How Do AI Language Models Work?
How Do AI Models Understand and Generate Human-like Text?
The advent of AI language models marks a transformative era in the realms of data science, technology entrepreneurship, and software development. As we delve into the intricacies of AI and language use, several key questions emerge. How do these models understand and generate human-like text? What makes them valuable in various industries, from enhancing communication to refining language corpora?
Understanding the mechanism of AI language models is pivotal for those leveraging these technologies to advance their capabilities in machine learning and artificial intelligence. High-quality data is the cornerstone of these advancements, where both speech and text are meticulously analysed and utilised. This article aims to dissect the functionality of AI language models and explore the factors that determine the quality of data used, ensuring that the technology not only meets but exceeds its intended capabilities.
Important Aspects of AI Language Models
Understanding AI Language Models
AI language models are complex algorithms trained to understand, interpret, and generate human language. They learn from vast amounts of language datasets, absorbing the nuances of human communication. These models are not just programmed; they evolve through continuous learning, making them highly adaptable and sophisticated tools in AI development.
AI language models are not just technological marvels; they are a testament to the incredible advancements in the field of artificial intelligence. These models function through complex algorithms that emulate the intricacies of human language, going beyond mere word recognition to grasp context, tone, and even cultural nuances. This advanced understanding is not hardcoded but is acquired through exposure to extensive language datasets. These datasets encompass a wide range of linguistic styles and contexts, enabling the models to simulate human-like language processing abilities.
The evolution of AI language models is continuous and dynamic. Unlike traditional software, these models do not remain static post-development; they are designed to adapt and refine their linguistic capabilities over time. This adaptability is key in a world where language is constantly evolving. For instance, the emergence of new slang, terminology, and even entire dialects necessitates a system capable of learning and integrating these changes seamlessly. This constant evolution makes AI language models invaluable tools in various sectors, transforming how machines interpret and generate human language.
The Role of Machine Learning
Machine learning is the backbone of AI language models. Through iterative learning processes, these models gradually improve their language understanding. This involves algorithms analysing large datasets, identifying patterns, and making predictions, which are crucial for natural language processing (NLP).
Machine learning stands at the core of AI language models, serving as the engine that drives their linguistic capabilities. These models use sophisticated algorithms to process and analyse vast amounts of text data, learning from patterns and intricacies within human language. This process is iterative, with the models continually refining their understanding and output based on new data. Unlike traditional programming, where responses are predefined, machine learning enables AI models to develop a more nuanced understanding of language over time.
The power of machine learning in AI language models is evident in their ability to make predictions and understand context. These capabilities are integral to natural language processing (NLP), which is the foundation of these models. NLP allows machines to interpret, decipher, and even respond to human language in a way that is both coherent and contextually relevant. This evolving understanding is crucial in applications ranging from voice assistants to automated customer service, where the ability to comprehend and respond appropriately to human language is paramount.
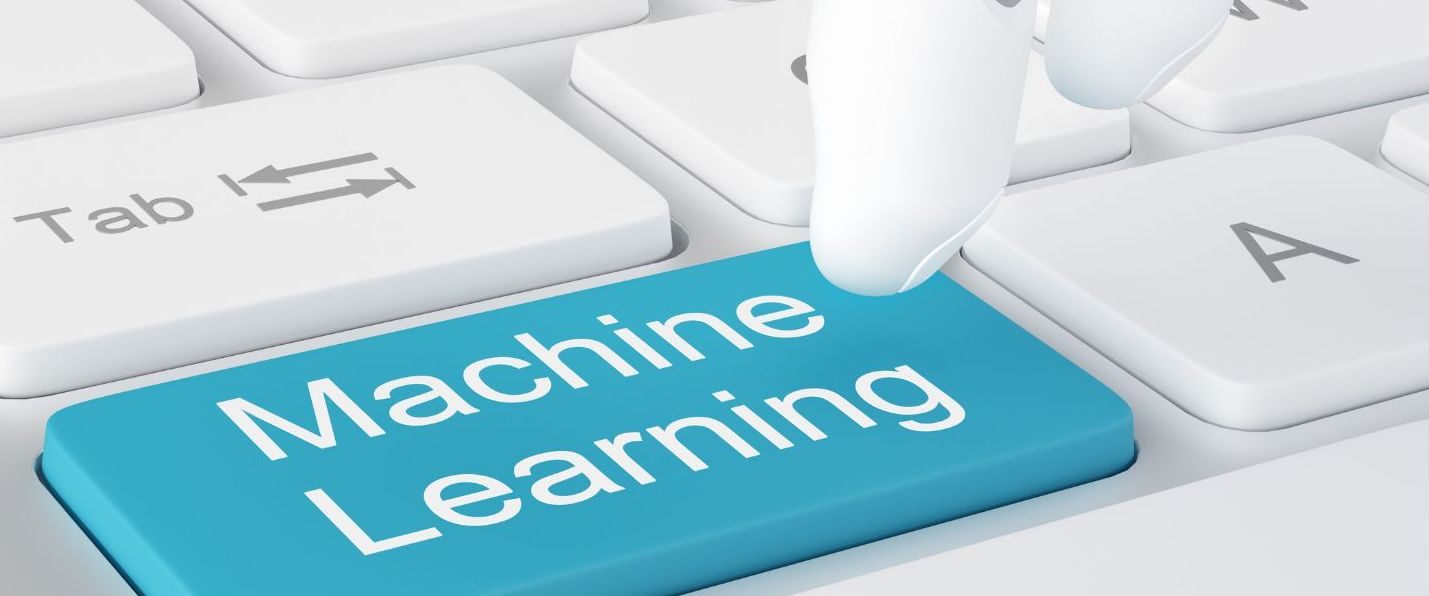
