Managing Background Noise in Speech Data Collection: Strategies for Clarity
How do I Deal with Background Noise in Speech Data Collection?
Background noise poses a significant challenge in the field of speech data collection, especially when striving to create datasets with high clarity and precision by validating speech data quality. Mitigating noise is crucial to maintain the quality of data used in AI training, transcription, and linguistic research. Successfully addressing these issues enables researchers and engineers to derive accurate insights and develop reliable technologies.
Some common questions that arise in this context include:
- How does background noise impact the quality of speech data?
- What practical techniques are available to reduce noise during data collection?
- Which tools and technologies are most effective for achieving clear speech recordings?
This short guide provides an in-depth exploration of these questions, offering actionable strategies to enhance the clarity of speech data.
10 Key Speech Noise Reduction Topics & Tips
1. Background Noise in Speech Data: Its Impact on Quality
The presence of background noise can severely compromise the integrity of speech recordings. Such distortions lead to difficulties in accurately analysing data, hinder the effectiveness of AI training, and impair user experiences in applications relying on speech recognition. Addressing this issue is critical for ensuring that collected speech data meets the requirements for advanced AI systems and professional applications.
- Data Integrity: Background noise reduces the signal-to-noise ratio (SNR), which is a key determinant of audio clarity. Low SNR values make it challenging to distinguish the speaker’s voice from surrounding sounds, thereby limiting the reliability of data for downstream applications. This can result in inaccuracies in linguistic annotations, poor-quality transcriptions, and errors in phonetic analysis. High SNR recordings, by contrast, yield datasets that are more precise and actionable.
- AI Training Challenges: AI models trained on noisy datasets are prone to generating errors, as they may misinterpret speech patterns or fail to recognise contextually significant cues. These issues become particularly evident in real-world applications, where noisy inputs are common. For instance, voice-activated systems like smart assistants often misfire in noisy environments due to suboptimal training data. Research suggests that increasing the SNR of training datasets can enhance speech recognition accuracy by 20-30%, reducing operational failures in AI systems.
- User Experience: Speech-based applications, such as virtual assistants, automated transcription tools, and voice-controlled devices, heavily depend on the quality of their underlying data. Background noise diminishes user satisfaction by causing miscommunications and erroneous outputs. For example, a virtual assistant might incorrectly interpret a spoken command, leading to user frustration. Ensuring noise-free data during collection mitigates these issues and improves the overall reliability of such technologies.
Furthermore, noise in speech recordings often affects the nuances of linguistic features like tone, pitch, and pauses. These elements are crucial in applications such as sentiment analysis, which requires detailed auditory information to assess emotional content. By minimising background noise, organisations can ensure that such subtleties are preserved, enhancing the depth and scope of their analyses.
The broader implications of background noise are evident in fields such as healthcare, where speech data is used to diagnose cognitive or speech disorders. In these scenarios, clean audio is not just preferable but essential for making accurate assessments. Noise contamination can obscure vital speech characteristics, leading to misdiagnoses or the need for repeat recordings—a time-consuming and costly process. As such, prioritising high-quality, noise-free data is an investment that directly impacts both efficiency and outcomes in critical applications.
2. Noise Reduction Techniques for Clear Speech Data Collection
Implementing noise reduction techniques during the data collection process is essential for obtaining clean and reliable speech recordings. By integrating both preventative measures and corrective tools, researchers can tackle noise at multiple stages of the process, improving the overall quality of the data. Preventative strategies focus on optimising the recording environment and equipment setup, ensuring that noise is minimised from the outset.
Corrective tools, on the other hand, refine the recordings post-collection, allowing for the removal of residual noise and other distortions. This dual approach not only enhances clarity but also ensures the integrity of speech data, which is vital for applications ranging from AI training to linguistic research.
- Environmental Control: Selecting locations with minimal background disturbances is key. Acoustic treatment of spaces using materials like foam panels or carpets helps absorb unwanted sounds.
- Directional Microphones: These microphones focus on capturing sound from a specific direction while suppressing ambient noise. They are particularly useful in environments with unavoidable distractions.
- Pop Filters and Windscreens: These inexpensive tools effectively minimise distortions caused by plosive sounds or wind interference, ensuring smoother recordings.
