10 Best Practices for Speech Data Annotation in African Languages
What Are Best Practices For Speech Data Annotation in African Languages?
The annotation of speech data in African languages presents unique challenges and opportunities. This task is pivotal for developing technologies that are inclusive, effective, and capable of understanding the rich linguistic diversity of the African continent.
As data scientists, technology entrepreneurs, software developers, and industries lean into the future of AI, several key questions emerge: How can we ensure the accuracy and consistency of speech data annotation in African languages? What best practices should be adopted to accommodate the linguistic diversity and complexity of these languages? This introduction will delve into the importance of high-quality speech data annotation, the challenges faced, and the potential impact on AI technologies.
10 Methods For Quality Speech Data Annotation
#1 Understanding Linguistic Diversity
African languages exhibit a wide range of phonetic, grammatical, and syntactic features. Best practices involve a deep understanding of these linguistic nuances to ensure accurate annotations.
The vast linguistic landscape of Africa, with over 2000 languages, presents a complex matrix of phonetic, grammatical, and syntactic features that vary significantly across regions and ethnic groups. This diversity is not just a challenge but also an opportunity for developing AI technologies that are truly inclusive. To navigate this complexity, best practices in speech data annotation must start with a profound understanding of these linguistic nuances.
Annotators need to be well-versed in the phonemic distinctions, tonal variations, and morphosyntactic structures unique to each language. This requires not only theoretical knowledge but also a practical understanding gained through exposure to the language in its natural context. By comprehensively understanding the linguistic features, annotators can ensure that the speech data is accurately represented, which is crucial for the development of effective speech recognition technologies.
Moreover, this understanding aids in the identification and correct annotation of linguistic phenomena that are unique to African languages, such as click sounds in some Khoisan languages or the extensive use of tone in Niger-Congo language families. Accurate annotation of these features is critical because they can significantly alter meaning, which, in turn, affects the performance of speech recognition systems. Collaborating with linguists and language experts who bring a deep cultural and linguistic knowledge ensures that the annotation process respects the complexity and richness of African languages, leading to more reliable and nuanced AI applications.
#2 Cultural Sensitivity and Relevance
Annotation teams need to be culturally aware, ensuring that speech data captures the socio-linguistic context of the language, including idioms, colloquialisms, and cultural nuances.
The task of speech data annotation in African languages goes beyond mere linguistic accuracy; it requires a deep cultural understanding and sensitivity. Languages are inseparable from the cultural contexts in which they are spoken. They carry idioms, colloquialisms, and references that are deeply embedded in the history, traditions, and daily lives of their speakers.
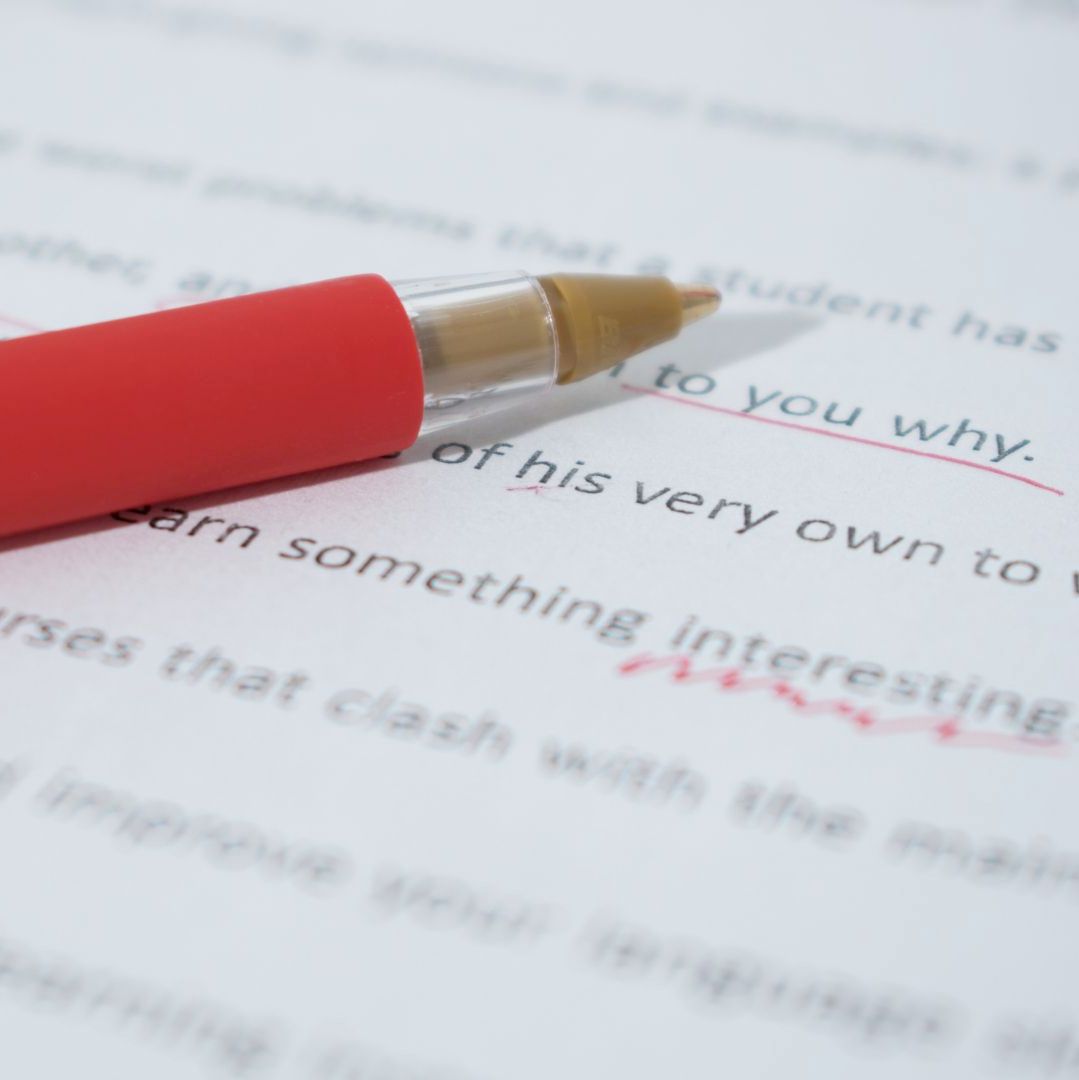
Annotation teams must be culturally aware to ensure that the speech data accurately captures these socio-linguistic nuances. This involves understanding the cultural context of phrases, the significance of certain expressions, and the social norms governing language use. For instance, the use of proverbs or indirect speech as a polite form of communication in many African societies must be accurately captured and annotated to maintain the natural flow and meaning of the speech.
