AI Language Processing: 10 Key Limitations
The Limitations of AI Language Processing
With technological advancements, Artificial Intelligence (AI) has made significant strides in language processing. However, like any technology, AI has its limitations, especially in understanding the complexities of human language. For professionals in data science, technology entrepreneurship, software development, and industries leveraging AI for communication enhancements, it’s vital to recognise these limitations.
The key questions to consider include: How well does AI understand context in conversation? Can it grasp the nuances and idioms unique to different languages? How does AI handle evolving language trends, including slang? Addressing these questions is crucial for anyone relying on AI for language processing, whether in developing chatbots, language translation services, or voice recognition software.
Quality data is the cornerstone of effective AI language processing. The calibre of data collected directly influences an AI system’s ability to perform accurately and efficiently. Data scientists and developers must ensure that their datasets are not only large and varied but also of the highest quality to advance the technology’s capabilities.
10 Key Limitations in AI Language Processing
#1 Understanding Language Context
One of the most significant challenges for AI in language processing is understanding context. Words or phrases can have different meanings based on the situation or the speaker’s intent. AI systems often struggle to decipher these subtleties, leading to misunderstandings or inaccurate interpretations. For instance, the phrase “I’m feeling blue” could indicate sadness or refer to the colour of a person’s clothing, depending on the context.
The challenge of context comprehension in AI language processing is akin to navigating a labyrinth with ever-shifting walls. Words and phrases, like chameleons, morph their meanings based on the subtlest of contexts. For AI, this is a formidable challenge. The phrase “I’m feeling blue” might denote melancholy in one instance and a preference for the colour in another.
The complexity deepens when you consider linguistic subtleties like irony, metaphor, or cultural references, which can entirely alter the meaning of a sentence. AI systems must be trained on a wide array of contexts to understand these shifts. However, this is easier said than done, as the requisite level of nuanced understanding often requires a degree of worldly knowledge and experience that AI currently lacks.
Moreover, contextual understanding in AI is not just about deciphering the direct meaning of words but also involves interpreting the speaker’s intent, which can be incredibly nuanced. The same sentence can convey different messages depending on the speaker’s tone, the situation’s context, or even the relationship between the speaker and the listener. For instance, a sarcastic remark might be understood as genuine praise without the contextual understanding of sarcasm. These subtleties present a significant hurdle in developing AI systems that can interact naturally with humans, as they must not only process language but also understand the intricate web of human interactions and social norms.
#2 Grasping Nuances and Idioms
AI systems frequently stumble over the nuances and idioms of language. Idioms, in particular, pose a challenge as they are expressions whose meanings cannot be inferred from the meanings of the words that make them up. For AI to effectively process these, it must not only translate words but also understand cultural implications and non-literal meanings.
AI’s struggle with the nuances and idioms of language is a significant barrier to achieving true linguistic fluency. Idioms, colloquialisms, and regional expressions represent a rich tapestry of language that often defies literal interpretation. For instance, “spilling the beans” or “kicking the bucket” are phrases whose meanings cannot be deduced from the words alone.
This linguistic complexity requires an AI to have not just a database of words and their meanings but also an understanding of cultural context and historical usage. The challenge compounds when idioms from different languages and cultures are brought into the mix, each with its unique background and usage.
Developing an AI capable of processing these idioms requires a deep dive into cultural studies, making it necessary for AI systems to be informed by a diverse range of linguistic and cultural inputs. Even then, the idiomatic expressions pose a unique challenge due to their often humorous, ironic, or sarcastic nature, which can be difficult to quantify and encode into AI algorithms. This complexity leads to a scenario where AI might be able to translate words accurately but still miss the mark in conveying the intended message or sentiment, especially in informal or creative language use.
#3 Language Evolution and Slang
Languages are continually evolving, and slang terms often emerge and change rapidly. AI systems need to be updated regularly to keep up with these changes. The challenge lies in the speed and diversity of language evolution, especially across different demographics and regions.
The rapid evolution of language and the proliferation of slang present a moving target for AI language processing. Slang, in particular, is a linguistic phenomenon that constantly evolves, with new terms and phrases emerging from various subcultures and social groups. This dynamism reflects the vibrant and ever-changing nature of human language, but it poses a significant challenge for AI. To keep up, AI systems need continual updates with the latest linguistic developments. However, the speed at which slang terms emerge and change can outpace the data collection and training processes.