- Software Filters: Noise cancellation software, such as those offering real-time filtering, can be integrated during live recording sessions to improve sound quality instantly.
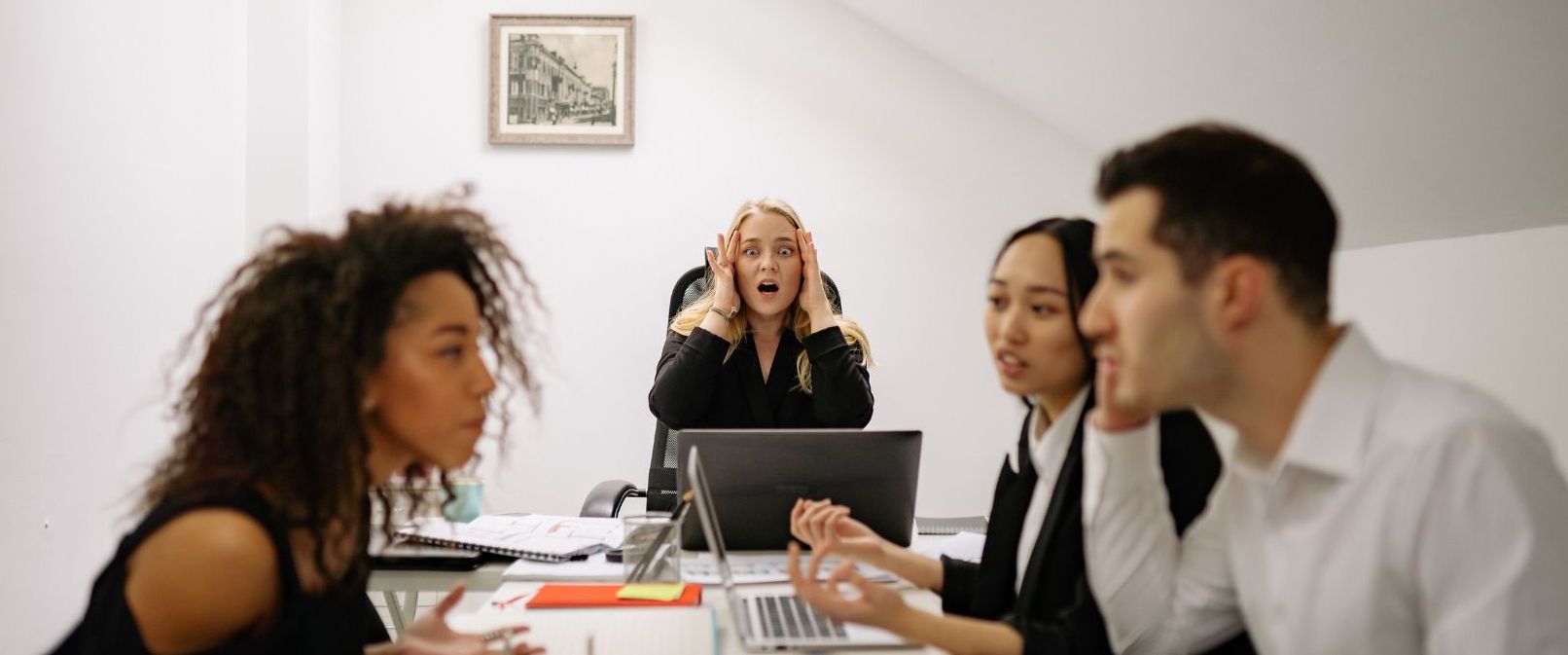
3. Tools and Technologies for Background Noise Reduction
Advanced tools and technologies continue to play a crucial role in mitigating background noise and enhancing the quality of speech data. As the demand for cleaner and more precise recordings grows, the available solutions are becoming more sophisticated and accessible.
- AI-Based Noise Cancellation: Artificial intelligence has transformed noise cancellation, with tools like Krisp and Nvidia RTX Voice leading the way. These solutions analyse audio input in real time, isolating speech from ambient noise using advanced machine learning algorithms. Their user-friendly interfaces make them ideal for professionals in remote work or data collection scenarios, allowing for consistent high-quality audio even in challenging environments.