Cultural sensitivity also extends to recognising and respecting the diversity within individual African countries, where multiple languages and dialects coexist. This means acknowledging and representing the linguistic variations and avoiding a one-size-fits-all approach to annotation. By ensuring that speech data reflects the rich socio-linguistic fabric of African communities, AI technologies can achieve greater relevance and acceptance among their users. Engaging with the communities themselves can enhance this process, providing insights into local expressions, variations, and preferences that might not be immediately apparent to outsiders. This approach not only enriches the data but also fosters a sense of ownership and participation among community members, contributing to the creation of AI solutions that are truly by and for Africans.
#3 High-Quality Audio Data Collection
The importance of clear, noise-free audio recordings cannot be overstated. This section will discuss best practices for collecting high-quality audio samples that accurately represent various dialects and accents.
Collecting high-quality audio data is foundational to the success of speech recognition technologies. In the context of African languages, this task is particularly challenging due to the diverse environments and dialectal variations present across the continent. Best practices for audio data collection emphasise the need for clarity and minimal background noise, ensuring that speech is captured as accurately as possible.
This involves using high-quality recording equipment and techniques that can adequately handle the nuances of African languages, from the tonal qualities of Yoruba to the click consonants of Xhosa. It also requires careful selection of recording environments to minimise interference from background noise, which can distort linguistic features and lead to inaccuracies in annotation.
In addition to technical quality, audio data collection must also consider representativeness. This means capturing a wide range of dialects, accents, and speaking styles to ensure that the speech recognition technology can function effectively across different regions and social groups. It involves recording speakers from various age groups, genders, and social backgrounds, reflecting the diversity within the language community. By prioritising both the technical quality and representativeness of audio data, researchers can build robust datasets that serve as a strong foundation for developing speech recognition models that are accurate, reliable, and inclusive of the linguistic diversity found in Africa.
#4 Expert Annotators and Linguists
Employing annotators and linguists who are native speakers or have deep expertise in the target language ensures the precision of annotations.
The precision of speech data annotations in African languages hinges significantly on the expertise of the annotators and linguists involved. Employing native speakers or individuals with deep expertise in the target language is crucial, as they bring an innate understanding of linguistic subtleties that cannot be easily replicated by non-native speakers. These experts are adept at recognising and interpreting nuanced phonetic distinctions, regional dialects, and idiomatic expressions that are critical for accurate annotation. Their knowledge ensures that the annotations reflect the true intent and meaning of the speech, a crucial aspect for training effective and nuanced AI models.