Additionally, the contextual and often localised nature of slang means that an AI system trained predominantly on standard language or data from specific regions might be completely baffled by slang from different areas or demographics. For instance, a slang term popular in one age group or geographical area might be unknown or have a different meaning in another. This linguistic diversity requires AI systems not only to have extensive and up-to-date datasets but also to understand the cultural and demographic context in which language is used.
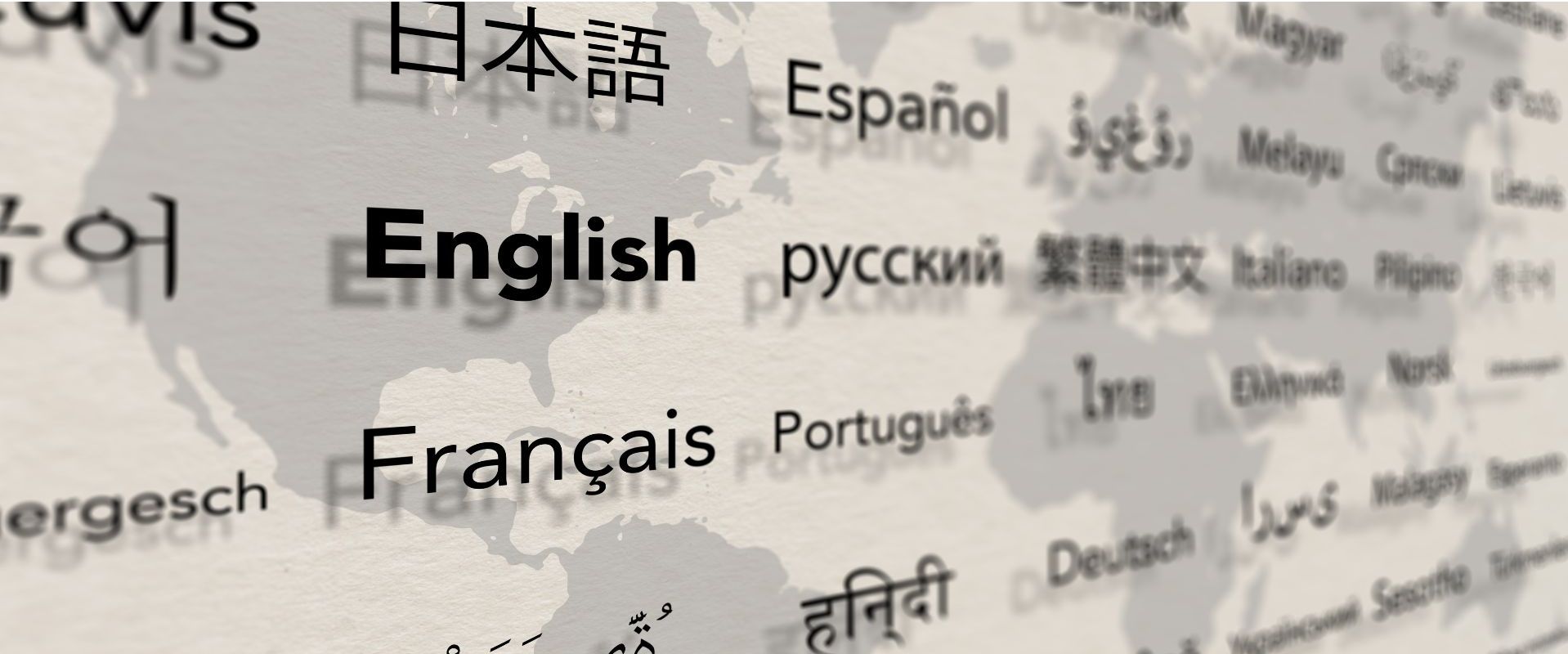
#4 Cultural and Regional Differences
Language is deeply rooted in culture, and regional differences can significantly impact meaning and interpretation. AI must be able to recognise and adapt to these differences to avoid misinterpretations. For instance, the same word might have entirely different meanings or connotations in different cultures.
Cultural and regional differences in language use are a significant obstacle in AI language processing. Language, inherently tied to culture, varies dramatically across different regions and communities. These variations are not just in vocabulary but also in syntax, semantics, and pragmatics. For AI to effectively process language from different cultures, it must have a deep understanding of these variations and the cultural context behind them. For instance, a word or phrase that is innocuous in one culture might be offensive or have a completely different meaning in another.
