Challenges Dialects Present to Speech Recognition Systems in African languages
What Challenges do Accents and Dialects Present to Speech Recognition Systems in African Languages?
The development and refinement of speech recognition systems have become pivotal in bridging communication gaps and fostering global digital inclusion. However, when it comes to African languages, these systems face unique challenges due to the rich tapestry of accents and dialects present across the continent. This diversity, while a cultural treasure, presents a significant hurdle for AI-driven speech recognition technologies aiming for accuracy and inclusivity.
Key questions that arise in addressing this challenge include:
- How do variations in pronunciation and vocabulary across African dialects affect speech recognition accuracy?
- What are the technical and data-related challenges in training AI models to understand and process these languages?
- How can developers and linguists work together to create more inclusive and accurate speech recognition systems for African languages?
These questions underscore the importance of a nuanced approach to developing speech recognition technologies that are both sensitive to and capable of navigating the linguistic diversity of Africa.
African Dialects Speech Recognition Challenges and Solutions
Diversity of African Languages
African languages exhibit a vast range of phonetic, syntactic, and lexical diversity, which poses a significant challenge for speech recognition systems designed with a one-size-fits-all approach.
The African continent is a mosaic of linguistic diversity, home to thousands of languages that span several major language families, including Afroasiatic, Nilo-Saharan, Niger-Congo, and Khoisan. Each of these families encompasses languages that differ dramatically not only in phonetics—the sounds used in speech—but also in syntax—the structure of sentences—and lexicon—the vocabulary.
Such diversity presents a colossal challenge for speech recognition systems, which are often developed with a more homogenous linguistic model in mind. These systems struggle to adapt to the myriad sounds and structures of African languages, from the tonal variations in Yoruba to the click consonants in Xhosa, leading to significant inaccuracies in voice recognition and processing.
Moreover, the lexical richness of African languages, where a single term can have multiple meanings depending on context, poses additional challenges. Many African languages are highly context-dependent, with meaning derived from not just the words themselves but the tone, pitch, and even the pace at which they are spoken.
This level of linguistic nuance is difficult for current speech recognition technologies to capture and interpret accurately, necessitating a shift towards more adaptable, context-aware AI models. Without a deep understanding and integration of these complex linguistic traits, speech recognition systems are likely to remain ineffective for a significant portion of Africa’s linguistic tapestry, sidelining many users and communities.
Data Scarcity for African Languages
The lack of extensive, high-quality speech datasets for many African languages limits the ability of machine learning models to learn the nuances of these languages, affecting recognition accuracy.
The development and refinement of speech recognition technologies heavily depend on access to large, diverse datasets of spoken language. Unfortunately, for many African languages, such datasets are scarce or, in some cases, non-existent. This data scarcity is a significant barrier to the development of effective speech recognition systems for African languages, as machine learning models rely on extensive datasets to learn the nuances of a language.
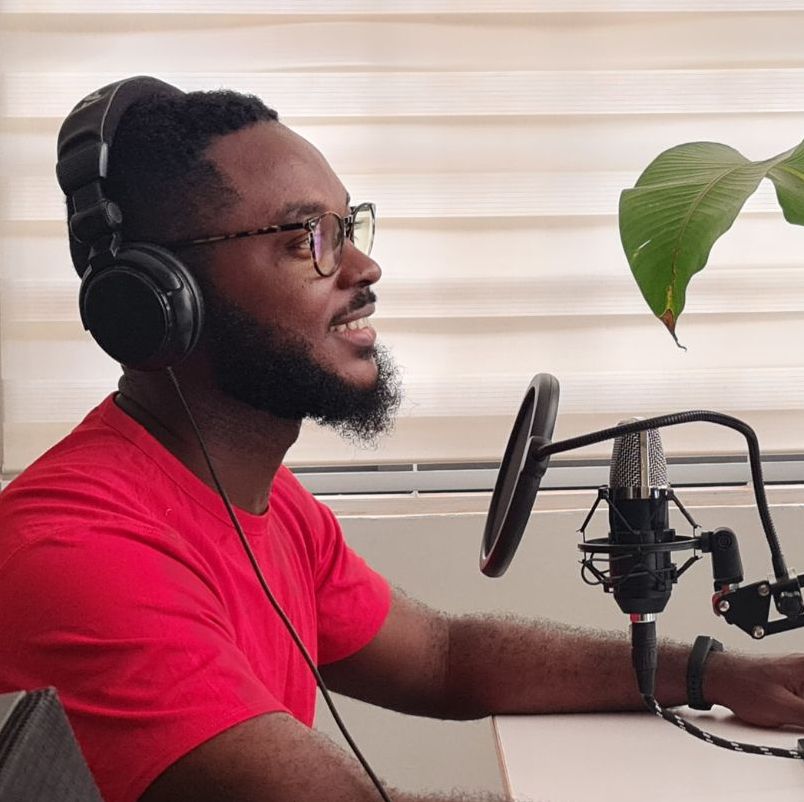
The absence of robust datasets results in models that are undertrained for the vast array of African languages, leading to poor recognition accuracy and a lack of usability for speakers of these languages. Addressing this issue requires a concerted effort to collect and compile speech data across the continent’s languages, a task that involves overcoming logistical, technical, and sometimes political challenges.
