Human Oversight in Automated Captioning: Ensuring Accuracy
What is the Role of Human Oversight in Automated Captioning?
Automated captioning technologies have brought transformative change to the way content is produced, disseminated, and consumed in the spheres of media, education, business, and beyond. This is especially true of short-form captioning services. These systems use AI-driven speech recognition to produce captions at high speed and scale, reducing the time and resources once needed for manual transcription. However, despite their increasing sophistication, these systems are far from flawless. Automated captioning often fails to capture context, emotional nuance, speaker intent, and specialised vocabulary—shortcomings that can significantly impact the quality and usefulness of captions.
Human oversight acts as a critical quality assurance layer in the captioning process. It involves professional caption editors reviewing, correcting, and enhancing machine-generated captions to ensure they are coherent, accurate, and contextually relevant. This practice is known as human-in-the-loop captioning and is widely regarded as essential to maintain standards of accessibility and user experience.
Integrating human oversight captions into workflows is not simply about fixing errors; it’s about preserving the integrity of the original content. Many organisations now face important questions as they consider using automated captioning in their workflows:
- How accurate is automated captioning without human involvement?
- What kinds of errors are most common in AI-generated captions?
- Is it cost-effective to include human oversight in every captioning project?
This short guide explores the role of human oversight in improving automated captioning quality and ensuring caption accuracy. By examining common pitfalls, real-world examples, tools, and best practices, we aim to provide a comprehensive look at how and why human intervention remains essential in the automated captioning process.
10 Key Topics on Human Oversight of Captioning
1. The Role of Human Oversight in Captions
Human oversight captions help align machine outputs with human communication standards. Automatic captions often miss nuances such as sarcasm, humour, or emotional tone. They may also produce mishearing’s or omit critical non-verbal sounds that are essential for comprehension, such as [laughter] or [door slams].
A 2023 study by the University of Edinburgh found that automated captioning systems reached an average of 85% accuracy for standard British English speech. However, for speakers with regional accents, overlapping dialogue, or domain-specific terminology, accuracy could fall to as low as 68%–72%. By introducing human oversight, these systems saw accuracy rates climb as high as 98.5%.
Human reviewers provide:
- Contextual corrections for words with multiple meanings
- Consistency in terminology across related videos
- Accessibility checks, such as flagging unintelligible segments
- Improved speaker identification for multi-speaker recordings
- Correction of homophones and malapropisms
Without human involvement, such nuances would go uncorrected and might lead to misunderstandings, miscommunication, or alienation of key audience groups.
2. Automated Captioning Quality: Where AI Falls Short
Speech-to-text algorithms have grown exponentially in recent years, yet there remain persistent shortcomings in AI-generated captions. Key quality gaps include:
- Homophone confusion: For example, “there,” “their,” and “they’re” are often interchanged
- Speaker mislabelling: Especially in interviews and panel discussions
- Poor punctuation: Run-on sentences and lack of pauses
- Inability to identify cross-talk or overlapping dialogue
- Cultural and linguistic nuances lost in translation
In practice, these errors can disrupt a user’s engagement and lead to false impressions about the speaker or topic. For content aimed at international, academic, or legal audiences, such inaccuracies are not just inconvenient—they can be harmful.
3. Caption Accuracy: Why it Matters
Caption accuracy isn’t just a technical metric—it’s a vital component of communication, inclusion, and trust. For example:
- Accessibility: Accurate captions are essential for users who are deaf or hard of hearing. Inaccurate captions may prevent them from participating equally.
- Education: In learning environments, even a single error in terminology can alter a learner’s understanding of a key concept.
- Legal compliance: In jurisdictions like the UK, Canada, and the EU, poor captioning can result in non-compliance with accessibility legislation such as the Equality Act 2010 or the EU Web Accessibility Directive.
Studies have shown that videos with high caption accuracy are 35% more likely to retain viewers for longer durations. Moreover, captioned content sees higher user satisfaction, with viewers 42% more likely to recommend such content to others.