Importance of Quality Data
The calibre of data fed into AI language models determines their effectiveness. High-quality language datasets ensure the models are accurately trained, leading to more reliable and efficient outputs. This includes diverse, well-annotated, and context-rich data.
The effectiveness of AI language models hinges on the quality of data they are trained on. High-quality language datasets are essential for accurate training, ensuring that the models can understand and generate language effectively. These datasets must be diverse, covering various languages, dialects, and linguistic styles. They also need to be well-annotated, providing clear examples of language use, and context-rich to help the model understand the subtleties and variations of language.
The quality of data also impacts the reliability and efficiency of AI language models. Inaccurate or biased data can lead to models that are ineffective or even harmful, as they might propagate stereotypes or misunderstandings. Therefore, it is crucial to source data responsibly, ensuring it represents a wide range of linguistic inputs. This diversity not only improves the accuracy of the models but also ensures they are inclusive and representative of the varied ways language is used globally.
Applications in Various Industries
AI language models find applications across numerous sectors. They aid in automating customer service, enhancing speech recognition systems, refining search engine algorithms, and even in creative industries for content generation.
AI language models have found a place in virtually every industry, revolutionising how businesses interact with customers and manage data. In customer service, these models power chatbots and virtual assistants, providing instant, accurate responses to customer inquiries. This automation leads to more efficient customer service and allows human agents to focus on more complex tasks. In the creative industries, AI language models are used for content generation, assisting in writing tasks, and even generating creative content like poetry or stories.
These models also play a crucial role in enhancing speech recognition systems, essential in industries from healthcare to automotive, where hands-free interaction is increasingly important. In the field of search engine technology, AI language models refine algorithms to understand and match user queries better, delivering more relevant search results. Their application in these diverse fields demonstrates their versatility and the vast potential they hold for future innovations.
Ethical Considerations and Bias
Ethical considerations, especially bias in AI language models, are crucial. The data used for training these models must be free from biases to avoid perpetuating stereotypes or unfair representations.
Ethical considerations are paramount in the development and deployment of AI language models. One of the most significant concerns is the issue of bias. If the training data for these models includes biases, whether intentional or not, the models may perpetuate these biases in their outputs. This can lead to unfair representations and decisions, particularly in sensitive areas like hiring, law enforcement, and credit scoring. Ensuring that training data is free from biases and represents a diverse range of perspectives is crucial to developing fair and unbiased AI systems.
Additionally, there’s the concern of the models being used in ways that could compromise privacy or propagate misinformation. The ability of AI language models to generate convincing human-like text has implications for the spread of fake news or impersonation. Therefore, it’s important to establish ethical guidelines and safeguards to prevent misuse of these technologies. This includes transparency in how the models are trained and used, as well as ongoing monitoring to ensure they are functioning as intended and not being abused.
The Evolution of NLP
Natural Language Processing (NLP) has evolved significantly with AI language models. They’ve enhanced machine understanding of human language, making interactions more seamless and intuitive.
