Unlocking Free Speech Data Resources: A Guide for AI Enthusiasts
Are There Any Free Resources For Speech Data?
In recent years, the demand for speech data has skyrocketed, driven by advancements in artificial intelligence (AI) and machine learning (ML). From voice recognition systems to language processing applications, all types of speech data play a crucial role in developing accurate and efficient models. However, obtaining high-quality speech data can be costly, posing a significant barrier for many AI developers, data scientists, and tech startups.
This guide aims to address the common question: Are there any free resources for speech data?
Understanding the availability of free speech data resources is essential for professionals and researchers looking to build and enhance AI models without breaking the bank. The availability of open-source and free resources can significantly reduce costs and democratise access to cutting-edge technologies. However, navigating these resources comes with its own set of challenges. In this guide, we will explore the top free speech data resources, their benefits and limitations, and how to make the most of open-source speech data.
Common Questions:
- Where can I find free speech data for my AI project?
- What are the limitations of using free speech data compared to paid options?
- How do I effectively utilise open-source speech data for machine learning models?
Top 10 Free Resources for Speech Data
(Note: These resources may not all be available or correct anymore at the time of reading)
Common Voice by Mozilla
Mozilla’s Common Voice project is one of the largest open-source speech datasets available. It offers a diverse range of voices across multiple languages. The dataset is crowdsourced, meaning it includes contributions from volunteers worldwide, making it ideal for projects that require varied speech patterns and accents. This resource is particularly valuable for training speech recognition models.
Mozilla’s Common Voice project is a groundbreaking initiative aimed at democratising access to speech data. The project’s crowdsourced nature means that anyone can contribute, making it one of the most diverse datasets available. It includes voices from various age groups, genders, and accents, which is invaluable for training robust speech recognition models. This diversity helps models generalise better across different speakers, reducing bias that can often be present in more homogenous datasets.
One of the key advantages of Common Voice is its accessibility. The dataset is freely available under a Creative Commons license, allowing developers to use it for both research and commercial purposes. This is particularly beneficial for startups and smaller organisations that may not have the budget for expensive proprietary datasets. Additionally, Mozilla provides a user-friendly interface for contributors, encouraging participation and ensuring that the dataset continues to grow.
However, the crowdsourced nature of Common Voice also presents some challenges. The quality of recordings can vary, as they are recorded using different devices in various environments. While this variability can be useful for creating robust models, it may require additional preprocessing to ensure consistent audio quality. Despite these challenges, Common Voice remains one of the most comprehensive and accessible speech datasets available, making it a go-to resource for many AI developers and researchers.
LibriSpeech
LibriSpeech is a well-known dataset derived from audiobooks, offering over 1,000 hours of English speech data. It is widely used in academic and research settings for developing and benchmarking speech recognition systems. The dataset’s high-quality audio and well-annotated transcriptions make it a reliable resource for AI developers and researchers.
LibriSpeech stands out as one of the most widely used speech datasets in the AI community, particularly for English language tasks. The dataset is derived from public domain audiobooks, which means that it features high-quality recordings of spoken English. The advantage of using audiobook data is that it often contains well-enunciated speech, which is beneficial for training models that require clear and precise audio input.
In addition to its high-quality recordings, LibriSpeech is also praised for its extensive metadata and well-annotated transcriptions. The dataset includes phonetic transcriptions, word-level alignments, and other valuable information that can be used to fine-tune models. This makes it a popular choice for academic research, where precise data is crucial for testing and benchmarking speech recognition systems. Moreover, the dataset is split into training, validation, and test sets, enabling researchers to evaluate their models in a structured manner.
Despite its strengths, LibriSpeech does have some limitations. Because it is derived from audiobooks, the speech patterns may not fully represent everyday conversational speech. Additionally, the dataset primarily features American English, which may not be suitable for projects that require more linguistic diversity. Nevertheless, for tasks that demand high-quality English speech data, LibriSpeech remains an essential resource.
