Unveiling Speech Data Collection: The Backbone of Modern AI
Introduction to Speech Data Collection
Speech Data Collection is an essential process that underpins advancements in artificial intelligence (AI) and machine learning (ML). By gathering vast amounts of speech data, researchers and developers can train AI systems to understand and respond to human language more effectively. This short guide aims to provide a comprehensive overview of speech data collection, its significance, techniques, challenges, and real-world applications.
In exploring speech data collection, several common questions arise:
- What is speech data collection?
- Why is speech data collection important for AI and ML?
- What are the main techniques and tools used in speech data collection?
Speech Data Applications & Collections – 10 Key Ingredients
What is Speech Data Collection?
Speech data collection involves gathering audio recordings of human speech for the purpose of training and improving AI systems. These datasets can vary in size, complexity, and content, depending on the specific requirements of the project. Speech data is critical for developing AI applications such as voice assistants, transcription services, and language translation tools.
Speech data collection is the process of gathering audio recordings of human speech for the purpose of training and improving AI systems. This involves creating a repository of speech samples that can be used to teach machines how to understand and interpret human language. These datasets can vary widely in terms of size, complexity, and content, depending on the specific requirements of the project. For instance, a project aimed at developing a voice assistant might need diverse speech samples from various demographics, accents, and speaking styles to ensure the system can understand a wide range of users.
The types of speech data collected can be categorised into several forms, including spontaneous speech, scripted speech, read speech, and conversational interactions. Spontaneous speech captures natural, unplanned dialogue, often rich in hesitations, interruptions, and informal language, making it highly valuable for realistic AI training. Scripted speech, on the other hand, involves pre-written text read aloud by participants, which can be useful for ensuring coverage of specific linguistic constructs or scenarios. Read speech, such as reading a passage from a book, provides clear and structured language, which is easier for initial model training.
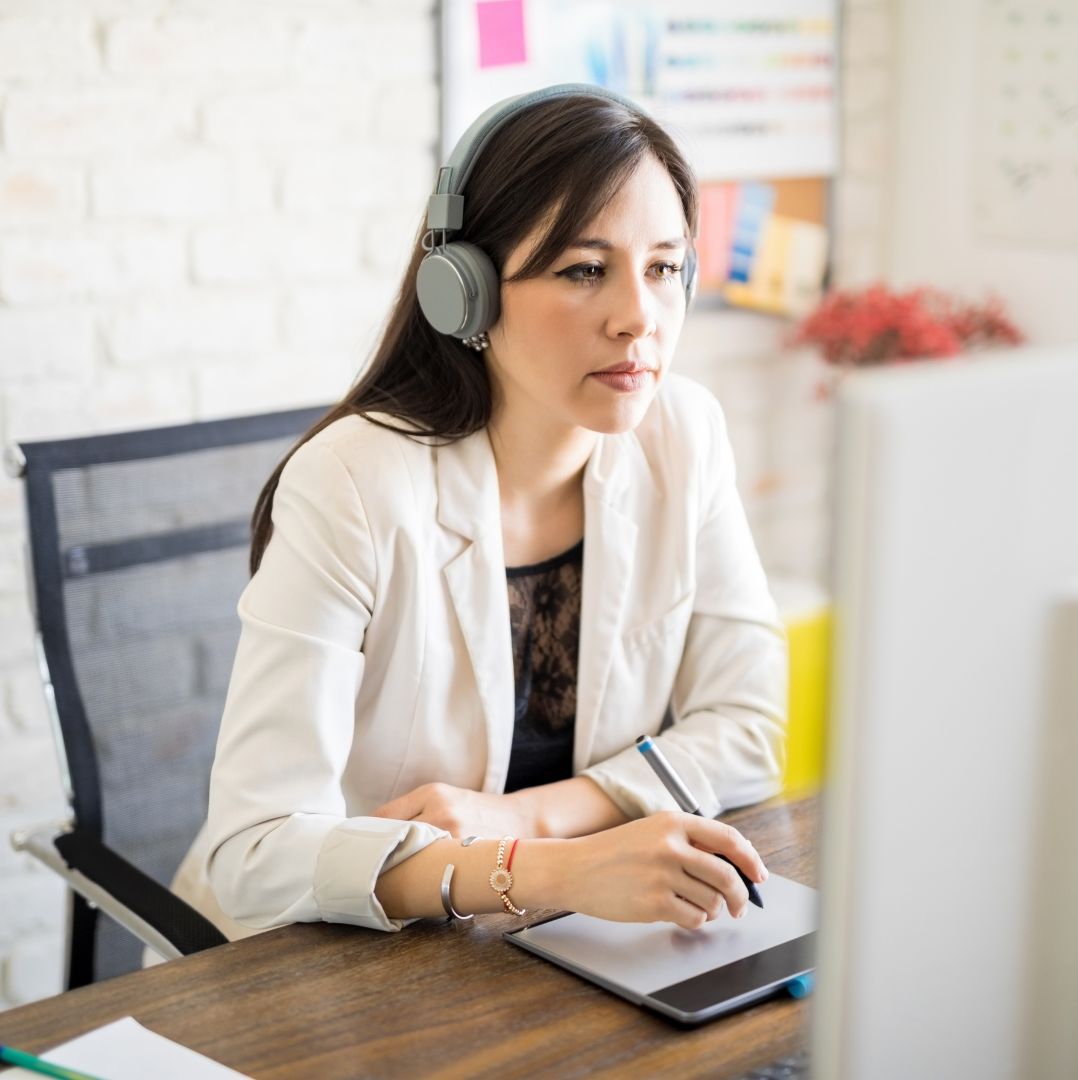
Moreover, speech data collection often requires meticulous planning and execution to ensure data quality and relevance. This includes selecting appropriate recording environments, using high-quality recording equipment, and employing standardised protocols for data collection.
