Choosing Demographics for Speech Data Collection
How Do I Choose the Right Demographics for my Speech Data Collection?
Speech data collection is fundamental to developing accurate, effective, and inclusive technologies like AI-driven speech recognition and language processing tools. In addition to how long it could take to collect, a critical aspect of this process is selecting the right demographics to ensure your data reflects the diversity of your target audience. This short guide addresses the question, “How do I choose the right demographics for my speech data collection?” while offering actionable strategies, insights, and ethical considerations.
Selecting demographics for speech data collection requires thoughtful planning, as the quality of your data directly impacts the outcomes of your research or product development. Misrepresentation or exclusion can lead to biased results, hindering inclusivity and accuracy in AI applications.
Here are some common questions often asked on this topic:
- What factors should I consider when defining my target demographics for speech data collection?
- What tools and techniques can help analyse demographics effectively?
- How do ethical considerations shape demographic selection in speech data collection?
In this short guide, we’ll explore strategies for demographic selection, practical tools for analysis, and the importance of balancing inclusivity with ethical practices.
Key Features & Thoughts On Demographics For Speech Collection
The Importance of Demographics in Speech Data Collection
Demographics form the foundation of effective speech data collection. They determine the representativeness of your dataset, influencing how well your AI system performs across different user groups. Neglecting diversity can result in models that fail to recognise accents, dialects, or linguistic nuances.
Key Points:
- Consider linguistic diversity: Accents, dialects, and regional languages.
- Include underrepresented groups to avoid biases.
- Analyse how demographics align with your project goals (e.g., developing tools for healthcare, education, or entertainment).
Demographics are not just statistical measures; they are the backbone of any speech data collection initiative, dictating the relevance, usability, and fairness of the dataset. Ignoring demographic diversity can render your AI tools ineffective in real-world applications, leading to user dissatisfaction and systemic bias. For example, systems trained predominantly on English speakers from urban areas often fail to recognise regional dialects or rural accents, highlighting the gap in inclusivity.
Accents and linguistic variations add a layer of complexity but also offer an opportunity for innovation. A well-curated dataset accounts for variations in speech due to age, gender, socioeconomic status, and cultural background. By including these factors, developers can create systems that understand users universally, improving accessibility in applications like virtual assistants, healthcare chatbots, and educational tools.
Beyond linguistic accuracy, demographic alignment ensures ethical AI practices. Speech data collection often intersects with cultural norms and sensitivities. Projects focused on healthcare might prioritise specific demographics, such as elderly users or individuals with speech impairments. This level of detail not only refines the dataset but also aligns the collection process with the project’s overarching goals.
