Why Speech Data is Crucial for Machine Learning Success
Why is Speech Data Important for Machine Learning?
Speech data has become an essential component in the success of machine learning (ML) models, particularly in the realm of natural language processing (NLP) and automated speech recognition (ASR). The importance of speech data cannot be overstated, as it forms the foundation upon which advanced AI systems are built. This short guide delves into the various facets of speech data’s role in ML, aiming to answer the following common questions:
- Why is speech data crucial for machine learning?
- How does speech data enhance AI accuracy?
- What are the real-world applications of speech data in machine learning?
Understanding the importance of speech data and its applications in machine learning is vital for AI developers, machine learning engineers, data analysts, tech companies, and academic researchers. This guide will provide an in-depth analysis of the role of speech data in ML, methods for collecting high-quality data, and future trends in this field.
Importance of Speech Data and AI Training
Role of Speech Data in Machine Learning
Speech data plays a critical role in the development and success of machine learning models. It serves as the primary input for training algorithms designed to recognise, interpret, and generate human speech. The importance of speech data in machine learning is multifaceted:
- Training Data for NLP and ASR: Speech data is used to train models that perform NLP and ASR tasks. High-quality, diverse speech datasets help in building models that can understand various accents, dialects, and languages, thereby improving their performance in real-world applications.
- Improving Model Accuracy: The quality and quantity of speech data directly impact the accuracy of ML models. By providing comprehensive datasets, models can better understand and predict speech patterns, leading to more accurate outputs.
- Real-world Applications: Speech data is crucial for a wide range of applications, including virtual assistants, customer service bots, transcription services, and language translation tools. Each of these applications relies on robust ML models trained on extensive speech datasets.
Speech data is foundational for developing and refining machine learning models, particularly those focused on natural language processing (NLP) and automatic speech recognition (ASR). This data forms the core of training algorithms, enabling them to recognise, interpret, and generate human speech. Its role is multifaceted, encompassing training data for various tasks, enhancing model accuracy, and supporting a wide range of real-world applications.
Firstly, training data for NLP and ASR is critical. NLP models, which enable machines to understand and respond to human language, and ASR systems, which convert spoken language into text, rely heavily on high-quality speech datasets. These datasets must be diverse, including various accents, dialects, and languages, to ensure the models can perform well in different linguistic environments. For instance, a virtual assistant like Amazon’s Alexa must understand English spoken by users from different parts of the world, each with unique pronunciation and intonation. By training on such diverse data, models become more robust and adaptable, improving their performance in real-world applications.
Moreover, improving model accuracy hinges on the quality and quantity of speech data. Comprehensive datasets enable models to learn intricate speech patterns, nuances, and variations. This learning process is crucial for predicting and interpreting speech accurately. For example, in customer service bots, accurate speech recognition can lead to better understanding of customer queries and more precise responses. The better the data, the more refined the model’s predictions, leading to higher accuracy and reliability in various applications.
Real-world applications of speech data are vast and varied, reflecting its critical role in modern technology. Virtual assistants, such as Siri and Google Assistant, use speech data to interact with users seamlessly, understanding commands and providing relevant information.