Collecting speech data in African languages is not just about quantity but also quality and representation. It requires capturing the diverse accents, dialects, and idiomatic expressions that characterise these languages, ensuring that the datasets reflect the linguistic reality of their speakers. Initiatives to gather such data must be inclusive, engaging communities in the data collection process and respecting cultural sensitivities and privacy concerns, laying the groundwork for more accurate and inclusive speech recognition technologies.
Accents and Dialects Variability
Within single African languages, there can be significant variations in accent and dialect, which can lead to misinterpretation or misunderstanding by speech recognition systems.
Accents and dialects within individual African languages introduce another layer of complexity to the development of speech recognition systems. Even within a single language, the pronunciation, vocabulary, and grammar can vary significantly from one region to another, or even among different social groups within the same region. These variations can lead to misunderstandings and misinterpretations by speech recognition systems not finely tuned to recognise such differences. For example, a speech recognition system trained predominantly on data from urban speakers of Swahili may struggle to accurately interpret utterances from rural dialects of the same language.
The challenge, then, is to develop speech recognition technologies capable of understanding and processing this intra-linguistic diversity. This requires not only more granular data collection efforts that capture the spectrum of dialectical variations but also the development of AI models that can adapt to and learn from these variations.
Innovations in machine learning, such as few-shot learning and transfer learning, offer promising avenues for creating more flexible and adaptable speech recognition systems. By leveraging these technologies, developers can create systems that better understand the rich tapestry of accents and dialects across African languages, improving accuracy and usability for a broader range of speakers.
Technical Limitations and Infrastructure
Many speech recognition technologies are developed and optimised for languages with extensive digital resources, leaving African languages underrepresented and inadequately supported due to technical and infrastructural limitations.
Many speech recognition technologies are initially developed with a focus on languages that boast extensive digital resources and infrastructure, such as English, Mandarin, or Spanish. This focus leaves African languages significantly underrepresented and unsupported, not due to a lack of complexity or global importance, but because of technical and infrastructural limitations.
The challenge extends beyond the mere availability of digital resources; it encompasses the broader digital divide that affects many African countries, where access to technology and the internet is not as ubiquitous as in other parts of the world. This gap hinders not only the collection of necessary linguistic data but also the deployment and utilisation of speech recognition technologies by the very populations that stand to benefit from them the most.
Addressing these technical and infrastructural challenges requires a multi-faceted approach. On the one hand, there’s a need for investment in digital infrastructure to ensure broader access to technology across the continent. On the other, speech recognition technologies themselves must be optimised to perform well under a variety of conditions, including low-resource environments.
This might involve developing lightweight models that require less computational power and can run on a wider range of devices, or creating offline versions of speech recognition systems that can function without constant internet access. By adapting technologies to the specific conditions prevalent in many African contexts, developers can help bridge the gap and bring the benefits of speech recognition to a wider audience.
Sociolinguistic Factors
Social and regional linguistic variations add another layer of complexity to the development of speech recognition systems that can accurately interpret African languages.
