Transforming Customer Service with Speech Data: Automation & Efficiency
What is the Impact of Automation on Speech Data Customer Service?
Customer service is undergoing a significant transformation. As operational demands grow and customer expectations shift, businesses are increasingly turning to automated technologies to enhance response times, reduce operational costs, and elevate customer experience. At the centre of this movement is speech data—a vast resource that, when harnessed correctly, can drastically improve the quality, speed, and consistency of customer support services.
Speech data refers to recorded spoken interactions, which, once processed, become powerful sources of information. These datasets offer real-time insights into customer needs, common queries, recurring problems, and overall sentiment. They allow companies to not only react to customer behaviour but also to anticipate and adapt proactively. This shift is more than a technical upgrade; it represents a fundamental change in how organisations understand and serve their customers.
However, with this opportunity comes complexity. Many businesses ask important questions when considering speech data-driven automation:
- How does speech data actually improve customer service workflows?
- What technologies are enabling the automation of voice-based customer support?
- What are the risks or limitations of automating customer service with speech data?
This short guide answers these questions and more. We examine the core role of speech data in automated customer service systems, explore enabling technologies, assess real-world case studies, and discuss potential risks and future directions. With speech data becoming increasingly integral to AI development, this is a time where businesses must decide whether to lead the change—or be left behind.
Speech Data for Customer Service
1. The Role of Speech Data in Customer Service Automation
Speech data, when captured and processed effectively, offers businesses a detailed look into customer behaviour, expectations, and pain points. It is not merely about transcribing phone calls—it’s about extracting usable intelligence from every interaction. The application of speech data customer service systems enables organisations to track sentiment trends, identify frequently asked questions, and highlight service gaps that might otherwise go unnoticed.
Natural language processing (NLP), in combination with speech recognition tools, facilitates the real-time transcription and classification of speech. The structured data that results from this process feeds directly into automated systems, informing response logic, routing decisions, and predictive analytics. Businesses that adopt such systems quickly notice an improvement in call handling quality and efficiency.
Key benefits include:
- Shorter resolution times: By understanding intent faster, systems resolve queries more quickly.
- Improved personalisation: Speech data reveals patterns in user behaviour, allowing for dynamic interaction.
- Operational consistency: Automation reduces variability in responses.
- Training optimisation: Real interactions inform better agent training protocols.
An international telco, for example, used speech analytics to improve churn prediction models. By flagging early signs of customer dissatisfaction—such as changes in tone, increased use of negative keywords, or frequent escalations—the company significantly reduced churn by over 25% in under a year.
Importantly, speech data doesn’t seek to replace human agents. Rather, it enhances their role—freeing them from repetitive tasks and empowering them to focus on higher-value interactions. This hybrid model is proving more effective than purely human or fully automated systems alone.
2. Technologies Driving Automation with Speech Data
Behind every automated speech data solution lies a combination of advanced technologies. These tools not only gather and process speech, but also learn from it—constantly improving how they handle customer service tasks.
Key technologies include:
- Automatic Speech Recognition (ASR): Converts live or recorded speech into structured text.
- Natural Language Understanding (NLU): Interprets the intent and context behind the speech.
- Text-to-Speech (TTS): Creates realistic spoken responses from machine-generated outputs.
- Conversational AI Frameworks: Drive multi-step, dialogue-based customer interactions.
- Sentiment and Emotion Detection: Evaluates vocal tone, stress, and emotional indicators.
These technologies operate in tandem. For instance, a virtual agent might use ASR to capture what a customer says, NLU to determine what they mean, and a knowledge base to craft a suitable reply. If the customer sounds frustrated, sentiment detection can flag the interaction for human escalation.
In a survey by McKinsey in late 2023, 68% of customer service leaders said they planned to implement voice-driven AI assistants within 18 months. Among those already using such tools, 79% reported significant improvements in customer satisfaction and handling times.
With improvements in computational power, speech models are becoming more efficient, less prone to bias, and more accessible for mid-sized businesses—not just large enterprises.
3. Case Studies on Successful Implementations
The theory behind speech data automation becomes compelling when we examine its real-world applications. Several industry leaders across different sectors have reported measurable successes after implementing speech data technologies.
- Retail Sector: A multinational fashion retailer integrated a speech analytics dashboard into its customer support centre. Within four months, they reduced average call handling time by 15% and improved CSAT scores by 12 points, thanks to better routing and sentiment detection.
- Healthcare Providers: A regional health network implemented automated voice response systems for after-hours triage and appointment scheduling. Their system handled over 10,000 calls per week and resulted in a 40% reduction in on-call staff requirements.