To address these differences, AI systems require training on diverse datasets that encompass a wide range of languages, dialects, and cultural contexts. However, this is a monumental task, as it involves not only collecting extensive data but also understanding the intricacies of cultural norms, idiomatic expressions, and contextual cues unique to each language and region. This complexity is further compounded when AI encounters languages or dialects with limited available data or those that are significantly different from the more widely spoken languages.
#5 Emotion and Tone Interpretation
AI’s ability to interpret emotion and tone in spoken or written language is limited. While some advances have been made, accurately detecting subtle emotional cues remains a challenge. This limitation impacts the effectiveness of AI in scenarios like customer service, where understanding emotional context is crucial.
Interpreting emotion and tone is a realm where AI still lags significantly behind human capabilities. The subtlety of emotional expression in language is a complex code that AI struggles to decipher. Emotions are often conveyed through subtle cues such as word choice, sentence structure, intonation, and even pauses in speech.
AI systems, although improving, still find it challenging to accurately interpret these cues, especially in nuanced or complex emotional expressions. This limitation becomes particularly evident in customer service interactions or therapeutic settings, where understanding the emotional context is as important as understanding the spoken words.
Advancements in natural language processing (NLP) and machine learning have led to some progress in this area, but the ability of AI to understand and respond to human emotions with the same depth and sensitivity as a human remains a distant goal. Emotional interpretation in language is not just about recognising specific words associated with emotions but also involves understanding the broader context, the relationship between the speakers, and the underlying social and cultural norms. This complexity requires a multifaceted approach to AI development that goes beyond mere linguistic analysis.
#6 Irony and Sarcasm Detection in AI Language Processing
Irony and sarcasm are complex language forms that AI often fails to detect. These forms of speech rely heavily on context, tone, and sometimes cultural knowledge, making them particularly challenging for AI systems to interpret accurately.
Irony and sarcasm detection in language processing is akin to navigating a minefield for AI. These forms of speech are particularly challenging because they often involve saying the opposite of what is meant, requiring an understanding of not just the literal words but the speaker’s intent, tone, and the context of the conversation. Irony and sarcasm are deeply rooted in cultural and linguistic nuances, making them difficult for AI to detect, especially when devoid of vocal cues or facial expressions. The challenge is compounded in written text, where tone and non-verbal cues are absent.
For AI to effectively detect irony and sarcasm, it needs to be trained on a wide range of examples that illustrate how these language forms are used in different contexts. This training requires not only linguistic data but also an understanding of the social and cultural contexts in which these forms of speech occur. However, even with extensive training, AI systems may struggle to accurately identify irony and sarcasm, leading to misunderstandings or incorrect interpretations of the intended meaning.
#7 Multi-language and Translation Challenges
Translation and multi-language processing are areas where AI has made significant strides but still faces challenges. Issues arise with languages that have fewer resources available or are structurally very different from the languages the AI has been trained on.
Translation and multi-language processing represent a formidable frontier for AI, where significant strides have been made, but substantial challenges remain. The difficulty lies in the inherent complexity of languages, each with its unique grammar, syntax, idioms, and cultural nuances. AI systems must be able to not only translate words and phrases accurately but also convey the intended meaning, tone, and cultural context. This task is particularly challenging when dealing with languages that are structurally different or have limited available resources for training AI.
Moreover, the nuances of translation go beyond mere word-for-word substitution. Effective translation requires an understanding of the cultural and contextual implications of language, which AI systems often struggle to grasp. This leads to translations that might be grammatically correct but lack the subtlety or cultural appropriateness of human translations. Additionally, languages evolve over time, and keeping AI systems updated with these changes, especially in less commonly spoken languages, is a continuous and resource-intensive exercise.