Furthermore, the role of expert annotators and linguists extends beyond mere transcription and annotation. They play a key role in the development of annotation guidelines, training of new annotators, and the quality control processes that ensure the reliability of the annotated datasets. Their expertise allows them to identify and address potential sources of bias or error, such as dialectal variations or ambiguous language use, ensuring that the speech data is both accurate and comprehensive. By leveraging the skills and knowledge of these experts, projects can significantly enhance the quality and utility of speech data annotations, leading to more effective and culturally attuned AI applications.
#5 Annotation Tools and Software
Selecting the right tools that support the specific needs of African languages, including text normalisation and diacritic marking, is crucial.
The selection of annotation tools and software is pivotal in the accurate and efficient speech data annotation in African languages. These tools must be specifically tailored to accommodate the unique characteristics of these languages, including text normalisation and diacritic marking, which are essential for capturing phonetic and tonal nuances. Advanced software that supports these features can significantly reduce the complexity of speech data annotation, enabling more precise and consistent annotations across datasets.
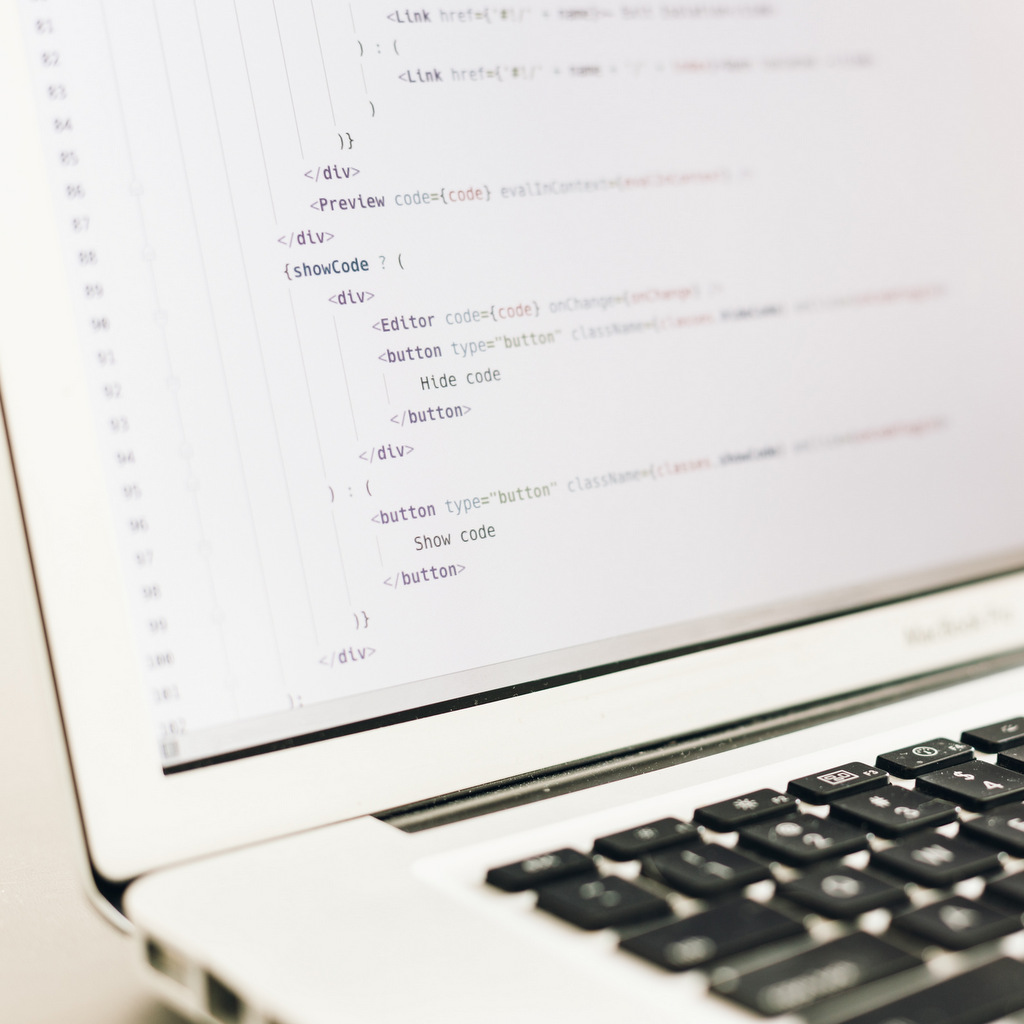
Additionally, these tools should offer a user-friendly interface and workflow that facilitates the annotation process, allowing annotators to focus on the quality of their work rather than struggling with technical issues. Moreover, the flexibility and adaptability of annotation tools are key. They should be capable of integrating with other technologies and platforms, supporting a seamless workflow from audio data collection to annotation and analysis.