- Banking Industry: A major bank deployed a fraud-detection tool that monitored real-time speech patterns. When tone, pace, or word choice deviated from the customer’s normal profile, the system flagged the interaction for further review. This reduced fraud losses by over £2 million in the first year.
Such case studies confirm the tangible business value of applying speech data to customer service automation—cutting costs, improving service metrics, and reducing human error.
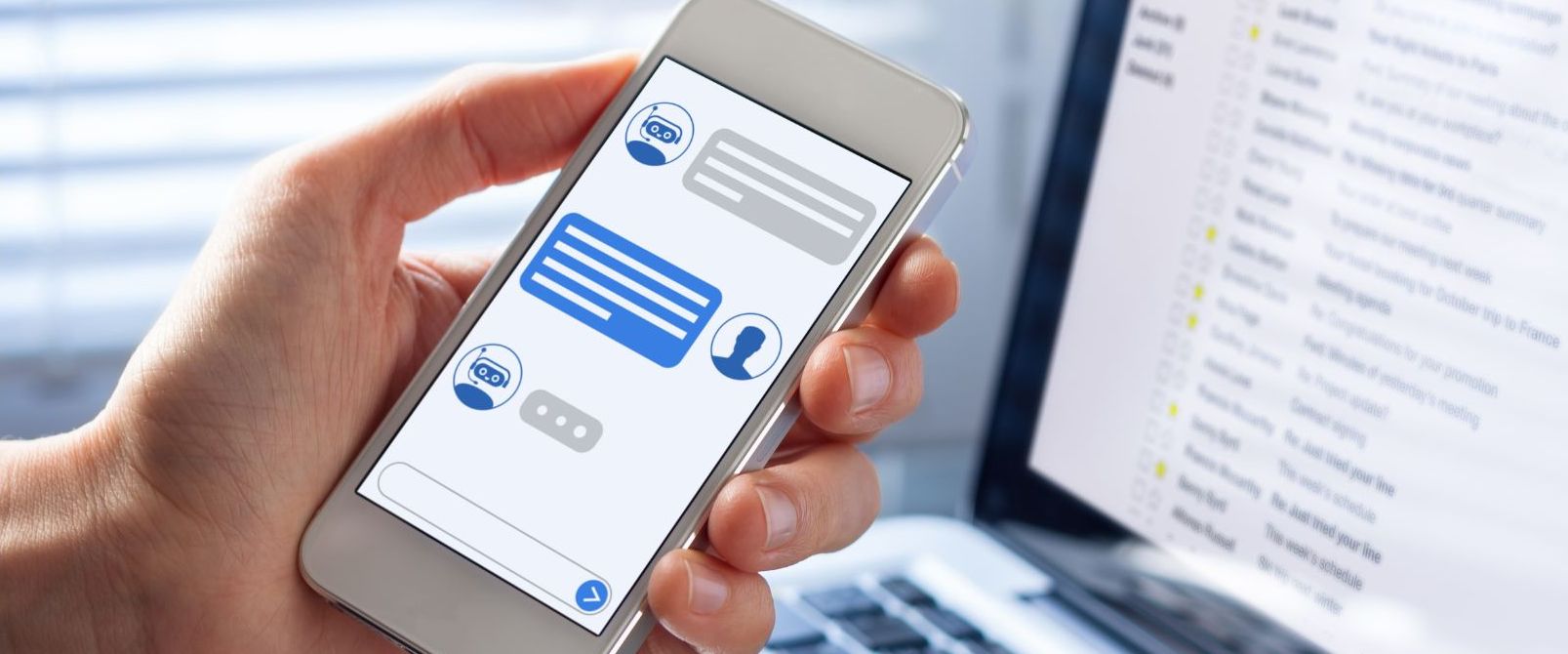
4. Personalisation and Sentiment Analysis
One of the more nuanced benefits of speech data customer service solutions is enhanced personalisation. By analysing tone, word choice, speech pace, and emotional signals, companies can tailor responses in a way that feels more human—despite being machine-driven.
Personalisation in automated environments involves more than using a customer’s name. Systems can:
- Adjust responses based on mood or stress levels.
- Reference past interactions or preferences.
- Prioritise support for high-value customers.
Sentiment analysis plays a key role in this process. Tools such as IBM Watson and Amazon Comprehend allow businesses to measure sentiment across thousands of interactions daily. These insights are then used to tweak bot scripts, improve escalation rules, and even reconfigure user interfaces.