The collected data is then typically annotated with various labels, such as speaker identity, emotional tone, and background noise, to provide additional context for AI training. This rich metadata helps AI systems learn not just the words being spoken, but also the nuances of human communication, including intonation, emotion, and context.
Importance of Speech Data in AI and ML
Speech data is vital for training AI and ML models to accurately recognise and process human language. The quality and diversity of the collected speech data directly impact the performance of these models. High-quality speech data enables AI systems to understand different accents, dialects, and speaking styles, making them more robust and versatile.
Speech data is a cornerstone in the training of AI and ML models for language processing. The ability of AI systems to accurately recognise and process human language hinges on the quality and diversity of the speech data they are trained on. High-quality speech data enables these systems to understand different accents, dialects, and speaking styles, which is crucial for developing robust and versatile AI applications. Without diverse and high-quality speech data, AI systems can become biased or ineffective in real-world scenarios where speech variability is the norm.
The impact of speech data on AI and ML can be seen in various applications. For instance, in voice assistants like Siri or Alexa, the accuracy of responses and the ability to understand user commands directly correlate with the diversity and quality of the underlying speech data. Similarly, in automatic transcription services, high-quality speech data ensures that the transcriptions are accurate and reliable, even in noisy environments or with speakers who have strong accents. Language translation tools also benefit from rich speech datasets, allowing them to accurately convert spoken language from one language to another, preserving meaning and context.
Furthermore, the importance of speech data extends to improving accessibility and inclusivity in technology. By training AI systems on diverse speech data, developers can create applications that cater to users with different linguistic backgrounds, speech impairments, or atypical speaking patterns. This not only enhances the user experience but also democratises access to technology, ensuring that it serves a broader population. In summary, the importance of speech data in AI and ML cannot be overstated, as it fundamentally determines the effectiveness, accuracy, and inclusivity of language-based AI applications.
Techniques and Tools for Collecting Speech Data
There are several methods and tools available for collecting speech data:
- Crowdsourcing: Using platforms like Amazon Mechanical Turk to gather speech samples from a diverse group of participants.
- Field Recording: Capturing speech data in various environments to ensure a wide range of audio conditions.
- Synthetic Data Generation: Creating artificial speech data using text-to-speech technologies.
These techniques, along with specialised software tools, help in accumulating large volumes of speech data efficiently.
