Can AI Language Models Speak Multiple Languages?
Exploring the Multilingual Capabilities of AI Language Models
In an ever-globalising world, the ability of artificial intelligence (AI) to understand and converse in multiple languages is a subject of increasing importance and curiosity. This capability, often embedded in large language models, is transforming how industries and individuals communicate across linguistic barriers.
As we delve into this topic, key questions emerge: Which languages are AI models proficient in? How effectively can they translate or converse in these languages? And, critically, how advanced are these models in understanding the nuances of different languages? These questions are pivotal for data scientists, technology entrepreneurs, software developers, and industries leveraging AI to enhance communication and language databases through machine learning.
Key Aspects of AI Language Models
Language Diversity in AI Language Models
AI language models are increasingly supporting a wide range of languages, from widely spoken ones like English and Mandarin to less common languages. The diversity in language support enhances the global applicability of these models, making them indispensable tools in international communication.
The realm of AI language models has witnessed a notable expansion in linguistic diversity, transcending boundaries that once limited technology. Today, these models proficiently cater to a spectrum of languages, ranging from global lingua francas such as English and Mandarin to regional and even endangered languages. This inclusivity not only democratises access to technology but also fosters a deeper cultural connection and understanding. For instance, the integration of indigenous languages in AI models not only preserves linguistic heritage but also empowers communities by providing them with access to modern technology in their native tongue.
Moreover, this linguistic inclusivity in AI language models is not just a matter of adding new languages to a list. It involves deep cultural immersion, understanding dialects, regional variations, and even the socio-linguistic contexts within which these languages operate. For businesses and services operating on a global scale, this diversity means being able to reach and communicate with a broader audience in a more personalised way. It heralds a new era of truly global digital inclusivity, where language is no longer a barrier but a bridge connecting diverse communities.
Translation Accuracy and Contextual Understanding
High translation accuracy and contextual understanding are hallmarks of advanced AI language models. These models are not just translating words but are also capturing cultural nuances and contextual meanings, thus making interactions more meaningful and accurate.
Advanced AI language models are redefining the parameters of translation, elevating it from mere word-to-word conversion to an art that encapsulates the essence of communication. These models are intricately designed to comprehend and translate not just the language but the context, cultural subtleties, and emotional undertones embedded within the text. This capability ensures that the translated content is not only linguistically accurate but also culturally resonant and contextually appropriate. For example, in literary translations, AI models are increasingly capable of preserving the author’s original tone, style, and intent, thereby maintaining the integrity of the original work.
This sophistication in translation is achieved through advanced algorithms and deep learning techniques that enable AI models to learn from a multitude of texts, including literature, colloquial conversations, and technical documents. By doing so, these models develop an understanding of language that goes beyond the surface level, capturing nuances such as sarcasm, idioms, and even regional humour. This evolution in AI-powered translation is a boon for global communication, ensuring that language barriers do not impede the free flow of ideas and information across borders.
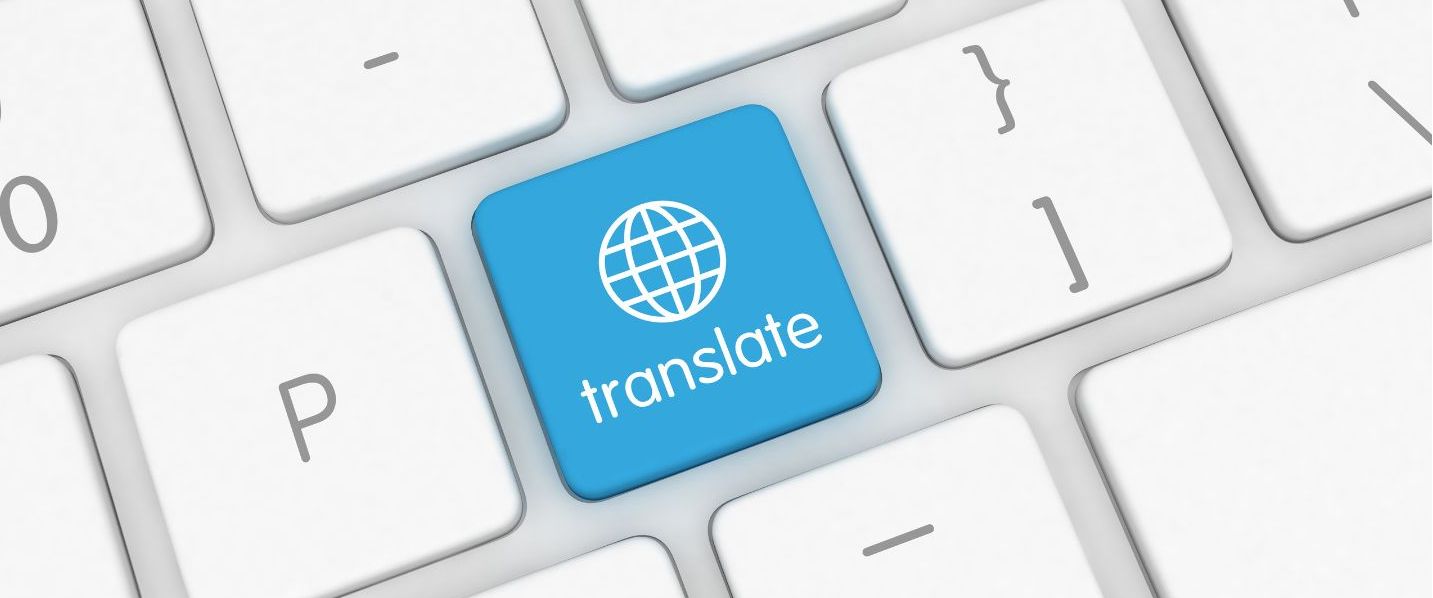
Speech Recognition and Processing
AI’s capability in speech recognition has seen remarkable progress, enabling the conversion of spoken language into text across various languages. This advancement supports real-time communication and assists in creating inclusive technologies for diverse linguistic groups.
