10 Open-source Speech Data Resources for Machine Learning
What Open-source Speech Datasets are Available for Machine Learning?
The foundation of effective speech recognition systems lies in the quality and diversity of the speech datasets they are trained on. Open-source speech data has become a cornerstone for researchers, data scientists, technology entrepreneurs, and software developers working to refine and innovate in the realm of speech recognition. But what exactly makes a speech dataset valuable for machine learning? And how do we navigate the vast sea of available datasets to find those that best meet our needs?
When evaluating speech datasets for machine learning purposes, several key questions arise: How diverse are the accents, dialects, and languages represented? Does the dataset include a wide range of speaking styles and environments? How well does the dataset handle noisy or real-world audio conditions? Addressing these questions is crucial for developing robust, adaptable speech recognition systems that can perform well across various applications, from data analytics to customer service solutions.
This short article will explore ten open-source speech datasets invaluable for machine learning, delving into their unique characteristics, strengths, and potential applications. By providing this overview, we aim to assist data scientists, developers, and AI-focused industries in making informed decisions about which datasets can best support their projects and drive technological advancements in speech recognition.
Top Open-source Speech Data Resources for Machine Learning
#1 LibriSpeech
LibriSpeech is a widely recognised open-source speech dataset derived from audiobooks in the LibriVox project, offering over 1000 hours of English speech. Its clean and noisy speech segments are perfect for training and testing speech recognition models, providing a diverse range of accents and recording conditions.
LibriSpeech, deriving its content from the LibriVox project, stands out as a monumental open-source speech dataset, boasting over 1000 hours of English speech. This dataset is meticulously crafted from audiobooks, which means it captures a wide array of literary genres, ensuring a rich diversity in vocabulary, intonation, and expression. The presence of both clean and noisy speech segments within LibriSpeech makes it an invaluable asset for training and testing speech recognition models.
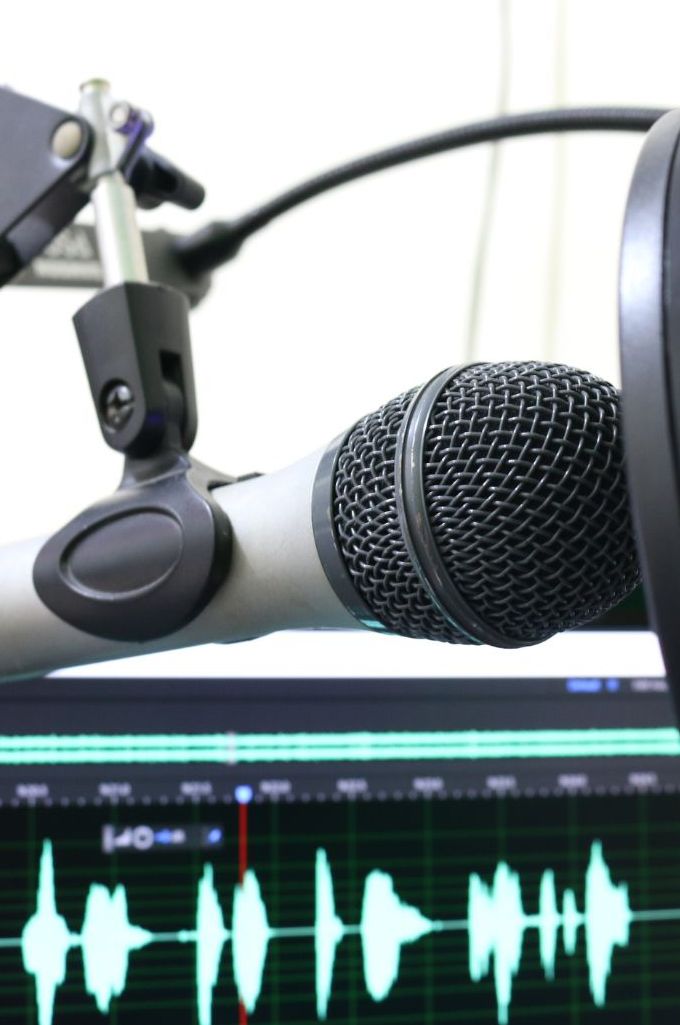
This diversity prepares models to handle a variety of acoustic environments, from studio-quality recordings to scenarios with background noise, reflecting real-world applications. Furthermore, the dataset’s extensive coverage of different accents and dialects enhances its utility, enabling the development of speech recognition systems that are inclusive and adaptable across global English-speaking populations.
