Transforming Healthcare with Speech Data: Innovations & Applications
How can Speech Data be Used in Healthcare Applications?
Speech data is increasingly recognised as a transformative force in healthcare, underpinning innovations across diagnosis, treatment, patient communication, and operational efficiency. With artificial intelligence (AI) and machine learning (ML) now capable of interpreting complex speech patterns and linguistic cues, the ability to extract clinical meaning from spoken interactions is becoming more refined and reliable.
From real-time transcription of patient consultations to the identification of voice-based biomarkers, speech data in healthcare, whether synthetic or real, is offering new ways to personalise and optimise care delivery across a broad spectrum of settings—from outpatient clinics and emergency services to long-term care facilities and telehealth platforms.
Beyond technical capacity, the growing acceptance of voice-based technology by healthcare professionals and patients alike is paving the way for speech data to become a core element of modern medical practice. The versatility of speech-driven tools allows for application in administrative automation, clinical diagnostics, mental health monitoring, and multilingual communication—each contributing to improved health system efficiency and better patient outcomes. Speech data not only makes it easier for clinicians to document and review patient interactions but also opens a path for more humane, patient-centred care by capturing subtle vocal indicators that may otherwise go unnoticed.
As speech AI technologies mature and become more integrated with hospital infrastructure and electronic medical record (EMR) systems, the reliance on traditional manual inputs is expected to decline. Innovations in natural language processing (NLP), edge computing, and federated learning are also improving the scalability and security of voice data applications. Importantly, stakeholders are increasingly mindful of the ethical and legal implications surrounding voice data—ensuring compliance with strict data protection laws, managing bias in AI training datasets, and securing informed consent from patients.
The integration of speech data in healthcare, however, also raises critical questions—many of which are being asked not only by technologists but also by frontline clinicians and administrators:
- How is speech data collected and used in clinical settings?
- Can voice analysis assist in early diagnosis of diseases?
- What are the legal and ethical implications of using patient voice recordings?
- How does speech data improve operational workflows in hospitals and clinics?
- Can AI-driven voice recognition systems handle multilingual and culturally diverse patient populations?
- How accurate are current speech recognition systems in real-world healthcare environments?
- What are the limitations of using voice data compared to other clinical data streams?
This short guide answers these and other pressing questions, drawing on real-world applications and supporting evidence to illustrate how speech data is changing the delivery of care at every level—from the examination room to national health policy. By exploring specific use cases, challenges, and future developments, we aim to equip professionals in healthcare, technology, and policy-making with the knowledge needed to understand and leverage speech data in responsible and impactful ways.
Speech Data in Healthcare Insights
1. Automating Clinical Documentation
The administrative burden faced by healthcare professionals is well-documented, with studies showing that up to 35% of a doctor’s time is spent on record-keeping. This inefficiency detracts from direct patient care. Enter speech recognition: when integrated into clinical workflows, speech-to-text tools allow for rapid and accurate input into electronic health records (EHRs). These systems are increasingly equipped with medical lexicons and can understand complex terminology, abbreviations, and regional accents.
Advanced systems use ambient clinical intelligence (ACI), which continuously listens in during consultations, captures relevant dialogue, and automatically populates structured fields in the EHR. For example, a physician could say, “Patient reports chest pain for the last two days,” and the ACI system will extract both the symptom and the duration, tagging them appropriately. This reduces the risk of missing important details and significantly cuts down documentation time.
Some institutions have reported a 45% reduction in time spent on administrative tasks, while also seeing improvements in the completeness of medical records. When implemented properly, this application of speech data not only eases clinician workload but also improves data accuracy and audit readiness.
