What is Speech Data And How Is It Used In Machine Learning?
Learn What Questions to Ask About Speech Data
The fields of artificial intelligence (AI) and machine learning (ML) are revolutionising how we interact with technology. At the heart of this transformation is speech data, a pivotal element that powers applications from virtual assistants to automated customer service platforms. But what exactly is speech data, and why is it so crucial for machine learning development?
Speech data encompasses the sounds, words, and phrases spoken by humans, captured and digitised for computational processing. It is the raw material used by machine learning algorithms to understand and generate human-like speech. This data not only includes the verbal cues but also the context, emotion, and nuances of how something is said.
As we delve deeper into the AI-driven world, asking the right questions about speech data becomes essential. How is it collected and processed? What makes it effective for training ML models? How can industries leverage speech data to enhance their technologies?
Understanding speech data’s fundamentals, characteristics, and applications in machine learning is crucial for data scientists, technology entrepreneurs, software developers, and industries aiming to advance their AI capabilities. By exploring these aspects, we can appreciate the value speech data brings to technology’s future.
Speech Data – Key Aspects To Consider For ASR And Other Technologies
Definition of Speech Data
Speech data is the digitised version of human speech, encompassing words, phrases, sentences, and non-verbal vocalisations. It serves as the foundation for training machine learning models to recognise, understand, and generate human language.
Speech data is the digitised representation of human speech, incorporating a wide array of sounds from articulated words and phrases to subtle non-verbal cues and vocalisations. This data is the linchpin in the interface between human language and machine processing, enabling computers to engage with human speech in a myriad of ways. It is the critical input for developing machine learning models that can recognise, interpret, and replicate human language patterns.
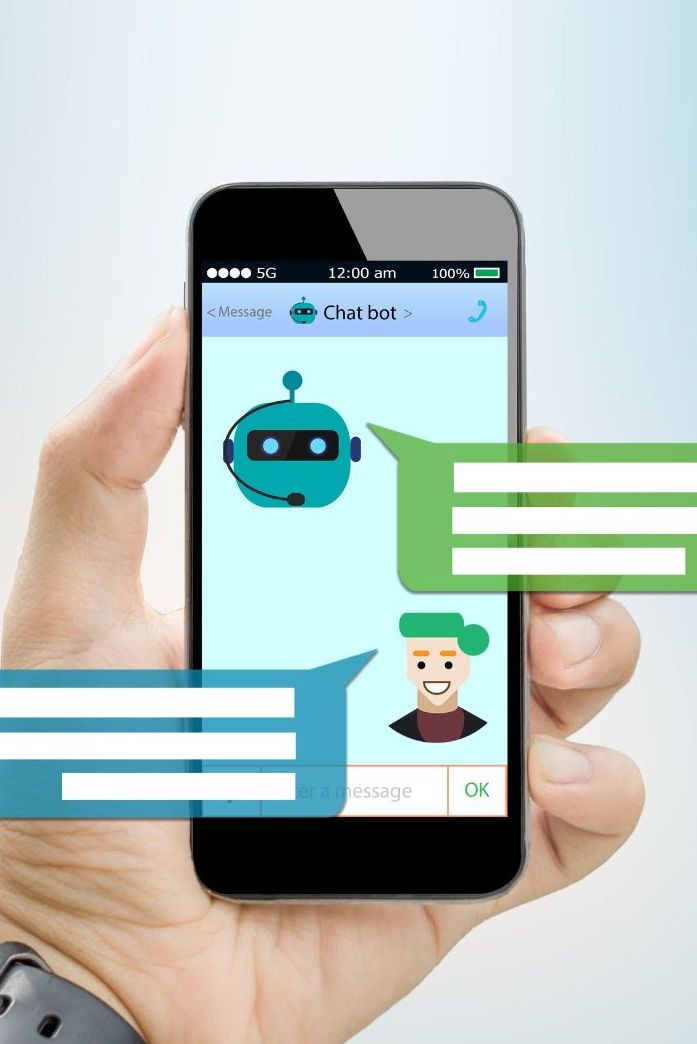
These models are trained to decipher the complexities of speech, including the intricacies of language, the nuance of expression, and the context in which words are spoken, making speech data an indispensable resource in the field of artificial intelligence.