- Digital Signal Processors (DSPs): DSPs are specialised hardware components designed to filter out specific frequencies associated with background noise. By applying precise algorithms, DSPs enhance the clarity of recordings, making them an essential tool for environments with persistent low-frequency interference like HVAC systems or machinery.
- Acoustic Echo Cancellation (AEC): Echoes can severely distort speech recordings, especially in large or poorly treated spaces. AEC technology addresses this by dynamically identifying and suppressing echo patterns in real time. These systems are invaluable in conference calls, virtual classrooms, and other applications where speech clarity is paramount.
- Microphone Arrays: Advanced microphone array setups utilise multiple microphones placed strategically to capture sound from a target source while suppressing ambient noise. These arrays, often used in conjunction with beamforming algorithms, focus on the speaker’s voice, making them highly effective in noisy environments such as open offices or public spaces.
- Noise Reduction Plugins: Software plugins like iZotope RX and Adobe Audition provide post-processing capabilities that allow users to fine-tune their recordings. These tools employ spectral editing and adaptive filtering to remove hums, static, and other noise artefacts, ensuring pristine audio output.
By integrating these technologies into their workflows, professionals can achieve significant improvements in the quality of their speech data, paving the way for more reliable and actionable outcomes.
4. Case Studies on Successful Noise Management
Real-world examples illustrate the transformative impact of implementing effective noise management strategies across diverse fields. Each case highlights the importance of tailoring approaches to specific use cases to achieve optimal results in speech data quality.
- Healthcare Research: Clinical studies often require precise audio data for patient interviews or therapy sessions. By conducting recordings in soundproof rooms equipped with acoustic panels and advanced microphones, researchers saw a remarkable 40% improvement in transcription accuracy. This enhancement allowed for better diagnostic accuracy and reduced the time spent on manual corrections, streamlining workflows and improving overall efficiency.
- Call Centres: Customer service operations are particularly vulnerable to noise interference, as background chatter and ambient office sounds can distort conversations. Several companies addressed these challenges by integrating AI-driven noise cancellation tools into their communication systems. As a result, they reported a significant reduction in customer complaints and a measurable increase in satisfaction rates. These technologies not only improved the clarity of live conversations but also enhanced the quality of recorded calls used for training and compliance purposes.
- Academic Research: Fieldwork often presents unpredictable challenges, including environmental noise and inconsistent recording conditions. A university linguistics department addressed these issues by deploying portable recording booths and high-quality directional microphones. These solutions ensured the integrity of their data, even in outdoor settings or crowded environments. By reducing noise-related disruptions, researchers were able to focus on analysing speech patterns and linguistic nuances without the need for extensive post-processing.
5. Future Innovations in Noise Reduction Techniques
Future advancements in noise reduction techniques are set to revolutionise how we manage background interference in speech data collection. Emerging technologies promise not only incremental improvements but also transformative changes in the way noise is addressed at every stage of the recording process. From cutting-edge machine learning algorithms capable of real-time noise identification and elimination to adaptive acoustic hardware that learns and evolves with the environment, the possibilities are vast.
Additionally, the integration of wearable noise-cancelling devices and mobile applications tailored for dynamic recording scenarios is expanding the boundaries of what can be achieved. As these innovations continue to develop, professionals in speech data collection can look forward to tools and methodologies that provide unprecedented control over audio quality, paving the way for more reliable and precise data.
- Neural Networks: Deep learning models are increasingly capable of distinguishing speech from background sounds, offering unprecedented levels of accuracy.
- Wearable Devices: The development of wearable noise-cancelling devices allows researchers and professionals to record high-quality audio in dynamic environments.
- Real-Time Adaptive Systems: Algorithms capable of dynamically adjusting to fluctuating acoustic conditions are making it easier to record in challenging settings without compromising quality.