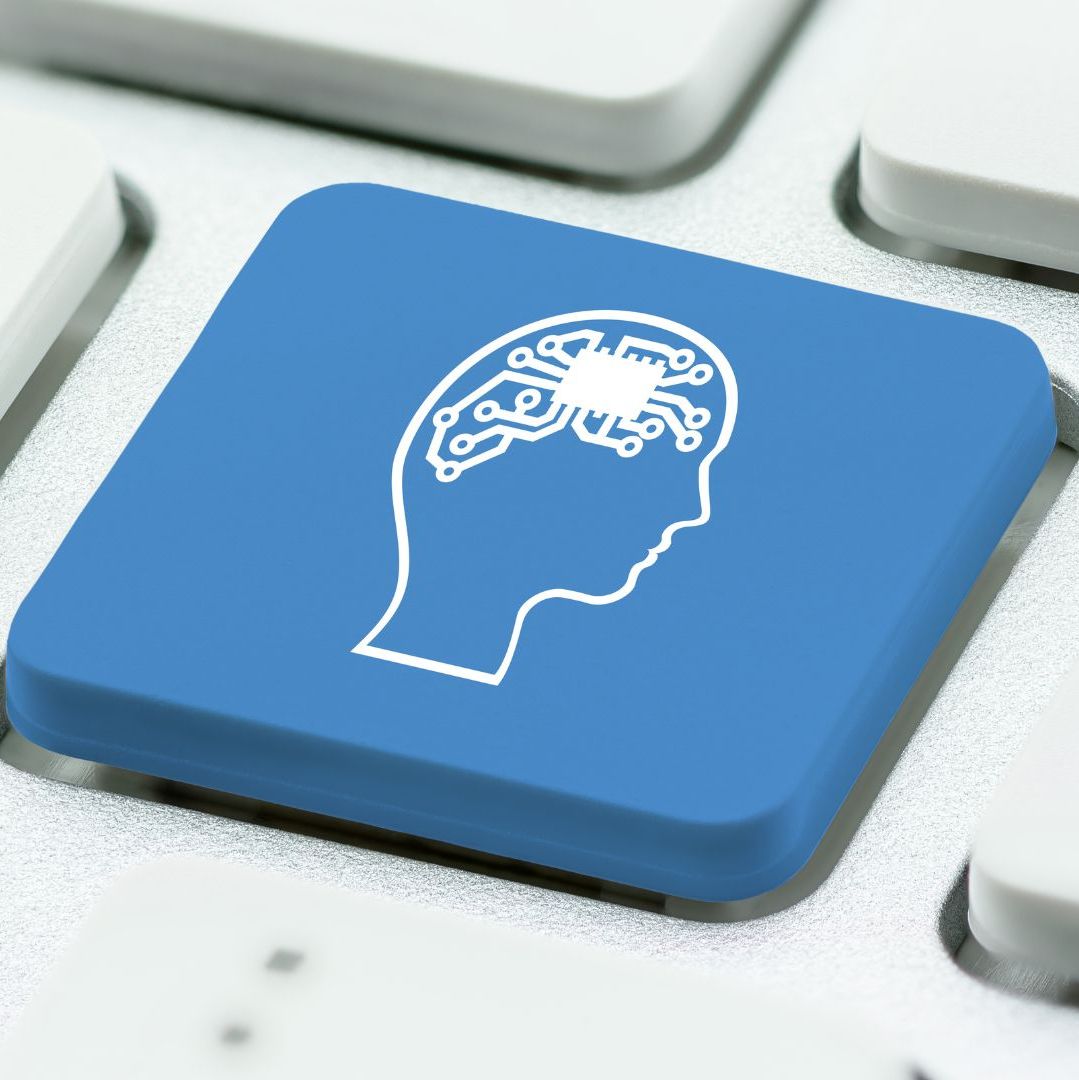
Customer service bots, another prime example, rely on speech data to handle customer inquiries efficiently, reducing wait times and improving user satisfaction. In the medical field, transcription services convert spoken medical notes into written records, ensuring accuracy and saving time for healthcare professionals.
Language translation tools, such as Google Translate, leverage speech data to break down language barriers, providing real-time translation and facilitating global communication. Accessibility tools for the visually impaired, like screen readers, use speech data to read out text, enhancing independence and quality of life.
Enhancing AI Accuracy with Speech Data
Enhancing AI accuracy with speech data involves several strategies that ensure the models are well-trained and capable of handling various speech-related tasks. Key strategies include:
- Diverse Data Collection: Gathering speech data from diverse demographics ensures that the models can understand different accents, speech patterns, and languages. This diversity helps in making the models more inclusive and accurate.
- Data Annotation: Properly annotated data is essential for training ML models. Annotators label the speech data with relevant information, such as speaker identity, language, and emotion, which helps the model learn more effectively.
- Data Augmentation: Techniques like noise addition, speed variation, and pitch alteration are used to create more robust models. Data augmentation increases the variability in the training data, which helps the model generalise better to unseen data.
- Quality Control: Ensuring the quality of speech data is crucial. This involves regular auditing of datasets, removing errors, and ensuring consistency in the annotations.
- Large-scale Datasets: Access to large-scale speech datasets is fundamental for training advanced ML models. These datasets provide the breadth and depth needed for the models to learn and adapt to various speech scenarios.
Enhancing AI accuracy with speech data involves implementing several key strategies that ensure models are well-trained and capable of handling various speech-related tasks. These strategies include diverse data collection, data annotation, data augmentation, quality control, and access to large-scale datasets.