Moreover, the evolution of speech data collection and its application in machine learning has opened up new vistas in technology, allowing for the creation of systems that can interact with users in a manner that is both intuitive and efficient. The ability to process and understand human speech with high accuracy is not just a technical achievement; it represents a fundamental shift in how we envision the interaction between humans and machines. As such, speech data stands at the forefront of efforts to create more natural, accessible, and engaging user interfaces, making it a cornerstone of modern AI research and development.
Characteristics of Speech Data
This data varies widely in terms of language, dialect, accent, intonation, and emotion. Such diversity is crucial for developing robust speech recognition and synthesis systems that can operate effectively across different speakers and contexts.
The variability of speech data is immense, reflecting the rich tapestry of human language and communication. This data spans across languages, dialects, accents, intonations, and emotional expressions, presenting a complex array of characteristics for machine learning models to interpret.
The diversity found in speech data is not merely a challenge to be overcome; it is essential for developing sophisticated speech recognition and synthesis systems capable of functioning effectively in a globalised world. These systems must be adaptable, able to understand and generate speech that accurately reflects the nuances of different languages and the idiosyncrasies of individual speakers.
This diversity also underscores the importance of inclusivity in speech technology. By encompassing a broad spectrum of speech characteristics, machine learning models can be trained to serve a wider range of users, enhancing accessibility and user experience.
The ability to accurately capture and process such a wide range of speech characteristics is critical for the creation of AI systems that can communicate naturally and effectively with users from different cultural and linguistic backgrounds. Therefore, the characteristics of speech data not only define the technical challenges in speech technology but also the potential for creating more inclusive and universal communication tools.
Collection and Processing of Speech Data
Collecting speech data involves recording spoken language from a variety of sources and demographics. Processing this data requires noise reduction, normalisation, and annotation to make it usable for machine learning algorithms.
The collection of speech data is a foundational step in building effective machine learning models, involving the recording of spoken language across diverse demographics and contexts. This process is critical for capturing the breadth of human speech variations, from different languages and dialects to various emotional states and speech impediments. The aim is to amass a dataset that is as representative as possible of the target user base, ensuring that the resulting machine learning models can understand and generate speech that is natural and accessible to all users.
Once collected, the speech data undergoes rigorous processing, including noise reduction to eliminate background interference, normalisation to ensure consistency across recordings, and annotation to label the data with relevant linguistic and phonetic information. These steps are crucial for transforming raw speech recordings into a structured format that machine learning algorithms can effectively learn from.
The complexity of speech data collection and processing underscores the challenges in creating machine learning models that can deal with the nuances of human language. The effort to capture a wide array of speech characteristics necessitates sophisticated recording equipment, diverse data sources, and advanced processing techniques.
Moreover, the need for high-quality annotations demands expertise in linguistics and a deep understanding of the specific requirements of speech-based applications. As such, the process of collecting and processing speech data is not just a technical task but a multidisciplinary effort that lies at the heart of advancing speech technology.
Role in Training Machine Learning Models
Speech data is used to train algorithms in speech recognition, natural language understanding, and speech synthesis. By learning from vast amounts of speech data, ML models can accurately interpret and generate human speech.
Speech data plays a pivotal role in training machine learning models for a variety of applications, from speech recognition and natural language understanding to speech synthesis. These models learn to interpret the complexities of human speech by analysing vast amounts of speech data, identifying patterns, and understanding the nuances of language, emotion, and context.
The quality and diversity of the speech data directly influence the ability of these models to accurately recognise and generate speech, making the collection and processing of speech data a critical factor in the development of effective speech technologies. Through iterative training and validation processes, machine learning models continuously refine their ability to understand and replicate human speech, leading to advancements in AI that can communicate more naturally and effectively with users.
