What is Text Annotation and How is it Different From Labelling?
What is Text Annotation – A Guide on the Differences between Annotation and Labelling
What is text annotation and labelling? Text annotation and labelling are two key concepts that are essential for the development and optimisation of speech recognition and machine translation systems. Text annotation involves the process of adding metadata or other descriptive information to a text document, while labelling refers to the process of assigning labels or tags to a given set of data. In the context of speech recognition and machine translation, these processes are critical for improving the accuracy and effectiveness of these systems.
Methods of Text Annotation in Speech Recognition
Speech recognition is the process of converting spoken words into written text. In order to do this accurately, a speech recognition system must be able to understand and interpret the sounds of human speech. This involves training the system on large amounts of audio data, which must be annotated and labelled in order to be useful.
One common method of text annotation in speech recognition is phonetic transcription. This involves transcribing spoken words into a written format using a system of phonetic symbols, which represent the sounds of human speech. Phonetic transcription allows researchers to analyse and compare the sounds of different languages and can help to improve the accuracy of speech recognition systems by providing a standardised way of representing speech sounds.
Another method of text annotation in speech recognition is the use of language models. Language models are statistical models that can be trained on large amounts of text data in order to predict the likelihood of different words or phrases occurring in a given context. Language models are used in speech recognition to help the system understand the meaning of spoken words and phrases, and to improve the accuracy of transcription.
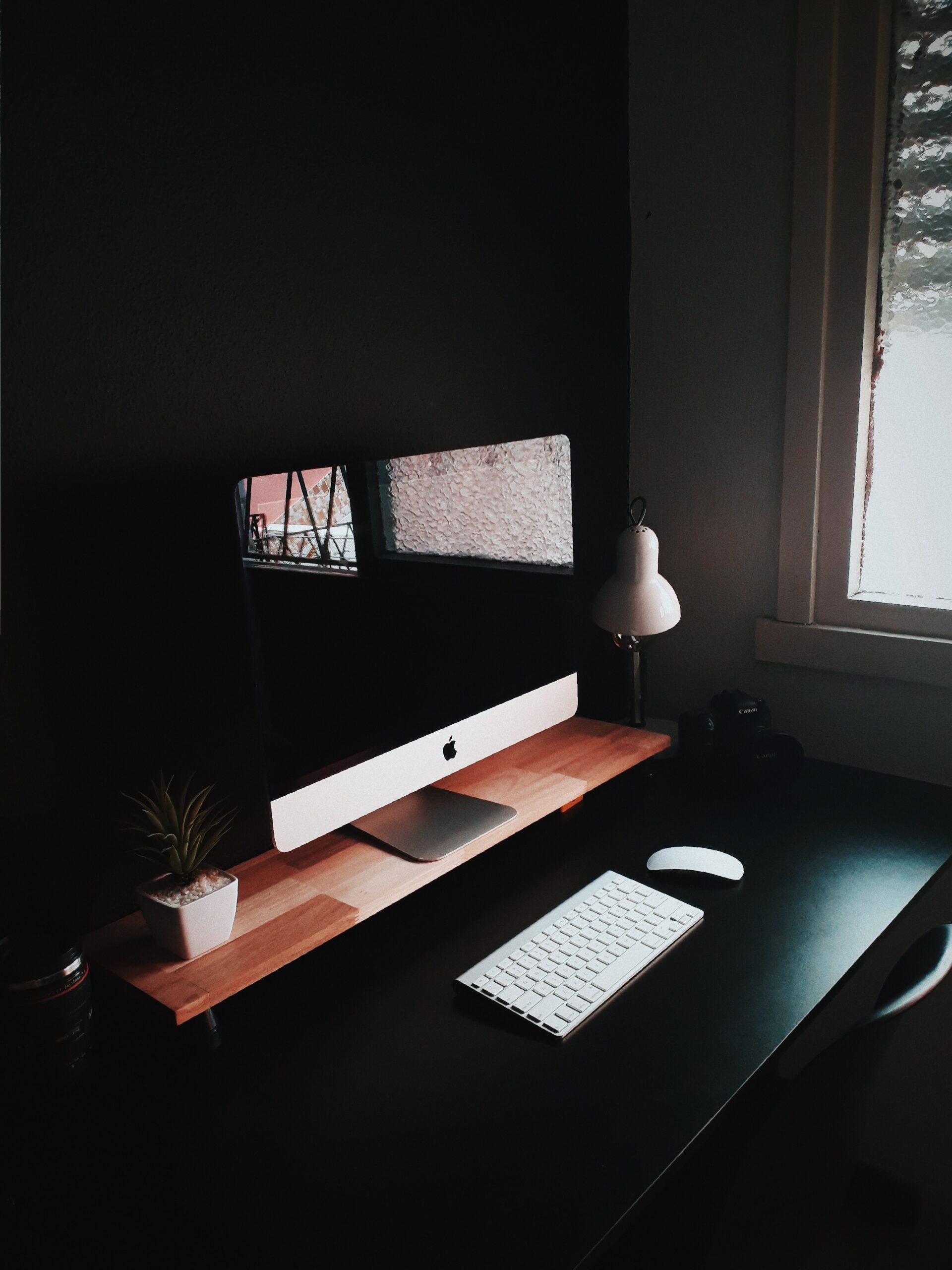
Methods of Labelling in Speech Recognition
Labelling is another important concept in the field of speech recognition. In order to train a speech recognition system, large amounts of audio data must be labelled with information about the words and sounds that are being spoken. This allows the system to learn the patterns and characteristics of different languages, and to recognise words and phrases more accurately.