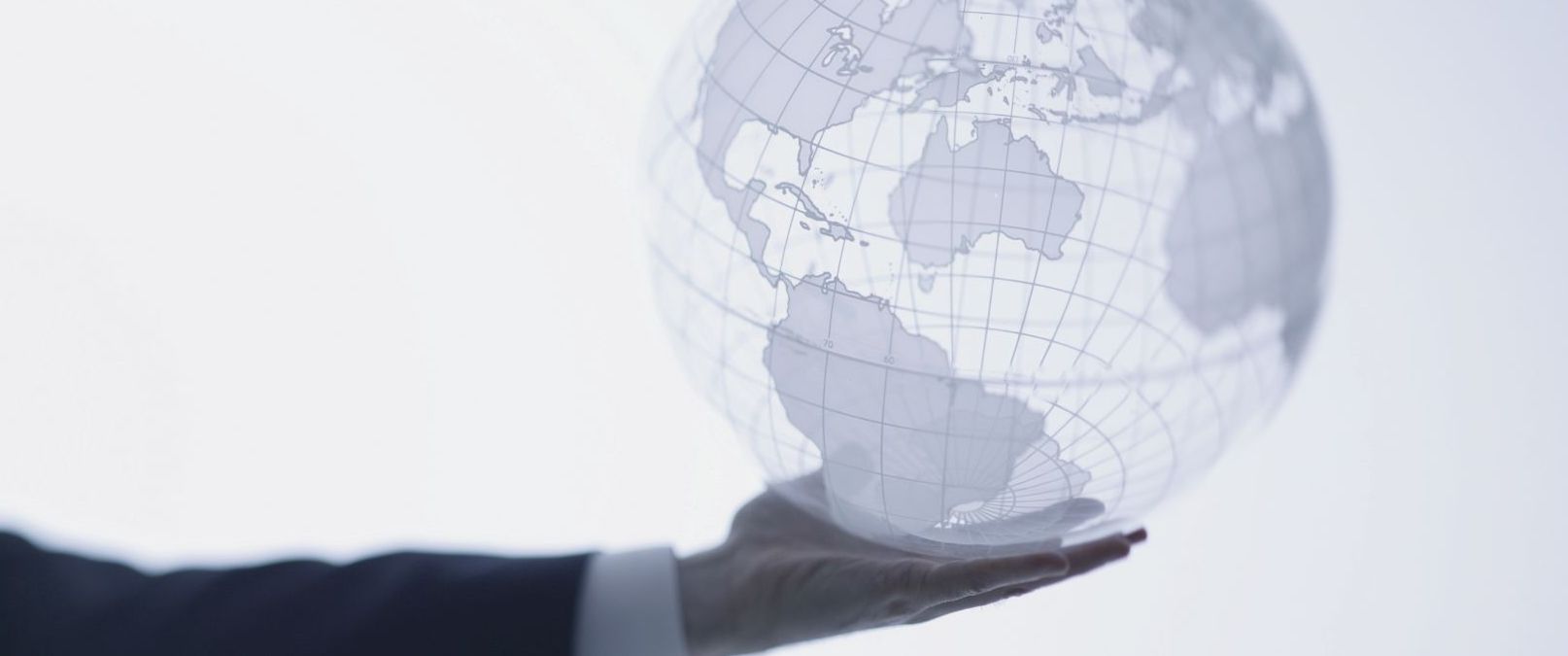
#8 Data Quality and Collection
The quality of data used in training AI systems profoundly affects their performance. High-quality, diverse datasets are necessary for AI to understand and process language effectively. Poor data quality can lead to biases, inaccuracies, and inefficiencies in AI language processing.
The adage “garbage in, garbage out” is particularly apt when it comes to AI language processing. The quality and diversity of the data used in training AI systems are paramount to their effectiveness. High-quality data allows AI to develop a more nuanced understanding of language, leading to more accurate and efficient processing. Conversely, poor-quality data can result in biases, inaccuracies, and inefficiencies. This issue is not just about the quantity of data but also its representativeness and relevance.
Collecting high-quality, diverse datasets is a challenging task, especially considering the vastness and variability of human language. It requires not only a significant amount of data but also data that is representative of different dialects, sociolects, and registers. Additionally, the data must be free from biases, which can be a challenging task given the inherent biases present in human language. Ensuring the quality and diversity of language data is crucial for developing AI systems that are accurate, fair, and effective.
#9 Ethical Considerations in AI Language Processing
Ethical considerations in AI language processing include concerns about privacy, bias, and misuse of technology. Ensuring ethical data collection and use is crucial in maintaining trust and integrity in AI applications.
Ethical considerations in AI language processing encompass a range of issues, including privacy, bias, and the potential for misuse. As AI systems become more integrated into our lives, the ethical implications of their use in language processing become increasingly important. One of the key concerns is privacy, especially when AI systems are used in applications that involve personal or sensitive information. Ensuring that these systems are secure and that the data is used ethically is crucial in maintaining public trust.