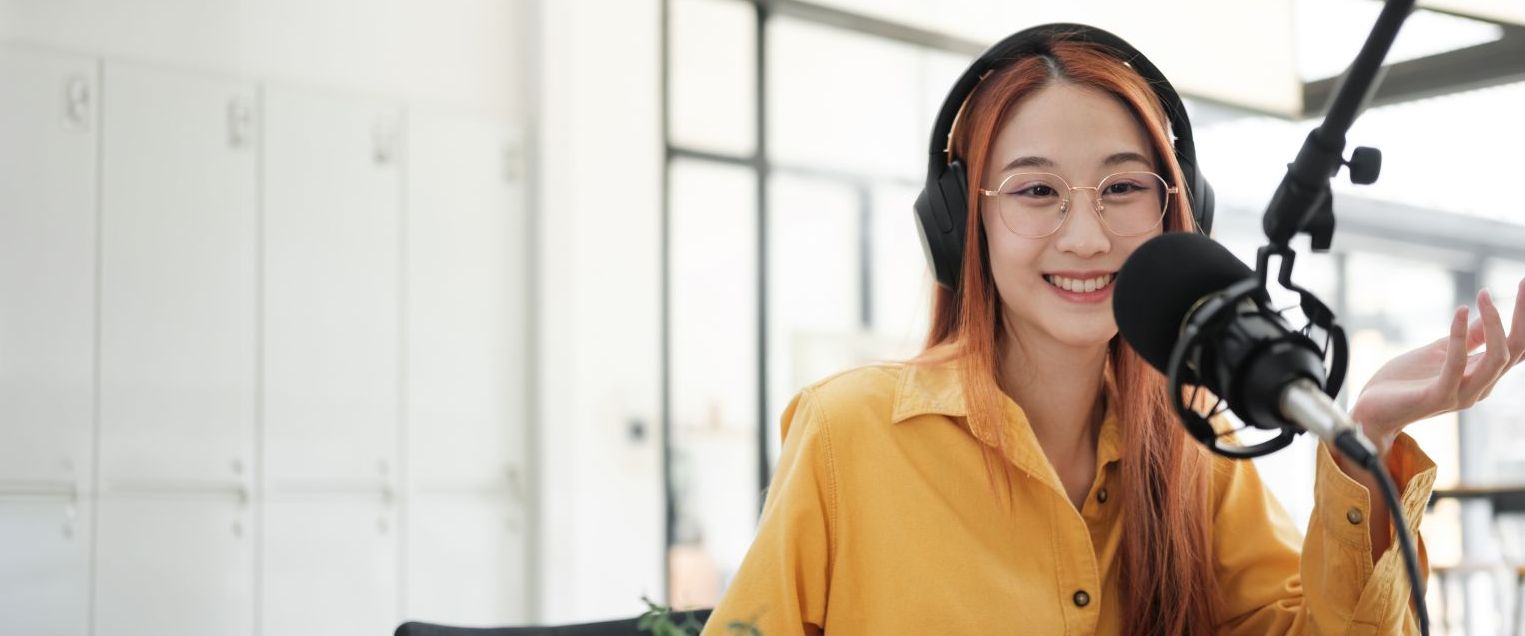
6. Importance of Speaker Positioning
Speaker positioning plays a crucial role in achieving clear and consistent audio quality by optimising the capture of vocal tones while minimising interference from ambient noise. Proper positioning ensures that the microphone accurately captures the intended speech with minimal distortion, contributing to higher signal-to-noise ratios and improved data clarity. Adjustments to microphone height, angle, and proximity to the speaker can significantly enhance the intelligibility and richness of the recorded audio.
- Optimal Distance: Maintaining a fixed distance between the speaker and microphone prevents fluctuations in volume and clarity.
- Angle Management: Aligning microphones to face the speaker directly reduces the capture of extraneous noise.
- Array Configurations: Utilising multiple microphones strategically placed can provide redundancy and enhance the overall quality of recordings.
7. Challenges in Outdoor Recordings
Recording speech outdoors presents distinct challenges due to the unpredictability of environmental factors such as wind, traffic noise, and ambient sounds from nature or human activity. In urban areas, for instance, constant traffic, construction noise, and crowded streets can create significant interference, while rural settings often introduce challenges such as wind gusts, animal sounds, and unpredictable weather.
These varied environmental factors necessitate tailored solutions to suit the specific recording environment. Addressing these challenges requires customised strategies to ensure high-quality audio capture, even in dynamic outdoor settings. Specific technologies, such as directional microphones with windshields and portable soundproofing panels, can mitigate environmental noise effectively. Procedural adjustments, like scouting for quieter locations or timing recordings during less noisy periods, further optimise the recording process. Combining these approaches ensures greater clarity and consistency in outdoor speech data collection.