This includes compatibility with machine learning algorithms for semi-automated annotation processes, which can enhance efficiency while maintaining high standards of accuracy. By investing in the right annotation tools and software, projects can streamline their annotation processes, reduce errors, and create high-quality datasets that are essential for developing robust and reliable AI technologies for African languages.
#6 Training and Guidelines
Comprehensive training sessions and detailed annotation guidelines are essential to maintain consistency and accuracy across annotators.
The foundation of any successful speech data annotation project lies in the comprehensive training and development of detailed guidelines for annotators. This is especially critical when dealing with African languages, which are characterised by rich linguistic diversity and complex nuances. Training sessions must be meticulously designed to cover not only the linguistic aspects of the languages being annotated but also the cultural contexts in which these languages are used.
This involves a curriculum that encompasses phonetics, grammar, syntax, and the socio-linguistic nuances that could affect the meaning of speech. Effective training ensures that annotators are well-equipped to handle the intricacies of the languages they work with, leading to annotations that accurately reflect the intended meanings and subtleties of speech.
Beyond the initial training, detailed annotation guidelines serve as a vital reference for annotators, providing clear standards and examples for handling various linguistic and technical scenarios they may encounter. These guidelines must be continually updated to reflect new insights, challenges, and solutions discovered during the annotation process.
They should include protocols for dealing with ambiguous cases, managing dialectal variations, and ensuring the consistent application of annotation rules. By establishing a strong framework of training and guidelines, projects can achieve a high degree of consistency and accuracy across annotators, which is crucial for the development of reliable and effective speech recognition technologies.
#7 Iterative Quality Control Processes
Implementing rigorous and iterative quality checks can help identify and correct errors, ensuring the high quality of the annotated dataset.
The dynamic nature of speech and the complexities inherent in African languages necessitate rigorous and iterative quality control processes to ensure the high quality of annotated datasets. Implementing a multi-tiered review system, where annotations are checked and rechecked at several stages, can help identify and correct errors that might have been overlooked initially.
This iterative approach allows for continuous improvement of the dataset’s accuracy and reliability, as each review cycle uncovers new insights and opportunities for refinement. Quality control should not be seen solely as a means of error correction but also as an integral part of the annotation process that contributes to the ongoing training and development of annotators.
In addition to internal review processes, engaging external experts for periodic audits of the dataset can provide an additional layer of validation. These experts, who may bring fresh perspectives and specialised knowledge, can help identify systemic issues or biases that internal reviewers might miss. Moreover, leveraging advanced technologies such as machine learning algorithms can complement human efforts by flagging inconsistencies or anomalies in the data for further review. Through a comprehensive and iterative approach to quality control, projects can ensure that their annotated datasets meet the highest standards of accuracy and reliability, paving the way for the development of superior AI applications.
#8 Ethical Considerations
Ethical practices, including respect for privacy and consent in data collection, are paramount, especially in languages spoken by vulnerable or underrepresented communities.
Ethical considerations are paramount in the collection and annotation of speech data, especially for languages spoken by vulnerable or underrepresented communities. Respecting the privacy and consent of individuals whose speech data is being collected involves clear communication about the purpose of the data collection, how the data will be used, and the measures in place to protect their privacy.
This is particularly important in communities where there may be sensitivities around language use or concerns about the potential misuse of data. Ensuring informed consent and maintaining transparency throughout the process are fundamental ethical principles that must be upheld to build trust and foster willing participation among speech data contributors.
Moreover, ethical considerations extend to the treatment and compensation of annotators, who play a critical role in the development of speech recognition technologies. Providing fair compensation and working conditions reflects the value of their contributions and supports the sustainability of high-quality annotation work.
Additionally, ethical practices should guide decisions about the use and sharing of annotated datasets, ensuring that they do not perpetuate biases or harm to the communities represented in the data. By prioritising ethical considerations at every stage of the speech data annotation process, projects can ensure that their work contributes positively to the advancement of AI technologies in a manner that is respectful and beneficial to all stakeholders.