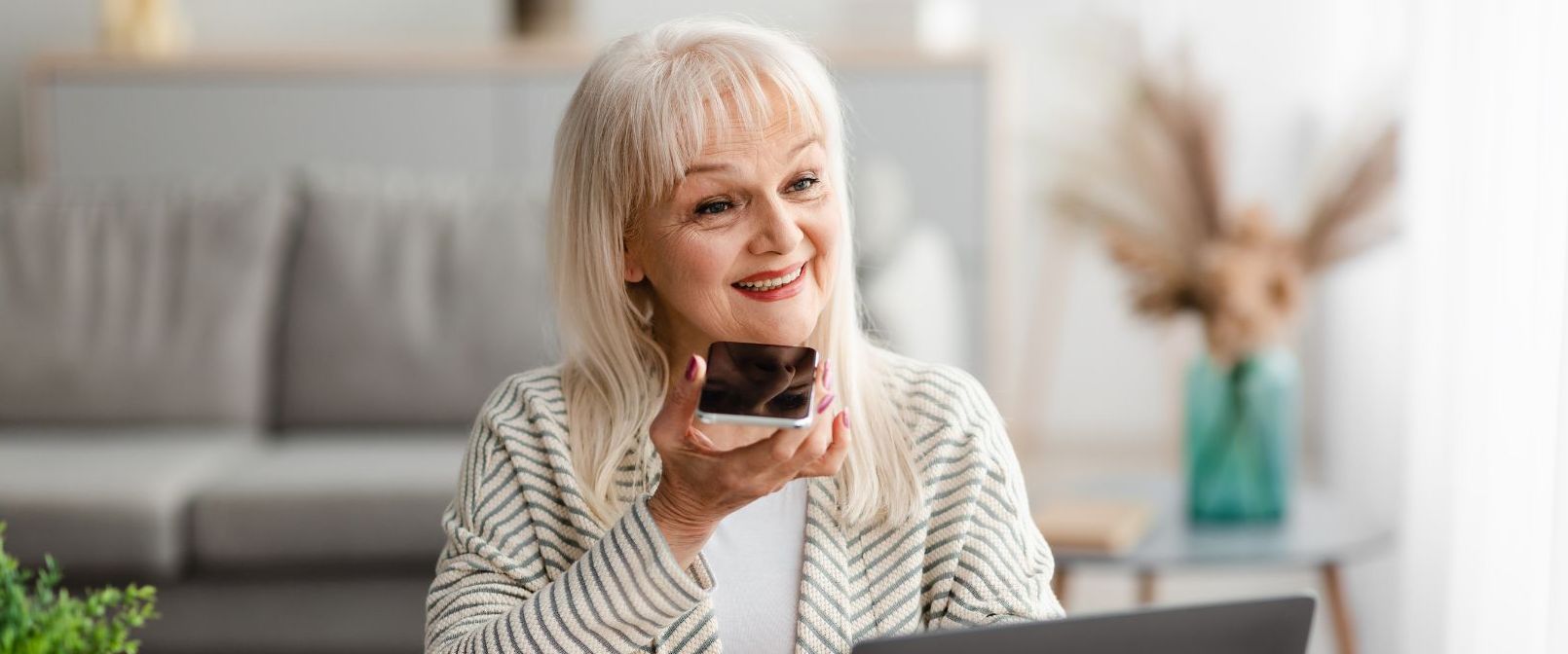
Google Speech Commands Dataset
Google’s Speech Commands dataset is designed for training models to recognise simple voice commands. It includes thousands of recordings of short phrases like “yes,” “no,” “stop,” and “go.” This dataset is particularly useful for developing voice-activated devices and applications that require simple, straightforward commands.
The Google Speech Commands dataset is tailored for specific use cases, particularly in the realm of voice-activated devices and applications. This dataset includes thousands of recordings of short phrases, making it ideal for tasks that involve recognising simple voice commands. Its focused nature allows developers to train models quickly and efficiently, without the need for extensive preprocessing or annotation.
