Real-Time Speech Data Applications: Enhancing Speed and Accuracy
Can Speech Data be Used for Real-time Applications?
Real-time speech data processing has revolutionised how we interact with technology, enabling applications to deliver faster, more accurate responses across diverse sectors using speech data to improve many AI models. Whether in customer service, healthcare, or education, real-time speech data fuels innovations that transform communication.
The question is: Can speech data truly support real-time applications, and how can it enhance speed and accuracy?
Many professionals often wonder:
- What are the key advantages of real-time speech data applications?
- Which technologies make real-time speech data processing possible?
- What challenges arise in real-time applications, and how can they be addressed?
This short guide explores these questions, delving into the benefits, technologies, and future trends in real-time speech data applications while providing actionable insights for AI developers, data scientists, and technology firms.
Key Topics Regarding Real-Time Speech Data Processing
Advantages of Real-Time Speech Data Applications
Real-time speech data offers distinct advantages:
- Speed and Efficiency: Real-time systems enable instantaneous responses, ideal for voice-activated assistants and live customer support.
- Improved Accuracy: Real-time applications continuously refine data through feedback loops, minimising errors.
- Enhanced User Experience: Interactive applications powered by real-time speech data provide personalised, context-aware experiences.
Supporting Data: For example, a study on customer service chatbots showed a 20% increase in customer satisfaction when real-time speech recognition was implemented.
Real-time speech data applications provide transformative benefits that make them indispensable across industries. These advantages are deeply rooted in their ability to handle dynamic interactions with speed and precision, enabling organisations to innovate and optimise user experiences.
Enhanced Operational Speed
One of the most significant advantages of real-time speech data applications is their ability to process and respond to voice inputs instantaneously. This capability is particularly beneficial in environments like customer service, where real-time responses are critical to maintaining user satisfaction. Unlike traditional systems, which often involve delays in processing, real-time applications eliminate these bottlenecks. For instance, voice assistants such as Siri and Alexa can quickly retrieve information or perform tasks, significantly improving workflow efficiency for both end-users and businesses.
Data-Driven Accuracy
Another key strength of real-time speech data systems lies in their ability to refine data continuously. Real-time feedback loops allow systems to learn from previous interactions, improving the precision of outputs over time. This dynamic adaptability is crucial in applications such as medical diagnostics, where even minor errors can have significant consequences. In the financial sector, trading platforms benefit from real-time speech analysis to capture and execute commands accurately, preventing costly mistakes.
Personalisation and Context Awareness
Real-time speech data applications enhance user experiences by providing contextually relevant and personalised interactions. By analysing not only the spoken words but also the tone, intent, and context, these systems deliver responses tailored to individual users. For example, e-commerce platforms use real-time voice recognition to recommend products based on user preferences. This level of personalisation fosters customer loyalty and increases engagement, as users feel understood and valued.
Technologies for Real-Time Data Processing
Several technologies underpin real-time speech data applications:
- Automatic Speech Recognition (ASR): Tools like Speechmatics and Google ASR enable efficient voice-to-text conversions.
- Natural Language Processing (NLP): NLP enhances understanding of context and intent.
- Edge Computing: By processing data locally, edge computing reduces latency.
- Cloud Integration: Cloud platforms like AWS provide scalability for handling large datasets.
Case Study:
A healthcare startup reduced response times by 30% using ASR integrated with edge computing to process patient enquiries.
The success of real-time speech data applications is underpinned by a sophisticated combination of technologies. These technologies work together to ensure that data is processed, analysed, and utilised effectively in real time.
Automatic Speech Recognition (ASR)
ASR serves as the backbone of real-time speech data applications, converting spoken language into text that machines can understand. Modern ASR tools, such as Speechmatics and Google Cloud Speech-to-Text, utilise deep learning algorithms to achieve high accuracy rates. These systems are trained on vast datasets, allowing them to recognise diverse accents, dialects, and languages. For example, in customer service scenarios, ASR enables seamless interaction between users and automated systems by accurately transcribing user inputs in real time.