The significance of LibriSpeech extends beyond its immediate utility in training algorithms. By offering a broad spectrum of recording conditions and speaker demographics, it challenges and pushes the boundaries of what machine learning dataset models can understand and interpret. This capability is crucial for creating technologies that can accurately transcribe and understand speech in educational software, audiobook readers, and voice-activated assistants.
The dataset not only serves as a foundation for developing robust speech recognition models but also stimulates innovation in AI and machine learning, encouraging researchers and developers to explore new methodologies and algorithms that can leverage the rich and complex nature of human speech.
#2 Common Voice by Mozilla
Mozilla’s Common Voice project is a global, multilingual dataset that includes voices from tens of thousands of individuals across various languages, making it one of the most diverse and scalable open-source speech datasets for machine learning.
Mozilla’s Common Voice project marks a pioneering step towards creating an open, accessible, and multilingual speech dataset. With contributions from tens of thousands of individuals across the globe, Common Voice stands as one of the most diverse and scalable machine learning datasets. Its inclusive approach to data collection embraces a wide variety of languages, accents, and dialects, making it a treasure trove for developers aiming to build and enhance speech recognition systems that cater to a global audience.
The project’s commitment to diversity not only aids in the development of more accurate models but also ensures that speech technologies become more equitable and accessible to users from different linguistic backgrounds. The ongoing expansion of Common Voice is fuelled by a crowdsourcing model, which continuously enriches the dataset with new voices and languages. This dynamic nature of Common Voice allows it to adapt to the evolving needs of machine learning projects and speech technologies, ensuring that the dataset remains relevant and reflective of the world’s linguistic diversity.
Moreover, its open-source nature fosters a collaborative environment where researchers, developers, and language enthusiasts can contribute and benefit from a shared resource. As AI and machine learning continue to integrate into everyday technologies, the importance of datasets like Common Voice in breaking down language barriers and building more inclusive digital experiences cannot be overstated.
#3 TED-LIUM
The TED-LIUM dataset comprises transcribed TED Talks, offering a rich source of varied speech topics, accents, and recording qualities. It’s an excellent resource for training models to recognise academic and professional speech.
The TED-LIUM dataset, with its foundation in the intellectually stimulating realm of TED Talks, offers an exceptional resource for speech recognition research. This dataset encompasses a broad spectrum of topics, from science and technology to art and education, spoken by experts from around the world.
Such diversity not only enriches the dataset with a variety of accents and delivery styles but also embeds a wealth of domain-specific terminology within its transcriptions. This makes TED-LIUM particularly suited for training machine learning dataset models to recognise academic and professional speech, enabling applications that require a high degree of understanding and contextual awareness, such as lecture transcription services, educational assistants, and professional dictation tools.
Beyond its immediate utility, TED-LIUM also challenges speech recognition systems to deal with the nuances of live presentations, including spontaneous speech, audience interactions, and varying acoustic environments. These characteristics mimic the complexities of real-world audio, preparing models to perform more reliably outside controlled settings.
The rich linguistic and acoustic landscape captured in the TED-LIUM dataset not only facilitates the development of sophisticated speech recognition technologies but also contributes to the broader goal of making information more accessible. By enabling machines to accurately transcribe and interpret these talks, TED-LIUM plays a pivotal role in democratising knowledge and fostering a more informed society.
#4 VoxForge
VoxForge was created to collect transcriptions of speech in multiple languages for use in open-source speech data recognition engines. It emphasises the diversity of speaker accents, making it valuable for developing more inclusive speech recognition technologies.
VoxForge represents a grassroots initiative aimed at democratising speech recognition technology through the creation of an open-source, multilingual speech dataset. Born from the community’s need for freely available speech data to train speech recognition engines, VoxForge emphasises the collection of diverse speaker accents. This focus addresses a critical challenge in speech recognition: the ability of systems to accurately understand and process speech from speakers of various linguistic backgrounds.
By providing data in multiple languages and accent variations, VoxForge contributes to the development of more inclusive and universally accessible speech technologies. This inclusivity is vital for applications ranging from language learning tools to global customer service solutions, ensuring that voice-activated technologies can serve a wider audience.