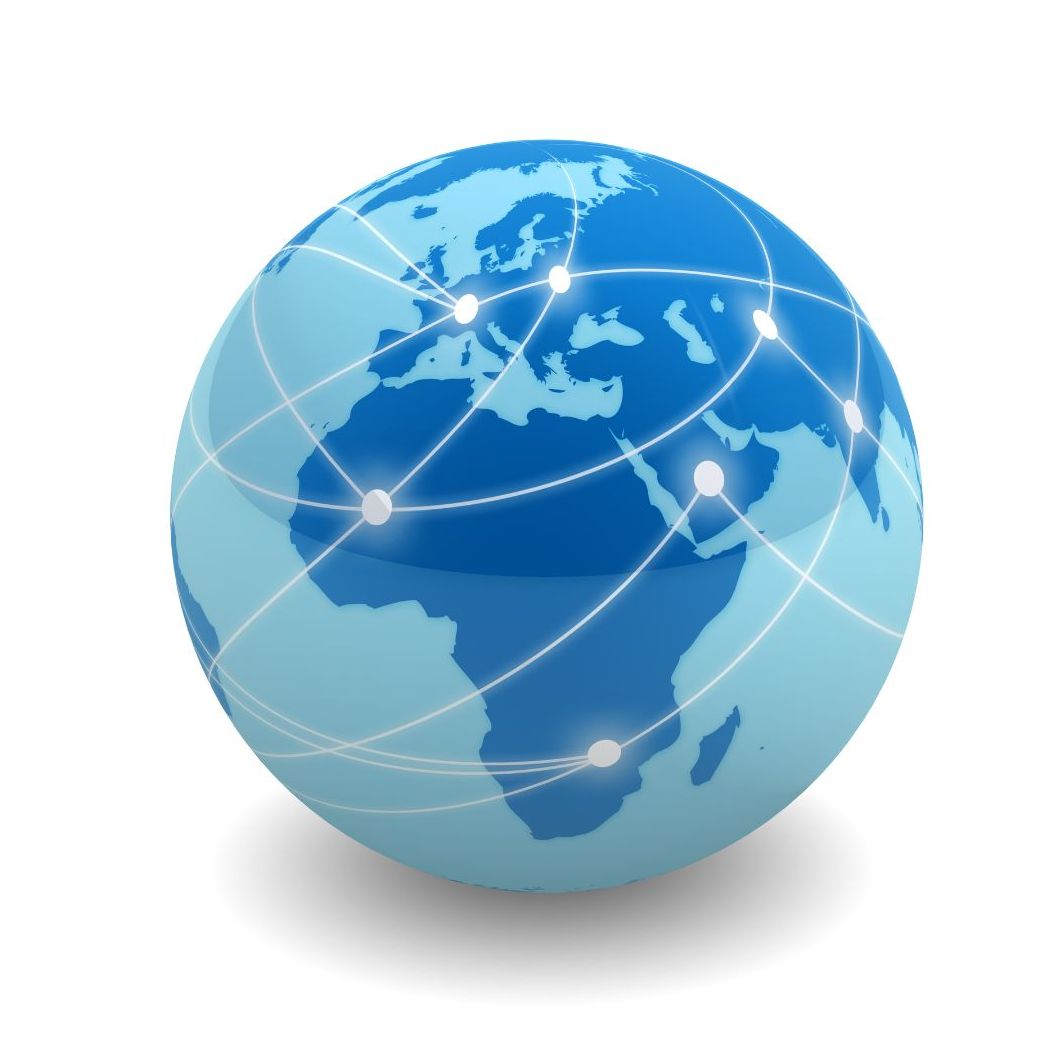
Strategies for Selecting Target Demographics
Develop a clear strategy to define your target demographics. Start by identifying the purpose of your project and the audience it will serve.
Steps to follow:
- Map your target audience’s geographic, cultural, and linguistic diversity.
- Conduct market research to identify primary and secondary demographic segments.
- Use surveys or focus groups to validate assumptions.
Effective demographic selection begins with a granular understanding of your project objectives. Whether you aim to enhance voice recognition software for a global audience or build region-specific AI tools, clarity on the end goal shapes the demographic scope. A one-size-fits-all approach rarely works, as it overlooks nuances like regional language variations or cultural speech patterns.
To begin, segment your target audience based on geography, language, and user context. For example, a voice assistant aimed at Latin American markets might prioritise Spanish speakers while accounting for variations in pronunciation and slang across countries. Conducting detailed surveys or interviews with stakeholders can help fine-tune these criteria.
Validation is equally important. Use pilot programs or focus groups to ensure your assumptions align with real-world needs. Small-scale testing provides insights into gaps or biases in your demographic strategy. Iterative improvements based on these findings help refine the approach, ensuring your dataset mirrors your user base accurately.
Tools and Techniques for Demographic Analysis
Accurate demographic analysis ensures your dataset includes diverse and representative voices. Several tools can streamline this process:
Recommended Tools:
- Statistical software for analysing demographic data (e.g., SPSS, R).
- GIS mapping for geographic representation.
- Voice recognition analytics tools for accent and dialect classification.
Modern tools offer powerful ways to analyse demographics for speech data collection. Statistical software like SPSS and R can process large datasets, revealing trends and gaps in demographic representation. For instance, these tools can highlight underrepresented age groups or linguistic minorities in your initial sampling, prompting corrective measures.
Geographic information systems (GIS) mapping adds another dimension. By visualising participant locations, you can assess geographic diversity at a glance. This is particularly useful for projects requiring regional accents or dialects. Coupled with demographic overlays, GIS tools provide actionable insights into areas requiring greater representation.
Voice recognition analytics tools enhance accuracy by identifying subtle differences in pronunciation and tone. These tools are invaluable for analysing multilingual datasets, ensuring dialectal variations are captured without oversimplification. Combining these technologies streamlines the demographic analysis process, making it easier to create balanced and inclusive datasets.
Case Studies on Effective Demographic Selection
Learn from successful projects that prioritised thoughtful demographic selection. For instance, a case study on a speech recognition app for multilingual users highlights the importance of involving native speakers across different age groups and regions.
