What Does it Mean to Annotate Text?
Annotating Text for Human Sentiment: What Does it Mean to Annotate Text?
What does it mean to annotate text? In today’s digital age, businesses are increasingly relying on text data for insights and decision-making. Whether it’s transcribing customer service interactions or creating subtitles for video content, accurate transcription and subtitling are critical to the success of any project. However, understanding the sentiment behind the text is equally important. This is where annotating text for human sentiment comes in.
What is Text Annotation for Human Sentiment?
Text annotation is the process of adding metadata or labels to text to make it more informative and easier to analyse. In the case of sentiment analysis, text annotation involves assigning labels or tags to indicate the emotional tone or sentiment expressed in the text. These labels can be positive, negative, or neutral, depending on the context and language used.
Why is Text Annotation Useful for Transcription and Speech Recognition Technology?
Transcription and subtitling clients can benefit greatly from text annotation for human sentiment for several reasons:
Improved Accuracy: Understanding the sentiment behind the text is crucial for accurate transcription and subtitling. Sentiment analysis helps identify sarcasm, irony, and other linguistic nuances that may be lost in the text without proper annotation.
Faster Turnaround Times: Text annotation can also help speed up the transcription and subtitling process. By using sentiment analysis to identify emotional tone, transcribers and subtitlers can work more efficiently and make decisions quickly.
Better Customer Insights: Text annotation also allows businesses to gain valuable insights into customer sentiment and preferences. By analysing customer interactions and feedback, companies can improve their products and services, resulting in increased customer satisfaction and loyalty.
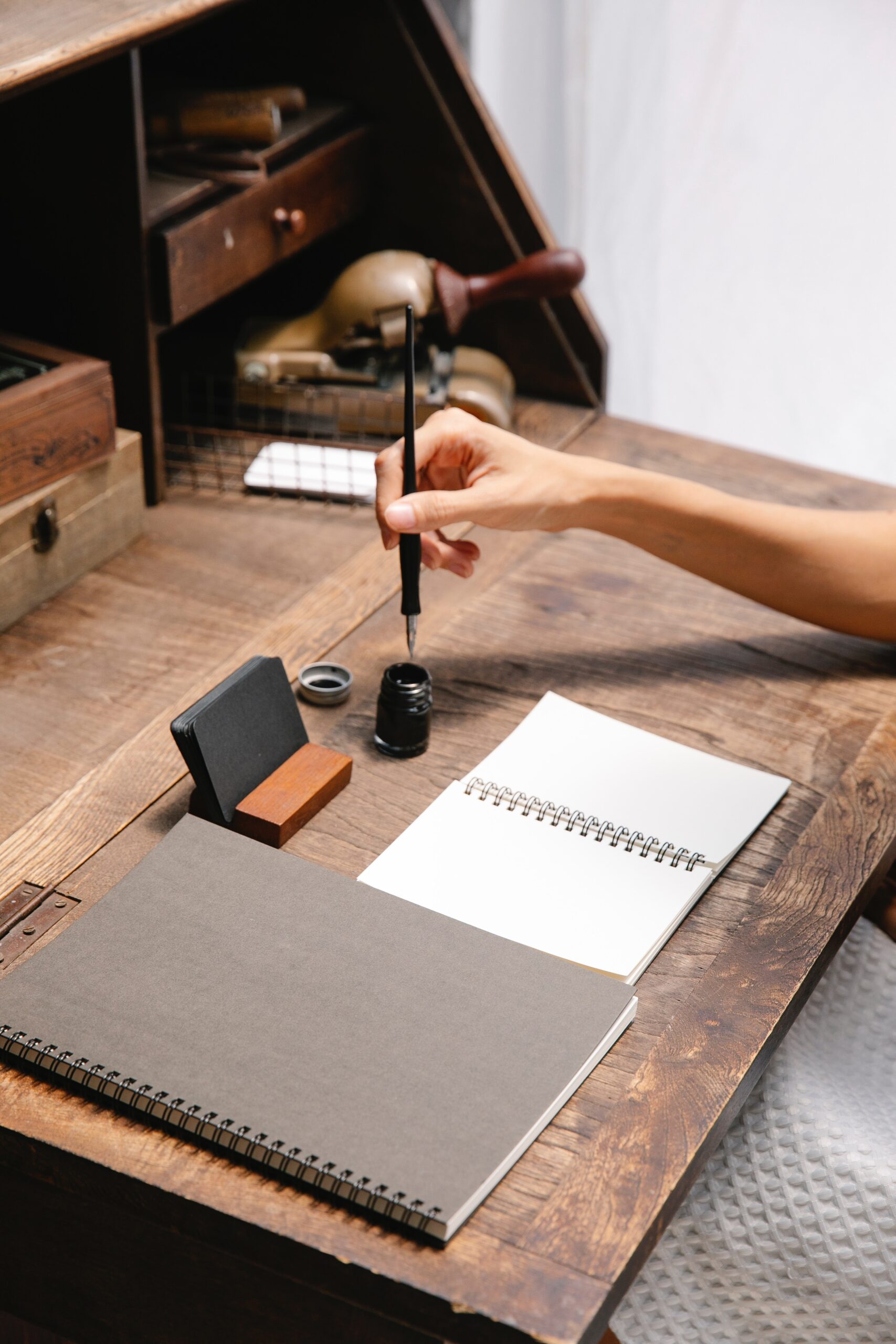
How to Effectively Annotate Text for Sentiment Analysis
To effectively annotate text for sentiment analysis, it’s essential to follow some best practices and guidelines:
Understand the Context: Before annotating text, it’s crucial to understand the context and language used in the text. This includes identifying the intended audience, tone, and style of the text.