One of the major benefits of the Google Speech Commands dataset is its simplicity. The dataset is designed to be easy to use, with clear labels and consistent audio quality. This makes it a popular choice for developers who are building applications for smart home devices, mobile apps, and other consumer electronics. The dataset’s focus on short phrases also means that it can be used for real-time applications, where low latency is essential.
However, the simplicity of the Google Speech Commands dataset can also be a limitation. It is not suitable for more complex speech recognition tasks, such as understanding full sentences or detecting nuanced speech patterns. Additionally, the dataset primarily features English phrases, which may not be ideal for multilingual projects. Despite these limitations, the Google Speech Commands dataset is a valuable resource for developers working on voice-activated applications.
VoxForge
VoxForge is an open-source project that collects transcribed speech for use in acoustic model training. The dataset includes contributions in various languages, making it a valuable resource for multilingual AI projects. Although it may not be as extensive as some other datasets, VoxForge is a great starting point for developing speech recognition models.
VoxForge is a community-driven project that aims to create open-source acoustic models for speech recognition. The project encourages users to submit recordings of their speech, which are then transcribed and made available to the public. This crowdsourced approach has resulted in a diverse dataset that includes various languages and accents, making it a valuable resource for multilingual AI projects.
One of the key strengths of VoxForge is its focus on open-source collaboration. The project is built on the idea that speech data should be freely available to everyone, and it has attracted a dedicated community of contributors. This collaborative spirit has led to the creation of a dataset that is constantly evolving and expanding, with new recordings being added regularly.
However, like other crowdsourced datasets, VoxForge faces challenges related to data quality and consistency. The recordings are made using a wide range of devices, which can result in varying audio quality. Additionally, the transcriptions are provided by volunteers, which means that they may not always be perfectly accurate. Despite these challenges, VoxForge remains a valuable resource for developers who need access to diverse and open-source speech data.
TED-LIUM
TED-LIUM is a dataset created from TED Talks, offering high-quality audio and transcriptions. This resource is ideal for projects that require a diverse range of topics and speakers, as TED Talks cover a wide array of subjects delivered by speakers from around the globe. The dataset is also used extensively in academic research and competitions.
The TED-LIUM dataset is derived from TED Talks, which are known for their high production quality and engaging content. This makes TED-LIUM an excellent resource for speech recognition projects that require clear and well-articulated speech. The dataset includes both the audio recordings and their corresponding transcriptions, providing a comprehensive resource for training and testing speech recognition models.
One of the unique aspects of TED-LIUM is its diversity in terms of both speakers and topics. TED Talks cover a wide range of subjects, from technology and science to arts and culture. This diversity allows models trained on TED-LIUM to generalise better to different types of speech and content. Additionally, the dataset includes speakers from various linguistic and cultural backgrounds, making it useful for projects that require a broad representation of voices.
However, TED-LIUM is not without its limitations. Because the dataset is based on TED Talks, the speech may be more formal and rehearsed compared to everyday conversation. This can make it less suitable for projects that require more natural, spontaneous speech. Despite these limitations, TED-LIUM is a valuable resource for academic research and commercial applications alike.