Furthermore, the role of speech data extends beyond the technical aspects of model training, impacting the design and implementation of user interfaces and interaction paradigms. By enabling machines to process spoken language accurately, speech data facilitates the creation of more intuitive and accessible technologies, reducing barriers to technology use and enhancing the user experience.
The integration of speech-based interaction in devices and applications signifies a shift towards more human-centred computing, where technology adapts to the natural modes of human communication. Thus, the role of speech data in training machine learning models is instrumental in bridging the gap between human users and digital technologies, fostering a more inclusive and engaging digital environment.
Speech Recognition Technology
Speech recognition involves converting spoken language into text. Machine learning models trained on diverse speech data can recognise spoken words with high accuracy, enabling voice-activated commands and dictation.
Speech recognition technology, which converts spoken language into text, stands as a testament to the strides made in machine learning and speech data processing. Trained on diverse and extensive datasets, machine learning models can now recognise spoken words with remarkable accuracy, enabling a myriad of applications from voice-activated search to real-time transcription services.
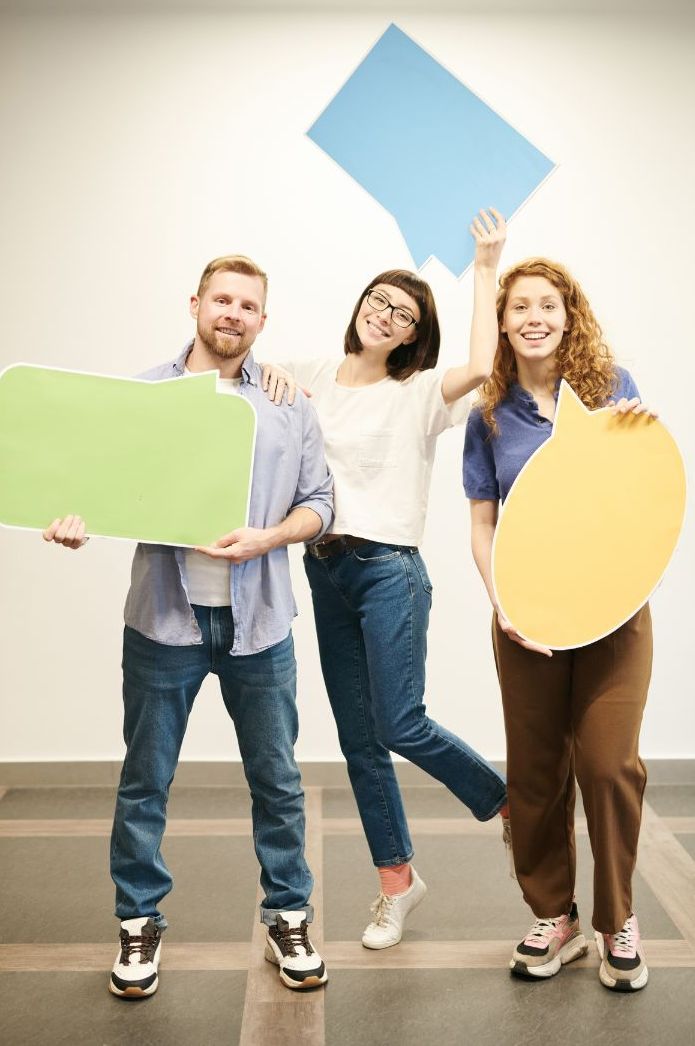
This capability has not only enhanced the convenience and efficiency of interacting with technology but has also opened up new avenues for accessibility, allowing individuals with physical or visual impairments to engage with digital content more freely. The advancement of speech recognition technology reflects a broader trend towards creating more natural and intuitive user interfaces, where the need for traditional input methods like keyboards and mice is diminished in favour of voice commands.
