Impacts of Dialectal Variation Within African Languages on Speech Recognition
How Does Dialectal Variation Within African Languages Affect Speech Data Collection and Application?
In this time of rapid technological advancement, speech data collection and its application in artificial intelligence (AI) hold a transformative potential for industries worldwide. Yet, for African languages, characterised by rich dialectal diversity, this venture poses unique challenges and opportunities.
This short article delves into the intricacies of collecting and applying speech data within the multifaceted linguistic landscape of Africa. It aims to shed light on key questions, such as how dialectal variation impacts speech recognition technologies, and the strategies necessary to navigate these challenges for linguists, developers, and cultural researchers. The focus is on understanding the implications for machine learning advancements and improving speech recognition solutions that cater to the diverse linguistic reality of the African continent.
Methods and Impact on Speech Recognition in Africa
The Landscape of African Linguistic Diversity
African languages exhibit a broad spectrum of dialectal variation, presenting a significant challenge for speech recognition technologies that often rely on homogeneity in language data.
The linguistic landscape of Africa is unparalleled in its diversity, with over 2,000 languages spoken across the continent. This rich tapestry of languages encompasses a broad spectrum of dialects, each with its unique phonetic, lexical, and syntactic characteristics. The variation is not just inter-language but also intra-language, where dialects of the same language can vary dramatically from one region to another.
This diversity presents a formidable challenge for speech recognition technologies, which typically require large volumes of homogeneous linguistic data to train effectively. Traditional models often falter when confronted with the vast array of pronunciation, vocabulary, and grammar found across African dialects, leading to a gap in speech technology applications that can accurately serve the continent’s linguistic reality.
Moreover, the linguistic diversity of Africa is a testament to its rich cultural heritage but also poses significant challenges for the development and deployment of speech recognition technologies. Most existing technologies are developed with a focus on languages with vast amounts of available data, like English or Mandarin, leaving African languages underrepresented and under-served.
This gap highlights a pressing need for a paradigm shift in speech technology development—one that embraces the complexity of African languages and dialects. Addressing this challenge requires innovative approaches to data collection, algorithm design, and model training that can handle the nuances of dialectal variation, ensuring that speech technologies are inclusive and effective for all Africans.
Challenges in Speech Data Collection
Dialectal variations necessitate a more nuanced approach to data collection, requiring extensive regional representation to ensure the inclusivity of different dialects.
Collecting speech data in the African context is fraught with challenges, primarily due to the sheer diversity of dialects and the geographical and socio-political factors that influence language use. Traditional data collection methods often fall short in capturing the full spectrum of dialectal variation, as they tend to focus on urban centres or regions with better technological infrastructure.
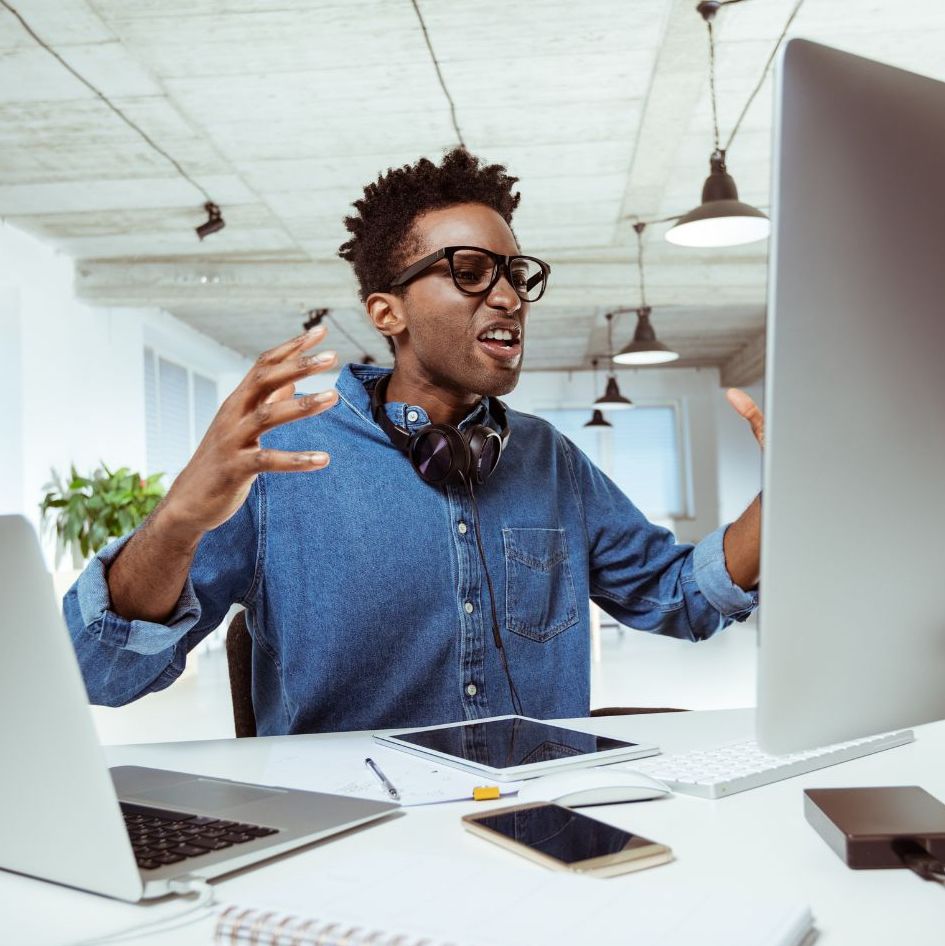
This bias leaves out a significant portion of the population whose dialects may differ substantially from those captured in the datasets. To build truly inclusive speech technologies, data collection efforts must extend into rural and remote areas, where dialectal variations are often most pronounced. This necessitates a more nuanced and localised approach to data collection, involving community engagement and the use of mobile technology to reach a broader swath of the population.