Collecting speech data involves a variety of techniques and tools to ensure the data is comprehensive and of high quality. One common method is crowdsourcing, where platforms like Amazon Mechanical Turk are used to gather speech samples from a diverse group of participants. This approach allows for the collection of large datasets quickly and cost-effectively, while also ensuring a wide demographic representation. Crowdsourcing can be particularly useful for obtaining spontaneous and natural speech samples, as participants can record themselves in their everyday environments.
Field recording is another technique used in speech data collection. This method involves capturing speech data in various real-world environments to ensure a wide range of audio conditions are represented. Field recording can provide valuable insights into how speech is affected by different background noises, acoustics, and speaking contexts. For example, collecting data in noisy urban environments, quiet rural settings, and different indoor spaces can help train AI systems to perform well under diverse acoustic conditions. High-quality microphones and portable recording devices are typically used in field recording to capture clear and accurate speech samples. Way With Words provides solutions for both models.
Synthetic data generation is an emerging technique in speech data collection, leveraging text-to-speech (TTS) technologies to create artificial speech data. This method can supplement real speech data, providing additional training examples for AI models. Synthetic data can be particularly useful for generating rare or hard-to-collect speech samples, such as specific accents, rare languages, or particular speech patterns.
However, it is important to combine synthetic data with real human speech data to ensure the AI models can generalise well to natural speech. Advanced software tools and platforms, such as Kaldi and Mozilla’s Common Voice, are often used to streamline the data collection process, providing frameworks for recording, annotating, and managing speech datasets efficiently.
Challenges in Speech Data Collection
Collecting speech data comes with its own set of challenges, including:
- Privacy and Consent: Ensuring that participants provide informed consent and that their data is handled securely.
- Diversity: Gathering speech data from a broad demographic to avoid biases in AI models.
- Quality Control: Maintaining high standards in audio quality and transcription accuracy.
Addressing these challenges is crucial for the success of any speech data collection project.
Collecting speech data comes with its own set of challenges that need to be carefully managed to ensure the success of AI projects. One of the primary challenges is ensuring privacy and obtaining informed consent from participants. Speech data can contain sensitive information, and it is crucial to handle this data responsibly. Participants must be fully aware of how their data will be used, and their consent must be explicitly obtained. This includes explaining the purpose of the data collection, how the data will be stored, and who will have access to it. Implementing robust data security measures is also essential to protect the collected data from unauthorised access and breaches.
Diversity in speech data collection is another significant challenge. To develop AI models that are inclusive and free from bias, it is important to gather speech data from a broad demographic. This includes different age groups, genders, ethnicities, and socioeconomic backgrounds. Collecting such diverse data ensures that AI systems can understand and respond accurately to a wide range of voices and speaking styles. However, achieving this diversity can be difficult, especially when certain groups are underrepresented in the data collection process. Active efforts are required to recruit participants from diverse backgrounds and to ensure that the collected data accurately reflects the target population.
Quality control is a critical aspect of speech data collection. Ensuring high standards in audio quality and transcription accuracy is essential for training effective AI models. Poor quality recordings, such as those with background noise, low volume, or unclear speech, can degrade the performance of AI systems. Similarly, inaccurate transcriptions can lead to incorrect training data, resulting in AI models that fail to understand or process language correctly. Implementing rigorous quality control measures, such as using high-quality recording equipment, standardising recording protocols, and employing skilled transcribers, is crucial to maintain the integrity of the collected data.
Case Studies of Successful Speech Data Collection Projects
Several organisations have successfully implemented speech data collection projects. For instance:
- Google’s Speech Recognition: Google’s extensive data collection efforts have significantly improved the accuracy of their voice recognition systems.
- Microsoft’s Conversational AI: Microsoft uses vast datasets to train their conversational AI, resulting in highly responsive and context-aware systems.
These case studies highlight the importance of comprehensive and well-executed speech data collection initiatives.
Several organisations have successfully implemented speech data collection projects, demonstrating the value of comprehensive and well-executed initiatives. For instance, Google’s speech recognition system has seen significant improvements in accuracy due to extensive data collection efforts. Google has invested in collecting diverse speech samples from around the world, ensuring their voice recognition technology can understand various accents, dialects, and languages. This extensive data collection has enabled Google to develop a highly accurate and robust speech recognition system that is used in various applications, from voice search to real-time translation services.