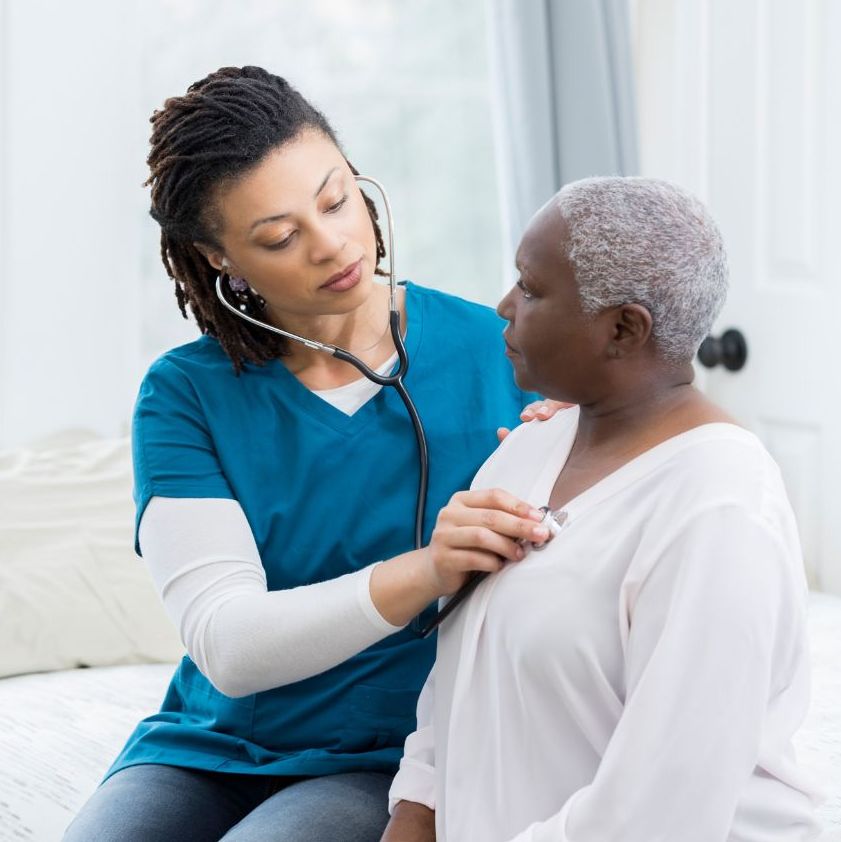
2. Voice Biomarkers for Early Disease Detection
One of the most promising applications of speech data in healthcare is the detection of disease through changes in speech. Known as voice biomarkers, these indicators are subtle variations in pitch, tone, frequency, and rhythm that correspond to physiological changes.
For example, research from the Mayo Clinic and Massachusetts Institute of Technology (MIT) has shown that patients with Parkinson’s disease often exhibit specific vocal tremors years before other symptoms appear. Similarly, Alzheimer’s disease can be flagged early through disruptions in sentence construction, word recall, and vocal cadence. Machine learning algorithms trained on large datasets of annotated speech recordings have achieved diagnostic accuracy rates of 80% or more in preliminary trials.
Depression and anxiety are also detectable through speech features such as monotone delivery, hesitation markers, and slower speech rate. Companies developing voice-based screening tools are now collaborating with mental health platforms and primary care providers to integrate such capabilities into routine assessments.
By enabling earlier intervention, these tools promise to lower healthcare costs and improve patient outcomes significantly.
3. Enhancing Telehealth Services
The widespread adoption of telehealth has accelerated the need for reliable speech processing tools. During remote consultations, ensuring accurate documentation and understanding between patient and provider is essential. Real-time transcription tools powered by AI can capture key details of the conversation, producing comprehensive summaries and updating patient records on the fly.
Additionally, sentiment analysis tools can evaluate emotional tone during video or audio consultations, potentially identifying distress or urgency that may not be explicitly communicated. This layer of nuance can aid clinicians in triaging patients more effectively.
Telehealth speech data systems also facilitate remote triage and follow-ups, enabling a more scalable model for healthcare delivery. When integrated with chatbot-style interfaces, these systems can gather preliminary patient information before a doctor is involved, helping to prioritise care and reduce consultation times.
4. Supporting Mental Health Assessments
Mental health services are often constrained by time and staffing, making scalable, non-invasive diagnostic tools highly valuable. Speech analysis can support clinicians by detecting emotional stress, depressive symptoms, and anxiety indicators through vocal parameters.
For instance, stress typically raises pitch and increases vocal tension, while depressive states may result in slower speech and diminished articulation. AI in healthcare speech data tools trained on such patterns can offer immediate feedback during calls to suicide prevention lines or therapy sessions. In some cases, these tools have helped detect signs of relapse before a patient reports any subjective symptoms.
Furthermore, mental health apps increasingly incorporate speech recognition as part of their self-assessment tools. Patients speak into their devices, and the system analyses their responses for signs of distress or emotional imbalance. These results can then be shared with healthcare providers for a follow-up, creating a seamless monitoring system for ongoing mental health support.
5. Streamlining Patient Intake and Scheduling
Administrative functions such as scheduling appointments, verifying insurance, or checking test results can become bottlenecks in the patient journey. Speech data applications are now being used to automate these processes.
Healthcare organisations are deploying voice-enabled virtual assistants and phone systems that handle patient queries with natural language processing. For example, a patient calling to schedule a follow-up visit can interact with a speech-enabled assistant that understands the request, checks availability, and books the appointment—all without human intervention.