VoxForge’s collaborative and open model of data collection encourages participation from individuals around the world, continuously enriching the dataset with new voices and accents. This dynamic and evolving nature of VoxForge not only keeps the dataset relevant in the face of changing linguistic trends but also fosters a sense of community among contributors who are collectively enhancing the capabilities of open-source speech data recognition technologies.
As speech interfaces become increasingly prevalent in devices and applications, the need for datasets like VoxForge that prioritise linguistic diversity and accessibility becomes more apparent. Through its contributions to the field, VoxForge not only advances the technical aspects of speech recognition but also champions the cause of linguistic equity in the digital age.
#5 TIMIT Acoustic-Phonetic Continuous Speech Corpus
The TIMIT corpus is fundamental for phonetic and linguistic research, providing detailed phonetic annotations. It’s instrumental in developing speech recognition systems that require precise phonetic detail.
The TIMIT Acoustic-Phonetic Continuous Speech Corpus stands as a cornerstone in the intersection of phonetic and linguistic research with speech technology. Renowned for its detailed phonetic annotations and broad sampling of dialects within American English, TIMIT provides a meticulously structured resource for developing speech recognition systems that require granular phonetic detail.
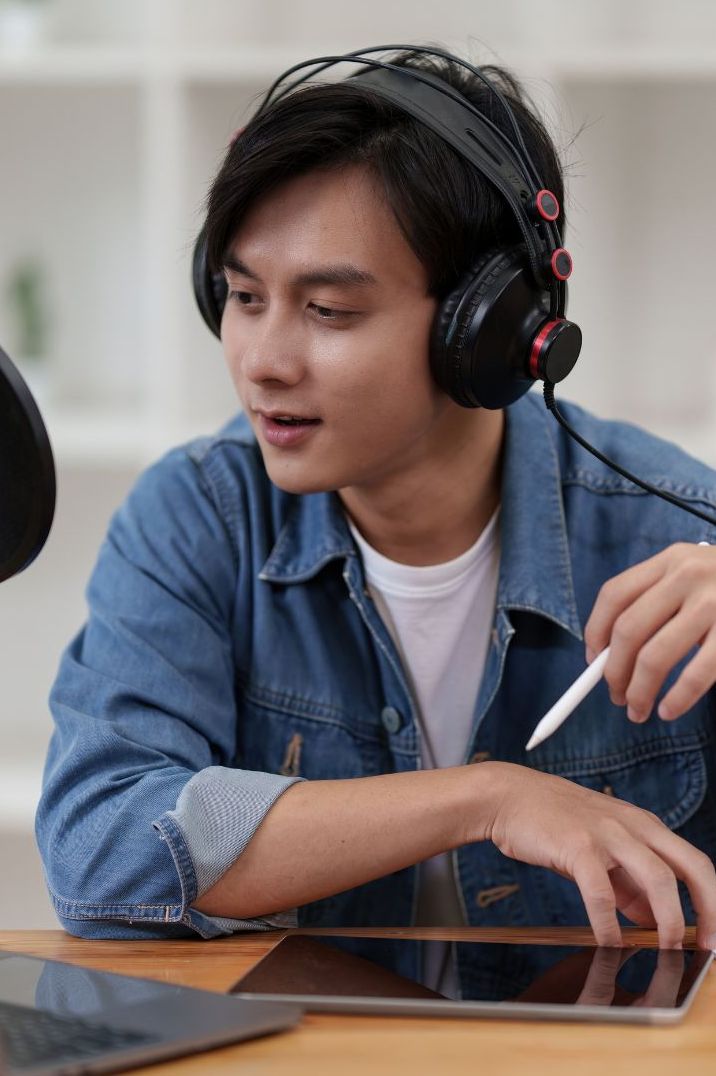
This level of detail supports a wide range of research and development activities, from studying the nuances of American English accents to training algorithms that can discern subtle differences in speech sounds. TIMIT’s structured approach to phonetic annotation enables researchers to delve deeply into the acoustic properties of speech, fostering advancements in speech synthesis, speech to text, and linguistic analysis technologies.
The enduring relevance of the TIMIT corpus in speech technology research underscores its value in refining speech recognition models. By offering a deep dive into the phonetic aspects of speech, TIMIT equips developers with the tools to improve the accuracy and naturalness of speech interfaces. The corpus has been instrumental in advancing the field, providing a benchmark for evaluating speech recognition algorithms and inspiring new approaches to understanding human speech.