Furthermore, the collection of dialect-specific speech data requires not only geographical diversity but also a deep understanding of the sociolinguistic landscape of the continent. Factors such as age, gender, and social status can influence dialectal variation within communities, adding another layer of complexity to data collection efforts.
Successfully navigating these challenges requires a concerted effort to involve local linguists and cultural experts in the data collection process, ensuring that the speech data reflects the rich linguistic diversity of the continent. By adopting strategies that prioritise inclusivity and cultural sensitivity, speech technologies can begin to bridge the gap between the current state of development and the real-world needs of African language speakers.
Impact on Speech Recognition Accuracy
The accuracy of speech recognition systems can be significantly affected by dialectal diversity, with systems often struggling to recognise speech from dialects not well-represented in training data.
The impact of dialectal diversity on speech recognition accuracy cannot be overstated. Speech recognition systems are typically trained on large datasets that are meant to represent the target language. However, when these datasets lack diversity in dialects, the systems struggle to recognise speech inputs from underrepresented dialects accurately. This discrepancy in accuracy not only affects user experience but can also have broader implications for accessibility and equity. For instance, a speech-to-text service that performs well for urban dialects but poorly for rural ones may inadvertently exclude significant portions of the population from accessing digital services and information.
The challenge of improving speech recognition accuracy in the face of dialectal diversity calls for a multifaceted approach. First, it necessitates the creation of more diverse and representative datasets that include a wide range of dialects. Second, it requires advancements in speech recognition algorithms to better accommodate the variability inherent in natural language. Techniques such as transfer learning, where a model trained on one task is adapted for another, show promise in enhancing the adaptability of speech recognition systems to new dialects.
Additionally, employing dialect identification as a preliminary step in speech recognition can enable systems to switch between specialised models tailored to specific dialects, thus improving overall accuracy. By addressing these challenges, speech recognition technologies can become more inclusive and effective across the diverse linguistic landscape of Africa.
Techniques for Dialect Identification and Modelling
Innovative methods, such as dialect identification algorithms and deep learning models, are essential for capturing the nuances of dialectal variation within African speech data.
Identifying and modelling dialectal variations within African languages is critical for developing effective speech recognition technologies. This challenge has spurred innovation in the field, leading to the adoption of sophisticated algorithms and deep learning models capable of discerning subtle linguistic differences. Dialect identification techniques, for instance, leverage the unique features of speech, such as intonation, pronunciation, and usage patterns, to classify speech samples into specific dialects. These techniques are instrumental in creating adaptive speech recognition systems that can dynamically adjust to the user’s dialect, significantly enhancing accuracy and user experience.
Deep learning models, particularly those based on neural networks, have shown great promise in capturing the nuances of dialectal variation. These models can learn complex patterns in speech data, allowing them to differentiate between dialects with a high degree of precision.