Diverse data collection is paramount for creating inclusive and accurate models. By gathering speech data from a wide range of demographics, including different age groups, genders, and ethnicities, models can better understand and interpret various accents and speech patterns. This diversity is particularly important in global applications where models must perform well across different linguistic and cultural contexts. For instance, an AI system designed for global customer service must be trained on speech data that includes voices from different regions to ensure it can handle queries from any customer accurately.
Data annotation plays a crucial role in training ML models. Annotators label speech data with relevant information, such as speaker identity, language, and emotion, providing the context necessary for the model to learn effectively. This process involves tagging audio files with specific labels that help the model differentiate between different types of speech. For example, in a dataset intended for emotion recognition, annotators might label segments of speech with emotions like happiness, sadness, or anger. These labels allow the model to learn the subtle cues associated with each emotion, enhancing its ability to recognise and respond to emotional speech accurately.
Data augmentation techniques, such as noise addition, speed variation, and pitch alteration, help create more robust models. These techniques introduce variability into the training data, enabling the model to generalise better to unseen data. For instance, adding background noise to speech data can help the model learn to filter out irrelevant sounds, improving its performance in noisy environments. Similarly, varying the speed and pitch of speech data ensures the model can handle different speaking rates and intonations, making it more adaptable to real-world conditions.
Quality control is essential for maintaining the integrity of speech data. This involves regular auditing of datasets, removing errors, and ensuring consistency in annotations. High-quality data is critical for training effective ML models, as inaccuracies in the data can lead to poor model performance. Implementing stringent quality control measures helps ensure that the data used for training is accurate and reliable, leading to better model outcomes.
Large-scale datasets are fundamental for training advanced ML models. These datasets provide the breadth and depth needed for the models to learn and adapt to various speech scenarios. Access to extensive speech data allows models to develop a comprehensive understanding of human speech, improving their ability to recognise and interpret different speech patterns accurately. For example, large-scale datasets that include thousands of hours of recorded speech can help train models to understand and respond to a wide range of queries, enhancing their overall performance and accuracy.
Real-world Applications of Speech Data in ML
Speech data is integral to numerous real-world applications, driving innovation and efficiency across various industries. Some prominent applications include:
- Virtual Assistants: Devices like Amazon’s Alexa, Google Assistant, and Apple’s Siri rely heavily on speech data to function effectively. These assistants use ML models trained on vast amounts of speech data to understand and respond to user commands.
- Customer Service Bots: Automated customer service systems use speech recognition to interact with customers, providing quick and efficient responses to queries. These systems rely on high-quality speech data to interpret and respond accurately.
- Transcription Services: Speech-to-text transcription services convert spoken language into written text. These services are invaluable in legal, medical, and business settings, where accurate documentation is essential.
- Language Translation Tools: Tools like Google Translate use speech data to offer real-time translation services. These tools help bridge communication gaps by translating spoken language into other languages accurately.
- Accessibility Tools: Speech data aids in developing tools for the visually impaired, such as screen readers and voice-operated devices, enhancing accessibility and independence.
Speech data drives innovation and efficiency across various industries, demonstrating its integral role in real-world applications. These applications include virtual assistants, customer service bots, transcription services, language translation tools, and accessibility tools.
Virtual assistants like Amazon’s Alexa, Google Assistant, and Apple’s Siri are prime examples of speech data applications. These devices rely heavily on speech data to function effectively, using ML models trained on vast amounts of speech data to understand and respond to user commands. For instance, when a user asks Alexa for the weather forecast, the device processes the speech input, interprets the command, and retrieves the relevant information to provide a response. This seamless interaction is made possible by the extensive training on diverse speech data, which enables the virtual assistant to understand different accents, speech patterns, and languages.
Customer service bots leverage speech recognition to interact with customers, providing quick and efficient responses to queries. These systems rely on high-quality speech data to interpret and respond accurately, improving customer satisfaction and reducing wait times. For example, a customer service bot in a bank might be trained on speech data to handle inquiries about account balances, recent transactions, and loan applications. By understanding and processing spoken requests accurately, these bots can provide timely and relevant information, enhancing the overall customer experience.