Natural Language Processing (NLP)
NLP enhances the capabilities of ASR by interpreting the meaning behind transcribed speech. By analysing syntax, semantics, and context, NLP systems allow applications to understand intent and generate appropriate responses. Advanced NLP frameworks like BERT and GPT-4 have been instrumental in improving the contextual awareness of voice-activated systems. For instance, NLP-powered chatbots can detect customer sentiment and adjust their responses accordingly, ensuring more empathetic and relevant interactions.
Edge Computing and Cloud Integration
Edge computing processes data locally on devices, reducing latency and ensuring faster response times. This approach is particularly useful in applications like IoT-enabled devices, where connectivity issues could otherwise delay processing. In contrast, cloud integration offers scalability and computational power, making it ideal for handling extensive datasets in applications such as language translation. The combination of edge and cloud computing enables a balance between speed and scalability, optimising performance for real-time speech data systems.
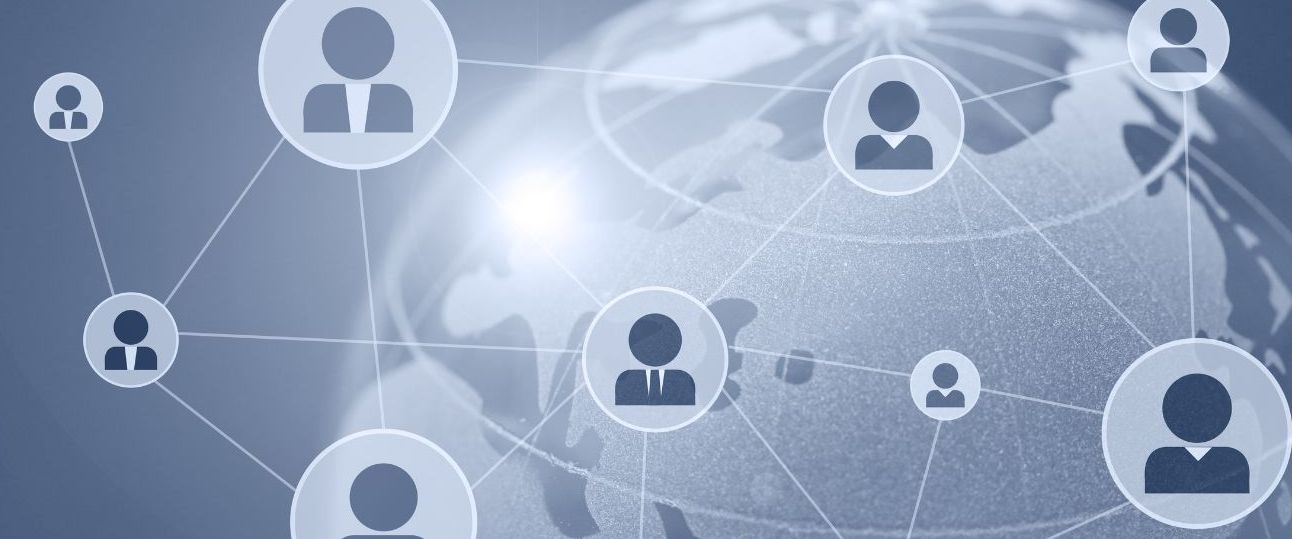
Challenges and Solutions in Real-Time Speech Data Processing
Despite its potential, real-time speech data processing faces challenges:
- Latency Issues: Delays in processing can disrupt user interactions.
- Data Security: Handling sensitive data in real-time requires robust encryption protocols.
- Accuracy in Diverse Environments: Background noise and varying accents can affect accuracy.
Solutions:
- Noise-Cancellation Technology: AI-powered noise filtering enhances clarity.
- Adaptive Algorithms: Machine learning models trained on diverse datasets improve adaptability.
While real-time speech data applications offer immense potential, implementing them effectively requires addressing several challenges. From technical hurdles to ethical considerations, these challenges demand innovative solutions to unlock the full potential of real-time systems.