The advancement in AI-driven speech recognition and processing is a monumental leap in bridging the gap between human interaction and machine interpretation. Today’s AI models can accurately transcribe and understand spoken language in real-time, across a diverse range of dialects and accents. This capability is crucial in creating technologies that are accessible to everyone, regardless of their language or accent. For example, voice-activated assistants, once limited to a few major languages, now understand and respond to a multitude of languages, making them more useful to a broader audience.
Furthermore, the impact of advanced speech recognition extends beyond convenience to empowerment. It plays a pivotal role in accessibility, aiding individuals with disabilities by providing them with tools to interact with technology using their voice. In educational settings, this technology supports language learning, enabling students to receive immediate feedback on pronunciation and fluency. The continuous improvements in speech recognition technology promise a future where interactions with AI are as natural and effortless as human conversation.
Natural Language Processing (NLP) in Multilingual Contexts
NLP is at the core of AI’s language capabilities, enabling machines to understand, interpret, and respond to human language in a way that is both natural and contextually relevant, across multiple languages.
NLP is the cornerstone of AI’s linguistic capabilities, endowing machines with an almost human-like ability to parse and understand language. In multilingual contexts, NLP enables AI to not just process text in different languages but to understand the cultural and contextual layers that language encompasses. This deep understanding is crucial in scenarios where the meaning goes beyond the literal words, such as in idiomatic expressions or when language is used figuratively.
The application of NLP in multilingual contexts is vast and varied. In customer service, AI-powered chatbots equipped with NLP can provide assistance in multiple languages, offering personalised and contextually relevant responses. In the realm of social media, NLP enables platforms to monitor and understand conversations in various languages, helping in content moderation and enhancing user experience. The evolution of NLP in handling multiple languages is a testament to the potential of AI in creating a more connected and understanding world.
Challenges in Language Nuances and Idioms
AI models sometimes struggle with understanding idioms, slang, and cultural nuances, which can lead to misinterpretations. Continuous improvements and learning from vast datasets are helping to overcome these challenges.
One of the most daunting challenges facing AI models in language processing is the accurate interpretation of nuances, idioms, and colloquialisms. These elements of language are often deeply rooted in culture and history, making them difficult for AI to decipher without context. Misinterpretations or literal translations of idioms can lead to confusion, miscommunication, or even offense. For instance, an idiom that is considered humorous in one culture might be nonsensical or inappropriate in another.
To mitigate these challenges, AI models are being trained on vast and diverse datasets that include not just standard language but also colloquial and idiomatic expressions. This training is coupled with advancements in contextual analysis, enabling AI to discern when an expression is used literally or figuratively. Despite these efforts, the complexity of human language means that this remains an area of ongoing research and development. The goal is to create AI models that are not just linguistically adept but also culturally aware and sensitive.
Continuous Learning and Model Training
AI language models are continuously learning from a vast array of text and speech data, becoming more proficient and nuanced in their language capabilities. This ongoing training is crucial for the models to remain effective and accurate.
The evolution of AI language models is an ongoing process, driven by continuous learning and iterative training. As language is dynamic and constantly evolving, AI models must adapt to changes in usage, slang, and even the emergence of new dialects. This adaptability is achieved through the analysis of vast datasets, encompassing everything from contemporary literature to social media posts. By continually learning from such diverse sources, AI models stay up-to-date and relevant.