#9 Technological Challenges and Solutions
Addressing technical challenges such as low-resource languages through innovative solutions like semi-supervised learning models or transfer learning.
Addressing the technological challenges presented by low-resource languages requires innovative approaches that leverage the latest advancements in machine learning and artificial intelligence. Many African languages fall into the category of low-resource languages, which means they lack the extensive datasets that are typically available for more widely spoken languages.
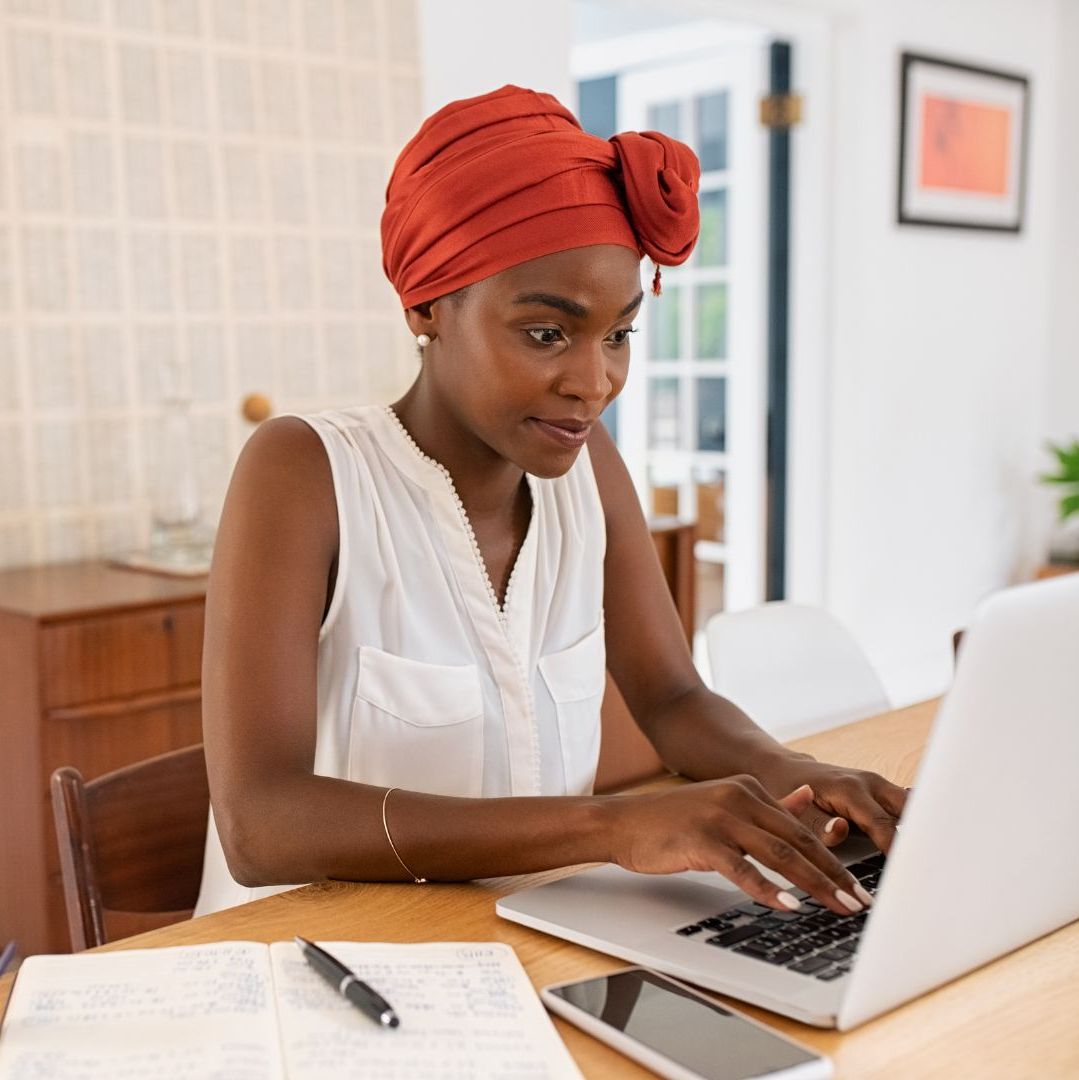
This scarcity of data poses significant challenges for training effective speech recognition models. Innovative solutions such as semi-supervised learning models and transfer learning have emerged as powerful tools to overcome these hurdles. Semi-supervised learning, for instance, allows for the utilisation of both labelled and unlabelled data, maximising the available resources and enhancing the model’s ability to learn from limited datasets. Transfer learning, on the other hand, leverages knowledge gained from working with high-resource languages to improve performance on low-resource languages, offering a shortcut to developing robust models without the need for extensive datasets.