The sociolinguistic landscape of Africa adds another layer of complexity to the development of effective speech recognition systems. Language in Africa is deeply intertwined with social identity, regional differences, and cultural practices, leading to a dynamic and fluid linguistic environment. Sociolinguistic factors, such as the use of language in specific social contexts, regional idioms, and the influence of social hierarchies on speech patterns, must be considered in the development of speech recognition technologies.
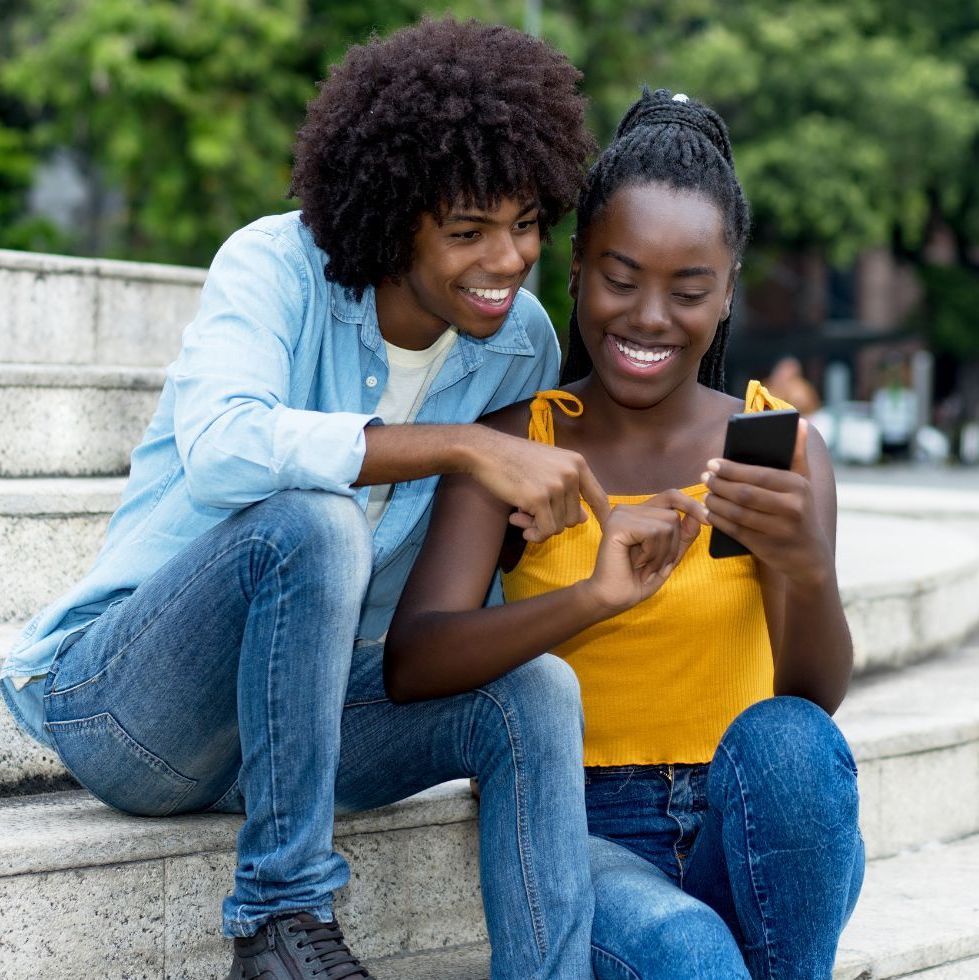
These factors can significantly impact the way language is used and understood, which, in turn, affects how speech recognition systems interpret and process language. Developing speech recognition technologies that can navigate these sociolinguistic nuances requires a deep understanding of the cultural and social contexts in which African languages are spoken. It involves more than just technical proficiency; it requires a sensitivity to and appreciation for the cultural dimensions of language use.
Collaboration with sociolinguists, anthropologists, and local communities can provide valuable insights into these contexts, informing the development of more nuanced and effective speech recognition systems. By incorporating sociolinguistic factors into the design and development of these technologies, developers can create systems that are not only technically accurate but also culturally sensitive and inclusive.