However, the success of these models hinges on the availability of comprehensive and diverse training data that encapsulates the full range of dialectal variation. As such, efforts to improve dialect identification and modelling must go hand in hand with initiatives to expand and diversify speech datasets. By advancing these techniques, developers can create more robust and flexible speech recognition systems that serve the needs of all speakers, regardless of dialect.
Data Annotation and Transcription Challenges
The process of annotating and transcribing speech data is complicated by dialectal diversity, requiring expert linguists with specific regional knowledge.
The process of annotating and transcribing speech data in a linguistically diverse setting such as Africa is fraught with challenges. Each dialect may have unique linguistic features that must be accurately captured and represented in the dataset. This task requires annotators and transcribers who are not only fluent in the language but also deeply familiar with the specific dialects.
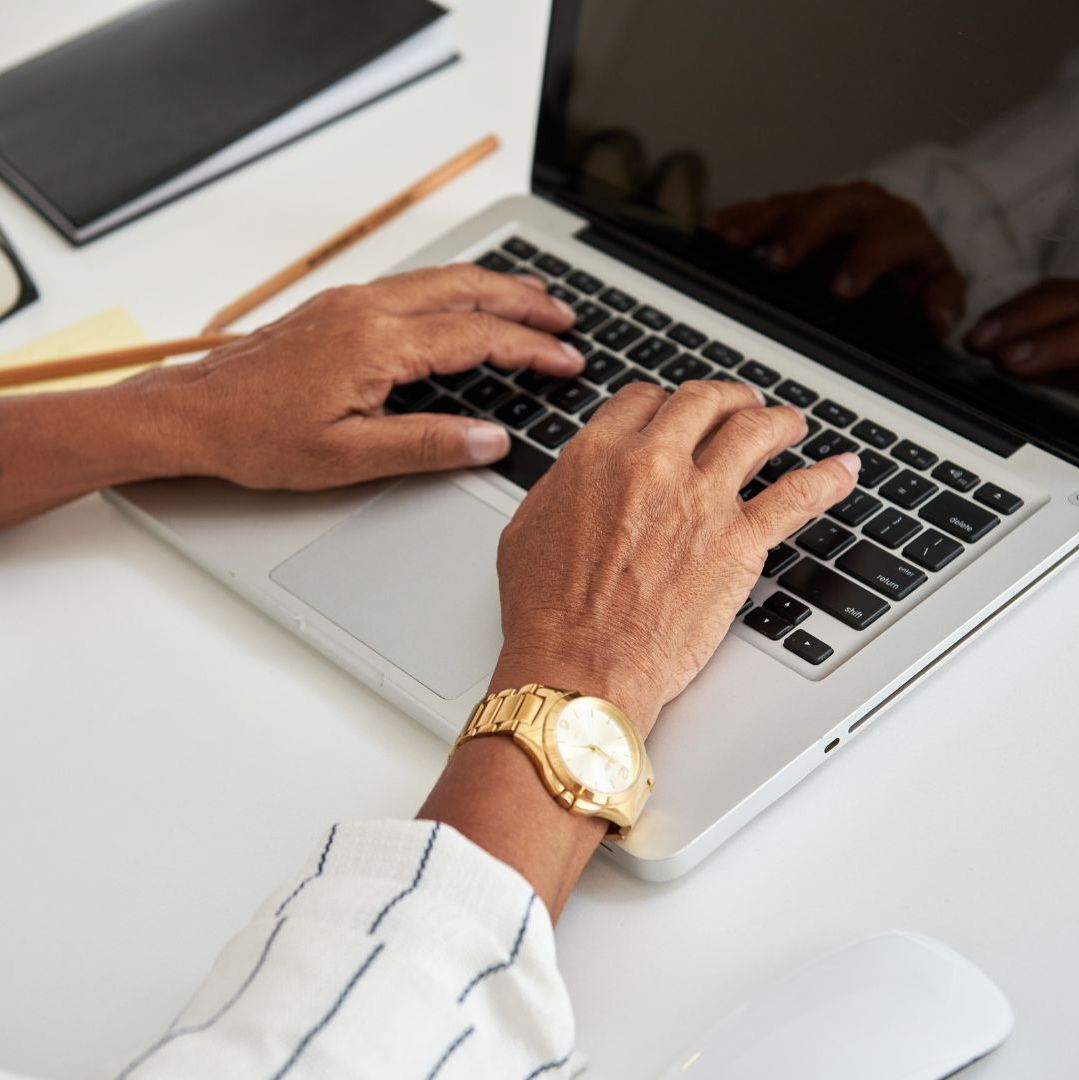
Finding such experts can be difficult, especially for lesser-documented dialects, and necessitates close collaboration with local communities and linguistic institutions. Moreover, the transcription process must account for regional variations in spelling and grammar, further complicating the task. These challenges underscore the need for a tailored approach to data annotation and transcription, one that respects the linguistic diversity of the continent and ensures the highest levels of accuracy and representativeness in speech datasets.