One common method of labelling in speech recognition is the use of automatic speech recognition (ASR) systems. These systems use machine learning algorithms to automatically transcribe spoken words into text and can be trained on large amounts of audio data in order to improve their accuracy over time. ASR systems can also be used to label audio data with information about the speaker’s accent, tone, and other characteristics.
Text Annotation in Machine Translation
Machine translation is another important area where text annotation and labelling play a critical role. Machine translation is the process of automatically translating written text from one language to another. In order to do this accurately, a machine translation system must be trained on large amounts of text data in both the source and target languages. This data must be annotated and labelled in order to be useful for machine translation.
One common method of text annotation in machine translation is the use of parallel corpora. A parallel corpus is a collection of text data in two or more languages that have been translated into each other. This allows researchers to compare and analyse the linguistic features of different languages, and to develop machine translation systems that can accurately translate between them.
Another method of text annotation in machine translation is the use of named entity recognition (NER). NER involves identifying and categorising named entities, such as people, places, and organisations, in a given text document. This can help to improve the accuracy of machine translation systems by providing additional context and information about the text being translated.
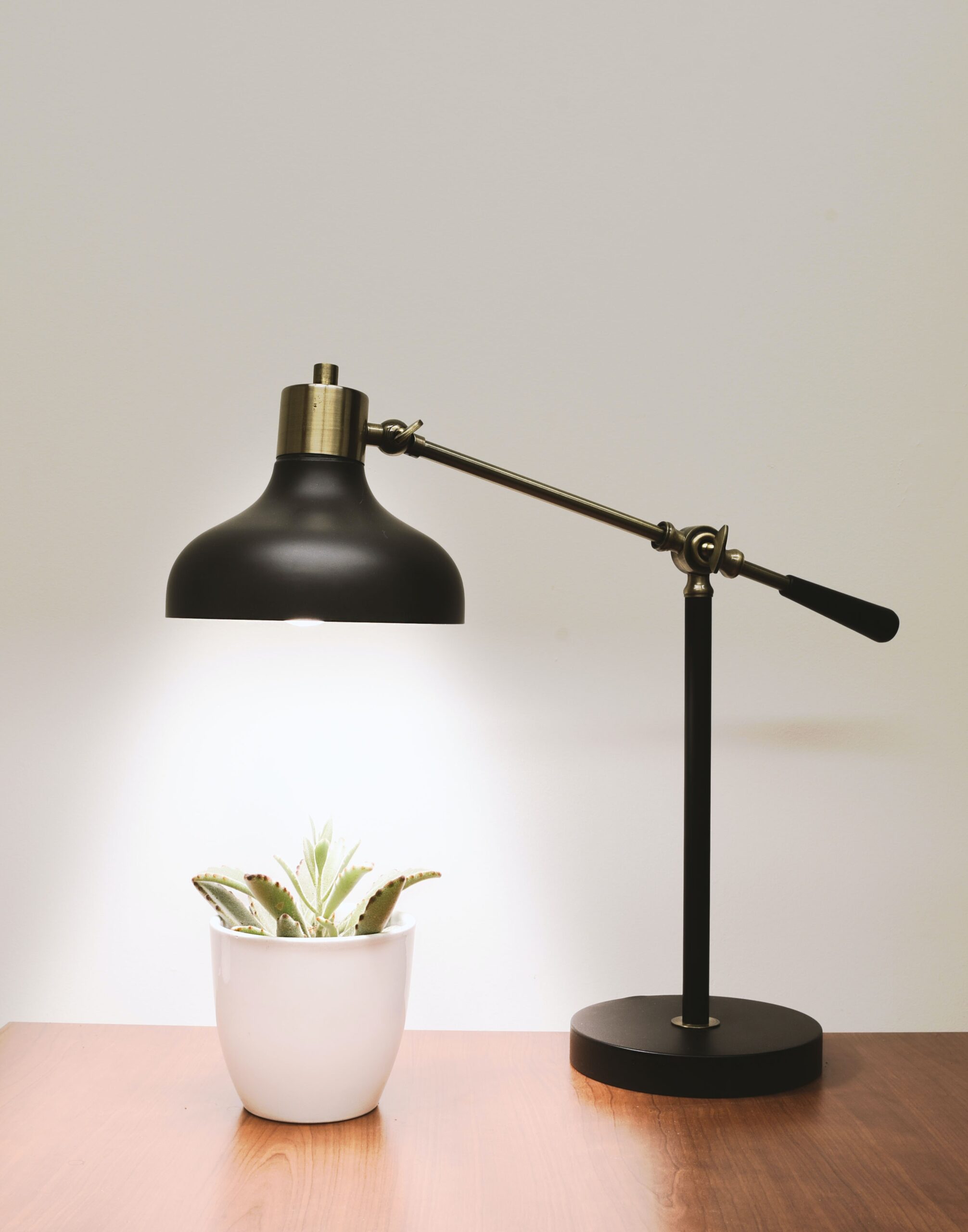
Labelling in Machine Translation
Labelling is also an important concept in machine translation. In order to train a machine translation system, large amounts of text data must be labelled with information about the source and target languages, as well as any relevant linguistic features. This allows the system to learn the patterns and structures of different languages, and to accurately translate between them.
