How AI Systems Navigate Dialects and Language Variations
How Do AI Systems Deal with the Maze of Regional Variations in Language?
In an increasingly interconnected world, the role of Artificial Intelligence (AI) in bridging language barriers is becoming indispensable. The challenge, however, is not just in translating languages but also in navigating the complex maze of dialects and regional variations. As we rely more on AI for languages, questions arise about its ability to understand and respond to these variations.
What are the benefits and risks involved in using AI for this purpose? How can we ensure the data collected is of the highest quality, and the technology is advanced enough to meet its intended capabilities? This article aims to explore these questions, providing valuable insights for translators, interpreters, data scientists, technology entrepreneurs, software developers, educationalists, and industries leveraging AI to enhance communication.
Key Aspects to Consider for Quality Data
Accuracy of AI Dialect Identification
AI dialects must accurately identify and differentiate between dialects for effective translation. This involves training AI models with extensive, dialect-specific datasets.
The accuracy of dialect identification in AI hinges on the technology’s ability to discern and accurately translate nuances specific to each dialect. This process is far from simple, as it involves more than just literal translation; it requires an understanding of the subtleties that define a dialect. To achieve this, AI models must be trained on extensive, dialect-specific datasets.
These datasets should include not only spoken dialects but also their written forms if available, capturing the full spectrum of dialectal variations. This training allows the AI to recognise and adapt to the unique phonetic, syntactic, and lexical features of each dialect. Furthermore, it’s important for AI to distinguish between closely related dialects, which often have subtle but significant differences.
The challenge extends beyond mere recognition; it’s about understanding the context in which these dialects are used. For instance, the same word or phrase might have different meanings or connotations in different dialects. AI must be sophisticated enough to discern these differences to provide accurate and contextually appropriate translations.
This requires not just technological expertise but also input from native speakers and linguists who can provide insights into the intricacies of their native dialects. Such comprehensive training enables AI to navigate the complex terrain of dialects, ensuring translations are not only accurate but also culturally and contextually relevant.
Cultural Nuances and Context
Language variation and AI must work hand-in-hand to understand cultural nuances and context, crucial for accurate translation and interpretation.
Understanding cultural nuances and context is essential for any AI dealing with language variation. AI must be equipped to handle the subtleties of language that are often deeply rooted in culture. This means going beyond the words themselves to grasp the cultural context in which they are used. Cultural references, humour, idioms, and even historical context play a significant role in how language is understood and interpreted. For instance, a phrase that is considered polite in one culture might be offensive in another. AI systems must be sensitive to these differences to avoid misinterpretation and ensure effective communication.
To achieve this, AI systems should be trained on a wide range of cultural contexts, incorporating examples from literature, media, and real-life conversations. This training helps AI understand not just the language but the cultural setting in which it is used. Additionally, input from cultural experts can provide AI with a deeper understanding of the nuances and subtleties of different cultures. Such comprehensive training ensures that AI can accurately interpret and translate language, taking into account the cultural context and ensuring that the essence of the message is not lost in translation.