Another notable case is Microsoft’s conversational AI, which utilises vast datasets to train its systems. Microsoft has focused on collecting high-quality speech data to develop conversational agents that are context-aware and highly responsive. By gathering speech data from diverse sources and environments, Microsoft has been able to create AI systems that can handle complex interactions, understand user intent, and provide relevant responses. This has been particularly beneficial in applications such as customer service chatbots and virtual assistants, where accurate and contextually appropriate responses are crucial for a positive user experience.
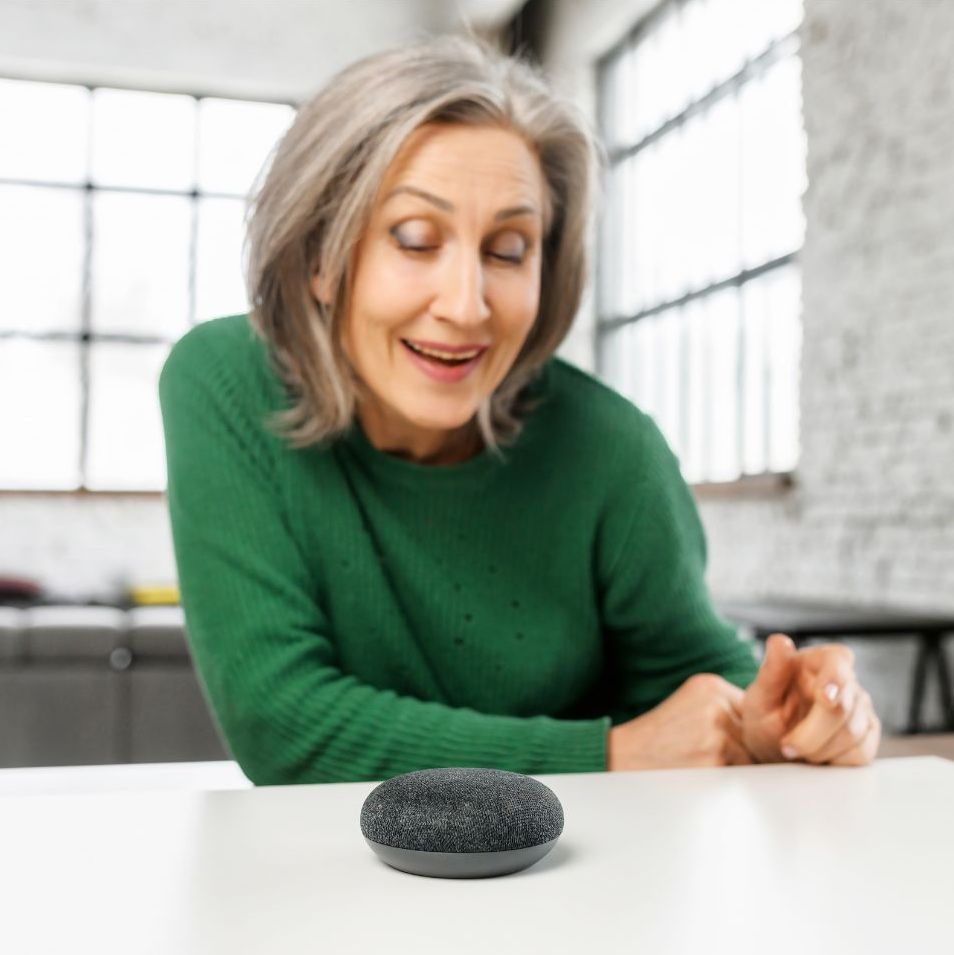
Amazon’s Alexa is another example of successful speech data collection. Amazon has continuously collected and analysed speech data to improve Alexa’s ability to understand and respond to user commands.
By leveraging data from millions of interactions, Amazon has refined Alexa’s natural language processing capabilities, enabling the assistant to perform a wide range of tasks, from controlling smart home devices to providing information and entertainment. The success of these projects highlights the importance of robust data collection strategies and the impact they can have on the development of advanced AI systems.
Key Factors in Successful Speech Data Collection
Successful speech data collection hinges on several key factors, including:
- Participant Recruitment: Ensuring a diverse and representative pool of participants.