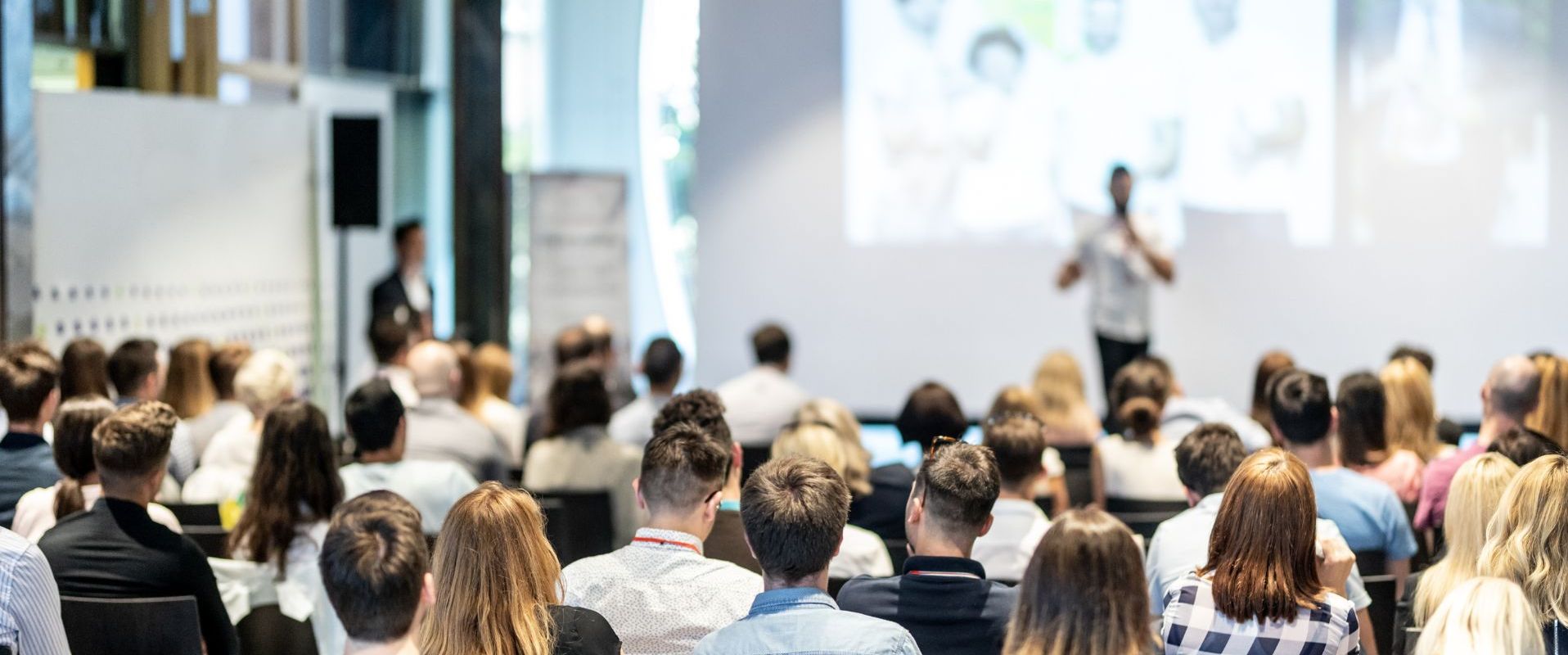
OpenSLR
Open Speech and Language Resources (OpenSLR) is a collection of datasets and tools for speech and language processing. It includes a variety of speech data, from read speech to conversational speech, in multiple languages. OpenSLR is a versatile resource for AI developers and machine learning engineers working on speech-related projects.
OpenSLR is a versatile resource that provides a wide range of datasets for speech and language processing. Unlike some other datasets, OpenSLR offers both read and conversational speech, making it suitable for a variety of projects. The collection includes data in multiple languages, which is particularly valuable for developers working on multilingual AI systems.
One of the key advantages of OpenSLR is its breadth and variety. The platform offers datasets for specific languages, dialects, and speech types, allowing developers to choose the data that best fits their needs. Additionally, OpenSLR includes tools and resources for processing and analysing the data, making it easier for developers to integrate the datasets into their projects.
However, the variety of datasets available on OpenSLR can also be a challenge. Some datasets may require significant preprocessing or cleaning before they can be used effectively. Additionally, the quality of the data can vary, depending on the source and the recording conditions. Despite these challenges, OpenSLR remains a valuable resource for developers and researchers who need access to a wide range of speech data.
Tatoeba Project
The Tatoeba Project offers a large collection of sentences recorded in various languages. While it primarily focuses on translation, the recorded sentences can be used for speech recognition and synthesis tasks. The dataset is crowdsourced, and the audio quality may vary, but it is still a valuable resource for multilingual projects.