These technological solutions not only address the immediate challenges of working with low-resource languages but also open up new possibilities for the inclusion of African languages in AI applications. By adapting and innovating in response to the unique challenges presented by these languages, researchers and developers can create speech recognition technologies that are more accessible, accurate, and relevant to speakers of African languages. This not only advances the field of AI but also contributes to the preservation and promotion of linguistic diversity on the continent.
#10 Community Engagement and Feedback
Involving the speech community in the annotation process can provide valuable insights and enhance the authenticity and relevance of the annotated data.
The involvement of the speech community in the annotation process is crucial for ensuring the authenticity and relevance of the annotated data. Engaging with community members not only provides access to a rich source of linguistic and cultural knowledge but also fosters a collaborative environment where the nuances of the language can be fully understood and appreciated.
This engagement can take many forms, from participatory workshops to gather feedback on annotation guidelines to involving community members as annotators or reviewers. Such collaboration allows for the annotation process to be informed by the lived experiences and insights of native speakers, ensuring that the annotated data accurately reflects the way the language is used in daily life.
Moreover, community feedback serves as a valuable mechanism for continuous improvement of the annotation process and the technologies developed from the annotated data. By listening to and incorporating feedback from community members, projects can identify areas for improvement, uncover biases in the data, and develop more inclusive and effective AI applications. This feedback loop not only enhances the quality of the speech data but also builds trust and rapport with the community, ensuring their continued engagement and support.
Through active community engagement and responsive feedback mechanisms, projects can create annotated datasets that truly represent the linguistic diversity and richness of African languages, contributing to the development of AI technologies that serve the needs and aspirations of their speakers.
Key Tips For Speech Data Annotation
- Understand the linguistic and cultural intricacies of each African language being annotated.
- Ensure high-quality audio data collection with minimal background noise.
- Employ expert annotators and linguists who are native speakers.
- Choose annotation tools and software that cater to the specific needs of African languages.
- Provide comprehensive training and detailed guidelines to maintain annotation consistency.
- Implement iterative quality control processes to ensure data accuracy.
- Adhere to ethical considerations, including privacy and consent.
- Tackle technological challenges with innovative solutions.
- Engage with the speech community for valuable insights and feedback.
Way With Words provides highly customised and appropriate speech data collections for African languages, catering to the unique needs of technologies that target these languages. Our services include creating custom speech datasets with transcripts for machine learning purposes, essential for improving existing automatic speech recognition models (ASR) using natural language processing (NLP). Additionally, our machine transcription polishing (MTP) service enhances the quality of machine transcripts, supporting a wide range of AI and machine learning applications intended for various African languages.
Speech data annotation in African languages is a complex task that requires careful consideration of linguistic diversity, cultural nuances, and technological challenges. The best practices outlined in this article emphasise the importance of understanding the unique features of each language, employing expert annotators, and utilising appropriate tools and methodologies. By following these guidelines, researchers and developers can create high-quality datasets that significantly enhance the performance of AI technologies in understanding and processing African languages.
Moreover, ethical considerations and community engagement play a crucial role in ensuring the data’s relevance and respect for the speech communities involved. Ultimately, the successful annotation of speech data in African languages will pave the way for more inclusive and effective AI technologies that serve the needs of diverse populations across the African continent.
Speech Data Annotation Resources
African Language Speech Collection Solution: Way With Words – We create custom speech datasets for African languages, including transcripts for machine learning purposes. Our service is used for technologies looking to create or improve existing automatic speech recognition models (ASR) using natural language processing (NLP) for select African languages and various domains.
Machine Transcription Polishing of Captured Speech Data: Way With Words – We polish machine transcripts for clients across a number of different technologies. Our machine transcription polishing (MTP) service is used for a variety of AI and machine learning purposes that are intended to be applied in various African languages.
Data Collection for Annotation: Tips, Tricks, and Best Practices: Data collection is an essential step in the process of machine learning, and when it comes to data annotation, it becomes even more critical. Data annotation involves the process of labeling data to make it more understandable for machine learning algorithms. Annotation is essential in various fields such as object detection, speech recognition, and natural language processing, to name a few. The quality of annotation data directly affects the accuracy and efficiency of machine learning models. Therefore, it is essential to follow best practices while collecting data for annotation.