The Role of Data Annotators in Machine Learning
The Unsung Heroes of AI: The Role of Data Annotators
Data annotators play a significant role in the development of AI. In the fast-paced world of artificial intelligence (AI) and machine learning (ML), we often marvel at the advancements and breakthroughs that AI technologies bring to our lives. From self-driving cars to voice assistants, AI has become an integral part of our society. However, behind the scenes, there is a group of unsung heroes who play a crucial role in the development of AI systems: data annotators. In this blog post, we will explore the vital role of annotators in machine learning and emphasise the significant impact they have on AI development.
What are Data Annotators?
Data annotators are individuals responsible for labelling and tagging data used to train machine learning models. They meticulously review and interpret data, adding annotations, labels, and metadata that help AI algorithms understand patterns and make accurate predictions. Data annotation involves various tasks, including image and video classification, object detection, sentiment analysis, speech recognition, and natural language processing.
The Importance of Data Annotation:
Data annotation is a labour-intensive and critical process in machine learning. The quality and accuracy of annotated data directly influence the performance and reliability of AI models. Data annotators play a pivotal role in creating high-quality training datasets that enable ML algorithms to learn and make accurate predictions. Here are some key reasons why data annotation is essential:
Training ML Models: Machine learning models require large amounts of labelled data to learn patterns and make predictions. Annotators create training datasets by meticulously labelling and annotating data, enabling ML algorithms to learn from diverse examples. These datasets are the foundation upon which AI systems are built.
Improving Model Performance: The accuracy of annotations directly impacts the performance of ML models. Data annotators ensure that annotations are precise, consistent, and representative of real-world scenarios. Well-annotated data allows ML models to generalise patterns effectively, leading to improved performance and robustness.
Handling Complex Data Types: AI systems operate on various types of data, including images, text, audio, and video. Annotators possess domain expertise and are skilled at interpreting and labelling complex data types accurately. They understand the nuances and context associated with the data, enabling ML models to grasp the subtleties necessary for accurate predictions.
Mitigating Bias: Bias in AI algorithms is a significant concern. Annotators play a critical role in mitigating bias by ensuring that the labelled data is diverse, inclusive, and representative of different demographics and perspectives. They follow guidelines and protocols to minimise bias and provide a balanced and fair representation of the data.
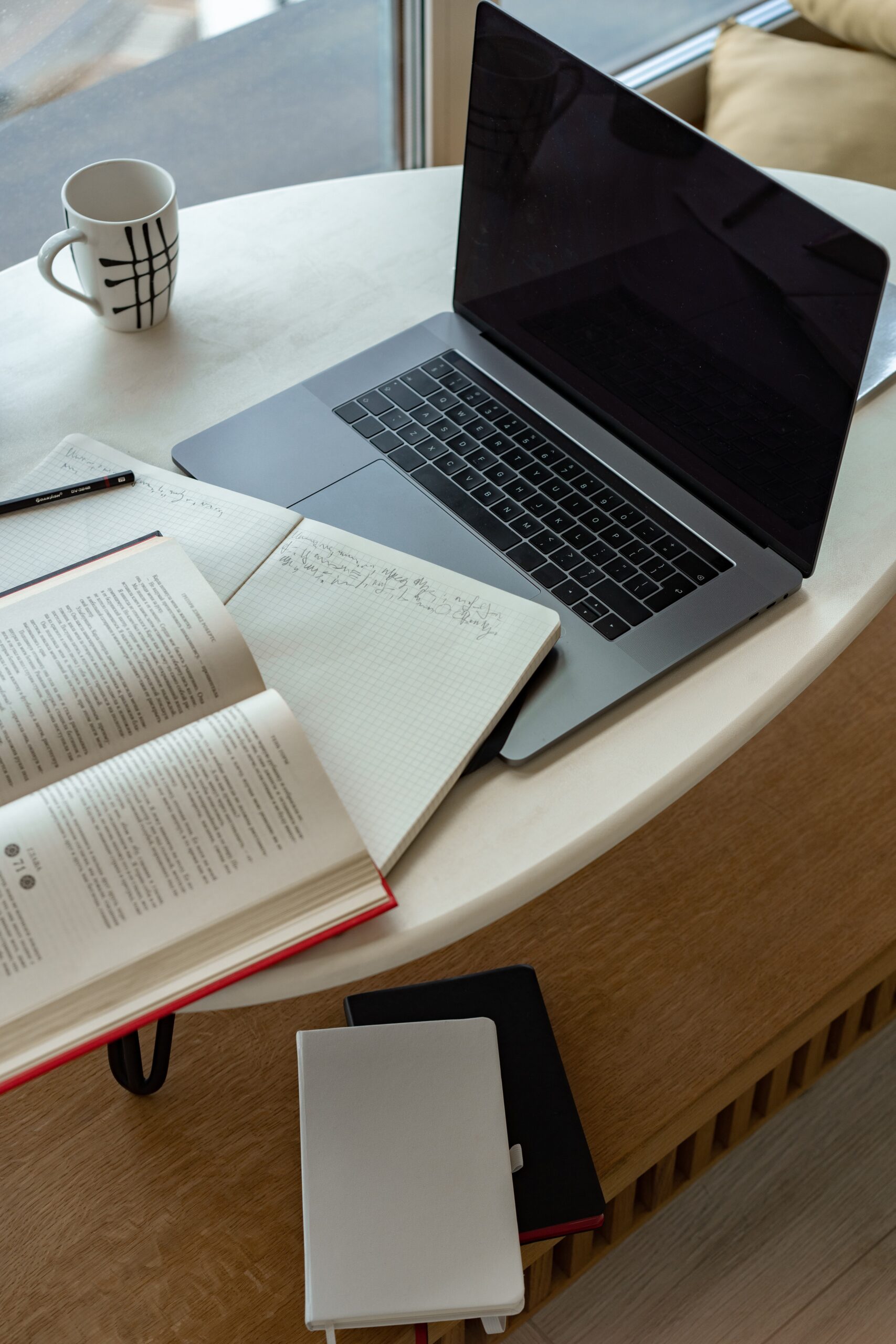
Challenges Faced by Data Annotators
Data annotation is not without its challenges. The work of data annotators can be demanding and time-consuming. Some common challenges faced by annotators include:
Subjectivity and Ambiguity: Interpreting data and adding annotations can involve subjective judgment calls, particularly in tasks like sentiment analysis or image classification. Data annotators must adhere to guidelines and maintain consistency while navigating ambiguous situations.