Transcription services convert spoken language into written text, playing a crucial role in various fields such as legal, medical, and business settings. Accurate transcription is essential for documentation, record-keeping, and accessibility. In the medical field, for example, doctors often use speech-to-text transcription services to dictate patient notes, which are then converted into written records for inclusion in patient files. This process saves time and ensures that important medical information is accurately documented. Similarly, in legal settings, court proceedings and depositions are often transcribed to create official records, requiring precise and reliable speech-to-text conversion.
Language translation tools like Google Translate use speech data to offer real-time translation services. These tools help bridge communication gaps by translating spoken language into other languages accurately, facilitating global communication. For instance, a traveller in a foreign country can use a language translation tool to communicate with locals by speaking into the device, which then translates the speech into the desired language. This real-time translation capability is made possible by extensive training on multilingual speech datasets, enabling the tool to accurately interpret and translate various languages.
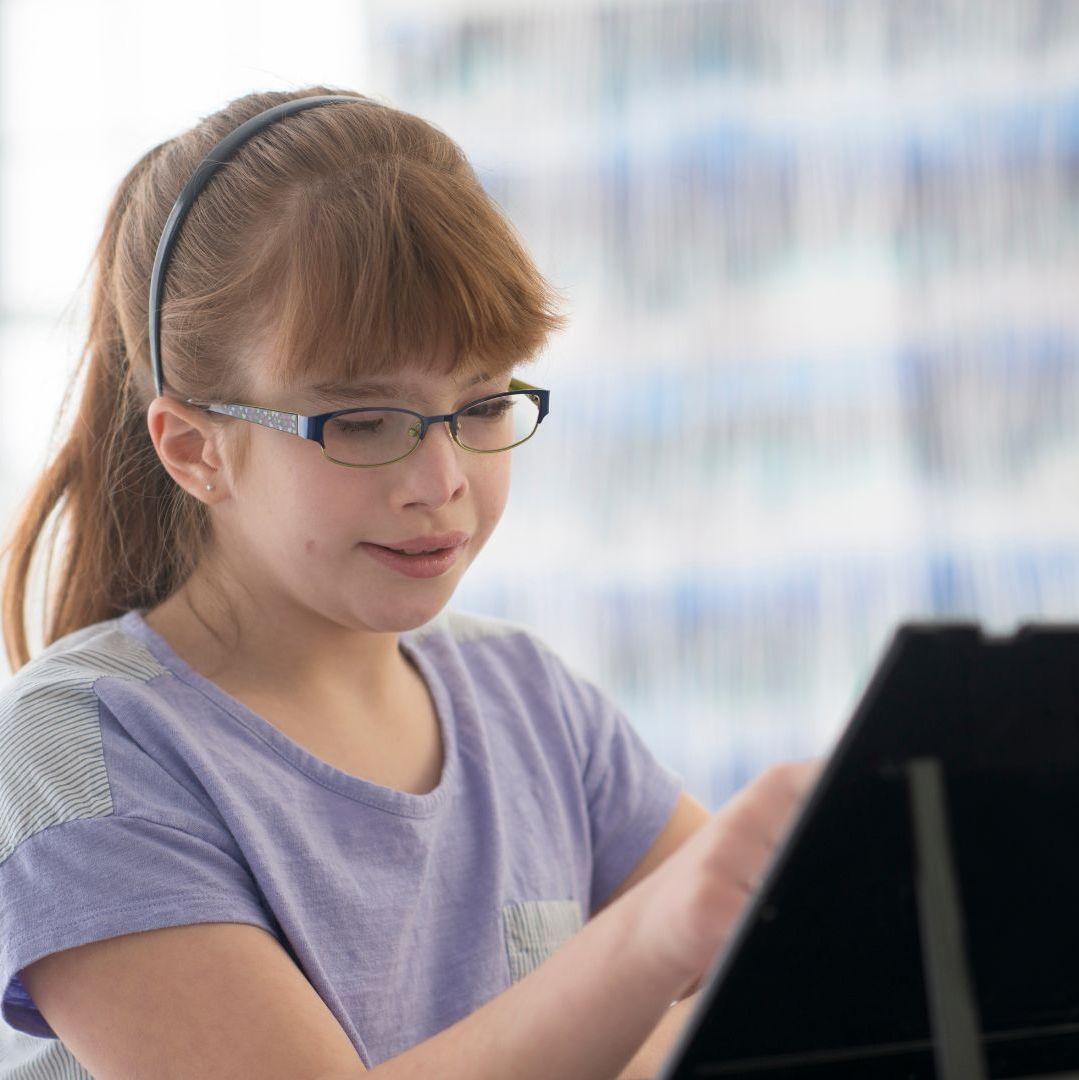
Accessibility tools for the visually impaired, such as screen readers and voice-operated devices, rely on speech data to enhance accessibility and independence. These tools convert written text into spoken language, allowing visually impaired individuals to access information and interact with technology more easily.