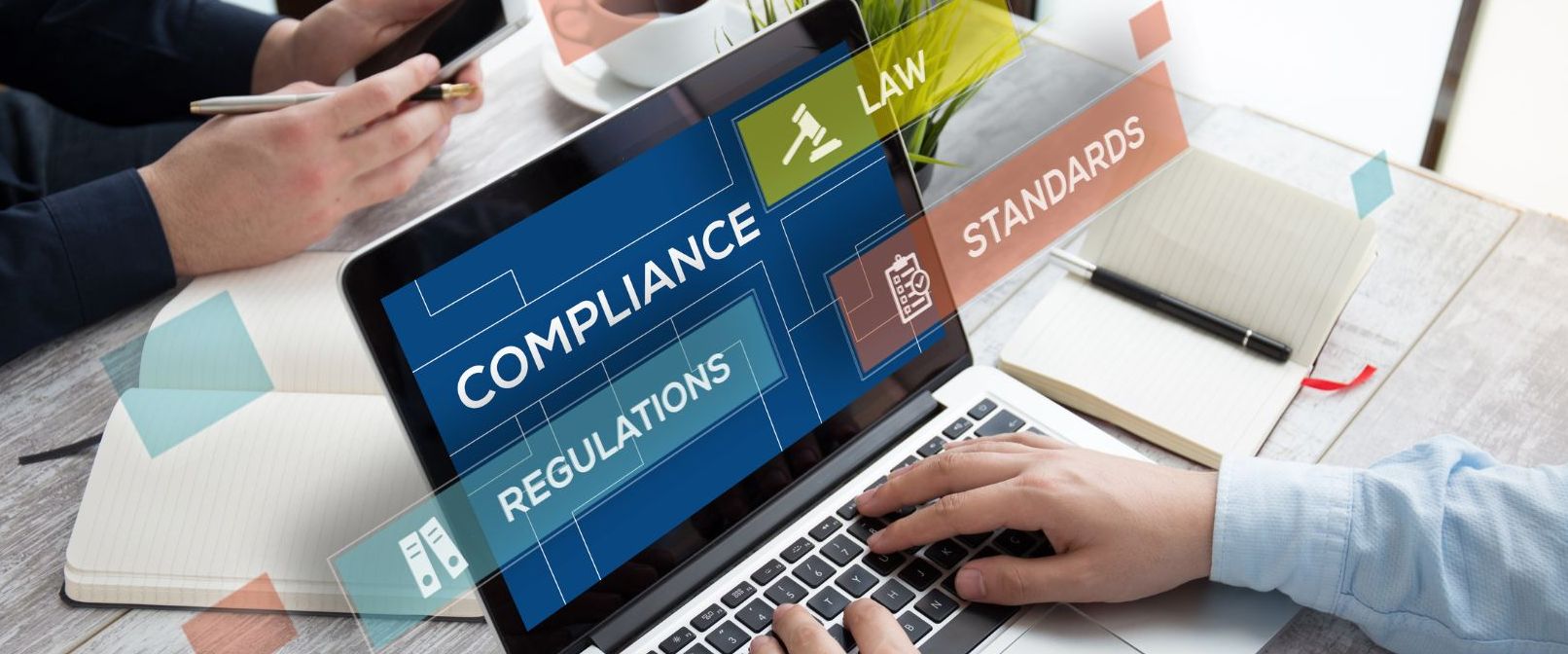
4. Tools Supporting Human Oversight in Captioning
Today’s market offers a wide range of captioning platforms that blend AI and human oversight. Popular tools include:
- Trint: Offers a web-based platform with editing tools that let users refine machine-generated captions, view timestamps, and align text to audio waveforms.
- Descript: Provides real-time transcription editing with the ability to correct text like a word processor, supporting collaborative editing.
- Way With Words: Combines automated technology with professional human editors to deliver premium captioning accuracy tailored for media, education, and corporate content.
These tools reduce the time and cost of captioning while allowing content producers to maintain editorial standards and legal compliance.
5. Case Study: Educational Content with Human Review
A leading UK-based digital university introduced AI-based captioning across all pre-recorded lecture content. Initial deployment saw an accuracy rate of 76%, with frequent issues including misinterpretation of technical terms in science and engineering, difficulties handling speakers with strong regional accents, and poor formatting of mathematical expressions.
After complaints from students with hearing impairments and those studying in English as a second language, the institution invested in a human review process. This included:
- Assigning a dedicated caption editor per subject area
- Verifying vocabulary with academic staff
- Creating course-specific glossaries for consistency
The results were dramatic. Student satisfaction scores related to content clarity increased by 30%, while the volume of support requests linked to misunderstood lectures fell by 45%. The revised accuracy of captions improved to over 99%.
6. Case Study: Media Production and Corporate Training
A media production company creating compliance training videos for a global pharmaceutical brand faced significant challenges when using automated captions. Technical jargon, names of drugs, and regulatory terms were routinely miscaptioned, and the captions failed to capture tone accurately in interview-style videos.
The company switched to a hybrid model that included human editors with backgrounds in life sciences. They implemented QA checks at each production stage and introduced a captioning style guide for consistency.
This adjustment resulted in:
- A 40% reduction in client complaints
- A 50% improvement in engagement analytics
- Recognition from internal stakeholders for compliance readiness
7. Common Errors Human Reviewers Catch
Human editors often identify mistakes that automated systems overlook:
- Speaker misattribution, especially in rapid exchanges
- Incorrect transcription of accents or colloquialisms
- Inappropriate censorship by AI, such as flagging neutral words
- Failure to denote on-screen actions or environmental cues
- Omitted filler words that can be important in some contexts (e.g., legal or journalistic content)
In multilingual projects, human oversight is vital for cultural adaptation and localisation, ensuring tone and phrasing align with regional norms.
