machine learning algorithms can learn to identify emotions, assess product reviews, or gauge public sentiment towards a particular topic.
Named Entity Recognition (NER): NER involves identifying and categorising named entities such as names, organisations, locations, or dates within a text. Text annotation allows for the manual or automatic labelling of named entities, enabling NLP models to extract valuable information and perform tasks like information retrieval, knowledge graph construction, or information extraction.
Use Cases of Text Annotation in NLP
Machine Translation: Text annotation is vital in training machine translation models. By aligning parallel texts in different languages and annotating them at the sentence or phrase level, NLP systems can learn to accurately translate between languages and bridge the linguistic divide.
Question Answering: Text annotation plays a significant role in question answering systems like chatbots and virtual assistants. By annotating question-answer pairs or passages of text with relevant information and relationships, NLP models can comprehend the context of a question and provide accurate and meaningful responses.
Information Extraction: Text annotation is crucial in information extraction tasks, where specific information needs to be extracted from unstructured text. By annotating the text with labels for entities, relationships, or events, NLP systems can identify and extract structured information from large volumes of text, enabling tasks such as knowledge base construction, data mining, or automatic report generation.
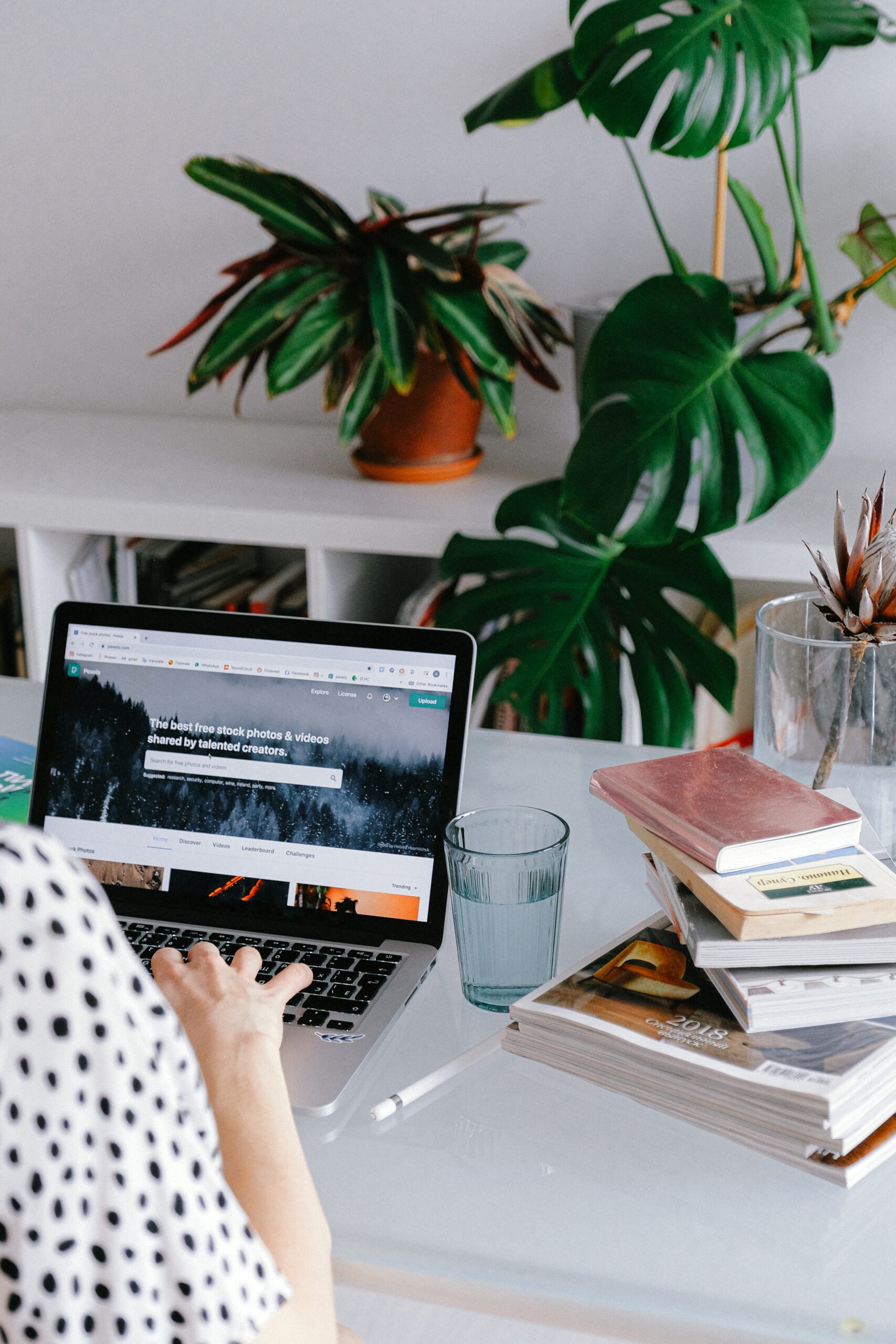
Challenges and Considerations in Text Annotation
While text annotation plays a vital role in SRT and NLP, there are challenges and considerations that need to be addressed:
Annotation Consistency: Ensuring consistent and high-quality annotations across a large volume of data is essential. Clear annotation guidelines, regular training, and inter-annotator agreement assessments help maintain consistency.
Annotation Bias: Annotation can be subjective, leading to potential biases. It is important to address biases that may arise from annotators’ perspectives or preconceived notions to ensure fair and unbiased training data.
Scalability: Annotating large datasets can be time-consuming and resource-intensive. Developing efficient annotation tools, leveraging active learning techniques, and exploring semi-supervised or unsupervised methods can help tackle scalability challenges.
Text annotation forms the foundation of SRT and NLP technologies, enabling accurate transcription, sophisticated language understanding, and effective communication between humans and machines. From improving speech recognition accuracy to training powerful language models, text annotation unlocks the potential of unstructured textual data. The applications and use cases discussed in this blog post highlight the importance of text annotation in various domains, paving the way for advancements in speech recognition, language understanding, and human-machine interaction. As technology continues to evolve, the role of text annotation will remain pivotal in empowering machines to comprehend and process human language more effectively.
With a 21-year track record of excellence, we are considered a trusted partner by many blue-chip companies across a wide range of industries. At this stage of your business, it may be worth your while to invest in a human transcription service that has a Way With Words.
Additional Services
About Captioning
Perfectly synched 99%+ accurate closed captions for broadcast-quality video.
Machine Transcription Polishing
For users of machine transcription that require polished machine transcripts.