Expertise and Training: Data annotators require domain expertise and training to accurately label and annotate data. They need to understand the context and nuances associated with the data they are annotating. Continuous learning and skill development are crucial for annotators to stay abreast of evolving AI technologies and annotation techniques.
Time Constraints: Building high-quality annotated datasets is a time-consuming process. Data annotators often face tight deadlines while ensuring accuracy and quality. Balancing speed and precision can be challenging, and it requires effective time management skills.
Data Privacy and Security: Data annotators handle sensitive and private information during the annotation process. Ensuring data privacy and security is paramount, and annotators must adhere to strict protocols and guidelines to protect the confidentiality of the data they work with. They follow strict protocols to maintain data privacy and security, ensuring that the information they handle is protected from unauthorised access or breaches.
The Impact of Data Annotators on AI Development
The contributions of data annotators to AI development cannot be overstated. Their meticulous work lays the foundation for building robust and accurate AI systems. Here are some ways in which annotators have a significant impact:
Enabling Training and Iteration: Data annotators provide the labelled datasets that are essential for training ML models. Without their efforts, AI algorithms would lack the necessary data to learn patterns and make predictions. Annotators also play a crucial role in the iterative process of AI development. They review and refine annotations based on feedback from model performance, continuously improving the accuracy and reliability of ML algorithms.
Enhancing AI Performance and Generalisation: High-quality annotations created by data annotators contribute to improved AI performance. Accurate annotations enable ML models to generalise patterns effectively and make accurate predictions on unseen data. Annotators ensure that the training datasets cover diverse scenarios and capture edge cases, enabling AI systems to perform well in real-world situations.
Quality Control and Validation: Data annotators are responsible for maintaining the quality and integrity of annotated datasets. They perform rigorous quality control checks, verifying the accuracy and consistency of annotations. By ensuring that annotations align with established guidelines and standards, annotators help minimise errors and enhance the reliability of AI models.
Bias Mitigation and Fairness: Addressing bias in AI algorithms is crucial for ethical and fair AI development. Data annotators play a pivotal role in mitigating bias by carefully considering factors such as representation, inclusivity, and fairness during the annotation process. By providing diverse and balanced annotations, annotators contribute to the development of AI systems that are more equitable and unbiased.
Domain Expertise and Contextual Understanding: Data annotators bring valuable domain expertise to the annotation process. Their understanding of the subject matter helps in accurately interpreting and labelling data. Whether it’s medical images, legal documents, or financial data, annotators possess the necessary knowledge to annotate data effectively, enabling ML models to make informed decisions in specific domains.
Continuous Learning and Improvement: Data annotators are constantly learning and evolving along with advancements in AI technologies. They stay updated with the latest annotation techniques, tools, and guidelines. This continuous learning allows annotators to adapt to changing requirements and improve their annotation skills, ultimately enhancing the quality and relevance of annotated datasets.