The Tatoeba Project is a collaborative initiative that focuses on creating a large, multilingual database of sentences and their translations. While the project is primarily aimed at language learners and translators, the recorded sentences can also be used for speech recognition and synthesis tasks. The dataset includes contributions from volunteers, making it a diverse resource that covers a wide range of languages.
One of the main benefits of the Tatoeba Project is its multilingual nature. The dataset includes sentences in hundreds of languages, making it an invaluable resource for developers working on projects that require speech data in less commonly spoken languages. Additionally, the dataset is freely available under a Creative Commons license, allowing developers to use it for both research and commercial purposes.
However, the crowdsourced nature of the Tatoeba Project means that the quality of the recordings can vary. Some sentences may be recorded in noisy environments or with low-quality equipment, which can affect their usability for speech recognition tasks. Despite these challenges, the Tatoeba Project is a valuable resource for developers who need access to multilingual speech data.
CHiME Challenges
The CHiME Challenges are a series of competitions focused on robust speech recognition in challenging environments, such as noisy or reverberant settings. The datasets released as part of these challenges include high-quality speech recordings in various acoustic conditions, making them ideal for developing and testing robust speech recognition systems.
The CHiME Challenges are a series of competitions that focus on developing robust speech recognition systems in challenging acoustic environments. The datasets released as part of these challenges include recordings made in noisy or reverberant conditions, making them ideal for projects that require robust speech recognition capabilities. The challenges are designed to push the boundaries of what is possible in speech recognition, making them a valuable resource for cutting-edge research.
One of the key strengths of the CHiME datasets is their focus on real-world scenarios. The recordings include a wide range of background noises, from street sounds to office environments, making them ideal for training models that need to perform well in noisy conditions. Additionally, the datasets include detailed annotations and transcriptions, making them a valuable resource for both training and evaluation.
However, the challenging nature of the CHiME datasets means that they may not be suitable for all projects. The noisy conditions can make it difficult to achieve high accuracy, particularly for models that are not specifically designed for robust speech recognition. Despite these challenges, the CHiME datasets are a valuable resource for developers who need to train and test their models in real-world conditions.
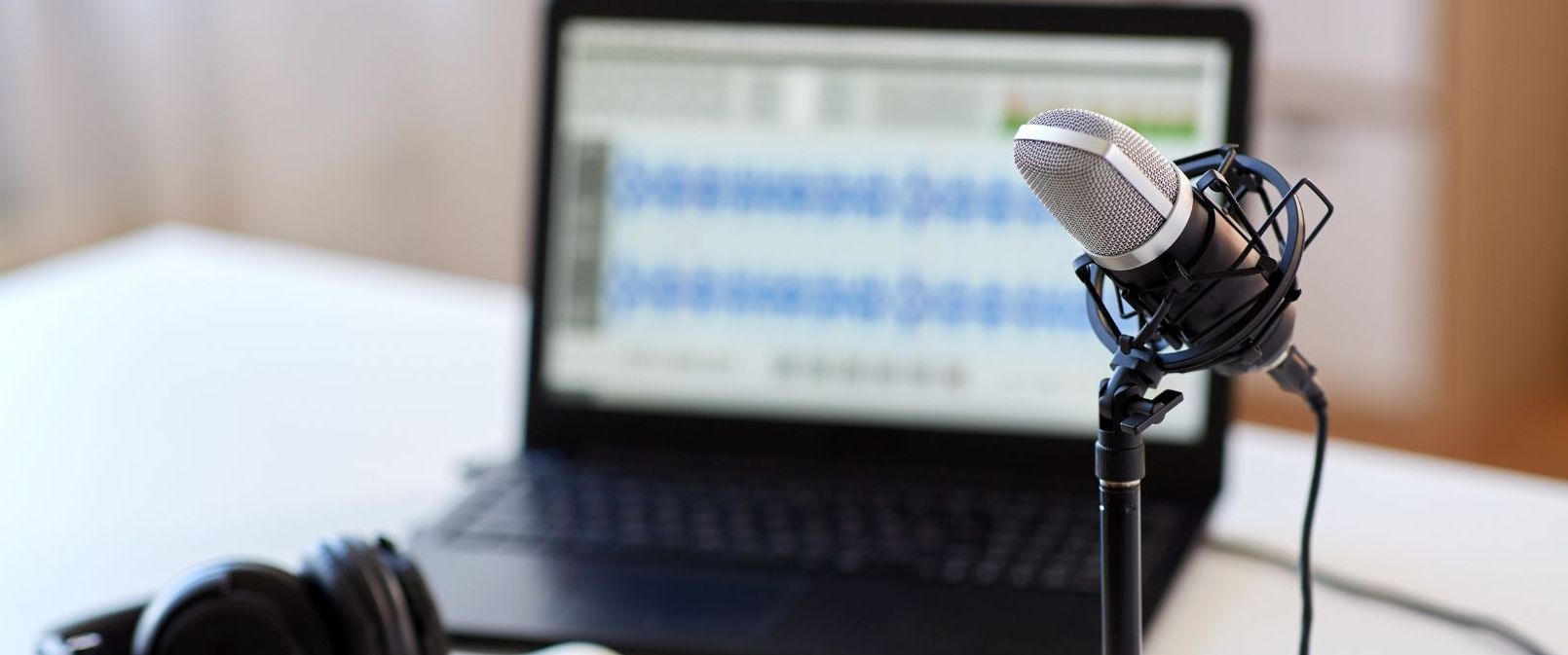
Aishell