According to Capgemini Research Institute, 77% of consumers are more loyal to brands that offer tailored experiences, and speech personalisation tools have become central to delivering on that expectation. With sentiment cues embedded in automated processes, systems are able to act with sensitivity, even when no human is directly involved.
5. Benefits of Speech Data-Driven Automation
Beyond personalisation, the benefits of speech data automation are substantial and quantifiable. Implementing these systems can lead to improvements across multiple operational areas:
- Scalability: Systems can scale to manage millions of interactions simultaneously without needing additional staff.
- 24/7 Availability: AI solutions do not require breaks, holidays, or downtime.
- Standardisation: Removes inconsistency in agent responses and ensures regulatory compliance.
- Cost Reduction: Automated handling of repetitive queries reduces staffing and operational overhead.
- Business Intelligence: Provides ongoing data that feeds into product development, marketing, and strategic planning.
A mid-sized software company reported a 50% reduction in support tickets by deploying a voice-activated assistant trained on speech data from previous calls. Not only were calls handled more efficiently, but customer feedback also improved due to faster resolution and fewer transfers.
6. Challenges and Limitations
Despite its advantages, speech data automation brings certain challenges that must be addressed for a successful deployment:
- Accuracy and Clarity: Accents, dialects, background noise, and speech impediments can affect recognition accuracy.
- Privacy and Consent: Businesses must comply with laws such as GDPR, CCPA, and POPIA when storing and processing speech data.
- Algorithmic Bias: Poor training datasets can lead to biased outputs and misinterpretations.
- Infrastructure Costs: Setting up real-time speech analytics requires significant initial investment.
- Integration Hurdles: Existing customer systems (e.g., CRMs) may need custom development to align with voice tech.
Mitigating these risks involves strong governance, continuous testing, and hybrid models that allow human override where needed. Organisations that maintain a transparent approach tend to gain more customer trust in the process.
7. Compliance and Ethical Use of Speech Data
The ethical use of speech data in customer service automation is not optional—it is critical. Companies must adhere to clear ethical frameworks and legal standards to avoid reputational damage and legal repercussions.
Responsible speech data practices include:
- Providing clear disclaimers about call monitoring.
- Gaining informed customer consent.
- Ensuring secure data storage and restricted access.
- Allowing customers to opt-out of voice analysis.
- Maintaining audit trails for all AI-driven decisions.
Recent legal actions against firms that failed to disclose voice data usage underscore the importance of transparency. Ethics should be embedded at every stage of the automation lifecycle, from data collection and processing to output and review.
8. Training and Workforce Impact
The shift to automated customer service has a profound impact on the workforce. However, rather than replacing staff, it redefines their roles. With automation handling simpler queries, human agents can focus on:
- Handling escalated or emotionally sensitive cases.
- Using analytics to develop strategic improvements.
- Managing and refining AI system responses.
Moreover, speech data itself serves as a training asset. By analysing real customer-agent interactions, organisations can identify top-performing behaviours, common errors, and training gaps. This creates a feedback loop that enhances both system accuracy and human capability.
Workforce reskilling programmes are now a key part of automation strategies. When staff are upskilled to manage AI tools and interpret speech data, they become more valuable, not less.
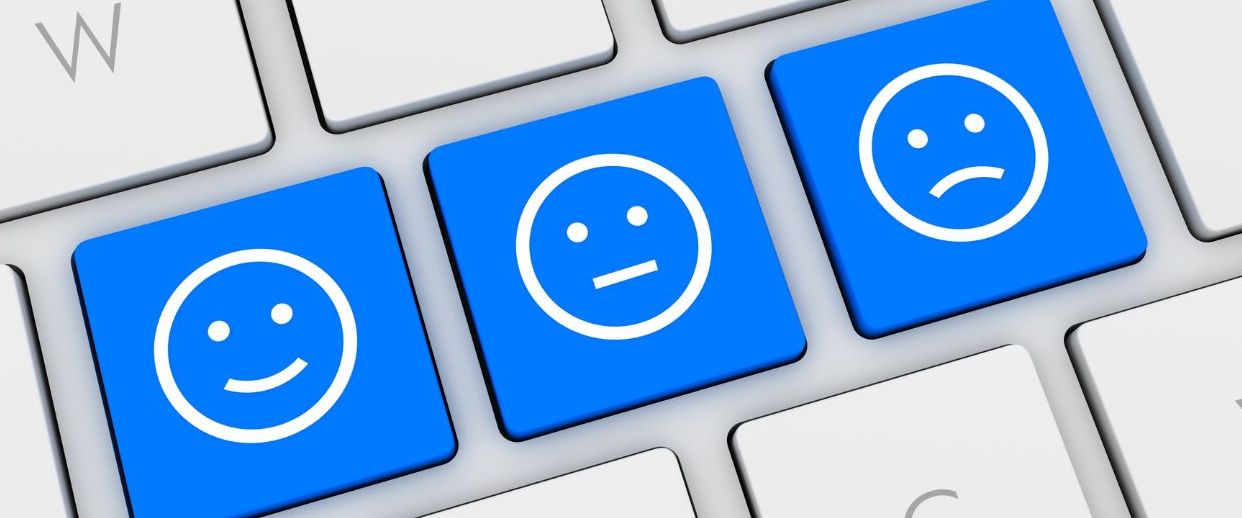
9. Measuring Automation Success
Success in speech data customer service automation isn’t just measured by how many queries are answered—it’s about outcomes. Performance measurement must be multi-dimensional, covering:
- Customer Satisfaction (CSAT): Are customers happier with the automated interaction?