The Role of Code-Switching
Code-switching, a common practice among African language speakers, presents additional challenges for speech recognition systems that struggle to navigate between multiple languages within a single utterance.
Code-switching, the practice of alternating between two or more languages or dialects within a single conversation or even a single sentence, is common among speakers of African languages. This linguistic fluidity poses a significant challenge for speech recognition systems, which often struggle to accurately recognise and process mixed-language speech. Traditional speech recognition models are typically designed to handle one language at a time, leading to errors and inaccuracies when confronted with the complexity of code-switched speech. This limitation is particularly problematic in African contexts, where multilingualism is the norm and code-switching a routine part of linguistic practice.
To effectively address the challenges posed by code-switching, speech recognition technologies need to become more sophisticated and flexible. This involves training models on datasets that include code-switched speech and developing algorithms capable of identifying and processing multiple languages simultaneously. Advances in natural language processing (NLP) and machine learning are paving the way for such developments, enabling the creation of more adaptable and intelligent speech recognition systems. By embracing the linguistic reality of code-switching, these technologies can better serve the needs of multilingual speakers, enhancing communication and accessibility for users across Africa.
Need for Inclusive Training Data
The importance of creating inclusive, diverse datasets that represent the full spectrum of accents, dialects, and linguistic nuances within African languages cannot be overstated.
The effectiveness of speech recognition systems hinges on the quality and diversity of the training data they are built upon. For African languages, the need for inclusive, representative datasets is particularly acute. These datasets must encompass the full spectrum of linguistic variation found across the continent, from the different accents and dialects of a single language to the diverse languages spoken within a region.
Currently, the scarcity of such inclusive datasets is a major obstacle to developing speech recognition systems that accurately reflect the linguistic diversity of Africa. Without access to comprehensive and diverse linguistic data, these systems are at risk of perpetuating biases and excluding many speakers from the benefits of speech technology.
Creating inclusive training data for African languages requires a concerted effort to collect and annotate speech from a wide range of speakers, regions, and social contexts. This effort should prioritise not only linguistic diversity but also inclusivity in terms of age, gender, and socioeconomic status, ensuring that the resulting speech recognition systems are accessible and usable for all.
Collaboration with local communities, linguists, and language experts is essential in this process, providing the cultural and linguistic insights needed to build representative datasets. By investing in the creation of inclusive training data, developers can lay the groundwork for speech recognition technologies that are more accurate, fair, and inclusive, unlocking the potential of these technologies for users across Africa.
Advances in AI and Machine Learning
Recent advances in AI and machine learning offer new opportunities for improving speech recognition accuracy for African languages, but these technologies require tailored approaches and datasets.
Recent advances in artificial intelligence (AI) and machine learning are opening new avenues for improving speech recognition accuracy for African languages. These technologies are becoming increasingly adept at handling the complexity and variability of human language, offering promising solutions to the challenges posed by linguistic diversity.
Machine learning models, such as deep neural networks, have shown remarkable progress in learning from large datasets, enabling them to recognise and interpret a wide range of linguistic patterns. For African languages, these advances provide an opportunity to overcome some of the traditional barriers to effective speech recognition, such as the scarcity of training data and the complexity of accents and dialects.
To fully leverage these technological advancements, however, a tailored approach is required. This means not only developing and training models specifically for African languages but also ensuring that these models are adaptable and flexible enough to handle the linguistic diversity encountered across the continent. Innovations in AI and machine learning, such as transfer learning and unsupervised learning techniques, offer potential pathways for achieving this. By adopting these advanced methodologies, developers can create speech recognition systems that are more responsive to the nuances of African languages, enhancing accuracy and usability for speakers of these languages.
Collaboration Between Linguists and Developers
Effective collaboration between linguists specialising in African languages and AI developers is crucial for overcoming the challenges presented by accents and dialects in speech recognition.
The development of effective speech recognition systems for African languages requires a collaborative effort between linguists specialising in these languages and AI developers. Linguists bring a deep understanding of the linguistic structures, variations, and cultural contexts of African languages, insights that are crucial for addressing the challenges of speech recognition in these languages.