The impact of speech recognition technology extends beyond individual user convenience, influencing sectors such as healthcare, where it facilitates hands-free documentation, and automotive, where it enhances safety by allowing drivers to keep their hands on the wheel and eyes on the road.
However, the success of speech recognition systems hinges on the quality of the speech data used for training, underscoring the importance of comprehensive datasets that capture the full spectrum of human speech variability. As technology continues to evolve, the ongoing challenge will be to ensure that speech recognition systems are inclusive and equitable, capable of understanding and serving users from all linguistic and cultural backgrounds.
Natural Language Understanding (NLU)
NLU is the ability of a machine to understand human language as it is spoken. Training models on speech data allows them to grasp context, emotion, and intent, enhancing interaction quality.
Natural Language Understanding (NLU) represents a significant leap forward in the quest for machines to comprehend human language in its spoken form. By training on nuanced speech data, NLU systems can parse the subtleties of conversation, recognising not just the words but the intent and emotion behind them.
This deep comprehension allows for interactions with technology that are more sophisticated and human-like, enabling machines to respond in ways that are contextually appropriate and emotionally resonant. NLU’s importance lies not only in its technical achievements but also in its potential to democratise technology, making digital services more accessible and intuitive for users regardless of their literacy or technical expertise.
The advancement in NLU technologies is paving the way for more meaningful interactions between humans and machines, fostering a new era of digital assistants that can understand and anticipate user needs with a high degree of accuracy. However, achieving this level of understanding requires a continuous effort to refine and expand the datasets used for training, ensuring that they reflect the diversity of human speech and language use.
As NLU systems become more integrated into our daily lives, they hold the promise of making technology an even more seamless and integral part of human experience, transforming how we communicate, work, and access information.
Speech Synthesis and Text-to-Speech (TTS)
Speech synthesis involves generating human-like speech from text. Training on varied speech data enables these systems to produce natural-sounding and emotive speech.
Speech synthesis and Text-to-Speech (TTS) technologies have undergone a remarkable transformation, driven by advances in machine learning and the availability of rich speech data. These technologies now have the capability to generate speech that closely mimics human intonation, rhythm, and emotion, making digital interactions more natural and engaging.
The development of TTS systems that can produce lifelike speech has significant implications for accessibility, enabling individuals with reading difficulties or visual impairments to access written content through auditory means. Furthermore, speech synthesis enhances the user experience across a wide range of applications, from virtual assistants to interactive educational tools, providing a more intuitive and accessible way for users to engage with digital content.
The progress in speech synthesis technology also underscores the importance of diverse and comprehensive speech datasets. To achieve a natural and expressive speech output, TTS systems must be trained on a wide variety of speech samples that capture different accents, dialects, and emotional states.
This requirement highlights the ongoing challenge of creating speech technologies that are inclusive and representative of the global population. As TTS technology continues to evolve, it holds the potential to revolutionise the way we interact with machines, making digital content more accessible and enriching the human-computer interface with more human-like communication capabilities.
Challenges in Speech Data Utilisation
Handling diverse accents, dialects, and noisy environments are significant challenges. Ensuring privacy and ethical use of speech data also poses concerns.
Utilising speech data effectively presents a range of challenges, from technical hurdles such as dealing with diverse accents and dialects to ethical considerations around privacy and consent. The variability of speech, influenced by factors such as regional accents, language diversity, and the presence of background noise, requires sophisticated algorithms and processing techniques to ensure high accuracy in speech recognition and synthesis. Moreover, as speech technologies become more pervasive, the imperative to address privacy concerns and ensure the ethical use of speech data becomes increasingly critical.