In addition to linguistic expertise, the transcription and annotation of speech data must be guided by rigorous quality control processes to ensure consistency and reliability. This involves establishing clear guidelines for transcription and annotation that account for dialectal variations, as well as regular training and assessment of annotators.
Such measures are critical in minimising errors and biases in the data, which can have significant impacts on the performance of speech recognition technologies. By addressing these challenges with a focus on quality, inclusivity, and linguistic expertise, the field can overcome some of the most significant barriers to developing speech technologies that are truly representative of Africa’s linguistic diversity.
Ethical and Privacy Considerations in Data Collection
Ethical data collection practices must prioritise the privacy and consent of participants, especially in diverse linguistic settings.
Ethical considerations and privacy concerns take on added significance in the collection of speech data in Africa, given the continent’s diverse linguistic landscape and the sensitivity of language to cultural and personal identity. Ensuring that data collection practices are respectful, transparent, and consensual is paramount.
This involves obtaining informed consent from participants, clearly communicating the purpose of the data collection, and how the data will be used. Moreover, it is essential to consider the potential implications of speech data collection on participants’ privacy and the security of their personal information. Data anonymisation and secure data storage practices must be rigorously applied to protect individuals’ identities and personal information.
Beyond these technical measures, ethical data collection also requires a deep understanding of and respect for the cultural and social contexts in which languages are spoken. This understanding can guide the development of data collection strategies that are not only effective but also culturally sensitive and inclusive.
For example, engaging with local communities through trusted intermediaries can facilitate a more ethical approach to data collection, ensuring that it benefits both the technology developers and the communities involved. By prioritising ethical considerations and privacy protection, the field can build trust with participants and communities, which is crucial for the sustainable and responsible development of speech technologies in Africa.
Strategies for Enhancing Data Collection and Application
Collaborating with local communities and linguists, and employing mobile technology for data collection can enhance the inclusivity and accuracy of speech data sets.
The effort to collect and apply speech data effectively in the African context demands innovative strategies that embrace the continent’s linguistic diversity. A central component of these strategies involves forging strong collaborations with local communities and linguists. Such partnerships are crucial for gaining deep insights into the nuances of local dialects and ensuring that data collection efforts are culturally sensitive and linguistically accurate.
Engaging with local experts not only facilitates the gathering of high-quality, dialect-rich speech data but also helps in building trust within communities, encouraging broader participation. Moreover, this collaborative approach allows for the development of speech technologies that are truly reflective of the linguistic diversity of the continent, ensuring that these technologies are accessible and useful to a wider segment of the population.
In parallel, leveraging mobile technology presents a formidable opportunity to enhance the inclusivity and accuracy of speech datasets. Mobile devices are widely used across Africa and can serve as effective tools for collecting speech data from remote and underserved regions. Mobile-based data collection campaigns can be designed to capture a wide range of dialects, offering participants the convenience of contributing speech samples directly from their devices.
This method not only expands the geographical and dialectal coverage of speech datasets but also allows for real-time data collection, ensuring that the datasets are current and reflective of contemporary language use. By integrating mobile technology with community collaboration, data scientists can overcome some of the most significant barriers to collecting diverse and representative speech data, paving the way for the development of more effective and inclusive speech recognition technologies.
The Role of Artificial Intelligence in Addressing Dialectal Variation
AI and machine learning technologies are pivotal in developing adaptable speech recognition systems that can learn from and adjust to dialectal variations.
Artificial Intelligence (AI) and machine learning technologies are at the forefront of tackling the challenges posed by dialectal variation in African languages. These technologies offer the potential to develop speech recognition systems that are not only adaptable but also capable of learning and evolving with the linguistic landscape. By harnessing the power of AI, developers can create models that accurately recognise and process speech from a multitude of dialects, significantly reducing the bias towards more widely spoken or documented languages.