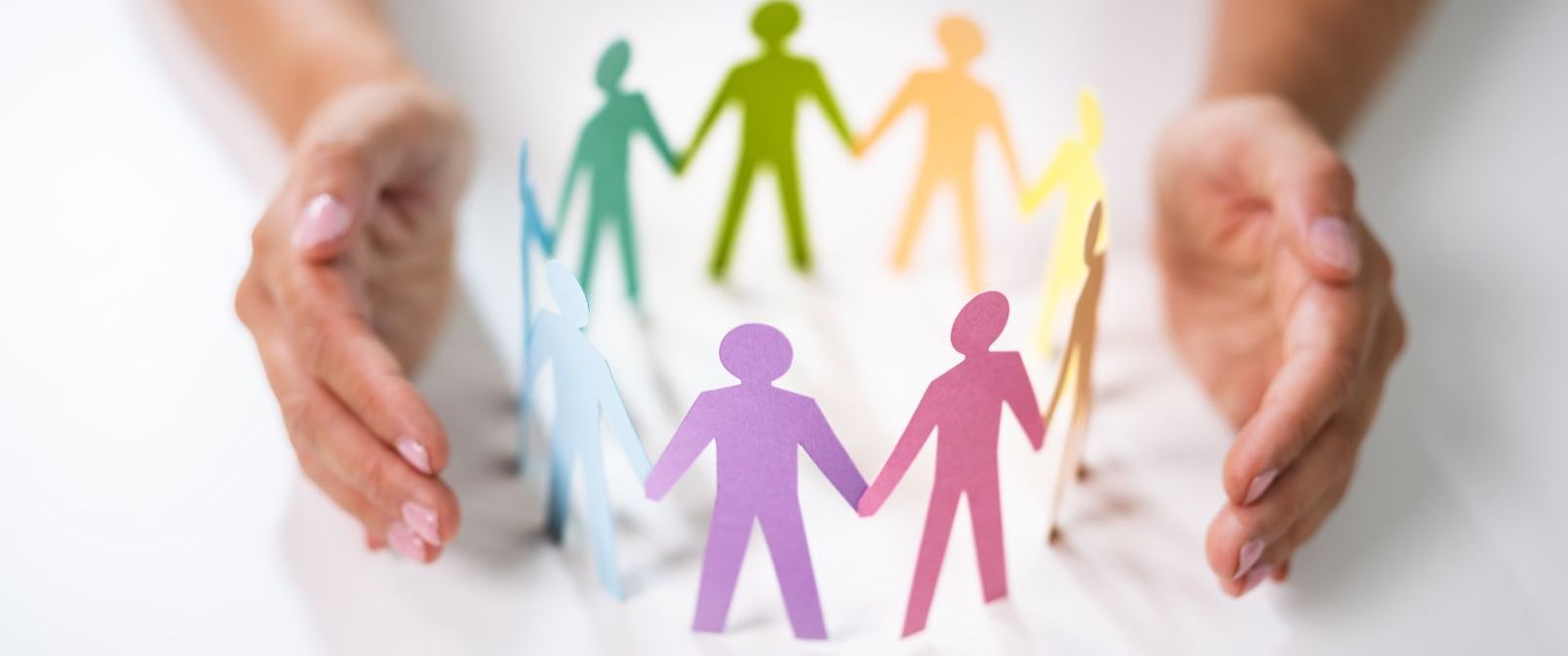
Data Diversity
Ensuring a diverse range of speech and text data from various dialects improves the AI’s ability to understand and translate different dialects accurately.
Data diversity is a cornerstone in the development of AI systems capable of understanding and translating various dialects. A diverse dataset includes a wide range of speech and text data from different regions, age groups, and social backgrounds. This diversity enables AI to recognise and process the myriad ways in which a language can be spoken or written.
For example, the way language is used by younger generations might differ significantly from its use by older generations. Similarly, urban dialects may differ from their rural counterparts. By exposing AI to this wide array of data, it becomes more adept at understanding and accurately translating different dialects.
However, collecting diverse data is not without its challenges. It requires extensive resources and effort to gather and annotate data from various dialects, especially those that are less commonly spoken or underrepresented. This is where partnerships with local communities, linguistic experts, and organisations can be invaluable. Collaborations can facilitate the collection of authentic and diverse data, ensuring that AI systems have a comprehensive understanding of different dialects. Moreover, a diverse dataset not only improves the AI’s performance but also ensures inclusivity, making AI tools more accessible and useful to a broader range of users.
Continuous Learning and Updating
AI systems must continuously learn and update their knowledge base to adapt to evolving language and dialects.
The landscape of language is constantly evolving, making continuous learning and updating a critical component of AI systems in language translation. AI must be designed to adapt to new linguistic trends, slang, and changes in dialects. This ongoing learning process can be achieved through machine learning algorithms that continuously analyse new data and refine the AI’s understanding of language. For instance, the introduction of new slang or colloquialisms can significantly change the meaning or usage of words. AI systems must be updated regularly to keep pace with these changes, ensuring their translations remain accurate and relevant.