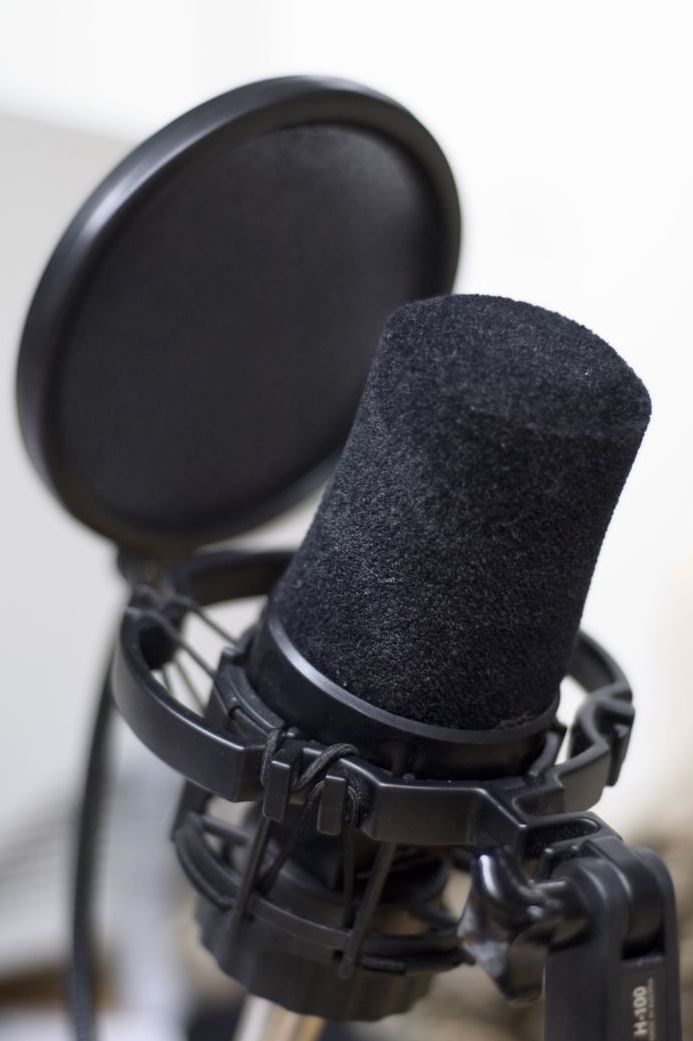
Balancing the need for comprehensive speech datasets with the rights of individuals to control their personal information is a complex challenge that requires careful consideration and robust data governance practices. In addition to these challenges, the development of speech technologies also faces the issue of bias, where systems may perform less effectively for certain demographic groups due to underrepresentation in training datasets.
Ensuring fairness and equity in speech technology requires a concerted effort to include diverse voices in speech data collection efforts, as well as ongoing research to identify and mitigate biases in machine learning models. As the field of speech technology continues to advance, addressing these challenges will be crucial for realising the full potential of speech data to create more inclusive, accessible, and effective digital tools and services.
Advancements in Speech Data and ML
Continuous improvements in ML algorithms and data processing techniques are making speech-based applications more accurate, efficient, and accessible across languages and dialects.
The landscape of speech data and machine learning is rapidly evolving, with continuous advancements in data processing techniques and algorithmic innovation driving improvements in speech-based applications. These advancements are making speech technologies more accurate, efficient, and capable of understanding and generating speech across a wider range of languages and dialects.
The integration of deep learning techniques, for example, has significantly enhanced the ability of machine learning models to process complex patterns in speech data, leading to breakthroughs in speech recognition accuracy and the naturalness of synthesised speech. Additionally, the development of more sophisticated data annotation tools and methodologies is enabling the creation of richer and more detailed speech datasets, further improving the training of speech-based machine learning models.
The progress in speech data and machine learning is not just a technical achievement; it represents a shift towards more natural and human-centric computing interfaces. As speech technologies become more refined, they are increasingly woven into the fabric of daily life, enabling more intuitive and accessible interactions with technology.
The future of speech data and machine learning holds the promise of breaking down language barriers, enhancing global communication, and creating digital experiences that are more inclusive and engaging for people around the world. The continued innovation in this field is a key driver in the ongoing transformation of how we interact with the digital world, making technology an ever more integral and seamless part of human life.