Bias in AI language processing is another significant concern. AI systems can perpetuate and even amplify existing biases if they are trained on biased data. This can lead to unfair or discriminatory outcomes, particularly in applications such as hiring, law enforcement, or credit scoring. Ensuring that AI systems are trained on unbiased, representative data and are regularly audited for biases is essential in developing fair and ethical AI systems.
#10 Continual Learning and Adaptability
AI systems must continually learn and adapt to keep up with the evolving nature of language. This requires ongoing data collection, analysis, and system updates to maintain relevance and accuracy.
The ability of AI systems to engage in continual learning and adaptability is paramount, especially in the realm of language processing and understanding. As languages are dynamic, constantly evolving entities influenced by cultural shifts, technological advancements, and global interactions, AI systems need to be designed with the capability to learn continuously and adapt accordingly. This necessitates a multifaceted approach involving ongoing data collection, rigorous analysis, and regular system updates.
The first step in this process is the collection of diverse and comprehensive datasets. These datasets should not only encompass a wide range of languages and dialects but also reflect the nuances of contemporary language use, including slang, new terminologies, and evolving grammatical structures. This collection process must be proactive and inclusive, ensuring that emerging trends and linguistic variations are captured. Moreover, it’s crucial to include data from various sources like social media, literature, scientific publications, and spoken language samples to ensure a holistic understanding of language use in different contexts.
Following data collection, the next critical phase is the analysis and integration of this data into the AI system. This involves advanced machine learning algorithms that can discern patterns, understand contextual nuances, and learn from new examples. The analysis must be thorough and iterative, constantly refining the AI’s understanding and capabilities. This is where the true challenge lies – in developing algorithms that can not only process vast amounts of data but also discern the subtle and complex rules that govern language.
The final step in this continual learning process is the regular updating of the AI system. This involves not just feeding new data into the system, but also re-evaluating and refining the underlying algorithms. As language trends come and go, the system must be agile enough to prioritise current relevancy while retaining historical understanding. This requires a delicate balance – ensuring the AI system is not only up-to-date but also comprehensive and not skewed by transient language fads.
However, this process is not without its challenges. One of the primary concerns is ensuring the ethical collection and use of data. As language is inherently tied to culture and identity, it’s crucial that data collection processes are respectful and do not encroach on privacy or cultural sensitivities. Furthermore, there is a risk of bias in the data, which could lead to skewed AI interpretations and responses. Mitigating these risks requires careful oversight and a commitment to ethical AI practices.
Key Tips For Quality AI Language Processing
- Ensure data quality and diversity for effective AI language processing.
- Regularly update AI systems to adapt to language evolution.
- Consider cultural and regional differences in language datasets.
- Focus on continual learning and adaptability of AI systems.
Way With Words excels in providing highly customised and appropriate data collections for speech and other use cases, crucial for the development of AI in language and speech technologies.
The AI Language Processing Debate and Useful Resources
In conclusion, while AI has revolutionised language processing, its limitations must be acknowledged and addressed. The challenges of understanding context, nuances, idioms, and emotional cues, among others, highlight the need for continuous improvement and ethical considerations in AI development.
Key advice for professionals in this field is to prioritise the quality and diversity of data, understand the cultural and regional aspects of language, and commit to the continual learning and adaptability of AI systems. By addressing these challenges, AI can move closer to a more nuanced and accurate understanding of human language.
Speech Dataset Collection: “Way With Words creates speech datasets including transcripts for machine learning purposes. Our service is used for technologies looking to create or improve existing automatic speech recognition models (ASR) using natural language processing (NLP) for select languages and various domains.”
Machine Transcription Polishing: “Way With Words polish machine transcripts for clients across a number of different technologies. Our machine transcription polishing (MTP) service is used for a variety of AI and machine learning purposes. User applications include machine learning models that use speech-to-text for artificial intelligence research, FinTech/InsurTech, SaaS/Cloud Services, Call Centre Software and Voice Analytic services for the customer journey.”
Cracks in the Facade: Flaws of Large Language Models: Data Science Dogo sets out the challenges of Large Language Models: LLMs are AI giants reshaping human-computer interactions, displaying linguistic marvels. However, beneath their prowess, lie complex challenges, limitations, and ethical concerns.