What Is Machine Learning vs AI
What is Machine Learning vs. Artificial Intelligence: Understanding the Similarities and Differences
What is machine learning vs AI and why is it important to understand the difference? In today’s rapidly evolving technological landscape, terms like “Machine Learning” (ML) and “Artificial Intelligence” (AI) have gained significant attention. These terms are often used interchangeably, but they are not synonymous. In this blog post, we will explore the similarities and differences between machine learning and artificial intelligence to help you better understand these concepts and their impact on our lives.
Defining Machine Learning
Machine Learning is a subset of artificial intelligence that focuses on training computer systems to learn from data and improve their performance without being explicitly programmed. ML algorithms can analyse and interpret patterns, make predictions, and uncover hidden insights from vast amounts of data. It enables computers to learn and make decisions or predictions based on that acquired knowledge. ML algorithms rely on statistical techniques to automatically learn from and adapt to data, enabling them to improve their performance over time.
Understanding Artificial Intelligence
Artificial Intelligence, on the other hand, is a broader concept that encompasses the development of intelligent machines capable of simulating human intelligence. AI aims to create systems that can perform tasks that typically require human intelligence, such as speech recognition, problem-solving, and decision-making. It involves a combination of various techniques, including machine learning, natural language processing, computer vision, robotics, and more. AI strives to build machines that can reason, learn, and exhibit behaviour similar to human intelligence.
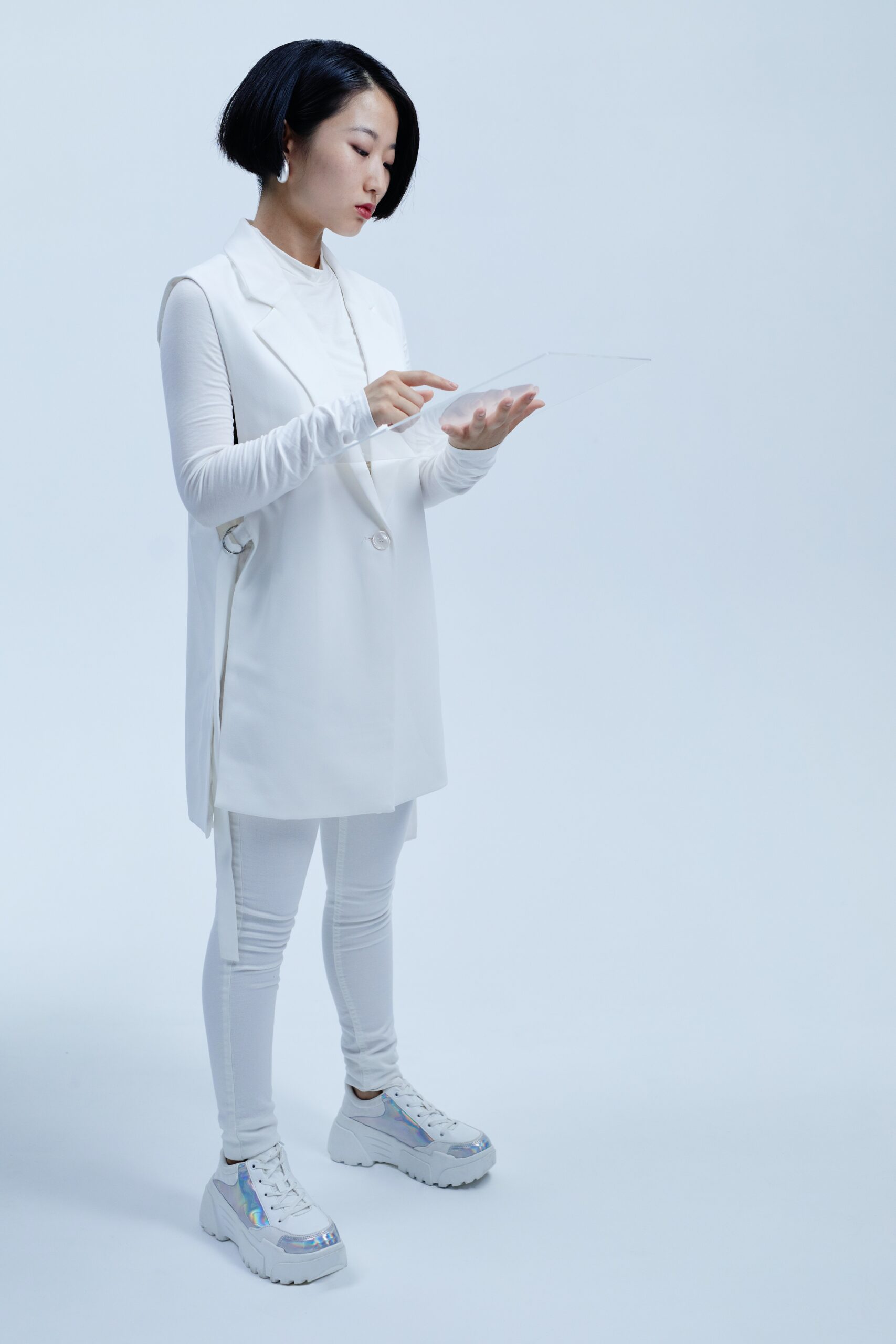
Similarities Between Machine Learning and Artificial Intelligence
Goal-Oriented: Both ML and AI strive to achieve specific goals by processing and analysing data. Whether it’s recognising objects in images or predicting customer behaviour, ML and AI are designed to achieve specific objectives.