Aishell is a Mandarin Chinese speech dataset that is widely used for research and development in the Chinese language. It offers high-quality recordings and transcriptions, making it a valuable resource for developers working on Mandarin speech recognition systems.
Aishell is a Mandarin Chinese speech dataset that has gained widespread use in both academic and commercial settings. The dataset includes high-quality recordings of Mandarin speech, along with detailed transcriptions and annotations. This makes Aishell an essential resource for developers working on Mandarin speech recognition systems.
One of the key advantages of Aishell is its focus on Mandarin Chinese, a language that is often underrepresented in global speech datasets. The dataset includes recordings from a diverse range of speakers, making it suitable for projects that require robust recognition of different accents and dialects within the Mandarin language. Additionally, Aishell is freely available for research purposes, making it accessible to a wide range of developers and researchers.
However, Aishell does have some limitations. The dataset is primarily focused on read speech, which may not fully represent the nuances of conversational Mandarin. Additionally, the dataset may require additional preprocessing to ensure consistency and accuracy in the transcriptions. Despite these limitations, Aishell remains a valuable resource for developers working on Mandarin speech recognition.
SpeechOcean
SpeechOcean offers free datasets for academic research, including multilingual speech data and labelled audio files. It’s a useful resource for projects that need diverse linguistic data but still might have some limitations in comparison to comprehensive paid options.
SpeechOcean offers a range of free datasets that are particularly valuable for academic research. The datasets include multilingual speech data, as well as labelled audio files that can be used for a variety of speech recognition and synthesis tasks. While SpeechOcean’s free offerings may not be as comprehensive as some paid options, they provide a useful starting point for projects that need diverse linguistic data.
One of the main benefits of SpeechOcean is its focus on multilingualism. The platform offers datasets in a wide range of languages, making it a valuable resource for developers working on projects that require speech data from different linguistic and cultural backgrounds. Additionally, SpeechOcean provides detailed metadata and annotations, making it easier for developers to integrate the data into their projects.
However, the free datasets available on SpeechOcean may have some limitations in terms of quality and scope. Some datasets may require additional preprocessing or cleaning, and the range of languages covered may not be as extensive as some other resources. Despite these challenges, SpeechOcean remains a valuable resource for developers and researchers who need access to multilingual speech data.