Case studies illustrate the impact of thoughtful demographic selection on the success of speech data projects. Consider a speech recognition app designed for multilingual markets in Africa. Developers incorporated voices from over 20 languages and dialects, ensuring the system could process diverse accents accurately. This inclusive approach not only improved functionality but also fostered user trust.
Another example is a healthcare AI project targeting elderly users. Researchers prioritised participants aged 65 and above, focusing on speech patterns affected by aging. The resulting tool excelled in recognising age-specific nuances, outperforming competitors limited to younger demographics.
These case studies underscore the importance of aligning demographic selection with project objectives. They also highlight the value of collaborating with local communities to ensure authenticity and inclusivity in data collection efforts.
Ethical Considerations in Demographic Data Collection
Ethical considerations are paramount when collecting speech data. Adhere to the following principles:
- Obtain informed consent from participants.
- Avoid exploiting vulnerable populations.
- Ensure data privacy and security.
Ethics in demographic data collection revolve around respect, consent, and privacy. Transparency is crucial—participants must understand how their data will be used and the potential implications. Providing clear, accessible consent forms is a fundamental step toward building trust.
Avoiding exploitation is equally critical. Vulnerable populations, such as refugees or economically disadvantaged groups, often provide valuable data but require additional safeguards. Ethical data collection avoids coercion and ensures fair compensation for participants’ time and contributions.
Data privacy and security cannot be overlooked. Employ encryption and secure storage protocols to protect sensitive information. Ethical considerations should extend to the analysis and usage of data, avoiding stereotypes or biases that might perpetuate societal inequities.
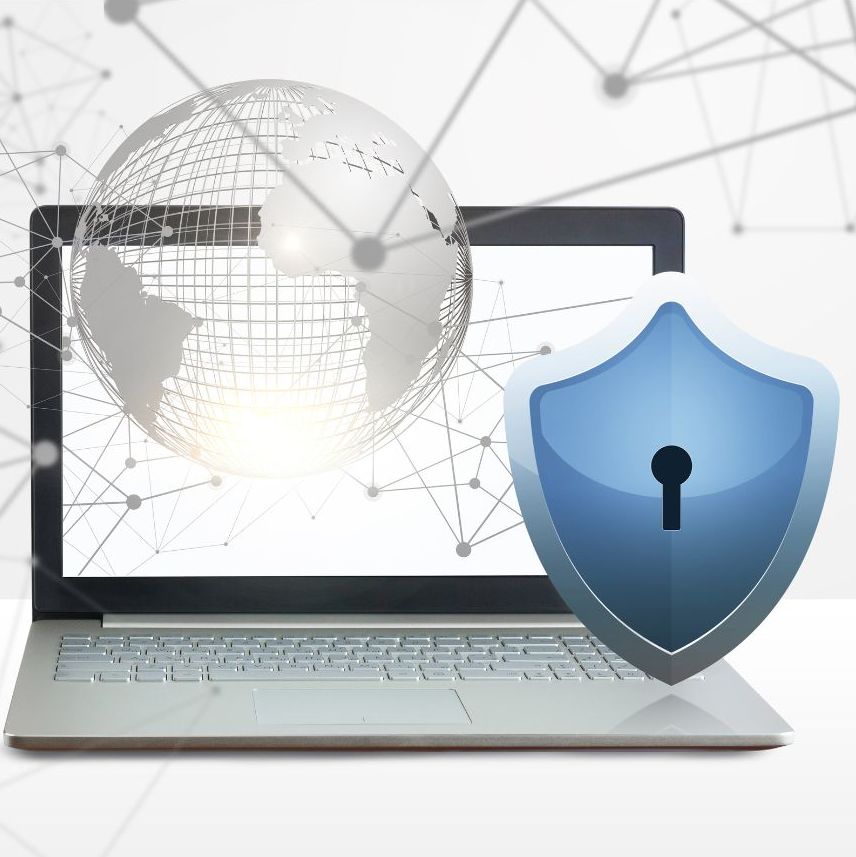
Diversity and Inclusion in Data Collection
Inclusive datasets are key to creating accessible and equitable technologies. For example, a voice assistant that works well in English but struggles with other languages can alienate users in multilingual regions.
Diversity and inclusion are more than ethical imperatives; they are strategic advantages. A speech dataset that reflects real-world linguistic and cultural diversity enables AI tools to perform effectively across a broader range of applications. For instance, including indigenous languages can make AI systems accessible to previously underserved communities.