Furthermore, continuous learning also involves correcting errors and improving performance over time. User feedback plays a crucial role in this process. By analysing feedback, AI systems can identify areas of improvement, whether it’s in grammar, syntax, or context interpretation. This feedback loop, combined with regular updates to the AI’s linguistic database, ensures that the AI remains up-to-date and effective in handling the dynamic nature of human languages and dialects.
User Feedback Integration
Incorporating user feedback helps in refining AI capabilities in dialect recognition and translation.
Incorporating user feedback is essential in refining AI’s capabilities in dialect recognition and translation. User feedback provides real-world insights into how effectively the AI is performing and highlights areas that need improvement. For instance, users can provide feedback on mistranslations, unclear interpretations, or cultural inaccuracies. This feedback is invaluable as it comes directly from the end-users who interact with the AI in practical scenarios. By analysing this feedback, developers can make targeted improvements to the AI, enhancing its accuracy and reliability.
Moreover, user feedback can also guide the development of new features and functionalities in AI systems. For example, users may identify the need for the AI to recognise regional idioms or specific cultural references. Integrating such features can significantly improve the user experience and the utility of the AI. Additionally, engaging with users creates a sense of community and collaboration, where users feel their input is valued and contributes to the development of better AI technology.
Regional Specificities
AI should be aware of regional specificities like idioms, slangs, and expressions peculiar to a dialect.
AI’s awareness of regional specificities such as idioms, slangs, and expressions peculiar to a dialect is crucial for accurate translation and interpretation. These specificities often carry meanings and connotations that are deeply ingrained in the local culture and may not have direct equivalents in other languages or dialects. For AI to effectively interpret and translate these expressions, it must have a deep understanding of the regional context in which they are used. This involves not only linguistic knowledge but also cultural awareness.
Training AI systems to recognise and understand regional specificities requires extensive data from those regions, including colloquial speech and local literature. Collaborations with regional language experts and native speakers can provide valuable insights into the nuances of local dialects.
Additionally, incorporating region-specific examples and scenarios in the AI training process can help the system learn the appropriate usage and interpretation of local expressions. This region-specific approach ensures that AI translations are not only linguistically accurate but also culturally relevant and appropriate.
Technology Accessibility
AI tools should be accessible to people from various regions, encouraging the collection of diverse data.