Data-Driven: Both ML and AI heavily rely on data for training and decision-making processes. Data serves as the fuel that powers these systems, enabling them to learn, identify patterns, and make informed predictions or decisions.
Iterative Process: Both ML and AI systems continuously learn and improve over time, adapting to new information and experiences. They can update their models and algorithms based on feedback and new data, enhancing their performance and accuracy.
Automation: Both ML and AI technologies automate tasks that would otherwise require human intervention. By automating repetitive or complex tasks, ML and AI systems free up human resources, increase efficiency, and enable humans to focus on more creative and strategic endeavours.
Differences Between Machine Learning and Artificial Intelligence
Scope: Machine Learning is a subset of Artificial Intelligence, focusing on algorithms that learn from data. It is a technique used to achieve AI’s broader goal of replicating human intelligence.
Human-like Intelligence: Artificial Intelligence aims to replicate human intelligence in its entirety, including understanding language, recognising emotions, and exhibiting creativity. Machine Learning, on the other hand, focuses more on pattern recognition and predictive modelling rather than simulating human-like cognition.
Programming vs. Learning: In traditional programming, developers explicitly instruct the computer on how to perform specific tasks by writing detailed code. In contrast, Machine Learning algorithms learn from data without explicit programming. They discover patterns, generalise from examples, and make predictions based on statistical models.
Dependency on Data: Machine Learning algorithms require a large amount of labelled data for training. They learn patterns from this data and use them to make predictions or decisions. In contrast, Artificial Intelligence techniques can incorporate various approaches beyond data analysis, such as rule-based systems, expert knowledge, or symbolic reasoning.
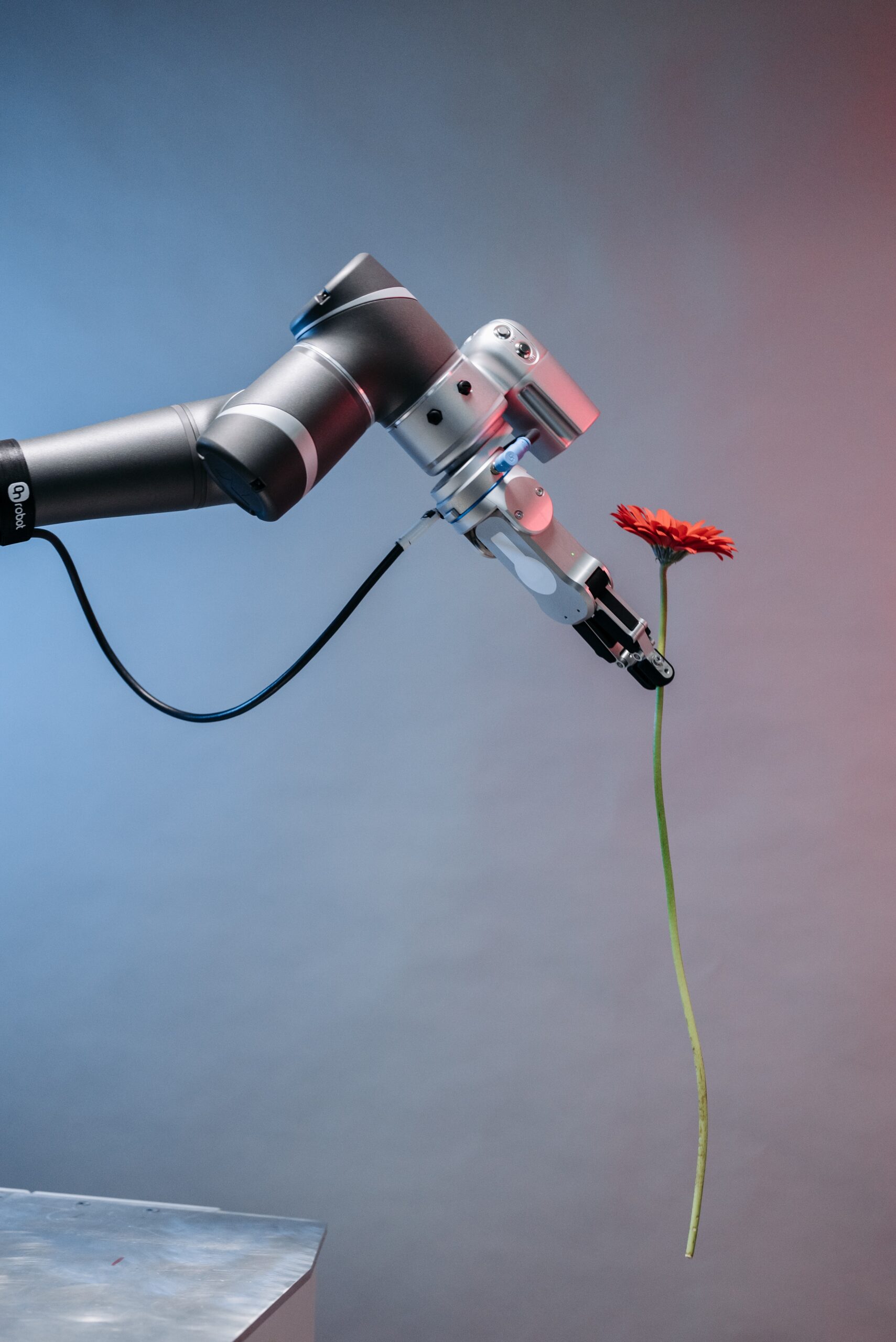
Real-World Examples
To better understand the differences between Machine Learning and Artificial Intelligence, let’s consider a few real-world examples. Suppose we want to build a system that can detect spam emails. Machine Learning algorithms would be used to analyse and classify emails as either spam or non-spam based on historical data. These algorithms would learn from historical data, identifying patterns and characteristics associated with spam emails. As more data is fed into the system, the ML model can continuously improve its accuracy in distinguishing spam from non-spam emails.