- Data Annotation: Accurately labelling speech data to facilitate effective training of AI models.
- Continuous Improvement: Regularly updating and refining speech datasets to keep pace with evolving linguistic trends.
Successful speech data collection hinges on several key factors, each contributing to the overall quality and utility of the collected data. Participant recruitment is one of the most crucial aspects, as it determines the diversity and representativeness of the dataset. Ensuring a diverse and representative pool of participants involves reaching out to different demographics, including various age groups, ethnicities, genders, and linguistic backgrounds. This diversity helps in creating AI models that are inclusive and capable of understanding and processing a wide range of speech patterns.
Data annotation is another critical factor in successful speech data collection. Accurate labelling of speech data, such as tagging speaker identity, emotional tone, and background noise, provides valuable context for training AI models. High-quality annotations enable AI systems to learn the nuances of human speech, improving their ability to recognise and respond accurately. Employing skilled annotators and using advanced annotation tools can enhance the accuracy and consistency of the labeled data, ensuring that the AI models are trained on reliable and comprehensive datasets.
Continuous improvement is essential to keep pace with evolving linguistic trends and technological advancements. Regularly updating and refining speech datasets ensures that AI models remain relevant and effective in real-world applications. This involves collecting new speech data, re-evaluating existing datasets, and incorporating feedback from AI system performance. By maintaining an iterative approach to speech data collection, organisations can address emerging challenges, adapt to changing user needs, and leverage the latest technological innovations to enhance the quality and utility of their AI models.
Legal and Ethical Considerations
When collecting speech data, it is essential to navigate legal and ethical considerations. This includes ensuring compliance with data protection regulations, obtaining explicit consent from participants, and implementing measures to safeguard their privacy.
Navigating legal and ethical considerations is crucial in speech data collection. Compliance with data protection regulations, such as the General Data Protection Regulation (GDPR) in Europe, is essential to ensure that the collection, storage, and use of speech data are conducted legally. These regulations mandate strict guidelines on obtaining consent, data anonymisation, and providing participants with the right to access, correct, or delete their data. Organisations must implement robust data governance frameworks to ensure compliance and avoid legal repercussions.
Ethical considerations go beyond legal compliance, focusing on the responsible and fair treatment of participants. This includes obtaining explicit consent, being transparent about the purpose of the data collection, and ensuring that participants are not exploited or misled. It is also important to consider the potential biases that can arise from the collected data. For instance, if the dataset predominantly features voices from certain demographics, the resulting AI models may perform poorly for underrepresented groups. Addressing these biases requires proactive efforts to ensure diversity and fairness in data collection.
Additionally, safeguarding the privacy of participants is paramount. Speech data can contain personal and sensitive information, and it is the responsibility of the collecting organisation to implement measures to protect this data. This includes secure storage solutions, encryption, and access controls to prevent unauthorised use or data breaches. Ethical guidelines, such as those provided by the Association for Computing Machinery (ACM) and the Institute of Electrical and Electronics Engineers (IEEE), offer valuable frameworks for conducting ethical speech data collection, emphasising respect for participant autonomy, beneficence, and justice.
Speech Data Applications
Speech data applications are vast and varied, ranging from voice-activated assistants and customer service chatbots to advanced transcription services and language learning apps. These applications leverage speech data to provide seamless and interactive user experiences.
Speech data applications are diverse and span across various industries, leveraging the power of AI to enhance user experiences and streamline operations. One of the most prominent applications is in voice-activated assistants, such as Apple’s Siri, Amazon’s Alexa, and Google Assistant. These assistants rely on vast amounts of speech data to understand and respond to user commands, enabling hands-free operation of devices, smart home management, and access to information and services. The ability to accurately recognise and process natural language is key to the effectiveness of these voice-activated systems.
Customer service chatbots are another significant application of speech data. By integrating speech recognition and natural language processing capabilities, these chatbots can interact with customers in a conversational manner, providing assistance, answering queries, and resolving issues. This not only improves customer satisfaction but also reduces the workload on human agents, allowing them to focus on more complex tasks. Advanced transcription services also benefit from speech data, converting spoken language into written text for various purposes, such as creating meeting minutes, generating subtitles for videos, and assisting individuals with hearing impairments.