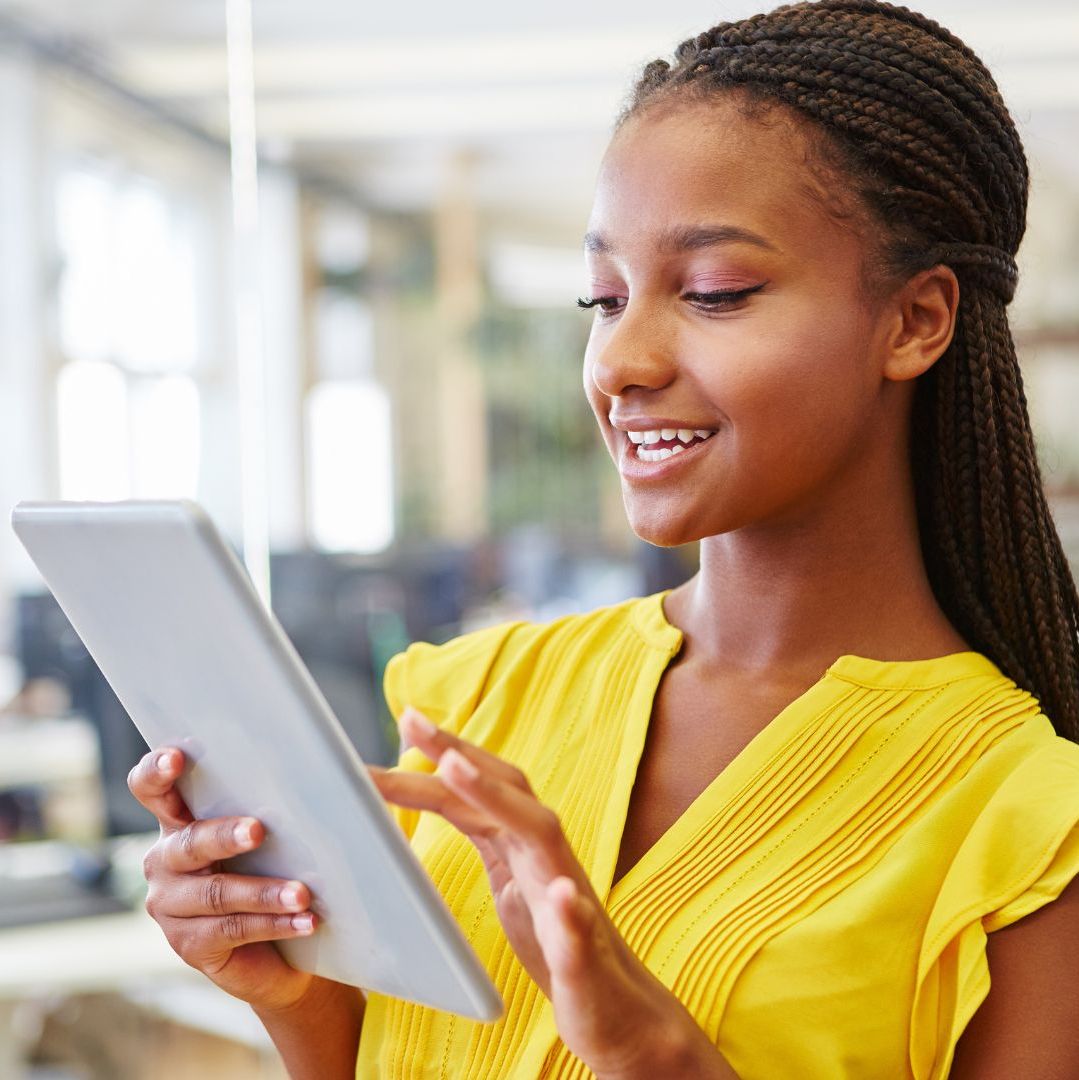
Developers, on the other hand, bring technical expertise in AI and machine learning, enabling the translation of linguistic knowledge into practical technological solutions. Together, linguists and developers can bridge the gap between linguistic complexity and technological capability, creating speech recognition systems that are both accurate and culturally sensitive.