This continuous training is not just about keeping pace with linguistic changes. It’s also about refining the accuracy and sophistication of the models. Every interaction, every piece of feedback, and every new data point contributes to the model’s learning, making it more nuanced and capable. In industries where communication is key, such as customer service or translation services, this ongoing refinement is critical in maintaining and improving the quality of service.
Language-Specific Models vs. Universal Models
There’s a debate between developing language-specific models that focus on the nuances of a single language versus universal models that cover multiple languages but may lack deep understanding in each.
The debate between developing language-specific models and universal models is central to the advancement of AI language capabilities. Language-specific models are tailored to the intricacies of a particular language, offering depth and accuracy in understanding and translation. These models are particularly effective in capturing the nuances, idioms, and cultural references unique to a language. However, the drawback is the resource-intensive nature of developing and maintaining individual models for each language.
On the other hand, universal models aim to cover multiple languages within a single framework. The advantage of this approach is its scalability and the ability to provide a broad range of language support. However, the challenge lies in ensuring that these models maintain a high level of accuracy and cultural sensitivity across all the languages they cover. The ideal approach may involve a hybrid model that combines the breadth of universal models with the depth of language-specific models, offering a balanced solution that caters to both global reach and local relevance.
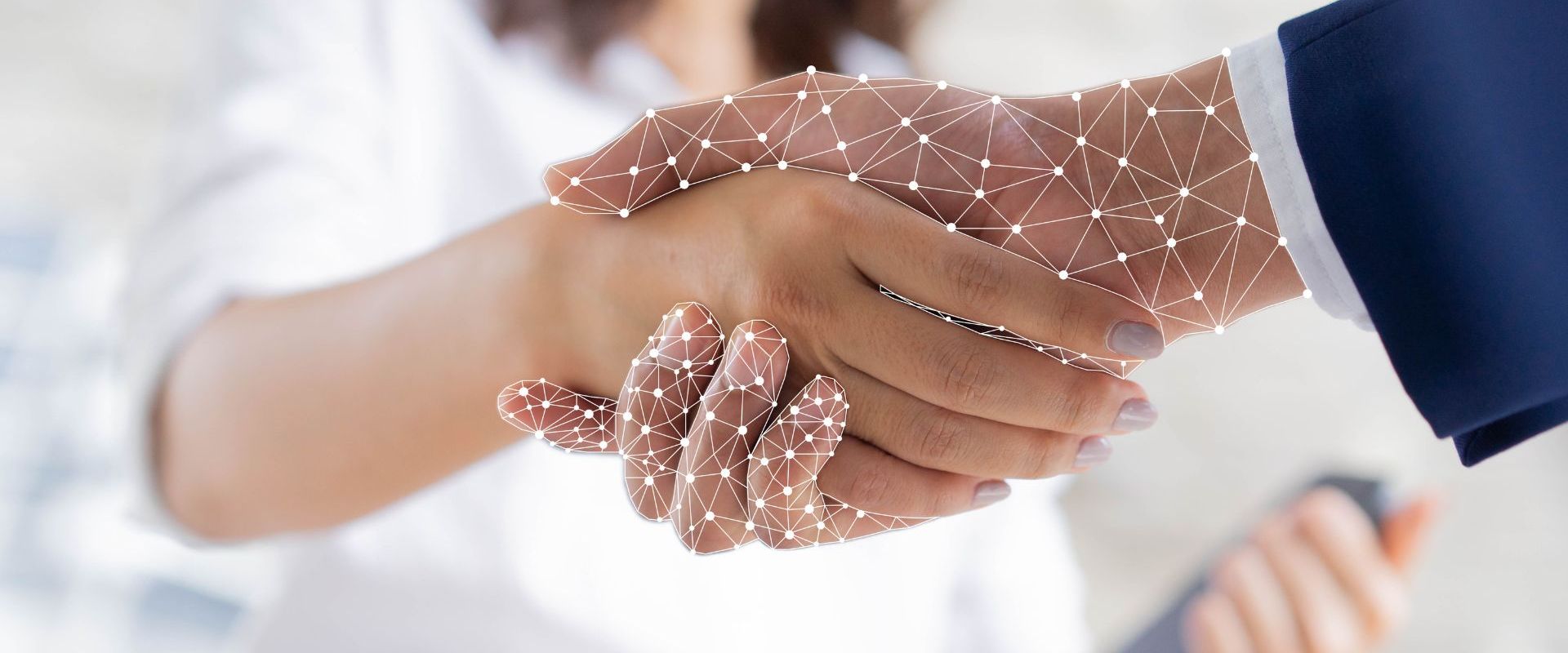
Impact on Global Business and Communication
The multilingual capabilities of AI are breaking down language barriers in global business and communication, enabling smoother interactions and opening up new markets.