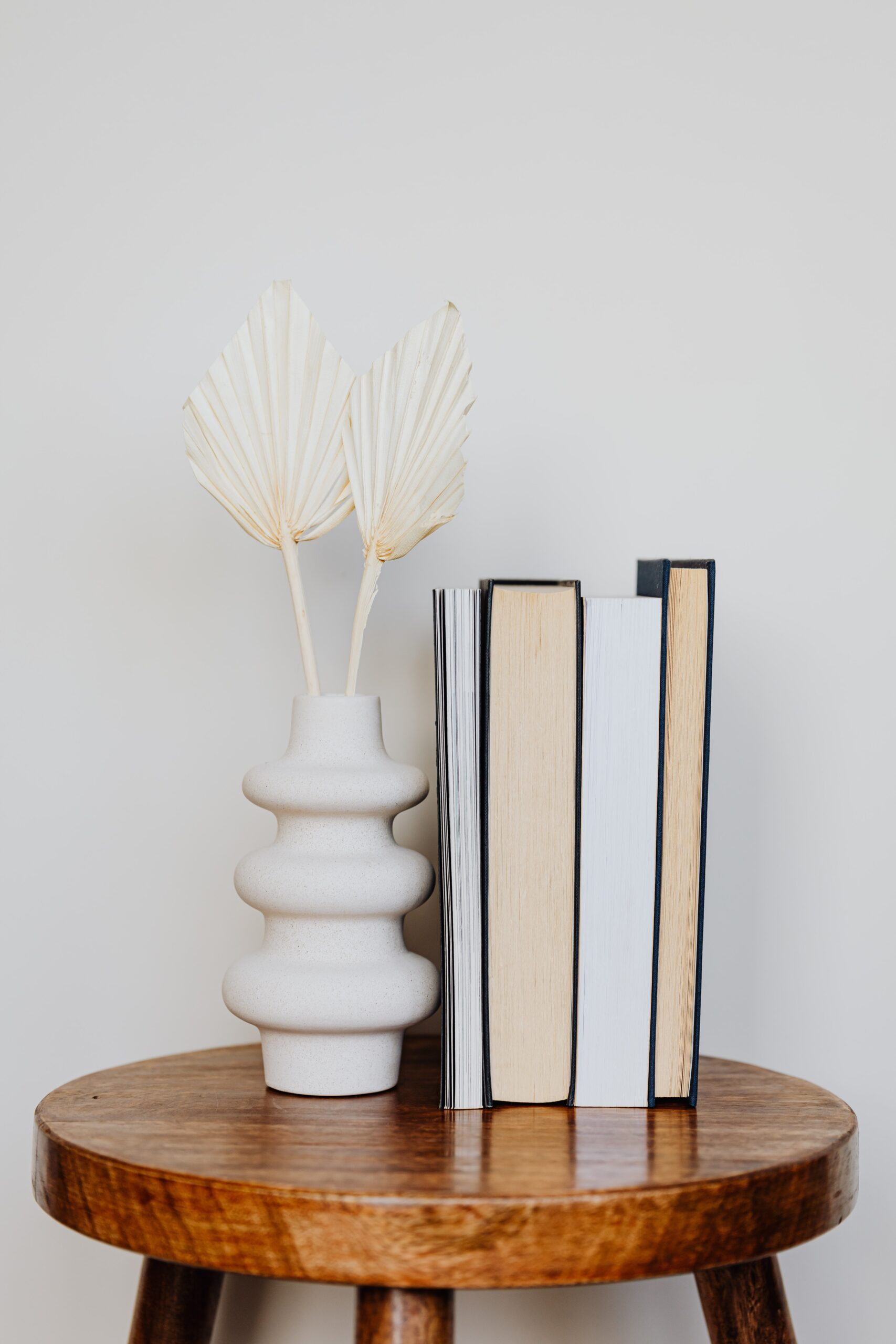
Data annotators are the unsung heroes behind the scenes of AI development. Their meticulous work in labelling and annotating data plays a vital role in training ML models and enabling AI systems to make accurate predictions. Annotators ensure the quality, accuracy, and fairness of annotated datasets, contributing to improved AI performance and mitigating bias. As AI technologies continue to advance, it is crucial to recognise and appreciate the significant impact that data annotators have on the development of AI systems. Their expertise and dedication are essential for building robust, reliable, and ethically responsible AI applications that benefit society as a whole.
With a 21-year track record of excellence, we are considered a trusted partner by many blue-chip companies across a wide range of industries. At this stage of your business, it may be worth your while to invest in a human transcription service that has a Way With Words.
Additional Services
About Captioning
Perfectly synched 99%+ accurate closed captions for broadcast-quality video.
Machine Transcription Polishing
For users of machine transcription that require polished machine transcripts.