On the other hand, an Artificial Intelligence system designed for email classification would go beyond analysing patterns in data. It could utilise natural language processing (NLP) techniques to understand the content and context of emails, detecting spam based on the semantic meaning of the text. The AI system might also consider additional factors such as the sender’s reputation, email metadata, and user preferences to make more informed decisions.
Another example can be found in the field of computer vision. ML algorithms, such as convolutional neural networks (CNNs), are commonly used to analyse and classify images. For instance, a ML model trained on a large dataset of images can learn to recognise objects like cars, dogs, or buildings by identifying key visual features and patterns.
In contrast, an AI system for computer vision might incorporate ML techniques but also go beyond them. It could leverage advanced algorithms and techniques to not only recognise objects but also understand the context of the scene, infer relationships between objects, and even generate descriptions or captions for images.
In conclusion, while Machine Learning and Artificial Intelligence are closely related, they have distinct characteristics and scopes. Machine Learning focuses on training algorithms to learn from data and make predictions or decisions, relying on statistical models and patterns. On the other hand, Artificial Intelligence encompasses a broader range of techniques, aiming to simulate human-like intelligence across various domains.
Understanding the similarities and differences between ML and AI is essential for navigating the ever-evolving landscape of technology. These concepts have the potential to revolutionise industries, improve efficiency, and enhance decision-making processes. By leveraging ML and AI technologies, businesses and individuals can unlock valuable insights from data, automate tasks, and develop intelligent systems that drive innovation.
As technology continues to advance, it’s crucial to stay informed about the latest developments in ML and AI. By gaining a foundational understanding of these concepts, you can actively participate in discussions, make informed decisions, and explore the exciting possibilities that these technologies offer.
With a 21-year track record of excellence, we are considered a trusted partner by many blue-chip companies across a wide range of industries. At this stage of your business, it may be worth your while to invest in a human transcription service that has a Way With Words.
Additional Services
About Captioning
Perfectly synched 99%+ accurate closed captions for broadcast-quality video.
Machine Transcription Polishing
For users of machine transcription that require polished machine transcripts.