What is AI Machine Learning and Deep Learning and How Can They Be Used Practically
What is AI Machine Learning and Deep Learning?
Unveiling the Distinctions Between Machine Learning and Deep Learning
What is AI Machine Learning and Deep Learning and why is it important in understanding AI today. In today’s digital era, the fields of machine learning and deep learning have become the cornerstones of technological advancement. These two domains of artificial intelligence (AI) are often used interchangeably, leading to confusion among experts and enthusiasts alike. To shed light on this matter, we delve into the differences between machine learning and deep learning, exploring their unique characteristics, advantages, and limitations. Furthermore, we showcase practical applications of both approaches across various industries.
Defining Machine Learning
Machine learning is a subset of AI that empowers computers to learn and make predictions or decisions without being explicitly programmed. It is a data-driven approach that utilises algorithms and statistical models to extract patterns and insights from data. Machine learning algorithms automatically improve their performance through experience, enabling them to make accurate predictions or take actions based on new, unseen data.
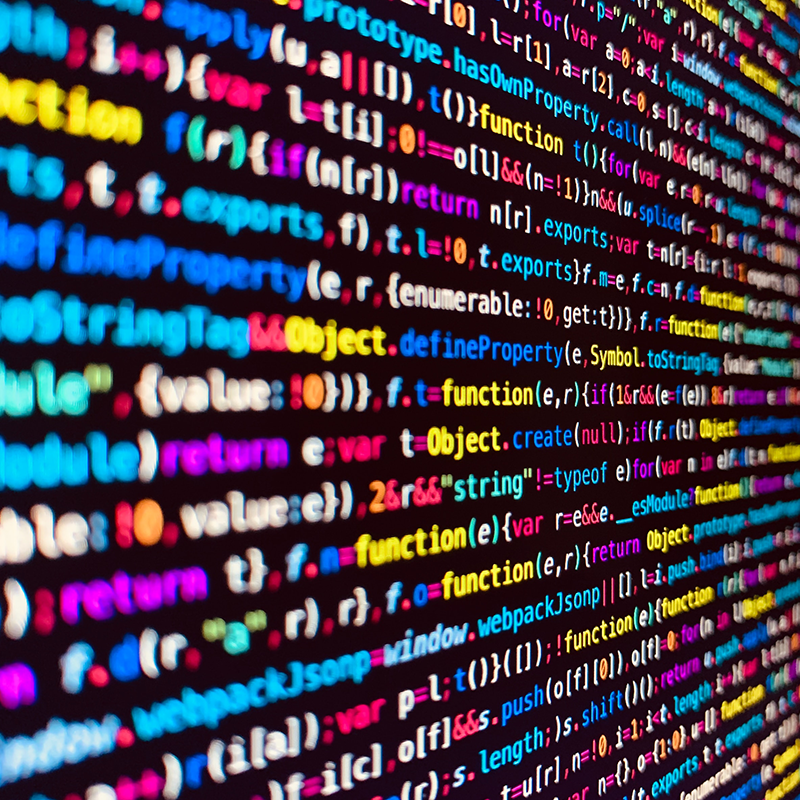
Machine Learning Models Can Be Broadly Categorised Into Three Types
Supervised Learning: In this approach, the machine learning model learns from labelled data, where each data point is associated with a known target output. It aims to generalise patterns from the training data and apply them to make predictions on new, unseen data.
Unsupervised Learning: Here, the model learns from unlabelled data, seeking to discover underlying patterns or structures without specific target outputs. Unsupervised learning is particularly useful for exploratory data analysis and clustering similar data points.
Reinforcement Learning: This learning paradigm involves an agent interacting with an environment and learning from the feedback received through rewards or penalties. The agent explores various actions to maximise its cumulative reward, thus learning an optimal policy over time.
Defining Deep Learning
Deep learning, on the other hand, is a subfield of machine learning inspired by the structure and function of the human brain’s neural networks. It focuses on the development of artificial neural networks with multiple layers of interconnected nodes, known as artificial neurons or perceptron’s. These networks can learn hierarchical representations of data, allowing them to automatically extract intricate features at different levels of abstraction.
Unlike traditional machine learning algorithms that require manual feature engineering, deep learning models have the ability to learn features directly from raw data. The complex architectures of deep neural networks, such as convolutional neural networks (CNNs) and recurrent neural networks (RNNs), enable them to excel in tasks involving images, speech, natural language processing, and sequential data.
Distinguishing Machine Learning from Deep Learning
The primary distinction between machine learning and deep learning lies in the representation and abstraction of data. While machine learning models rely on handcrafted feature engineering, deep learning models learn hierarchical representations of features from raw data. This key difference gives deep learning an advantage in tasks where the underlying patterns are complex and difficult to articulate explicitly.