Impact of Speech Data on Industries
Speech data is transforming industries by enabling more natural user interfaces, improving customer service through voice bots, and creating accessible technologies for diverse user bases.
The impact of speech data on various industries is profound and far-reaching, driving innovations that are transforming the way businesses operate and interact with their customers. In the customer service sector, for example, voice bots and virtual assistants powered by advanced speech recognition and NLU technologies are enhancing the customer experience by providing prompt, accurate, and personalised service.
In the automotive industry, speech technologies are improving driver safety and convenience through hands-free controls and voice-activated navigation systems. The healthcare sector is witnessing the integration of speech recognition technologies to streamline documentation processes and facilitate more efficient patient care.
The widespread adoption of speech technologies across industries is not only improving operational efficiency and customer satisfaction but is also paving the way for more innovative and accessible services. As industries continue to harness the power of speech data, the potential for creating more natural and engaging interactions between humans and technology grows exponentially.
The ongoing advancements in speech data analysis and machine learning are key enablers for this transformation, offering new opportunities for businesses to differentiate themselves and provide value to their customers. The impact of speech data is thus a testament to the transformative power of technology when applied thoughtfully and inclusively, heralding a future where digital interactions are as natural and intuitive as human conversation.
Some Key Tips For Quality Speech Data Collecting
- Ensure diversity and inclusivity in your speech data collection to develop more robust and versatile applications.
- Pay attention to the ethical considerations and privacy issues surrounding the collection and use of speech data.
- Utilise noise reduction and normalisation techniques to improve the quality of your speech data before training models.
- Annotation and labelling of speech data are critical for effective machine learning training, particularly for NLU tasks.
- Continuously update and expand your speech data sets to cover new accents, dialects, and linguistic nuances.
How Way With Words Provides Value
Way With Words specialises in creating high-quality, customised speech datasets, including transcripts for machine learning purposes, particularly in the realms of automatic speech recognition (ASR) and natural language processing (NLP). Their services cater to technologies looking to develop or enhance existing speech recognition models across various languages and domains. Additionally, their machine transcription polishing service ensures that machine-generated transcripts reach the highest level of accuracy, supporting a range of AI and machine learning applications from research to customer service technologies.
Speech data stands as a cornerstone in the advancement of machine learning technologies, enabling systems to interact with humans in the most natural way possible: through spoken language. From its collection and processing to its application in speech recognition, natural language understanding, and synthesis, speech data is integral to developing AI technologies that are more intuitive, accessible, and effective. As we look to the future, the continuous evolution of speech data collection, processing techniques, and ethical standards will be paramount in pushing the boundaries of what machine learning can achieve.
For data scientists, technology entrepreneurs, and software developers, understanding the intricacies of speech data and its application in machine learning is essential. Leveraging high-quality speech datasets and staying abreast of advancements in the field are key to creating technologies that not only understand and generate human speech but do so in a way that is respectful of privacy and ethical considerations.
Key Piece of Advice: Invest in the quality and diversity of your speech data. The success of machine learning models, particularly in speech recognition and natural language processing, hinges on the richness and comprehensiveness of the data they are trained on. Embrace the complexity and diversity of human speech as an opportunity to innovate and lead in the creation of AI technologies that truly understand and engage with users across the globe.
Speech Data and Machine Learning Resources
Way With Words Speech Collection Services: “We create speech datasets including transcripts for machine learning purposes. Our service is used for technologies looking to create or improve existing automatic speech recognition models (ASR) using natural language processing (NLP) for select languages and various domains.”
Way With Words Machine Transcription Polishing: “We polish machine transcripts for clients across a number of different technologies. Our machine transcription polishing (MTP) service is used for a variety of AI and machine learning purposes. User applications include machine learning models that use speech-to-text for artificial intelligence research, FinTech/InsurTech, SaaS/Cloud Services, Call Centre Software and Voice Analytic services for the customer journey.”
Top Sources For Sample Speech Collection: Listed 5 sources to collect speech data for automatic speech recognition-based models with the algorithmic point of view.