Use a Consistent Approach: To ensure accuracy and consistency, it’s essential to use a standardised approach to text annotation. This includes using clear guidelines for assigning labels and ensuring that all annotators are following the same approach.
Consider Cultural Nuances: When annotating text for sentiment analysis, it’s important to consider cultural nuances and language variations. For example, sarcasm and irony may be more prevalent in some cultures than others, and certain words may have different connotations in different languages.
Quality Control: To ensure accuracy and consistency, it’s important to have a quality control process in place. This includes checking for errors and inconsistencies in the annotations and ensuring that all annotators are following the same guidelines.
Continuous Improvement: Text annotation for sentiment analysis is an iterative process. It’s important to continuously review and improve the approach based on feedback and results.
Why Human Annotation is Necessary for Accurate Sentiment Analysis
Automated sentiment analysis tools use algorithms and machine learning models to identify emotional tone and sentiment in text. While these tools have become increasingly sophisticated, they still have limitations when it comes to accurately identifying sentiment. This is where human annotation comes in.
Contextual Understanding: Humans have a deeper understanding of language and context than machines. We are able to recognise sarcasm, irony, and other linguistic nuances that automated tools may miss. For example, consider the following sentence: “Great, I just missed my flight!” An automated sentiment analysis tool may classify this as positive, but a human annotator would recognise the sarcasm and classify it as negative.
Flexibility: Humans can adapt to different language variations and cultural nuances. Language is complex and constantly evolving, with variations in dialect, slang, and colloquialisms. Automated tools may struggle to accurately classify sentiment in texts that use different language variations or that are written in a different cultural context. Human annotators can understand these nuances and adjust their annotations accordingly, resulting in more accurate sentiment analysis.
Customisation: Human annotation allows for customisation and flexibility in the annotation process. Different industries or contexts may require different emotional categories or sentiment labels to be used. For example, in the healthcare industry, emotions such as empathy and concern may be more relevant than in other industries. Human annotators can customise their approach to meet the specific needs of the client or project, resulting in more accurate sentiment analysis.
Quality Control: Human annotation allows for quality control in the annotation process. Annotators can review each other’s work and ensure that the annotations are consistent and accurate. This is important to avoid errors and inconsistencies that can occur when relying solely on automated sentiment analysis tools.
Adapting to New Trends: Humans can adapt to new trends and changes in language use more quickly than machines. For example, new slang terms or emojis may become popular, and human annotators can quickly recognise and adapt to these changes, resulting in more accurate sentiment analysis.