Ensuring the accessibility of AI tools to people from various regions is fundamental to promoting language diversity and inclusivity. Accessible AI technology allows for the collection of diverse data, which in turn enhances the AI’s ability to understand and translate different dialects. To achieve this, AI tools should be designed with user-friendliness in mind, accommodating users with varying levels of technical expertise. This includes intuitive interfaces, multilingual support, and easy-to-follow instructions.
Moreover, accessibility also involves making AI technology available to people in different geographical regions, including those with limited access to advanced technology. This can be achieved through mobile applications, web-based platforms, and other technologies that are widely accessible. By extending the reach of AI tools, a broader range of dialects and languages can be included in the AI training process, enhancing the system’s overall performance and utility.
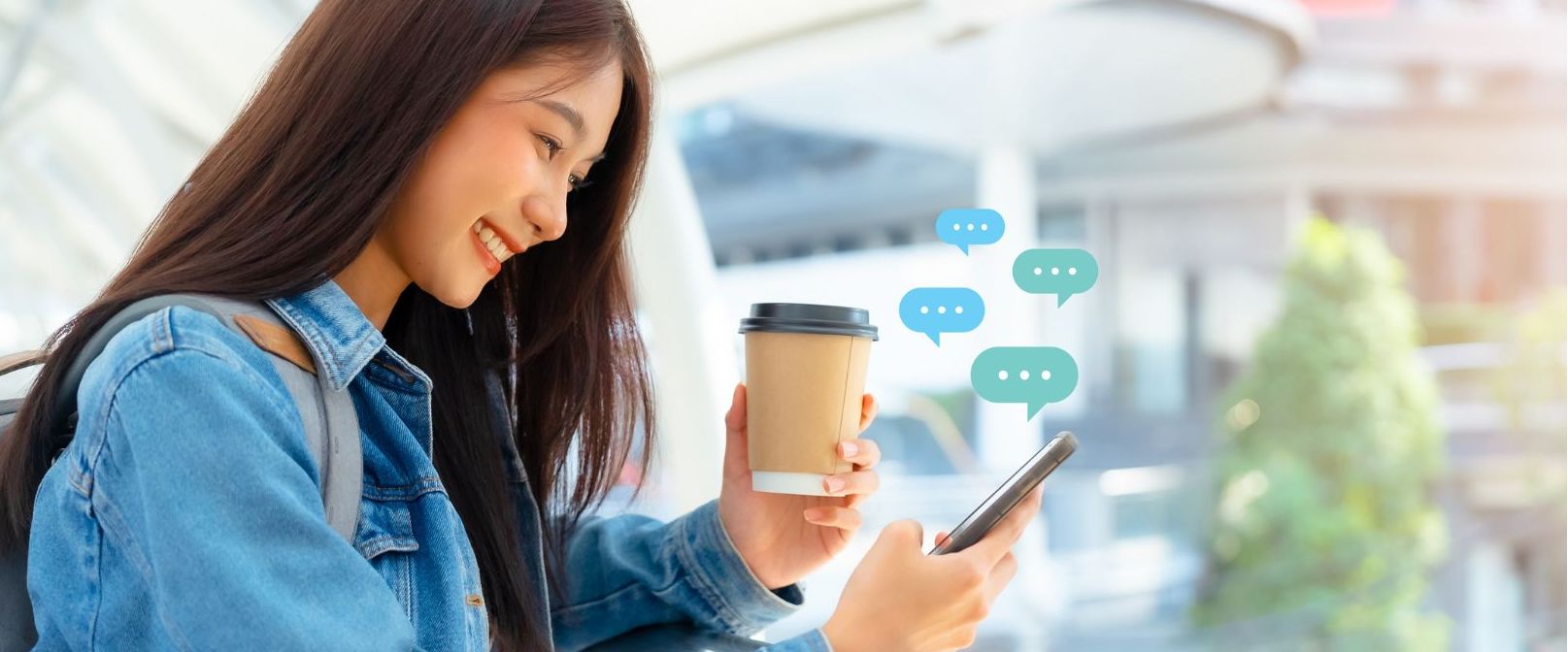
Ethical Considerations
Ethical use of data, especially in dialect-rich, sensitive cultural contexts, is crucial.
Ethical considerations are paramount, especially in the context of dialect-rich, sensitive cultural environments. The use of AI in language translation and interpretation must be guided by principles of respect, inclusivity, and cultural sensitivity. This involves ensuring that the data used for training AI is collected and used in an ethical manner, with proper consent and consideration for privacy. It’s also important to avoid biases in AI algorithms that could lead to the underrepresentation or misrepresentation of certain dialects or cultural groups.
Additionally, ethical AI should aim to preserve and respect the linguistic diversity of different cultures. This means recognising the value of all dialects, including those that are less commonly spoken or at risk of being overshadowed by dominant languages. Ethical AI development should contribute to the preservation of linguistic heritage, ensuring that all dialects are represented and valued in the digital landscape.
Collaboration with Language Experts
Collaborating with linguists and dialect experts can significantly enhance AI’s dialect understanding.
Collaborating with linguists and dialect experts significantly enhances AI’s understanding of different dialects. Language experts bring a depth of knowledge and insight that is essential for developing accurate and nuanced AI translation systems. They can provide guidance on linguistic subtleties, cultural contexts, and regional specificities that are difficult for AI to learn solely from data. For instance, linguists can help identify idiomatic expressions, regional slangs, and cultural references that are unique to a specific dialect.
These collaborations can take various forms, including consultancy, joint research projects, or participation in the data annotation process. Language experts can help ensure that the data used for training AI is accurate, relevant, and culturally sensitive. They can also assist in evaluating the AI’s performance, providing feedback and recommendations for improvement. By combining the technological expertise of AI developers with the linguistic expertise of language professionals, the development of AI in language translation and interpretation can achieve greater accuracy and cultural sensitivity.
Handling Ambiguity and Variability
AI must be adept at handling the ambiguity and variability inherent in human languages and dialects.