The evolution of Natural Language Processing (NLP) through AI language models has been nothing short of revolutionary. NLP, once limited to basic text interpretation and response generation, now encompasses a broad spectrum of language understanding and generation capabilities. These advancements have been driven largely by the development of more sophisticated AI language models, which can understand context, sentiment, and even the subtleties of different languages and dialects.
The impact of these advancements in NLP is wide-ranging. In the field of linguistics, it has enabled a deeper understanding of language structures and usage. In technology, it has led to the development of more intuitive and human-like interactions with machines. The advancements in NLP have also had significant implications for accessibility, with improved voice recognition and text-to-speech technologies helping to break down barriers for people with disabilities. As NLP continues to evolve, it promises to further bridge the gap between human and machine communication, making technology more accessible and efficient.
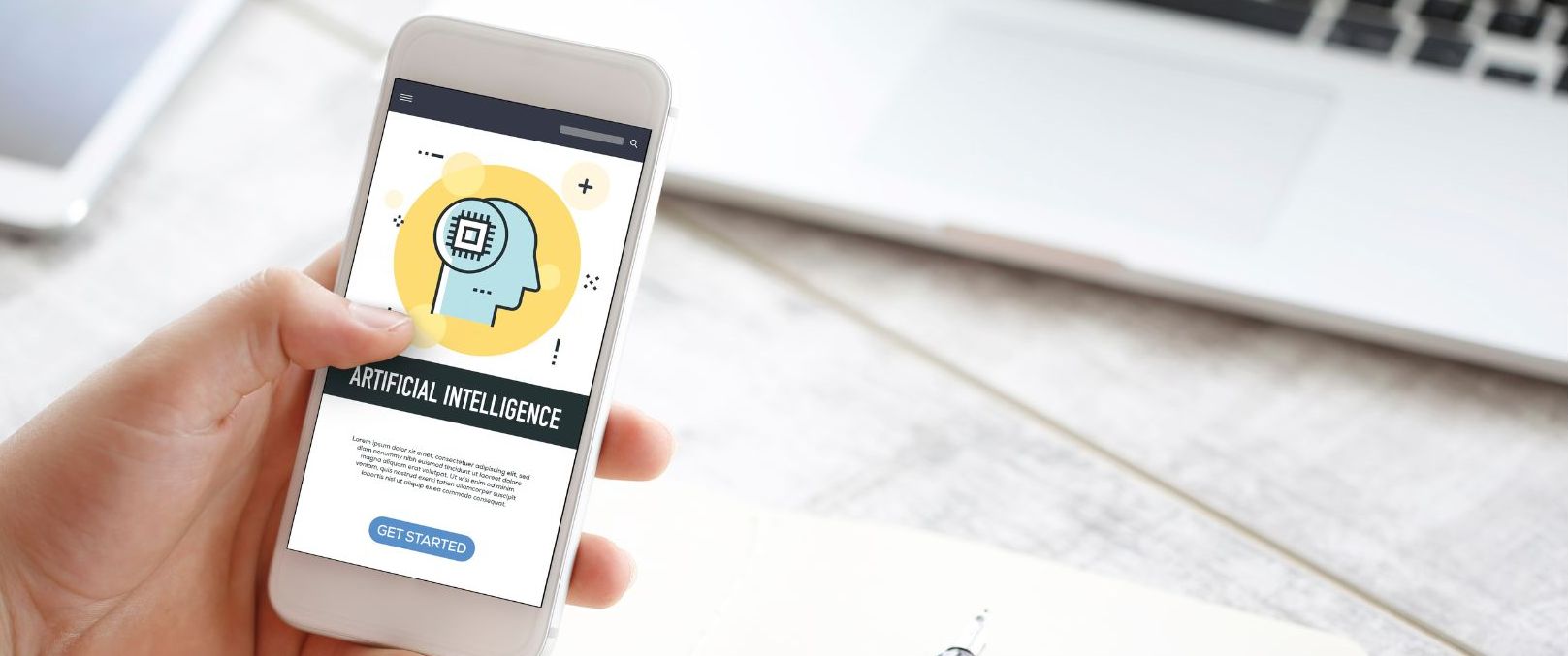
Challenges in Language Model Training
Training AI language models is not without challenges. It requires massive computational resources, and there’s always the risk of overfitting or under-fitting the model to the training data.