What are the Advantages?
Advantages of Machine Learning
- Interpretable models: Machine learning algorithms often produce models that are interpretable and offer insights into the decision-making process.
- Efficient for small to medium-sized datasets: Machine learning algorithms can deliver effective results with limited data availability, making them suitable for various applications.
- Computationally efficient: Compared to deep learning, machine learning algorithms often require less computational resources, making them more accessible for certain scenarios.
Advantages of Deep Learning
• Automatic feature learning: Deep learning models learn features directly from raw data, eliminating the need for manual feature engineering and reducing human bias.
• Superior performance on complex tasks: Deep learning excels in tasks involving large-scale data, intricate patterns, and high-dimensional inputs, such as image and speech recognition.
• Scalability: Deep learning models can handle vast amounts of data and scale to large neural network architectures, enabling them to capture intricate relationships.
What are the Limitations?
Limitations of Machine Learning
Data dependency: Machine learning algorithms heavily rely on high-quality and representative data for training. If the available data is insufficient, biased, or of poor quality, it can negatively impact the performance and accuracy of the models. Acquiring and preparing large, diverse, and labeled datasets can be a challenging and resource-intensive task.
Interpretability: Many machine learning algorithms, particularly complex ones like deep neural networks, lack interpretability. They operate as “black box” models, making it difficult to understand how they arrive at their predictions. This lack of transparency can be a significant limitation, especially in critical applications where decision-making needs to be explainable and justifiable.
Ethical considerations and biases: Machine learning models can inadvertently inherit biases present in the training data, leading to biased predictions and discriminatory outcomes. If the training data is unrepresentative or reflects societal biases, the models can perpetuate and amplify those biases. Addressing and mitigating these biases is a critical challenge in deploying fair and unbiased machine learning systems.
Limitations of Deep Learning
Data requirements: Deep learning models typically require a large amount of labelled data to achieve optimal performance. Obtaining labelled data can be costly and time-consuming, especially in domains where annotations are scarce or expensive.
Computationally intensive: Deep learning models often demand significant computational resources, including high-performance GPUs or specialised hardware, to train and deploy. This can pose challenges for organisations with limited computational infrastructure, as they may face constraints in terms of budget and availability of suitable hardware. Training deep learning models can take a considerable amount of time.
Lack of interpretability: The complex architectures and hierarchical representations of deep learning models make it difficult to interpret their internal workings. This lack of transparency can be a concern, especially in domains where explainability is crucial, such as healthcare and finance. It becomes challenging to understand why a deep learning model made a specific prediction or decision, limiting its adoption in critical applications where human interpretability and accountability are essential.
Practical Applications
Machine Learning
Recommendation Systems: E-commerce platforms and streaming services leverage machine learning to provide personalised recommendations based on users’ preferences and behaviour patterns.
Fraud Detection: Machine learning algorithms can analyse large volumes of financial data to identify patterns indicative of fraudulent activities, helping financial institutions mitigate risks.
Natural Language Processing (NLP): Machine learning powers language translation, sentiment analysis, chatbots, and voice recognition systems, enhancing communication and user experiences.
Deep Learning
Computer Vision: Deep learning models have revolutionised image recognition, enabling applications such as autonomous driving, object detection, facial recognition, and medical imaging analysis.
Speech and Audio Processing: Deep learning is extensively used in speech recognition, speaker identification, speech synthesis, and audio classification, enhancing voice assistants and transcription services.
Natural Language Understanding: Deep learning techniques are employed in tasks like machine translation, text generation, sentiment analysis, and question-answering systems, facilitating effective language understanding.
In summary, while machine learning and deep learning are both powerful subfields of artificial intelligence, they differ in their approaches and capabilities. Machine learning relies on handcrafted feature engineering and is suitable for various applications with limited data. Deep learning, on the other hand, automatically learns hierarchical representations of features from raw data, excelling in complex tasks with large-scale data.
Understanding the distinctions between machine learning and deep learning is crucial for experts and practitioners to make informed decisions when applying AI techniques. By leveraging the strengths of both approaches, organisations can harness the potential of AI to drive innovation and address real-world challenges across diverse industries.
With a 21-year track record of excellence, we are considered a trusted partner by many blue-chip companies across a wide range of industries. At this stage of your business, it may be worth your while to invest in a human transcription service that has a Way With Words.
Additional Services
About Captioning
Perfectly synched 99%+ accurate closed captions for broadcast-quality video.
Machine Transcription Polishing
For users of machine transcription that require polished machine transcripts.