- Windshields: These attachments effectively reduce wind noise, which is a common issue in open environments.
- Location Scouting: Identifying quiet or secluded areas helps mitigate disturbances caused by traffic or human activity.
- Post-Processing Tools: Software designed for outdoor recordings can isolate and reduce environmental noise after the recording is complete.
8. Collaboration with Participants
Participant cooperation is essential for achieving high-quality recordings, as their actions directly influence the clarity and integrity of the captured audio. Effective collaboration begins with a comprehensive briefing, where participants are educated on how their movements, voice modulation, and ambient behaviours can contribute to or detract from the quality of the recording. Detailed guidelines, such as avoiding sudden movements or minimising clothing rustles, can significantly reduce unintended noise.
Additionally, conducting preliminary test recordings offers an opportunity to identify potential issues and acclimatise participants to the recording environment. These tests allow technicians to fine-tune microphone placement, volume levels, and other variables while providing participants with feedback to optimise their performance.
Finally, creating an open feedback loop encourages participants to voice any concerns or challenges they face during recording sessions. Addressing issues like discomfort from prolonged standing or background distractions ensures a smoother process and results in higher-quality data. This collaborative approach fosters a shared commitment to excellence in the recording process.
- Briefing: Educating participants about minimising movements or background sounds during recordings can reduce inadvertent noise.
- Testing: Conducting preliminary tests ensures that potential noise sources are identified and addressed.
- Feedback Loops: Encouraging participants to voice concerns about recording conditions can help improve the overall process.
9. Post-Processing for Noise Reduction
Post-processing remains a critical step in cleaning up recordings, ensuring that the final audio is of the highest quality possible. One effective technique involves spectral noise reduction, where software like Audacity or iZotope RX identifies and removes specific noise frequencies without altering the core speech frequencies. This is particularly useful for eliminating persistent hums or static sounds.
Another approach is multi-band equalisation, which allows for the adjustment of different frequency ranges to enhance speech clarity while suppressing unwanted noise. For example, raising the mid-range frequencies, where most human speech resides, while lowering the bass and treble regions can make voices more intelligible in recordings with background interference.
AI-driven tools like Adobe Enhance Speech or Auphonic further streamline post-processing. These platforms use machine learning algorithms to detect and isolate speech, reducing the need for manual adjustments. By automating complex tasks such as echo removal or gain normalisation, these tools save time and improve consistency across recordings. With these advanced techniques, even recordings made in suboptimal conditions can achieve near-professional clarity.
- Software Solutions: Tools like Audacity and Adobe Audition offer features specifically designed to remove noise from recorded audio.
- Machine Learning Models: AI-driven tools can analyse and refine audio quality without compromising the integrity of the original speech.
- Frequency Targeting: Focusing on human speech frequencies during processing ensures better results without affecting intelligibility.
10. Balancing Budget and Quality
Balancing financial constraints with the goal of achieving high-quality audio is a common challenge for organisations. Many struggle to allocate sufficient resources to soundproof environments, advanced equipment, or specialised personnel while adhering to tight budgets. However, achieving optimal audio quality doesn’t always require a significant financial investment.
Creative approaches such as utilising readily available materials for soundproofing, like blankets or foam, can offer substantial improvements without straining resources. Additionally, leveraging free or open-source software tools for noise reduction allows smaller teams to maintain professional standards at no extra cost.
For short-term projects, renting high-end recording equipment can be a cost-effective strategy. This allows organisations to access the latest technologies, such as directional microphones or advanced noise-cancellation devices, without committing to the high upfront costs of purchasing. By adopting these strategies, organisations can ensure a balance between cost management and the production of clear, high-quality audio recordings.
- DIY Acoustic Solutions: Affordable materials such as thick curtains or foam tiles can significantly reduce echo and noise.
- Open-Source Software: Free tools provide basic noise reduction capabilities, making them ideal for smaller projects.
- Equipment Rentals: Renting high-end recording equipment can be a cost-effective solution for short-term needs.