Latency and Performance Bottlenecks
Latency remains one of the primary challenges in real-time applications. Even milliseconds of delay can disrupt the user experience, particularly in critical scenarios like emergency response systems. To overcome this, developers are employing technologies such as edge computing and lightweight algorithms. Additionally, optimising network infrastructure and leveraging high-speed connections can minimise latency and ensure smooth performance.
Security and Privacy Concerns
Processing speech data in real time often involves handling sensitive information, such as personal details or financial data. Without robust security measures, these systems can become vulnerable to breaches. Encryption protocols like TLS and end-to-end encryption are essential for safeguarding data during transmission. Moreover, compliance with regulations such as GDPR and HIPAA ensures that user privacy is protected.
Accuracy in Complex Environments
Background noise, diverse accents, and varying speech patterns can impact the accuracy of real-time speech recognition. To address this, developers are incorporating noise-cancellation technologies and training machine learning models on diverse datasets. Adaptive systems that can learn and adjust to new environments further enhance accuracy, making them more reliable across different use cases.
Case Studies on Real-Time Applications
- Customer Support Automation: Companies like Amazon use real-time speech data for Alexa, offering seamless customer interactions.
- Healthcare Diagnosis Tools: Real-time processing enables voice-controlled medical devices, improving accessibility for disabled individuals.
- Educational Tools: Language learning platforms leverage real-time speech analysis for pronunciation corrections.
Real-world applications of real-time speech data demonstrate its versatility and effectiveness in solving practical challenges across industries.
Customer Support Automation
Companies like Amazon and Google have revolutionised customer support by integrating real-time speech recognition into their virtual assistants. Alexa, for example, uses ASR and NLP to handle millions of user queries daily, providing quick and accurate responses. This automation has reduced wait times and operational costs, while improving customer satisfaction.
Healthcare Diagnosis Tools
In healthcare, real-time speech data applications are enabling voice-controlled diagnostic tools that assist clinicians in documenting and analysing patient information. For example, a healthcare provider implemented a voice recognition system to capture patient symptoms and generate real-time reports, reducing documentation time by 40%. These tools also improve accessibility for disabled individuals, offering voice-activated controls for medical devices.
Educational Platforms
Language learning platforms like Duolingo utilise real-time speech data to analyse pronunciation and provide instant feedback. By leveraging ASR and NLP, these applications create an engaging learning experience for users. For instance, a feature that corrects pronunciation in real time has been shown to improve language acquisition rates by 25%, demonstrating the effectiveness of this technology in education.
Future Trends in Real-Time AI
Emerging trends include:
- Multilingual Support: Real-time applications are expanding to support multiple languages, making them more inclusive.
- Context-Aware AI: Advanced NLP models understand nuanced conversations.
- Integration with IoT: Smart home devices are increasingly utilising real-time speech data for intuitive controls.
Projection: By 2028, the market for real-time speech data applications is expected to grow at a CAGR of 14.6%, driven by innovations in AI.
The evolution of real-time speech data applications is driving the development of innovative solutions that expand their scope and functionality.
Multilingual and Inclusive Systems
Future real-time applications will increasingly support multiple languages, making them more accessible to global users. Advances in multilingual ASR models, such as Whisper by OpenAI, allow systems to process and translate speech across dozens of languages. This inclusivity is crucial for industries like international customer service and global e-commerce.
Context-Aware and Emotionally Intelligent AI
Next-generation AI systems are becoming more context-aware, capable of understanding nuanced conversations and detecting emotions. By integrating sentiment analysis with real-time processing, applications can offer empathetic responses tailored to individual users. For example, a virtual therapist could use emotional cues to provide more effective mental health support.
IoT Integration and Smart Homes
As IoT adoption grows, real-time speech data applications will play a central role in smart home ecosystems. Devices like thermostats, lights, and security systems will rely on real-time voice commands for seamless control. These integrations will create more intuitive and efficient living environments, where devices anticipate user needs based on speech patterns and preferences.