As speech technologies continue to evolve, the foundational insights provided by TIMIT will remain crucial in pushing the boundaries of what is possible in speech recognition and synthesis, ensuring that future innovations are built on a solid understanding of the intricacies of speech.
#6 Google’s Speech Commands Dataset
This dataset from Google contains short audio clips of spoken words designed for command recognition in applications. It’s an essential resource for developing voice-controlled interfaces.
Google’s Speech Commands Dataset is an invaluable tool in the realm of voice-controlled technology, offering a collection of short audio clips that cover a wide range of spoken commands. This dataset is designed to cater specifically to the development and enhancement of voice-activated interfaces, where accuracy and responsiveness are paramount.
With thousands of samples spanning over 30 unique commands, such as “yes,” “no,” “stop,” “go,” and numbers from zero through nine, it provides a foundational dataset for training machine learning models to recognise and act on verbal instructions. The diversity in the dataset, including variations in speaker age, accent, and recording quality, mirrors the complexity of real-world usage, ensuring that systems developed using this dataset are robust and versatile.
The strategic compilation of Google’s Speech Commands Dataset addresses a critical need in the tech industry for reliable voice command recognition, a feature increasingly integrated into smartphones, home automation devices, and in-car systems. By focusing on concise, commonly used commands, the dataset simplifies the development process for researchers and developers, allowing them to create more intuitive and user-friendly voice interfaces.
Additionally, the dataset serves as a benchmark for evaluating the performance of voice recognition algorithms, facilitating advancements in the field by providing a clear standard against which to measure progress. As voice-activated technologies continue to proliferate, the significance of having a high-quality, accessible dataset like Google’s Speech Commands cannot be overstated, driving innovation and improving the way we interact with our devices.
#7 CHiME
The CHiME datasets focus on challenging speech recognition in noisy environments, such as streets or cafes, making them invaluable for creating systems that perform well in real-world settings.
The CHiME dataset series stands out for its focus on addressing one of the most challenging aspects of speech recognition: performance in noisy environments. This dataset is meticulously curated to include a variety of noise conditions, from the bustling activity of a street to the background chatter of a café, simulating real-life scenarios where speech recognition systems often struggle.
The CHiME challenges, associated with these datasets, push the boundaries of what’s possible in speech recognition technology, driving researchers and developers to innovate solutions that can effectively filter out background noise and accurately identify spoken words. The datasets have evolved through several iterations, each increasing in complexity and realism, thereby providing a progressive platform for enhancing noise-robust speech recognition systems.
The practical applications of the advancements spurred by the CHiME datasets are vast and impactful. In today’s world, where voice-activated assistants, telecommunication devices, and automated transcription services have become integral to daily life, the ability to accurately recognise speech in noisy environments significantly enhances the utility and user satisfaction of these technologies.
For instance, improved noise-robust speech recognition can revolutionise how voice commands are used in smart homes and vehicles, making interactions more natural and reliable. Moreover, the CHiME datasets contribute to the development of assistive technologies, enabling clear voice communication for individuals in noisy workplaces or those with hearing impairments. As the demand for sophisticated speech recognition capabilities grows, the insights and breakthroughs derived from the CHiME challenges will undoubtedly play a crucial role in shaping future technologies.
#8 LibriTTS
An extension of LibriSpeech, LibriTTS targets text-to-speech systems with high-quality, large-scale English speech data. It includes varied speaking styles and environments for comprehensive training.
Building on the foundation of LibriSpeech, LibriTTS extends its predecessor’s vision by focusing specifically on high-quality, large-scale English speech data for text-to-speech (TTS) systems. This dataset is designed to facilitate the development of TTS technologies that can generate natural, human-like speech, accommodating a wide range of speaking styles and environmental conditions. With its comprehensive collection of read speech, LibriTTS includes both clean and noisy audio samples, along with corresponding textual transcriptions.