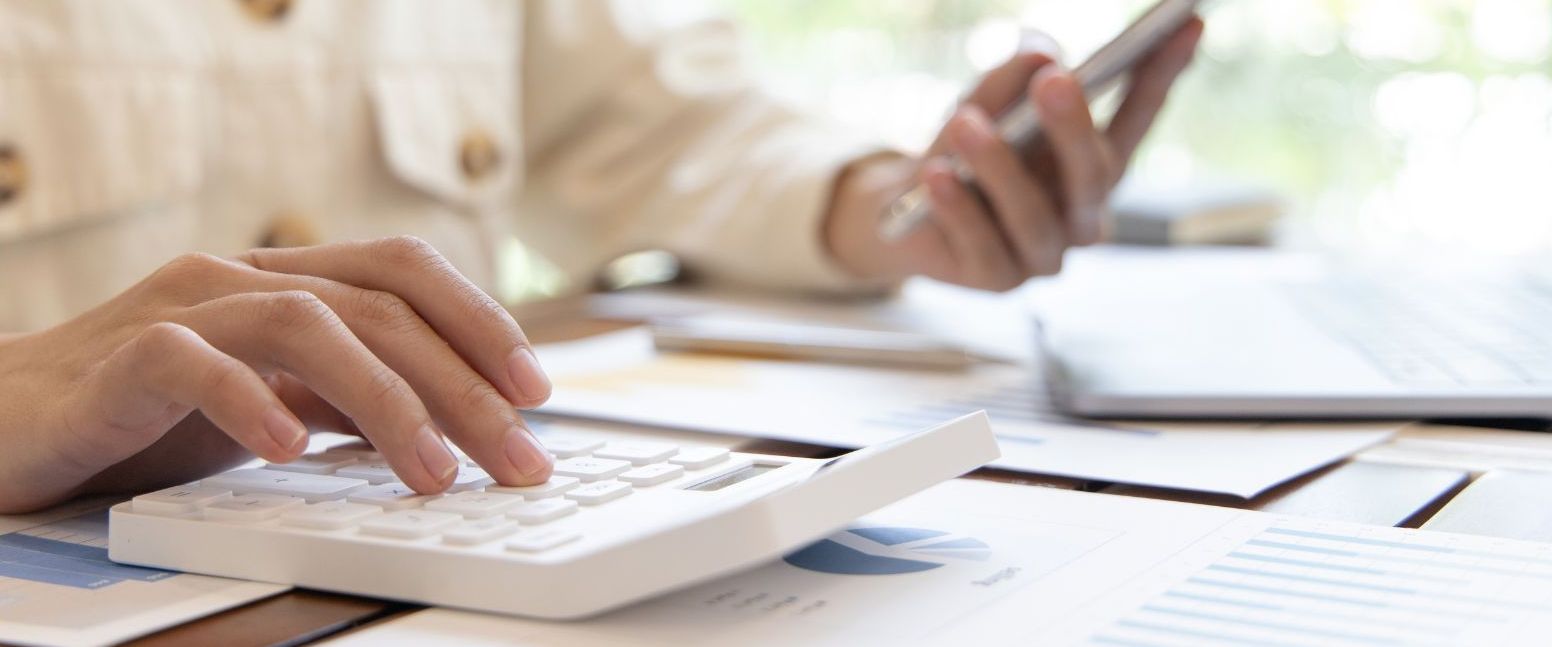
Key Speech Recording Tips
- Scout Locations: Evaluate recording spaces for potential noise sources before starting.
- Invest in Equipment: Quality microphones and headphones are long-term investments that enhance data quality.
- Utilise Filters: Incorporate real-time noise cancellation software during live recordings.
- Train Teams: Ensure participants and staff are knowledgeable about effective noise reduction practices.
- Monitor Quality: Regularly review recordings to maintain consistency and identify areas for improvement.
Effectively managing background noise is a cornerstone of successful speech data collection. By addressing noise issues through a combination of preventive measures, advanced tools, and strategic planning, organisations can achieve datasets that are both accurate and reliable. Whether you are designing AI systems or conducting academic research, prioritising noise reduction ensures that your efforts yield meaningful and impactful results.
A proactive and comprehensive approach—spanning environmental control, technological solutions, and post-processing techniques—is essential for ensuring the clarity and usability of speech data.
Further Speech Collection Resources
Noise Reduction: Explore techniques, methods, and technologies for managing background noise in speech data collection.
Way With Words: Speech Collection: Discover tailored speech collection services designed to ensure high-quality datasets for advanced AI projects.