This collaboration can take many forms, from joint research projects to interdisciplinary teams within technology companies. Regardless of the specific format, the key is to foster an environment of mutual learning and respect, where linguistic insights inform technological development and vice versa. Such collaboration can lead to innovative approaches to speech recognition, leveraging the strengths of both fields to tackle the unique challenges presented by African languages. By working together, linguists and developers can push the boundaries of what is possible in speech technology, opening up new possibilities for communication and digital inclusion across Africa.
Ethical Considerations and Representation
Ethical considerations around representation, privacy, and consent in the collection and use of speech data are paramount, particularly when dealing with the linguistic diversity of African languages.
Ethical considerations are paramount in the development and deployment of speech recognition technologies, especially in the context of the diverse linguistic landscape of Africa. Issues of representation, privacy, and consent are at the forefront, as the collection and use of speech data raise important questions about who is being recorded, how this data is being used, and who ultimately benefits from these technologies. Ensuring ethical practices in speech technology requires a commitment to transparency, inclusivity, and respect for the rights and dignity of all individuals.
Representation is a critical aspect of this ethical framework, as speech recognition systems must accurately reflect the linguistic diversity of their intended users. This means actively working to include underrepresented languages and dialects in speech data collections, thereby avoiding biases that could exclude or marginalise certain speakers. Privacy and consent are equally important, with clear protocols needed to protect the personal information of those whose voices are recorded. This includes obtaining informed consent from participants, securing data against unauthorised access, and ensuring that the use of speech data aligns with ethical standards and local regulations.
Addressing these ethical considerations requires a proactive approach from developers, researchers, and companies involved in speech technology. By prioritising ethical practices in the collection, use, and development of speech recognition systems, stakeholders can ensure that these technologies are developed in a way that respects the rights and reflects the diversity of speakers across Africa. Ethical speech technology is not only a matter of technical achievement but also a commitment to social responsibility, paving the way for more equitable and inclusive digital futures.
Key Thoughts on Data Collection for Speech Recognition Systems in African Languages
- Prioritise the creation of extensive, diverse datasets for African languages.
- Collaborate with linguists and communities to ensure accurate representation of dialects and accents.
- Utilise recent AI and machine learning advances to tailor speech recognition technologies to the specific needs of African languages.
- Address ethical considerations in speech data collection and use, focusing on consent, privacy, and representation.
- Way With Words provides highly customised and appropriate speech data collections for African languages, addressing these challenges through comprehensive services tailored to the needs of technologies targeting African languages.
It becomes evident that the path forward is both challenging and ripe with opportunity. The diversity of accents and dialects, while presenting significant hurdles, also offers a unique chance to innovate and push the boundaries of what these technologies can achieve in terms of inclusivity and accuracy. The key to unlocking this potential lies in recognising the value of collaboration between linguists, developers, and communities, and in leveraging the latest advancements in AI and machine learning.
The main content subtopics highlight the multifaceted nature of the challenges at hand, from the need for diverse and inclusive training data to the technical and ethical considerations that underpin this work. These challenges are not insurmountable; rather, they invite a reimagining of how speech recognition technologies are developed and deployed, with a strong emphasis on tailoring solutions to the rich linguistic landscape of Africa.
A key piece of advice for developers, linguists, and industries invested in this space is to approach the task with humility, openness, and a commitment to ethical practices. By doing so, we can ensure that speech recognition technologies not only advance in technical capability but also in their capacity to serve and empower all users, irrespective of language or dialect.
Some African Speech Data Resources
- Global African Speech Data Collection Resource: https://speechcollection.co.za/ This comprehensive service offers access to essential information and materials for gathering African speech data on an international scale.
- African Language Speech Collection Solution: https://waywithwords.net/services/speech-collection/ We create custom speech datasets for African languages for machine learning purposes, improving ASR models using NLP.
- Machine Transcription Polishing of Captured Speech Data: https://waywithwords.net/services/machine-transcription-polishing/ Our service polishes machine transcripts across technologies for AI and machine learning in African languages.