- Resolution Rates: Is the system resolving issues without human escalation?
- Call Handling Time: Is time per interaction reduced?
- Operational Savings: Are automation investments generating a measurable ROI?
- Compliance Metrics: Is the system staying within legal boundaries?
Regular audits, paired with user feedback and technical logs, help organisations refine their systems for continuous improvement.
10. Future Innovations in Customer Service Technologies
The field of customer service automation continues to evolve, with several innovations on the horizon that promise to elevate the impact of speech data:
- Voice Biometrics: Provides secure customer authentication based on speech patterns.
- Multilingual NLP Engines: Supports seamless automation across diverse languages.
- Predictive Behaviour Models: Anticipate customer needs before they make contact.
- Emotion AI: Goes beyond words to detect complex emotional states.
- Proactive AI Agents: Contact customers before they encounter issues.
These developments are not hypothetical—they’re in testing or already in use by early adopters. Businesses that invest in innovation now will enjoy long-term gains in loyalty, efficiency, and brand differentiation.
Summary of Future Innovations in Customer Service
Upcoming innovations in customer service automation are expected to significantly enhance how speech data is used to improve customer interactions. One of the most promising developments is voice biometrics, which allows customers to be securely identified by their unique vocal patterns, reducing fraud and streamlining verification processes. Another advancement is the expansion of multilingual natural language processing (NLP) engines, which enable seamless, native-language support for global users—an essential tool for companies serving diverse populations.
Predictive behaviour models are also gaining traction, helping businesses anticipate customer needs based on historical speech interactions and current behaviour cues. This allows companies to proactively address issues before customers even reach out. Meanwhile, emotion AI offers the ability to detect subtle emotional shifts during conversations—providing context beyond spoken words and enabling more empathetic automated responses.
Finally, proactive AI agents are being designed to initiate contact with customers based on predefined triggers, such as account activity or unresolved issues, creating more engaging and supportive experiences.
These innovations are already being tested or implemented by leading businesses. Companies that invest early in these technologies will gain a competitive edge through improved customer loyalty, greater operational efficiency, and a more responsive service framework.
Key Tips on Speech Data for Customer Service
- Start with a pilot programme: Implement speech automation in one customer service area before expanding.
- Maintain transparency: Let users know when AI and voice data are being used.
- Combine automation with empathy: Design systems that pass control to humans at critical moments.
- Update systems regularly: Ensure models evolve with language trends and user expectations.
- Choose strategic partners: Work with vendors who prioritise data security and ethical AI.
Speech data has become a cornerstone of advanced customer service strategies. By enabling automation that is both intelligent and adaptive, speech data customer service tools are creating faster, more personalised, and more efficient experiences for users.
In this short guide, we’ve explored ten key areas—from core technologies and implementation case studies to ethics, challenges, and future directions. The impact of speech data is expansive, influencing not just how businesses respond to customers, but how they learn from them.
The key takeaway is simple: speech data must be approached as a strategic asset. Businesses that invest in its proper collection, management, and ethical application will gain a measurable advantage in both operational performance and customer satisfaction.
Speech Data Further Resources
Wikipedia: Customer Service – This article provides an overview of customer service principles and technologies, essential for understanding the impact of speech data on customer service automation.
Way With Words: Speech Collection –Way With Words enhances customer service automation with advanced speech data solutions, improving response times and customer satisfaction. Their tailored approaches streamline operations, making automation efficient and effective.