In the realm of education, speech data applications include language learning apps that use AI to help users practice pronunciation, learn new vocabulary, and improve their conversational skills. These apps leverage speech data to provide personalised feedback and adaptive learning experiences, making language acquisition more effective and engaging. Additionally, speech data is used in developing assistive technologies for individuals with disabilities, such as speech-to-text software for those with hearing impairments and voice-controlled devices for individuals with mobility challenges. These applications demonstrate the broad impact of speech data on enhancing accessibility, efficiency, and user engagement across various domains.
Advances in Speech Data Technology
The field of speech data collection is continuously evolving, with advancements in technology making the process more efficient. Innovations such as automated speech recognition (ASR) systems and improved recording devices contribute to higher quality datasets.
The field of speech data collection is continuously evolving, with advancements in technology making the process more efficient and effective. One significant innovation is the development of automated speech recognition (ASR) systems, which can transcribe spoken language into text with high accuracy. ASR technology has greatly improved over the years, benefiting from deep learning algorithms and large speech datasets. This has enabled the creation of more reliable and accurate transcription services, voice assistants, and real-time translation tools.
Another advancement is in the area of recording devices and software. Modern recording equipment, such as high-fidelity microphones and portable recorders, allows for the capture of clear and high-quality speech data in various environments. Additionally, software tools for managing and annotating speech data have become more sophisticated, providing functionalities such as automated tagging, noise reduction, and quality assessment. These tools streamline the data collection process, making it easier to gather, organise, and analyse large volumes of speech data.
The use of synthetic data generation is also gaining traction, providing a complementary approach to traditional data collection methods. Text-to-speech (TTS) technologies have advanced to the point where they can produce highly realistic speech samples that can be used to augment real-world datasets.
This is particularly useful for generating speech data in rare languages, specific dialects, or underrepresented speaking styles. By combining synthetic data with real speech samples, AI models can be trained more effectively, ensuring they perform well across a wide range of scenarios.
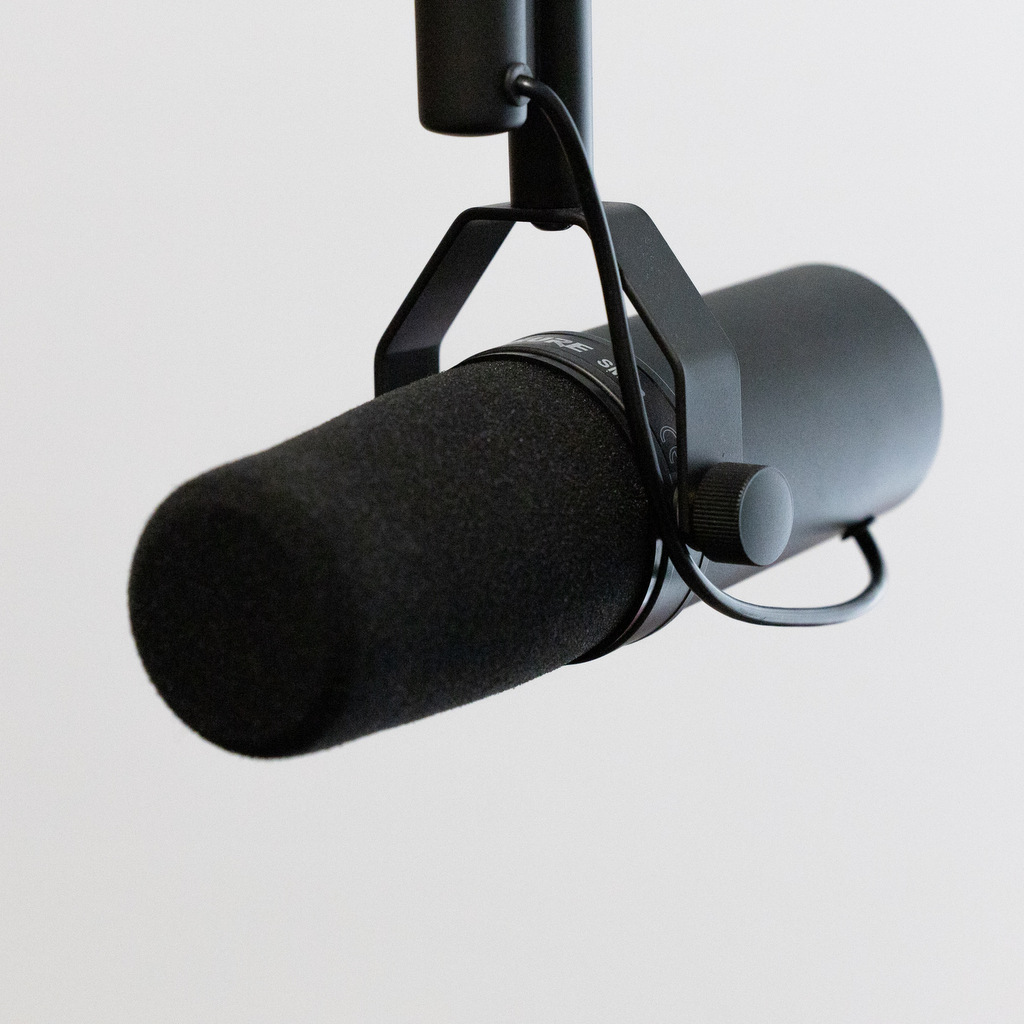
The Future of Speech Data Collection
The future of speech data collection looks promising, with ongoing research focusing on enhancing data collection techniques, improving data diversity, and addressing privacy concerns. As AI continues to advance, the demand for high-quality speech data will only increase.
The future of speech data collection looks promising, with ongoing research and innovation poised to enhance the techniques and tools used in this field. One area of focus is improving the diversity and inclusivity of speech datasets. Researchers are exploring new methods to recruit participants from underrepresented groups, ensuring that AI models are trained on data that reflects the full spectrum of human speech. This includes collecting data from different age groups, ethnicities, genders, and socio-economic backgrounds to reduce biases and improve the accuracy and fairness of AI systems.