For example, a screen reader might read out the text on a webpage, enabling a visually impaired user to navigate the internet and access information. Similarly, voice-operated devices allow users to perform tasks like sending messages, making phone calls, and setting reminders using speech commands, enhancing their ability to interact with technology.
Data Collection Methods for Machine Learning
Collecting high-quality speech data is critical for developing successful ML models. Various methods are employed to gather this data, each with its own advantages and challenges:
- Crowdsourcing: Platforms like Amazon Mechanical Turk allow researchers to collect speech data from a diverse pool of contributors. This method is cost-effective and provides access to a wide range of speech samples.
- Synthetic Data Generation: Techniques like text-to-speech (TTS) are used to generate synthetic speech data. While this method can quickly produce large datasets, ensuring the realism and variability of the synthetic data is crucial.
- Field Recording: Recording speech data in natural environments helps capture realistic background noises and variations in speech. This method is valuable for training models that need to perform well in real-world conditions.
- Partnerships with Organisations: Collaborating with organisations that have access to speech data, such as call centres or media companies, can provide rich datasets. These partnerships often come with data privacy and security considerations.
- Publicly Available Datasets: Utilising open-source speech datasets, like the LibriSpeech or Common Voice datasets, provides a valuable resource for training ML models. These datasets are often well-annotated and cover a wide range of speech patterns.
Collecting high-quality speech data is critical for developing successful ML models. Various methods are employed to gather this data, each with its own advantages and challenges. These methods include crowdsourcing, synthetic data generation, field recording, partnerships with organisations, and utilising publicly available datasets.
Crowdsourcing is a popular method for collecting speech data, leveraging platforms like Amazon Mechanical Turk to gather data from a diverse pool of contributors. This method is cost-effective and provides access to a wide range of speech samples, ensuring diversity in the training data. For example, a researcher might use crowdsourcing to collect speech samples from people of different ages, genders, and ethnicities, ensuring that the resulting dataset is representative of the broader population. This diversity is crucial for training models that need to perform well across various demographics and linguistic backgrounds.
Synthetic data generation involves using techniques like text-to-speech (TTS) to create synthetic speech data. While this method can quickly produce large datasets, ensuring the realism and variability of the synthetic data is crucial. TTS systems generate speech from written text, allowing researchers to create datasets with specific characteristics and control over various parameters. However, synthetic data must be carefully evaluated to ensure it accurately reflects natural speech patterns and nuances. For instance, synthetic speech might lack the subtle variations in tone and intonation found in natural speech, potentially impacting the model’s ability to generalise to real-world scenarios.
Field recording involves collecting speech data in natural environments, capturing realistic background noises and variations in speech. This method is valuable for training models that need to perform well in real-world conditions. For example, recording speech data in a busy café or on a bustling street can help train models to filter out background noise and focus on the primary speech signal. This type of data is particularly useful for developing applications like virtual assistants and customer service bots, which must operate effectively in noisy environments.
Partnerships with organisations can provide rich datasets, particularly when collaborating with entities that have access to large amounts of speech data, such as call centres or media companies. These partnerships often come with data privacy and security considerations, requiring careful handling to ensure compliance with relevant regulations. For instance, a partnership with a call centre might provide access to recorded customer service interactions, offering valuable training data for developing customer service bots. However, ensuring that the data is anonymised and protected is critical to maintaining user privacy and trust.
Publicly available datasets offer a valuable resource for training ML models, providing access to well-annotated speech data that covers a wide range of speech patterns. Open-source datasets like LibriSpeech and Common Voice are widely used in the research community, offering extensive collections of speech data for various applications. These datasets are often annotated with detailed metadata, such as speaker information and transcription text, providing rich training material for ML models. For example, the Common Voice dataset, developed by Mozilla, includes contributions from volunteers worldwide, covering numerous languages and dialects. Utilising such datasets can accelerate the development of speech-based applications, providing a solid foundation for training robust models.