The multilingual capabilities of AI are revolutionising global business and communication, breaking down barriers that have historically impeded cross-cultural interaction. In the business realm, AI-powered translation and communication tools enable companies to operate in multiple markets without the language barriers that once limited expansion. This global reach is not only beneficial for large corporations but also for small and medium-sized enterprises, which can now access international markets more easily.
In the realm of communication, AI’s multilingual capabilities foster a greater understanding and connection between people of different linguistic backgrounds. Whether it’s through social media, online forums, or international conferences, AI-powered translation and interpretation tools are facilitating conversations that were once hindered by language barriers. This newfound ease of communication is not just enhancing business interactions but also fostering cultural exchange and understanding on a global scale.
Ethical Considerations and Bias in AI Language Models
Ethical considerations, such as bias in AI language models, are crucial. Ensuring that AI models are unbiased and equitable across different languages is a significant focus in their development.
Ethical considerations, particularly concerning bias in AI language models, are critical in the development of AI. Biases in AI can manifest in various forms, from gender bias to cultural insensitivity, often stemming from the datasets used to train these models. For instance, if a model is trained predominantly on data from a particular region or demographic, it may not accurately represent or understand the language use of other groups. This can lead to misinterpretations or even the perpetuation of stereotypes and biases.
To address these issues, it is essential to ensure that the datasets used for training AI language models are diverse and representative of the different ways in which language is used across cultures and communities. Moreover, ongoing monitoring and refinement are crucial in identifying and correcting biases that may emerge over time. Ethical AI development also involves transparency in how models are trained and used, as well as ensuring that they are accessible and equitable across different languages and communities.
Future Prospects and Innovations
The future of AI in language promises more seamless communication, better understanding of cultural contexts, and more robust support for less common languages, driving forward global connectivity.
The future of AI in language is poised to further transform how we communicate and interact across linguistic boundaries. One exciting prospect is the development of AI language models that can not only translate but also interpret the emotional and cultural context of communication. This advancement would enable more empathetic and nuanced interactions, bridging not just language gaps but also cultural differences.
Another area of innovation is the support for less commonly spoken languages, which are often underrepresented in technology. By developing AI language models that can understand and interact in these languages, technology becomes more inclusive, preserving linguistic diversity and providing access to digital resources for a broader range of people. The continuous evolution of AI in language promises a future where communication is more inclusive, empathetic, and effective, transcending the barriers of language and culture.
Key Tips For Using Multi-Language Models in AI
- Utilise diverse and comprehensive datasets to train AI language models for better understanding and accuracy across multiple languages.
- Focus on continuous model training and updates to keep up with the evolving nature of languages and their usage.
- Prioritise ethical considerations and strive to eliminate biases in AI language models.
- Balance the development between language-specific and universal models to ensure depth and breadth in language understanding.
- Leverage advancements in NLP and speech recognition to enhance AI’s multilingual capabilities.
- Acknowledge and address challenges like idiomatic expressions and cultural nuances for more accurate translations and interactions.
- Explore AI’s potential in breaking language barriers in global business and communication sectors.
- Stay updated with innovations and advancements in AI language technologies to remain competitive and effective.
- Utilise services like Way With Words for highly customised and appropriate data collections, enhancing AI language and speech developments.
The exploration of AI’s multilingual capabilities reveals a landscape rich in potential and challenges. From enhancing global communication to advancing technological frontiers, the proficiency of AI in multiple languages is a testament to the remarkable strides made in machine learning and natural language processing.
However, the journey is not without its hurdles. Issues like language nuances, idiomatic expressions, and cultural differences highlight the need for continuous improvement and ethical vigilance in AI development. As we advance, the integration of customised and targeted data collections, like those offered by Way With Words, becomes increasingly vital. These collections not only feed the ever-growing appetite of AI language models for quality data but also ensure that these models evolve in a way that is nuanced, culturally aware, and globally relevant.
Multilingual Large Language Models Resources
Speech Data Collection: Way With Words creates speech datasets including transcripts for machine learning purposes. Their service is used for technologies looking to create or improve existing automatic speech recognition models (ASR) using natural language processing (NLP) for select languages and various domains.
Machine Transcription Polishing: Way With Words offers machine transcription polishing (MTP) services for a variety of AI and machine learning purposes. Applications include machine learning models that use speech-to-text for AI research, FinTech/InsurTech, SaaS/Cloud Services, Call Centre Software, and Voice Analytic services for customer journey analysis.
The State of Multilingual AI: Ruder.io takes a closer look at the state of multilingual AI. How multilingual are current models in NLP, computer vision, and speech? What are the main recent contributions in this area? What challenges remain and how we can we address them?