Deep learning algorithms, in particular, have shown great promise in identifying subtle linguistic features that distinguish one dialect from another, enabling more precise and nuanced recognition of spoken language. This adaptability is crucial for creating speech technologies that can serve the diverse needs of the African population, ensuring that no dialect is left behind.
Moreover, AI and machine learning are instrumental in automating the process of dialect identification and transcription, which are key steps in the creation of speech datasets. Through advanced algorithms, speech samples can be automatically categorised by dialect, streamlining the data annotation process and enhancing the accuracy of the resulting datasets.
Furthermore, machine learning models can be trained to transcribe speech accurately, even in dialects for which written standards are not well-established. This capability not only accelerates the development of speech recognition technologies but also contributes to the documentation and preservation of linguistic diversity. As AI technologies continue to advance, their role in addressing dialectal variation will become increasingly significant, offering new avenues for innovation in speech technology development.
Case Studies: Successes and Challenges
Examples from projects in African countries highlight both the potential and the hurdles in deploying speech recognition technologies across diverse dialects.
Exploring case studies from various African countries illuminates both the successes and challenges encountered in deploying speech recognition technologies across diverse dialects. For instance, projects aimed at creating speech-to-text applications for underrepresented languages have demonstrated the potential of AI and machine learning to bridge the gap in technological accessibility.
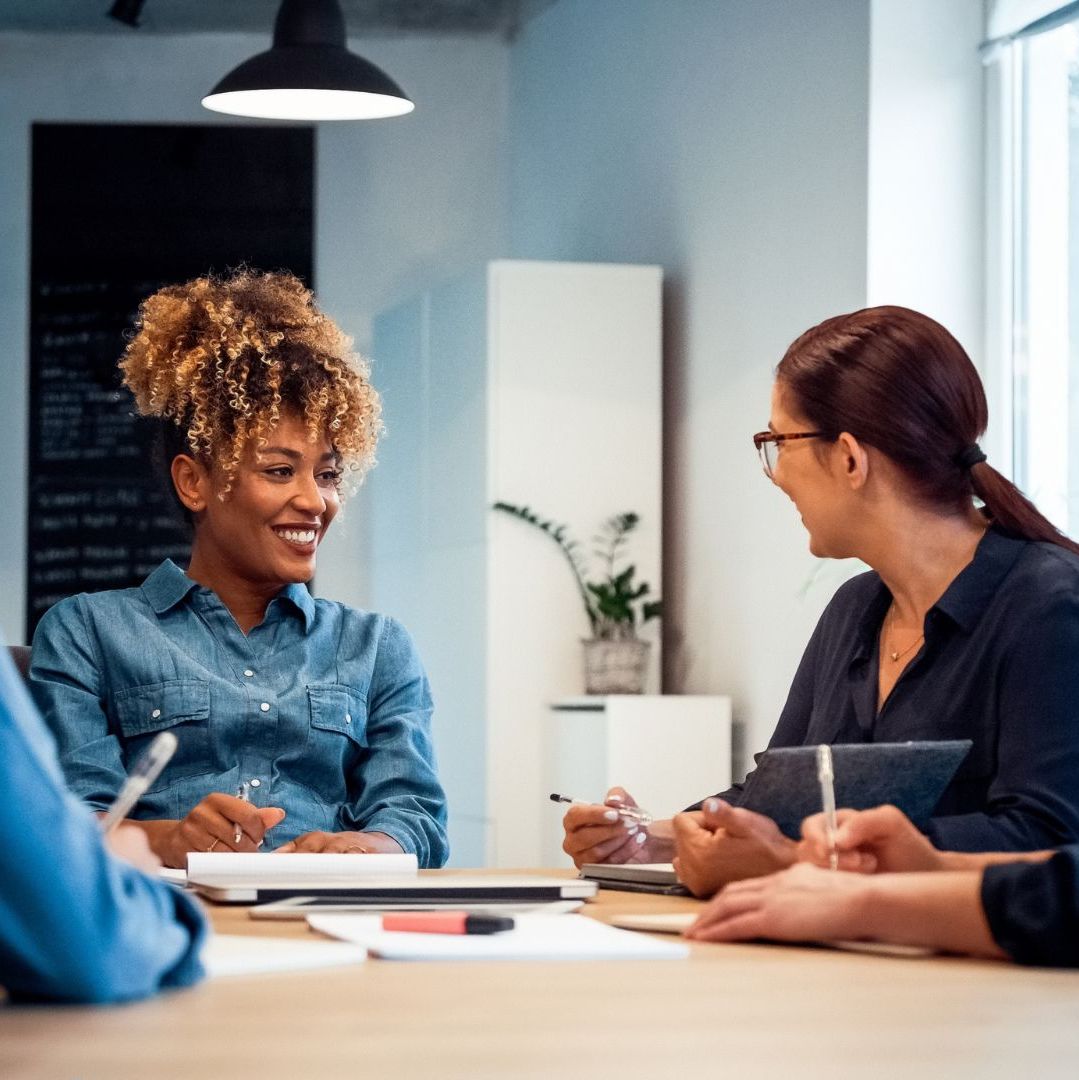
In countries like Kenya and Nigeria, where multiple languages and dialects coexist, localised speech recognition applications have enabled more efficient communication and access to digital services for speakers of indigenous languages. These successes underscore the importance of localised data collection and the development of AI models trained specifically on the linguistic features of target dialects.