Practical strategies for fostering inclusion include partnerships with community organisations and local researchers. These collaborations provide access to participants who might otherwise be difficult to reach. Engaging with these groups also builds trust, ensuring the authenticity of your dataset.
Inclusion also involves continuous evaluation. Regular audits of your data collection methods can identify biases or gaps, allowing for corrective actions. This iterative process ensures your dataset remains representative and relevant as user demographics evolve.
Balancing Cost with Representation
While including diverse demographics is essential, it can be resource-intensive. To strike a balance:
- Prioritise key demographics while leaving room for expansion.
- Leverage open-source datasets to supplement gaps.
The financial cost of achieving demographic balance can be significant, but strategic planning helps mitigate these expenses. Begin by prioritising demographics that align most closely with your project goals. For example, a global voice assistant might initially focus on major languages before expanding to niche dialects.
Leveraging open-source datasets is another cost-saving measure. While these resources may not meet all your needs, they provide a solid foundation for augmenting your data. Combine this with targeted recruitment in underrepresented groups to fill gaps without overspending.
Collaborative funding models also reduce costs. Partnering with academic institutions or technology firms allows you to pool resources for larger, more representative datasets. Sharing the financial burden ensures demographic inclusivity without sacrificing quality.
Challenges in Demographic Selection
Recognise common challenges:
- Limited access to underrepresented groups.
- Cultural nuances that impact data quality.
- High costs of large-scale demographic sampling.
Selecting the right demographics is fraught with challenges. Limited access to certain groups, such as rural or low-income populations, can skew your dataset. Overcoming this requires creative solutions, like offering mobile data collection options or partnering with local organisations.
Cultural nuances further complicate the process. For instance, some communities may be hesitant to participate due to mistrust of technology or concerns about data misuse. Building relationships and providing transparency can help address these barriers.
Cost is another hurdle. Comprehensive demographic sampling requires significant investment, particularly for large-scale projects. Allocating resources strategically and seeking external funding can alleviate financial pressures while maintaining data quality.
Role of Technology Firms in Enhancing Demographic Representation
Technology firms play a critical role in pushing the boundaries of inclusive AI:
- Establish partnerships with local communities for authentic data.
- Fund initiatives to address gaps in data representation.
Technology firms hold significant influence over demographic representation in AI. By investing in diverse datasets, these companies ensure their products cater to global audiences. Initiatives like Google’s AI for Social Good demonstrate how corporate resources can address gaps in demographic data.
Partnerships with academic institutions and non-profits also advance inclusivity. These collaborations provide access to underrepresented groups while fostering innovation in data collection methods. Firms that prioritise demographic representation set industry standards, encouraging competitors to follow suit.
Education and advocacy are equally important. By raising awareness of the importance of diversity in AI, technology firms can inspire broader participation in speech data projects, benefiting the entire industry.
Future Trends in Speech Data Collection
As technology evolves, demographic selection will need to adapt. Anticipate trends such as:
- Greater demand for datasets from multilingual and underserved populations.
- Advanced tools for demographic simulation and modelling.
As AI technologies grow more sophisticated, speech data collection will evolve to meet emerging demands. Multilingual datasets will become increasingly vital, reflecting the global nature of AI applications. Expect greater emphasis on minority languages and dialects as companies seek to expand their reach.
Advanced simulation tools will revolutionise demographic selection. These technologies allow researchers to model demographic diversity without exhaustive fieldwork, reducing costs and accelerating data collection. Virtual environments may even enable synthetic data generation, complementing real-world datasets.
Ethics will remain a central concern. As demographic data becomes more granular, safeguarding privacy and preventing misuse will be critical. Anticipating these trends ensures your speech data collection efforts remain relevant and responsible.