One common method of labelling in machine translation is the use of translation memory (TM) systems. These systems store previously translated text segments in a database and can be used to automatically translate similar text segments in the future. TM systems can be trained on large amounts of text data, which can be annotated and labelled with information about the source and target languages, as well as any relevant linguistic features. This allows the system to learn the patterns and structures of different languages, and to accurately translate between them.
Challenges of Text Annotation and Labelling in Speech Recognition
Despite the many benefits of text annotation and labelling in speech recognition and machine translation, there are also several challenges associated with these processes. One of the biggest challenges is the need for high-quality annotated and labelled data. This can be difficult to obtain, especially for languages that are not widely spoken or for specialised domains, such as medical or legal terminology.
Another challenge is the need for standardisation in annotation and labelling practices. Different researchers and organisations may use different annotation and labelling schemes, which can make it difficult to compare and combine data from different sources. Additionally, annotation and labelling can be time-consuming and labour-intensive, which can be a significant bottleneck in the development of speech recognition and machine translation systems.
Despite these challenges, text annotation and labelling remain essential for the development and optimisation of speech recognition and machine translation systems. These processes allow researchers and developers to better understand and analyse the characteristics of different languages, and to train systems that can accurately transcribe and translate between them. With the continued development of new tools and methods for annotation and labelling, the accuracy and effectiveness of speech recognition and machine translation systems are likely to continue to improve in the years to come.
With a 21-year track record of excellence, we are considered a trusted partner by many blue-chip companies across a wide range of industries. At this stage of your business, it may be worth your while to invest in a human transcription service that has a Way With Words.
Additional Services
About Captioning
Perfectly synched 99%+ accurate closed captions for broadcast-quality video.
Machine Transcription Polishing
For users of machine transcription that require polished machine transcripts.