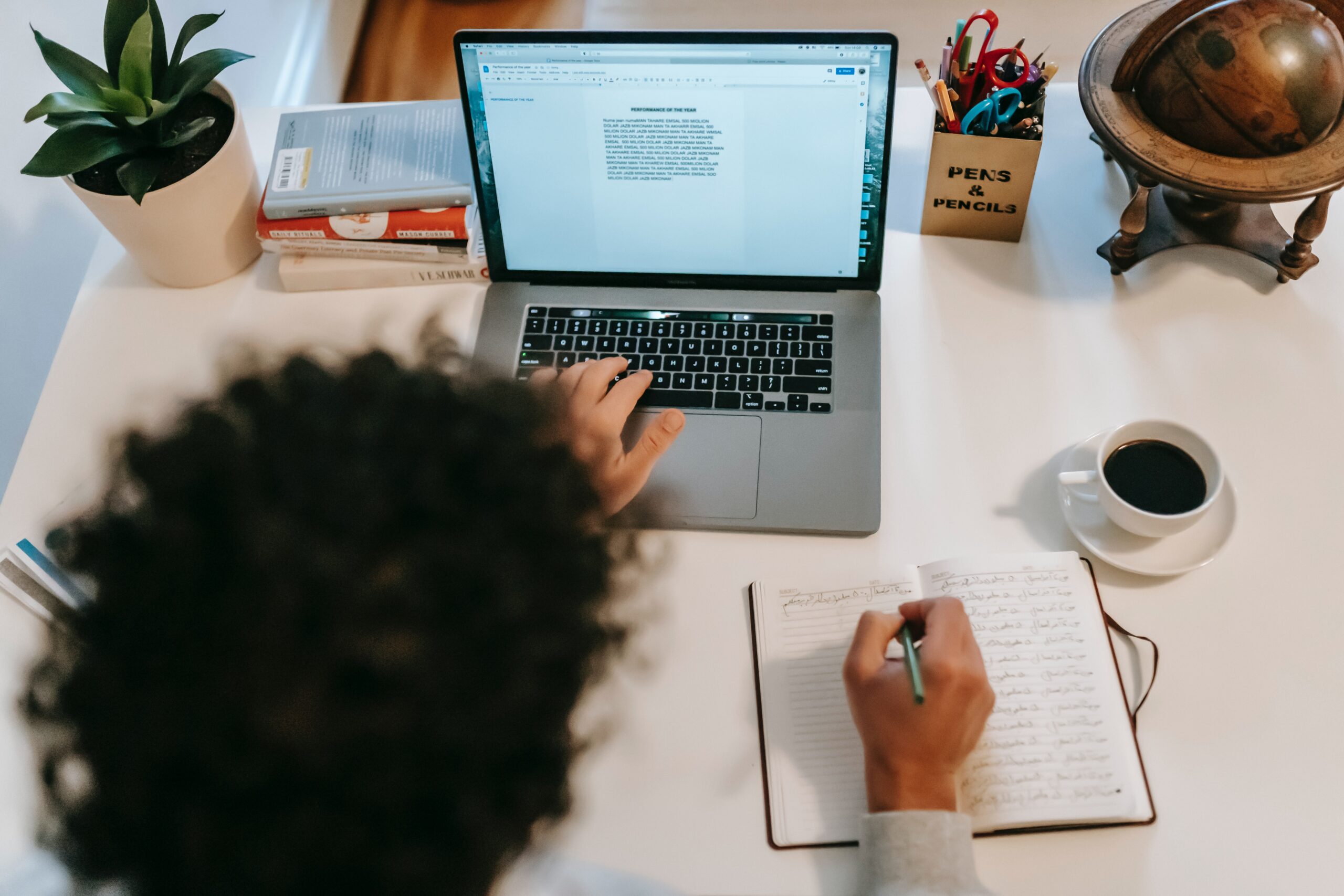
Text annotation for human sentiment is a critical tool for transcription and SRT clients. By accurately identifying emotional tone and sentiment in text, businesses can gain valuable insights into customer sentiment and preferences, improve their products and services, and increase customer satisfaction and loyalty. However, to effectively annotate text for sentiment analysis, it’s essential to follow best practices and guidelines, including understanding the context, using a consistent approach, considering cultural nuances, implementing quality control, and continuously improving the approach. Additionally, while automated sentiment analysis tools are becoming more sophisticated, human annotation remains necessary for accurate sentiment analysis due to humans’ contextual understanding, flexibility, and customisation abilities.
In summary, annotating text for human sentiment is a crucial aspect of transcription and SRT services. It enables businesses to gain deeper insights into customer sentiment and preferences and make data-driven decisions. By following best practices and guidelines for text annotation, businesses can improve the accuracy and efficiency of their transcription and subtitling services and gain a competitive edge in their industry.
Additional Services
About Captioning
Perfectly synched 99%+ accurate closed captions for broadcast-quality video.
Machine Transcription Polishing
For users of machine transcription that require polished machine transcripts.