However, these projects also highlight significant challenges, such as the difficulty of collecting sufficient and diverse speech data in environments where resources are limited, and digital literacy is varied. Additionally, the variability of dialects within a single language can present obstacles to achieving high accuracy in speech recognition, particularly for dialects with fewer speakers or those that are geographically isolated.
These challenges emphasise the need for continued innovation in AI and data collection methodologies, as well as the importance of community engagement and support from local governments and institutions. By addressing these challenges, future projects can build on the successes of previous efforts, further advancing the inclusivity and effectiveness of speech recognition technologies in Africa.
Future Directions in Speech Technology for African Languages
The future holds promising advancements in AI that could revolutionise speech technology, making it more inclusive of dialectal diversity within African languages.
The future of speech technology for African languages is poised for significant advancements, driven by breakthroughs in AI and a growing recognition of the need for linguistic inclusivity. As AI technologies become more sophisticated, their ability to learn from and adapt to the rich tapestry of African dialects will improve, opening up new possibilities for speech recognition applications that are truly universal in their reach. These advancements are expected to not only enhance the accuracy and reliability of speech technologies but also to expand their applications, from education and healthcare to commerce and governance, thereby contributing to societal advancement and digital inclusivity.
Moreover, the future direction of speech technology in Africa is likely to emphasise ethical AI development and the protection of linguistic diversity. This includes ensuring that the development of speech technologies does not inadvertently lead to the erosion of linguistic identities but rather supports the preservation and appreciation of Africa’s linguistic heritage.
Collaborative efforts between technologists, linguists, and local communities will be key in shaping these technologies to meet the specific needs and preferences of users across the continent. As we look to the future, the potential of AI to revolutionise speech technology for African languages is immense, promising a more connected and linguistically inclusive world.
Key Tips For Dialect Diversity in African Languages
- Ensuring regional diversity in speech data collection to represent various dialects.
- Employing advanced AI techniques for dialect identification and adaptation.
- Prioritising ethical and privacy considerations in data collection efforts.
- Collaborating with local experts and communities for accurate data annotation.
- Leveraging mobile technology to facilitate widespread data collection.
Way With Words provides highly customised and appropriate speech data collections for African languages, addressing these challenges and employing strategies to enhance speech technology applications. Our services include:
- African Language Speech Collection Solution: We create custom speech datasets for African languages, including transcripts for machine learning purposes, facilitating the creation or improvement of ASR models using NLP for select African languages across various domains.
- Machine Transcription Polishing of Captured Speech Data: Our MTP service is utilised across numerous technologies, polishing machine transcripts for AI and machine learning purposes in various African languages, supporting applications in research, FinTech, SaaS, and more.
Some Final Thoughts Regarding Dialectical Variation Within African Languages
The dialectal diversity within African languages presents both a significant challenge and a remarkable opportunity for the advancement of speech technology. To harness the full potential of speech data collection and application in such a varied linguistic landscape, it is essential to adopt strategies that ensure inclusivity, accuracy, and ethical consideration.
By leveraging local expertise, innovative AI techniques, and collaborative efforts, it is possible to overcome the barriers posed by dialectal variation. The key piece of advice for stakeholders in this field is to remain adaptable and open to the rich linguistic complexity of Africa, viewing it not as an obstacle but as a valuable resource for technological innovation and cultural preservation.
Read more on this thought topic – The Many Languages of Africa: A Mosaic of Linguistic Diversity.