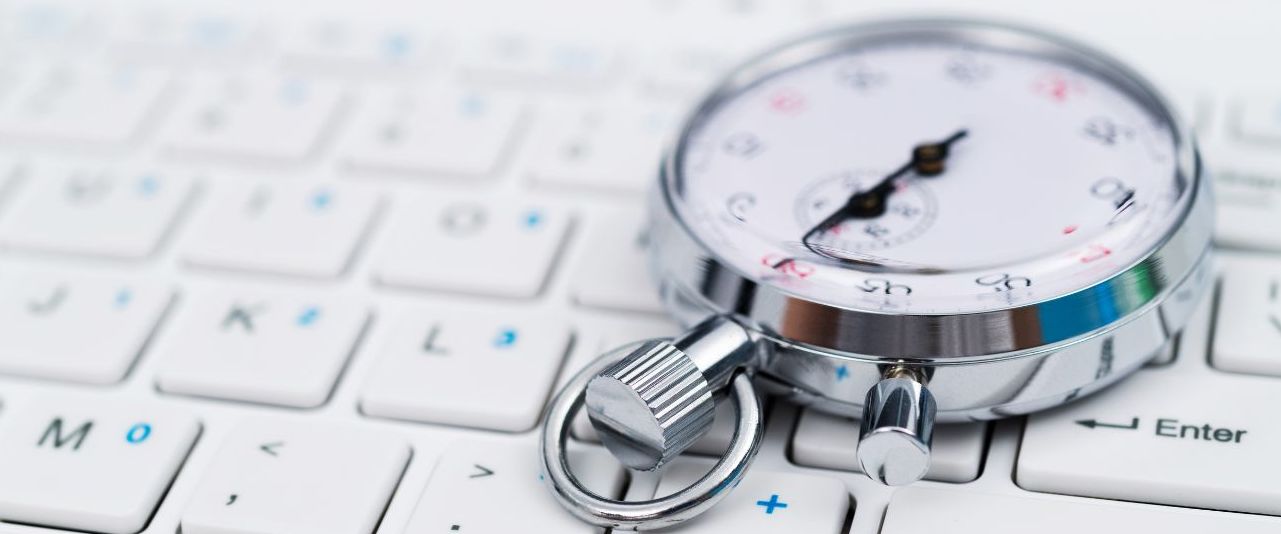
8. Balancing Speed with Quality in Captioning Workflows
Automated captioning can transcribe a 30-minute video in minutes, but without human review, the result may be unusable. The most effective captioning workflows adopt a staged approach:
- Stage 1: Generate a rough caption transcript using AI
- Stage 2: Pass the transcript through an editorial review process
- Stage 3: Verify accuracy with subject matter experts (where applicable)
- Stage 4: Conduct usability testing with sample viewers
This approach balances volume with quality, allowing for scalable yet accurate captioning solutions. Many organisations schedule post-editing into their content delivery timelines as standard.
9. Cost-Effectiveness of Human Oversight
While the cost of manual captioning may initially seem higher, the benefits are tangible and often measurable. Consider the following ROI metrics:
- Lower legal risk: Avoid fines or non-compliance penalties
- Increased content usability: Leads to longer viewer retention and better learning outcomes
- Brand trust and reputation: Clear, accurate captions reflect a commitment to quality and accessibility
- Operational efficiency: Reduced need for support and follow-up clarification
According to a 2022 survey by Captioning Market Insights, 62% of companies using human oversight in captioning reported better overall engagement metrics and a reduction in customer support costs.
10. Best Practices for Combining AI and Human Expertise
For organisations seeking to build a sustainable, high-quality captioning workflow, consider these best practices:
- Use automation for first drafts to save time
- Assign human reviewers with domain-specific knowledge
- Establish editorial guidelines and style references
- Implement feedback loops from viewers and stakeholders
- Regularly retrain AI models based on common errors observed
Combining automation and human intervention allows teams to scale up production while maintaining the fidelity and integrity of their captions.
Key Human Oversight of Captioning Tips
- Use layered captioning workflows: Combine AI speed with human precision.
- Train editors in domain-specific language: Avoid miscaptioning industry-specific terms.
- Create a captioning style guide: Maintain consistency and quality across videos.
- Audit samples regularly: Ensure your process remains effective over time.
- Involve viewers in feedback: Let users report issues and suggest improvements.
Automated captioning offers incredible potential for reaching broad audiences quickly. However, caption accuracy remains a serious concern, particularly in sectors where miscommunication can lead to legal or educational consequences. Human oversight captions are not a luxury—they are a necessity for ensuring that content is inclusive, reliable, and valuable.
This short guide explored ten crucial areas where human involvement transforms captioning from merely functional to truly effective. From educational institutions and global enterprises to media houses and tech developers, the lesson is clear: quality requires a human touch.
By strategically integrating human oversight into automated workflows, organisations can significantly enhance the reliability of their captioning, increase user satisfaction, and uphold accessibility standards. The cost of not doing so—missed opportunities, legal exposure, alienated audiences—is too great.
As technology continues to evolve, the best approach is one that respects both the capabilities and the limitations of automation. Pairing AI with human insight is the surest way to ensure your captions speak as clearly as your content.
Further Captioning Resources
Human-in-the-loop – This Wikipedia article explains the concept of human-in-the-loop systems, which are crucial for maintaining accuracy and quality in automated captioning.
Featured Captioning Solution: Way With Words Captioning Services – Ensure the highest accuracy with our combined automated and human-reviewed captioning services. We provide precise and reliable captions for all your content needs.