Future Trends in Speech Data for AI
The future of speech data in AI is marked by several emerging trends that promise to enhance the capabilities and applications of ML models:
- Multilingual Models: As global communication becomes increasingly important, the development of models that can understand and process multiple languages is a key trend. This requires comprehensive multilingual speech datasets.
- Context-aware Models: Future models will be designed to understand the context in which speech occurs, improving their ability to interpret and respond accurately. This involves training models on context-rich speech data.
- Emotion Recognition: Recognising emotions in speech is a growing area of interest. Emotionally aware models can provide more personalised and empathetic responses, enhancing user experience.
- Privacy-preserving Data Collection: With increasing concerns about data privacy, new methods for collecting and using speech data while preserving user privacy are being developed. Techniques like differential privacy and federated learning are gaining traction.
- Adaptive Learning Models: Models that can continuously learn and adapt to new speech patterns and languages will become more prevalent. This requires ongoing data collection and model training to keep up with evolving speech trends.
The future of speech data in AI is marked by several emerging trends that promise to enhance the capabilities and applications of ML models. These trends include the development of multilingual models, context-aware models, emotion recognition, privacy-preserving data collection, and adaptive learning models.
Multilingual models are becoming increasingly important as global communication expands. These models are designed to understand and process multiple languages, requiring comprehensive multilingual speech datasets. For example, a multilingual virtual assistant must be able to switch seamlessly between languages, understanding and responding accurately in each one. Developing such models involves training on diverse datasets that include speech samples from various languages, ensuring the model can handle different linguistic structures and phonetic variations.
Context-aware models represent another significant trend, focusing on understanding the context in which speech occurs. These models aim to improve their ability to interpret and respond accurately by considering the surrounding context, such as the speaker’s intent and the environmental conditions. For instance, a context-aware customer service bot might use additional information, such as the customer’s previous interactions and the current conversation’s context, to provide more relevant and accurate responses. Training these models involves using context-rich speech data that includes metadata about the situation and environment in which the speech was recorded.
Emotion recognition in speech is a growing area of interest, aiming to develop models that can recognise and respond to human emotions. Emotionally aware models can provide more personalised and empathetic responses, enhancing user experience. For example, a virtual assistant that can detect frustration in a user’s voice might respond more calmly and offer additional support. Training these models requires speech data annotated with emotional labels, capturing the nuances of different emotions and their vocal expressions.
Privacy-preserving data collection is becoming increasingly important with growing concerns about data privacy. New methods for collecting and using speech data while preserving user privacy are being developed, such as differential privacy and federated learning. Differential privacy involves adding noise to the data to protect individual privacy while maintaining overall data utility. Federated learning, on the other hand, allows models to be trained across multiple devices without sharing the raw data, ensuring user privacy. These techniques are crucial for maintaining trust and compliance with data protection regulations.
Adaptive learning models represent a future trend where models continuously learn and adapt to new speech patterns and languages. These models require ongoing data collection and training to keep up with evolving speech trends.
For example, an adaptive virtual assistant might learn new slang and colloquial expressions over time, improving its ability to understand and respond to users. Implementing adaptive learning involves creating systems that can update their training data and models regularly, ensuring they remain accurate and relevant.