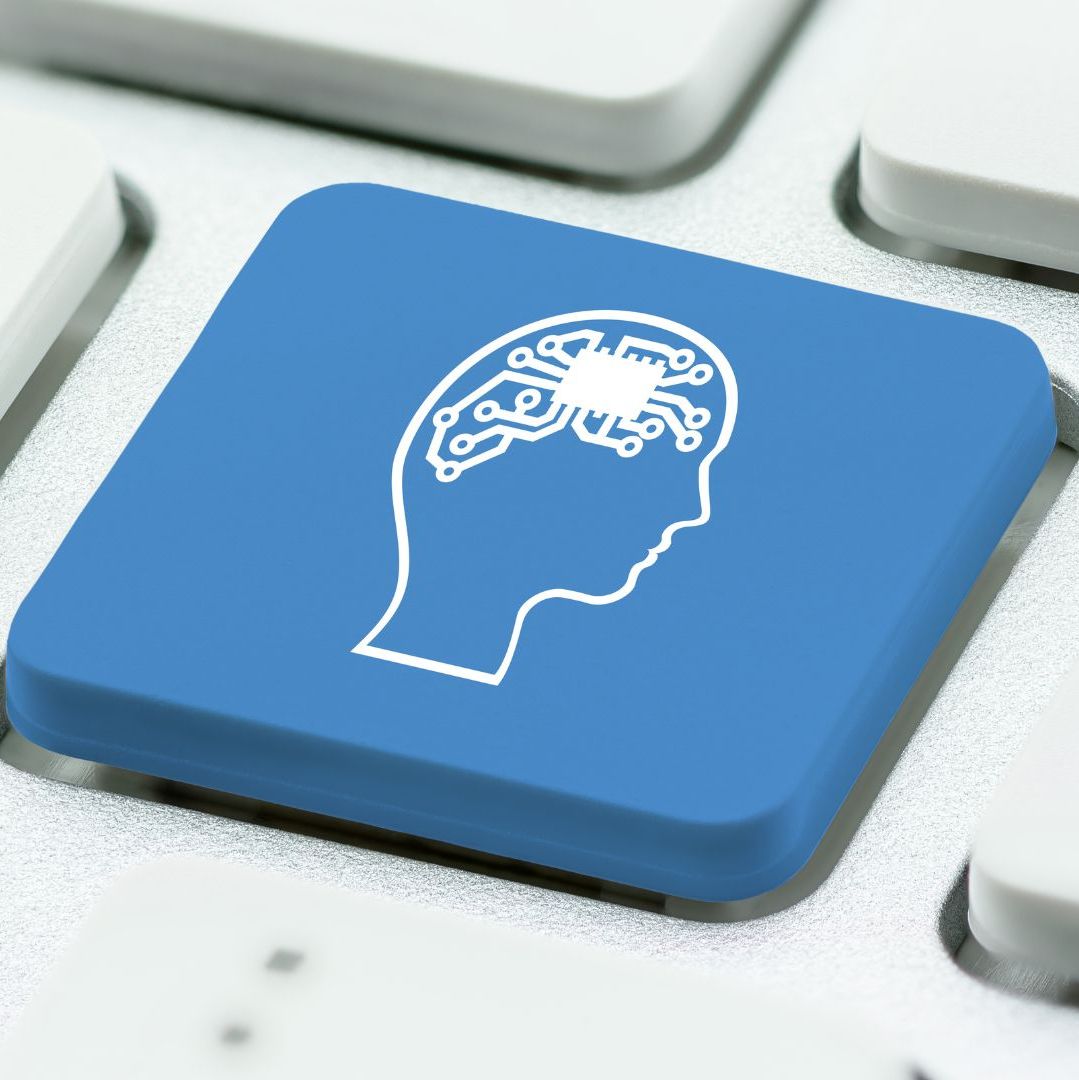
Key Tips For Best Demographic Targeting
- Define Your Goals: Start with a clear understanding of your project’s purpose and its target users.
- Use Advanced Tools: Leverage demographic analysis software to streamline data collection.
- Ensure Ethical Compliance: Always prioritise participant consent and data privacy.
- Invest in Diversity: Include voices from underrepresented demographics to reduce bias.
- Validate Your Approach: Regularly assess the representativeness of your dataset and adjust as needed.
Choosing the right demographics for speech data collection is a nuanced process that combines strategic planning, ethical responsibility, and technological tools. By prioritising diversity and inclusivity, you can create robust datasets that drive innovation and inclusiveness in AI technologies. A critical takeaway is to focus on clear project goals, use advanced tools, and ensure ethical practices to achieve a well-rounded dataset.
Further Resources:
Wikipedia: Demographics: This article provides an overview of demographics, including methods for demographic analysis and their applications, essential for understanding how to choose demographics for speech data collection.
Way With Words: Speech Collection: Way With Words offers bespoke speech collection projects tailored to specific needs, ensuring high-quality datasets that complement freely available resources. Their services fill gaps that free data might not cover, providing a comprehensive solution for advanced AI projects.