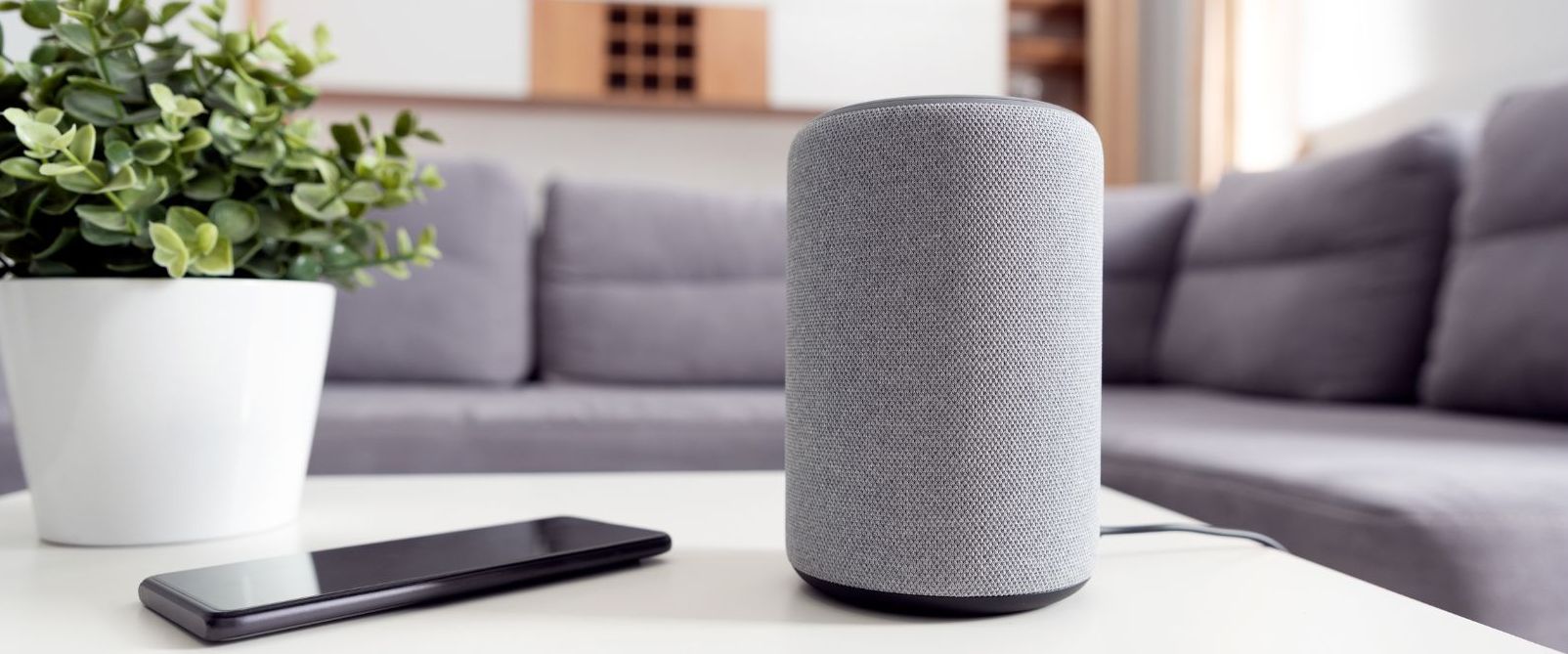
Key Speech Data Processing Tips
- Invest in Robust ASR Tools: Choose tools optimised for your industry’s needs.
- Prioritise Security: Implement encryption and comply with regulations.
- Leverage Edge Computing: Minimise latency for applications requiring immediate responses.
- Test for Accuracy: Continuously refine models using diverse datasets.
- Stay Updated on Trends: Follow industry advancements to remain competitive.
Real-time speech data applications hold immense potential to enhance speed, accuracy, and user satisfaction across industries. By understanding their advantages, leveraging cutting-edge technologies, and addressing key challenges, businesses can unlock opportunities for innovation. Whether you’re developing a virtual assistant or automating processes, investing in real-time speech data ensures a competitive edge in a time of rapid technological progress.
For More Insights, Explore These Resources
Wikipedia: Real-Time Computing: A comprehensive overview of real-time computing principles and applications.
Way With Words: Speech Collection: Tailored solutions for high-quality speech data collection.