Benefits and Limitations of Free Speech Data
While free speech data resources are invaluable, they come with both advantages and drawbacks.
Benefits:
- Cost-Effective: Free resources significantly reduce the financial burden of acquiring speech data, making it accessible to a broader audience.
- Diverse Datasets: Many free resources, such as Common Voice and VoxForge, offer diverse datasets that include various accents, languages, and speech patterns.
- Open Source Community: Free speech data often benefits from community contributions, leading to continuous updates and improvements.
Limitations:
- Data Quality: The quality of free speech data can vary, especially in crowdsourced datasets where audio clarity and transcription accuracy may not be consistent.
- Limited Scope: Free datasets may not cover all languages, accents, or specific use cases required for certain projects.
- Licensing Issues: Some free resources may have restrictions on commercial use, so it’s essential to review licensing terms before incorporating them into your projects.
How to Access and Use Open Source Speech Data
Accessing open-source speech data is generally straightforward. Most datasets are available for download through their respective websites or repositories like GitHub. However, using these resources effectively requires a clear understanding of your project’s goals and requirements.
- Download the Dataset: Start by downloading the dataset that best fits your needs. Ensure you have sufficient storage and processing power, as speech datasets can be large.
- Preprocess the Data: Clean and preprocess the audio files to ensure consistency and remove any unwanted noise or artefacts.
- Label and Annotate: If the dataset lacks annotations, consider using tools to label the data accurately.
- Integrate with ML Models: Incorporate the processed data into your machine learning models for training and evaluation.
Comparing Free and Paid Speech Data Options
While free speech data resources offer significant advantages, paid options may be necessary for more specialised or high-stakes projects.
- Quality: Paid datasets typically offer higher quality audio and more accurate transcriptions, reducing the need for extensive preprocessing.
- Scope: Paid resources often cover a broader range of languages, accents, and scenarios, making them suitable for complex projects.
- Support: Paid services often include customer support and updates, ensuring that you have access to the latest data and tools.
Case Studies on Utilising Free Speech Data
Case Study 1: OpenAI’s Whisper OpenAI’s Whisper model, developed for automatic speech recognition, utilised free speech datasets like Common Voice and TED-LIUM during its development phase. By leveraging diverse, open-source datasets, Whisper was able to achieve state-of-the-art performance across various languages and accents.
Case Study 2: Google Translate’s Voice Input Google Translate initially incorporated free datasets like VoxForge to improve its voice input functionality. The use of diverse and multilingual speech data helped Google refine its algorithms for more accurate translations.
Key Captioning Tips
- Check Licensing: Always review the licensing terms of free datasets to ensure compliance with your project’s requirements.
- Diversify Your Data: Combine multiple free datasets to create a more comprehensive and varied dataset for training your models.
- Preprocess Thoroughly: Clean and preprocess the data to enhance its quality and usability in your models.
- Evaluate and Test: Regularly test your models with different datasets to ensure robustness and accuracy.
- Stay Updated: Keep an eye on updates and new releases of free speech datasets to take advantage of the latest resources.
Unlocking free speech data resources offers AI enthusiasts and professionals a cost-effective way to access the data needed to develop and refine their models. While free resources provide numerous benefits, they also come with limitations that must be carefully considered. By understanding how to access, utilise, and supplement these resources, you can create more robust and accurate AI systems without the need for expensive data purchases.
For those with specific requirements, combining free resources with paid options can strike the right balance between cost and quality. As you navigate the landscape of free speech data, remember to stay informed about the latest developments and continuously evaluate the performance of your models.
Further Free Speech Data Resources
Wikipedia: Open Data – This article discusses open data, including sources, benefits, and challenges, providing context for understanding the availability and use of free speech data.
Way With Words: Speech Collection – Way With Words offers bespoke speech collection projects tailored to specific needs, ensuring high-quality datasets that complement freely available resources. Their services fill gaps that free data might not cover, providing a comprehensive solution for advanced AI projects.