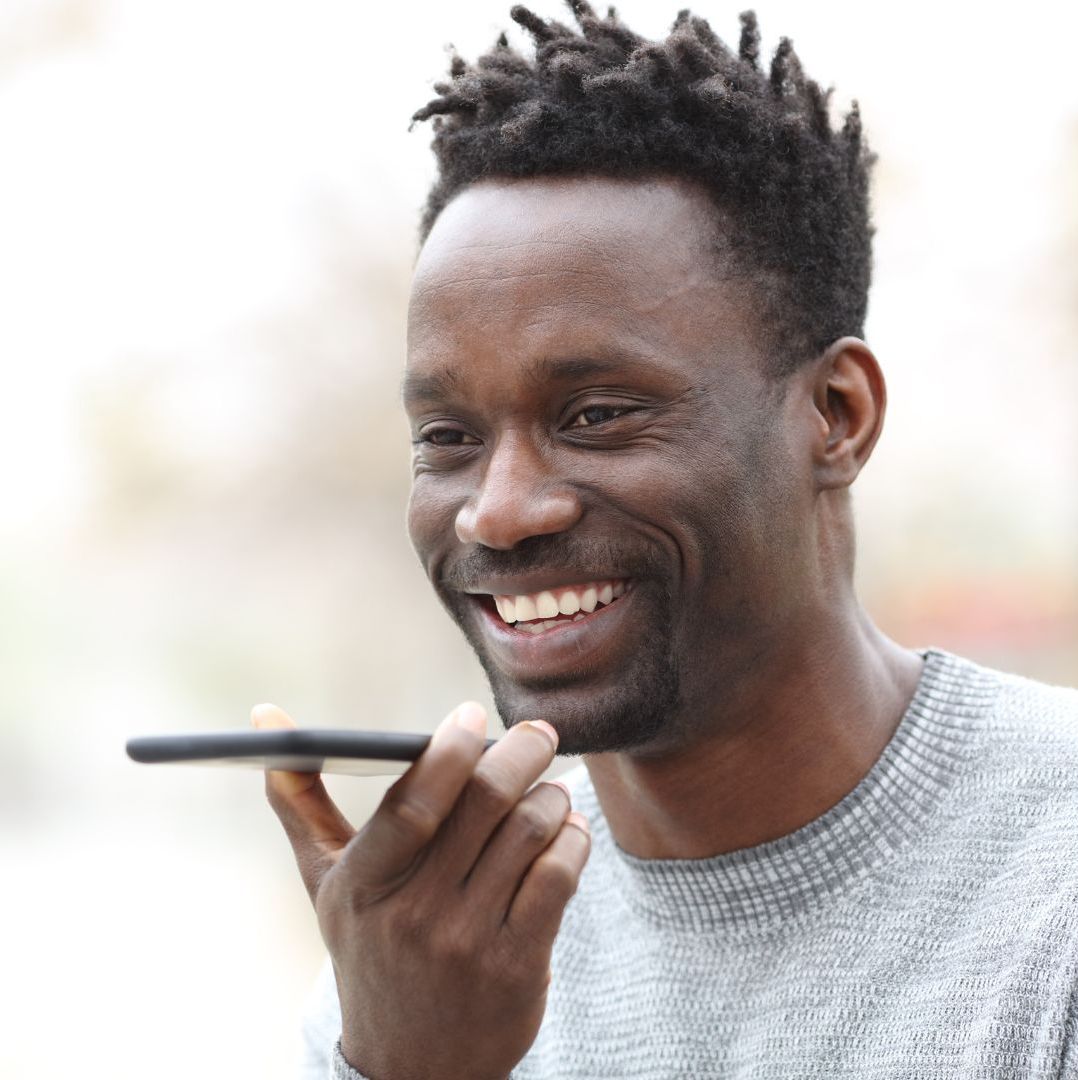
Key Tips on the Importance of Speech Data
- Ensure Data Diversity: Collect speech data from diverse sources to improve model robustness and inclusivity.
- Focus on Data Quality: High-quality, accurately annotated data is crucial for training effective ML models.
- Implement Data Augmentation: Use data augmentation techniques to enhance the variability and robustness of your datasets.
- Stay Updated on Trends: Keep abreast of emerging trends in speech data and incorporate them into your data collection and model training strategies.
- Prioritise Privacy: Ensure that your data collection methods prioritise user privacy and comply with relevant regulations.
The importance of speech data in machine learning cannot be overstated. From training robust NLP and ASR models to enhancing AI accuracy and driving real-world applications, speech data is foundational to the success of AI systems. As we move forward, the methods for collecting and utilising speech data will continue to evolve, driven by the need for more inclusive, accurate, and privacy-preserving AI solutions.
Understanding and leveraging the importance of speech data is essential for AI developers, machine learning engineers, data analysts, tech companies, and academic researchers. By focusing on data diversity, quality, and the latest trends, these stakeholders can develop more effective and impactful AI models.
Resources on the Importance of Speech Data
Wikipedia: Machine Learning – Machine learning is a field of study in artificial intelligence concerned with the development and study of statistical algorithms that can learn from data and generalise to unseen data and thus perform tasks without explicit instructions.
Way With Words: Speech Collection – Way With Words specialises in creating comprehensive speech datasets, including transcripts for machine learning purposes. This service supports the development of advanced automatic speech recognition models using natural language processing for specific languages and various domains. Each dataset can be tailored to specific dialects, demographics, or other required conditions.