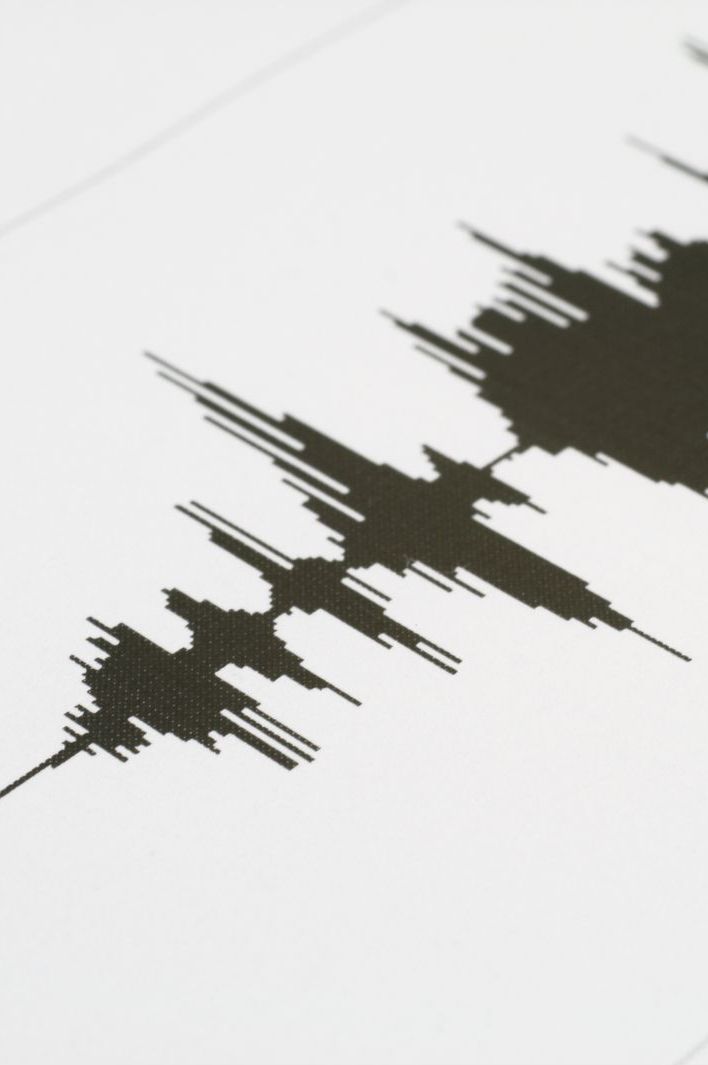
This alignment between audio and text is crucial for training TTS models to produce accurate and intelligible speech output. The dataset not only covers a broad spectrum of accents and dialects but also incorporates diverse recording settings, from studio-quality to more casual environments, mirroring the variable quality of input that TTS systems must handle in real-world applications.
The advent of LibriTTS has significant implications for the advancement of text-to-speech technology, particularly in enhancing the naturalness and expressiveness of synthesised speech. By providing a rich dataset that reflects the nuances of human speech, including intonation, emotion, and emphasis, developers can create TTS systems that offer a more engaging and lifelike user experience.
This progress is especially relevant in the development of educational tools, audiobooks, and virtual assistants, where the quality of speech output directly affects user engagement and comprehension. Furthermore, the detailed annotations and variety of speech samples in LibriTTS enable research into new techniques for speech synthesis, including deep learning approaches that can learn from the subtleties of human speech patterns. As TTS technologies become increasingly integrated into digital platforms, the contributions of LibriTTS to creating more natural and accessible speech interfaces will continue to be invaluable.
#9 MUSAN
MUSAN is a free dataset offering music, speech, and noise components. It’s particularly useful for training models on speech activity detection and speaker identification in mixed audio scenarios.
The MUSAN dataset is a comprehensive collection of music, speech, and noise recordings that serve as a versatile resource for training machine learning models on a variety of audio processing tasks. This free dataset is particularly valuable for applications involving speech activity detection and speaker identification, providing a rich array of audio samples that include background noises, musical segments, and spoken words from multiple speakers.
The diversity of the MUSAN dataset enables developers to train models that can accurately differentiate between speech and non-speech elements in audio recordings, an essential capability for voice-activated systems, surveillance technologies, and automated transcription services. Additionally, the inclusion of various noise types, from environmental sounds to instrumental music, allows for the development of noise-resistant speech recognition systems that maintain high accuracy levels even in challenging acoustic environments.
MUSAN’s contribution to the field of speech technology extends beyond its immediate applications. By offering a broad spectrum of audio components, it supports the exploration of new methods for speech enhancement, speaker diarisation, and audio segmentation. These capabilities are crucial for improving the performance of communication devices, content analysis tools, and audio archiving systems.