Training AI language models is a complex and resource-intensive process, presenting several challenges. One of the primary challenges is the sheer amount of computational power required. These models often need to process and learn from enormous datasets, which can be computationally expensive and time-consuming. This requirement can limit the accessibility of AI language model development to organisations with significant resources.
Another challenge is the risk of overfitting or underfitting the model to the training data. Overfitting occurs when a model is too closely tailored to the training data, making it less effective at generalising to new, unseen data. Underfitting, on the other hand, happens when the model is not complex enough to capture the intricacies of the data, leading to poor performance. Balancing these two extremes is critical for creating effective AI language models. Additionally, ensuring that the models are trained on diverse and representative datasets is essential to avoid biases and ensure the models perform well across different languages and contexts.
Future of AI Language Models
The future of AI language models is promising, with continuous advancements leading to more sophisticated, accurate, and versatile applications.
The future of AI language models is incredibly promising, with continuous advancements leading to more sophisticated and versatile applications. These models are expected to become even more accurate and capable of understanding and generating language in a way that is indistinguishable from human interaction. This will have far-reaching implications for various fields, from enhancing human-computer interaction to revolutionising how we process and analyse large volumes of text data.
One exciting prospect is the development of models that can understand and translate between multiple languages seamlessly, breaking down language barriers and facilitating global communication. There’s also the potential for these models to be used in education, providing personalised learning experiences and language tutoring. As these technologies continue to evolve, they will likely become more integrated into our daily lives, making interactions with technology more natural and intuitive.
Importance of Data Privacy
In collecting language datasets, data privacy is paramount. Ensuring that the data used respects individual privacy rights is crucial for ethical AI development.
Data privacy is a critical concern in the collection and use of language datasets for AI models. With the increasing amount of personal and sensitive information being processed by these models, ensuring that this data is used responsibly and securely is paramount. This includes adhering to data protection regulations, such as the General Data Protection Regulation (GDPR) in Europe, and ensuring that individuals’ privacy rights are respected.
There’s also the need to balance the collection of diverse and comprehensive datasets with the protection of individual privacy. This can involve anonymising data, obtaining consent from data subjects, and implementing strict data governance policies. Ensuring data privacy not only protects individuals but also helps to build public trust in AI technologies, which is essential for their continued development and adoption.
Enhancing User Experience
Ultimately, AI language models aim to enhance user experience. Whether in customer service, content creation, or speech recognition, the goal is to make technology more accessible and efficient for users.
The ultimate goal of AI language models is to enhance user experience, making interactions with technology more efficient, intuitive, and accessible. In customer service, for instance, AI-powered chatbots can provide instant, accurate responses to customer inquiries, improving response times and customer satisfaction. In content creation, these models can assist in generating creative and engaging content, reducing the workload on human writers.
In speech recognition, AI language models have led to significant improvements in accuracy and speed, making voice-activated technologies more reliable and user-friendly. This is particularly important in fields like healthcare, where hands-free technology can greatly enhance efficiency and safety. As AI language models continue to improve, they will further enhance the usability of technology, making it more accessible to a wider range of users and applications.
Language Dataset Creation – Key Tips
- Ensure the use of high-quality, diverse, and well-annotated language datasets for training AI language models.
- Regularly update AI models to adapt to changing language use and trends.
- Address ethical considerations, particularly biases, in AI language model development.
- Recognise the importance of data privacy in the collection and use of language datasets.
- Way With Words provides highly customised data collections for speech and other use cases, enhancing AI language and speech development technologies.
In this exploration of AI language models, we’ve uncovered their foundational role in modern technology. From the intricacies of their learning mechanisms to the importance of high-quality data, these models are reshaping how we interact with technology. The key piece of advice for those venturing into this field is to prioritise the quality and ethical sourcing of language datasets. It’s this attention to detail that will drive the advancement of AI language models, ensuring they not only meet but exceed the dynamic needs of various industries.
Some AI Language Resources
Way with Words create speech datasets including transcripts for machine learning purposes. Their service is used for technologies looking to create or improve existing automatic speech recognition models (ASR) using natural language processing (NLP) for select languages and various domains.
Way with Words polish machine transcripts for clients across a number of different technologies. Their machine transcription polishing (MTP) service is used for a variety of AI and machine learning purposes. User applications include machine learning models that use speech-to-text for artificial intelligence research, FinTech/InsurTech, SaaS/Cloud Services, Call Centre Software and Voice Analytic services for the customer journey.
TechTarget explain what are Large Language Models (LLMs)?