The challenge of handling ambiguity and variability in human languages and dialects is a pivotal aspect of AI’s linguistic capabilities. Human language is inherently complex and nuanced, often laden with contextual meanings that can vary significantly across different regions and cultures.
For AI to be effective in this domain, it must be equipped not only with advanced algorithms but also with a deep understanding of linguistic subtleties. This necessitates a multifaceted approach where AI systems are not just programmed for literal translations but are also attuned to the contextual, idiomatic, and cultural nuances that language encompasses.
To navigate this complexity, AI systems need to be built with sophisticated Natural Language Processing (NLP) techniques capable of discerning and interpreting the various shades of meaning that words and phrases may carry in different contexts. For instance, a phrase that is colloquially acceptable in one dialect might have a completely different connotation in another. The AI must recognise these differences and adjust its interpretations accordingly.
Moreover, the inherent variability of language, with its ever-evolving slang, idioms, and expressions, requires AI systems to be dynamic and adaptable. They should continually learn from new data inputs, user interactions, and linguistic research to stay updated with the latest language trends and usage patterns.
Furthermore, the integration of Machine Learning (ML) enables AI to identify patterns in language use, adapt to new linguistic variations, and make informed predictions about meanings in ambiguous contexts. This adaptability is crucial in handling the fluidity of human language.
By analysing vast datasets of spoken and written language from various sources, AI can identify regional dialects, slang, and evolving language trends, thereby refining its ability to handle linguistic ambiguity and variability. This continuous learning process, supported by feedback loops and data updates, ensures that AI systems remain relevant and efficient in deciphering the intricacies of human language.
In conclusion, handling the ambiguity and variability of language is a complex task that requires AI to be highly sophisticated and continually evolving. Through the integration of advanced NLP techniques, continuous learning, and data analysis, AI can effectively navigate the complexities of human language, making it an invaluable tool in bridging communication gaps across different languages and dialects.
Key Tips For Language Variation and AI Systems
- Ensure AI models are trained with extensive, diverse dialect-specific datasets.
- Prioritise understanding cultural nuances and context in translations.
- Continuously update AI knowledge bases to adapt to evolving language and dialects.
- Integrate user feedback to refine AI’s dialect recognition and translation capabilities.
- Collaborate with linguists and dialect experts to enhance AI’s understanding.
Way With Words provides highly customised data collections for speech and other use cases. These collections are pivotal in developing AI technologies where language and speech recognition are key. By focusing on quality and diversity, Way With Words ensures that the AI systems developed are not only technologically advanced but also culturally and linguistically inclusive.
Navigating the complex landscape of dialects and regional language variations is a formidable challenge for AI. However, with the right approach, which includes diverse and quality data collection, continuous learning, and ethical practices, AI can significantly bridge language barriers. The key is in understanding the nuances and context of different dialects, and continuously adapting to the ever-evolving language patterns. As we move forward, collaborations between AI developers and language experts will be crucial in enhancing the capabilities of AI in language translation and interpretation.
Way With Words plays a crucial role in this ecosystem by providing tailored services that feed into the development of sophisticated AI systems. From creating speech datasets to polishing machine transcripts, their services are integral to advancing AI’s understanding of human language in all its complexity and diversity.
AI For Languages Resources
Way With Words – Speech Collection Service: “We create speech datasets including transcripts for machine learning purposes. Our service is used for technologies looking to create or improve existing automatic speech recognition models (ASR) using natural language processing (NLP) for select languages and various domains.”
Way With Words – Machine Transcription Polishing: “We polish machine transcripts for clients across a number of different technologies. Our machine transcription polishing (MTP) service is used for a variety of AI and machine learning purposes. User applications include machine learning models that use speech-to-text for artificial intelligence research, FinTech/InsurTech, SaaS/Cloud Services, Call Centre Software and Voice Analytic services for the customer journey.”
AI and Linguistic Evolution: The Digital Catalyst in Language Dynamics: Language is an ever-evolving and dynamic force. It continuously changes, adapts, and in some cases, fades away entirely. In the past, language changes were often driven by events such as migrations, conquests, and the blending of cultures. Today, the swift advancement of technology in the digital era plays a key role in influencing how languages develop and transform.