The open availability of the MUSAN dataset encourages widespread innovation, enabling researchers and developers to experiment with novel approaches to audio processing. As the demand for sophisticated audio analysis and speech recognition technologies continues to grow, the MUSAN dataset remains a key resource for driving advancements and overcoming the challenges associated with processing complex audio signals.
#10 AMI Meeting Corpus
The AMI Meeting Corpus provides audio recordings from meetings, making it a unique resource for developing speech recognition systems tailored to corporate and organisational settings.
The AMI Meeting Corpus is a distinctive resource tailored to the development of speech recognition systems for corporate and organisational settings. This dataset provides audio recordings from real and simulated meetings, complete with annotations, transcriptions, and speaker diarisation information. The unique context of meetings presents specific challenges for speech recognition, including overlapping speech, varying speaking styles, and the use of domain-specific jargon.
The AMI Meeting Corpus addresses these challenges by offering a rich dataset that captures the complexity of conversational dynamics in a meeting environment. This enables the development of speech recognition models that can accurately transcribe meetings, recognise individual speakers, and understand nuanced conversations. The practical benefits of such advancements are substantial, facilitating more efficient meeting documentation, enhanced communication accessibility, and the development of intelligent meeting assistants.
Beyond its immediate utility, the AMI Meeting Corpus also supports research into more advanced topics in speech technology, such as emotion recognition, automatic summarisation, and the detection of decision-making processes. By providing a detailed snapshot of natural speech in a structured yet dynamic setting, the dataset paves the way for innovations that can improve how we capture and interpret the flow of information in collaborative environments.
As businesses and organisations increasingly rely on digital tools for communication and documentation, the insights gained from the AMI Meeting Corpus will play a crucial role in enhancing the effectiveness and accessibility of these technologies. The dataset not only advances the technical capabilities of speech recognition systems but also contributes to the creation of more intelligent and responsive tools that can support the diverse needs of the modern workplace.
Key Machine Learning Dataset Tips
- Ensure the dataset you choose aligns with your project’s language, dialect, and acoustic requirements.
- Consider the legal and ethical implications of using speech data, especially regarding user consent and data privacy.
- Regularly update and test your models with new data to improve accuracy and adaptability.
- Way With Words provides highly customised and appropriate data collections for speech and other use cases, aiding technologies in developing advanced AI language and speech capabilities.
The exploration of open-source speech datasets is more than just an academic exercise; it’s a journey into the heart of what makes human-computer interaction natural and intuitive. As we’ve seen through the diverse range of datasets discussed, there is no one-size-fits-all solution. Each dataset offers unique insights and challenges, pushing the boundaries of what’s possible in speech recognition technologies.
For technology entrepreneurs, software developers, and industries leveraging AI, the choice of dataset can significantly impact the success of speech recognition applications. From enhancing customer service solutions to developing sophisticated data analytics platforms, the potential applications are as varied as the datasets themselves.
The key piece of advice is to approach the selection of speech datasets with a clear understanding of your project’s specific needs. Consider the linguistic diversity, acoustic variability, and real-world applicability of the datasets you evaluate. By doing so, you can harness the full power of open-source speech data to drive innovation and improve machine learning dataset models.
In closing, we encourage you to explore the services offered by Way With Words for creating custom speech datasets and polishing machine transcripts. Their expertise in speech collection and transcription can provide invaluable support for your projects, ensuring that your speech recognition technologies meet the highest standards of accuracy and reliability.
Open-source Speech Data Resources
Way With Words Speech Collection Services: “We create speech datasets including transcripts for machine learning purposes. Our service is used for technologies looking to create or improve existing automatic speech recognition models (ASR) using natural language processing (NLP) for select languages and various domains.”
Way With Words Machine Transcription Polishing Services: “We polish machine transcripts for clients across a number of different technologies. Our machine transcription polishing (MTP) service is used for a variety of AI and machine learning purposes. User applications include machine learning models that use speech-to-text for artificial intelligence research, FinTech/InsurTech, SaaS/Cloud Services, Call Centre Software and Voice Analytic services for the customer journey.”
OpenSLR is a site devoted to hosting speech and language resources, such as training corpora for speech recognition, and software related to speech recognition. We intend to be a convenient place for anyone to put resources that they have created, so that they can be downloaded publicly.