Another promising direction is the integration of privacy-preserving technologies in speech data collection. Techniques such as differential privacy and federated learning are being explored to protect participant data while still enabling the training of robust AI models. Differential privacy adds noise to the data in a way that preserves individual privacy while maintaining the overall utility of the dataset. Federated learning, on the other hand, allows AI models to be trained across multiple decentralised devices without the need to transfer raw data to a central server, enhancing data security and privacy.
Advancements in AI and machine learning algorithms will also drive the future of speech data collection. Improved models for automated transcription, speaker identification, and emotion detection will enable more nuanced and accurate analysis of speech data. Additionally, the development of real-time speech processing technologies will open up new applications and use cases, such as live translation and interactive AI systems that can engage in natural, fluid conversations with users. As these technologies continue to evolve, the demand for high-quality speech data will only increase, driving further innovation and research in this critical area.
Key Tips on Speech Data
- Ensure Diversity: Collect speech data from a wide range of demographics to avoid biases.
- Prioritise Privacy: Implement stringent privacy measures to protect participant data.
- Quality Control: Maintain high standards in audio quality and transcription accuracy.
- Use Advanced Tools: Leverage the latest technologies and tools for efficient data collection.
- Stay Compliant: Adhere to legal and ethical guidelines to ensure responsible data collection.
Speech data collection is a critical component in the development of modern AI systems. By understanding the importance, techniques, and challenges involved, AI developers, machine learning engineers, data scientists, technology researchers, and educational institutions can effectively contribute to advancing this field. The future of AI and ML depends on the continuous collection and refinement of high-quality speech data, ensuring that these systems become more accurate, inclusive, and responsive.
As we move forward, it is essential to keep privacy and diversity at the forefront of speech data collection efforts. By doing so, we can create AI systems that truly understand and respond to the nuances of human language.
Further Resources
Wikipedia: Speech Recognition
This article provides an overview of speech recognition, its history, technologies, and applications in various fields, offering foundational knowledge for understanding speech data collection.
Way With Words: Speech Collection
Way With Words specialises in creating comprehensive speech datasets, including transcripts for machine learning purposes. This service supports the development of advanced automatic speech recognition models using natural language processing for specific languages and various domains. Each dataset can be tailored to specific dialects, demographics, or other required conditions.