This reduces overheads, speeds up response times, and allows staff to focus on more complex or sensitive interactions. More importantly, these tools improve accessibility for patients who may have difficulty navigating digital interfaces, such as the elderly or visually impaired.
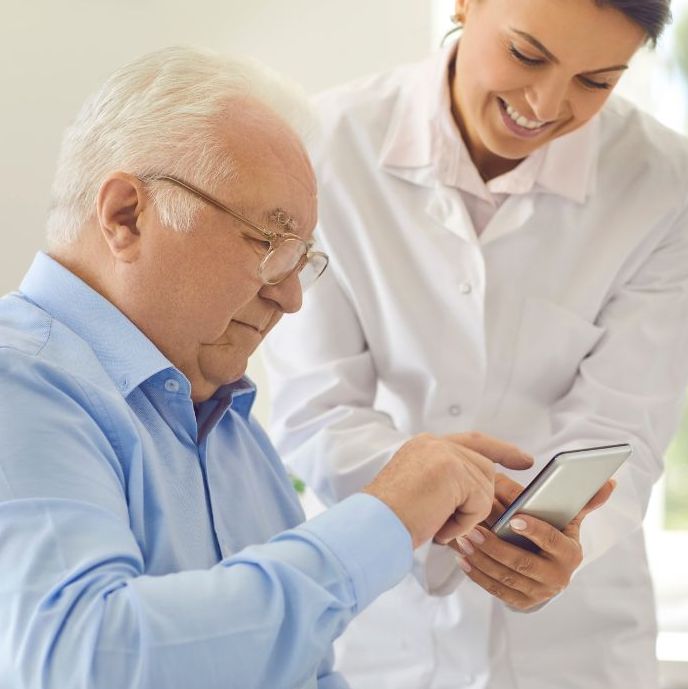
6. Language Translation for Multilingual Care
In multilingual societies, language barriers can compromise healthcare delivery. AI-powered speech-to-text systems with integrated translation features are helping bridge this gap by converting spoken language in real time.
For instance, during a consultation, a non-English speaking patient can speak in their native language while the clinician hears or reads a translated version. Simultaneously, the clinician’s responses are translated back for the patient. These systems are particularly useful in emergency departments, community health clinics, and rural outreach programmes.
Accuracy is continually improving, with some systems incorporating context-aware translation models to understand idiomatic expressions or culturally specific terms. As a result, patient understanding and trust improve, and clinical errors due to miscommunication are reduced.
7. Training and Education for Healthcare Professionals
Medical training increasingly includes analysis of communication styles, empathy, and patient interaction quality. Speech data analytics allows training programmes to capture and review conversations between trainees and simulated or real patients.
Using automated transcription and sentiment analysis, educators can provide structured feedback on elements such as clarity, patient engagement, and response appropriateness. For example, a student’s tendency to interrupt or use overly complex jargon can be identified and corrected.
Additionally, training libraries consisting of annotated speech recordings from real scenarios help students and clinicians-in-training develop listening skills and pattern recognition for diagnosis. These resources are especially useful in preparing healthcare workers for multilingual or cross-cultural settings.
8. AI-Powered Clinical Decision Support
Speech data is also fuelling the next generation of clinical decision support tools. Rather than typing queries into a search system, doctors can now speak directly to an AI-powered assistant.
These systems respond to natural language requests with relevant guidelines, drug information, or differential diagnosis lists. In emergency scenarios, this hands-free interaction is particularly beneficial, allowing clinicians to access information while focusing on patient care.
For example, a doctor might ask, “What are the recommended antibiotics for community-acquired pneumonia in elderly patients?” The system interprets the query, consults clinical databases, and presents evidence-based recommendations within seconds. Such functionality enhances speed, accuracy, and consistency in care delivery.
9. Legal and Ethical Safeguards
While the clinical benefits of using speech data in healthcare are substantial, legal and ethical compliance must not be overlooked. Healthcare organisations handling voice data are subject to regulations like the General Data Protection Regulation (GDPR) in Europe and the Health Insurance Portability and Accountability Act (HIPAA) in the US.
Informed consent is a cornerstone requirement—patients must be clearly told when and how their voice data is recorded, stored, and used. Systems should implement robust encryption protocols and access controls to prevent unauthorised usage.
Ethical considerations also include algorithmic bias, especially when speech data systems are trained predominantly on specific demographics. This can result in reduced accuracy for underrepresented voices. Developers must prioritise diverse training datasets and continuous system auditing to avoid perpetuating health inequities.
10. Future Trends: Personalised and Predictive Care
As AI models become more sophisticated, speech data will play a growing role in personalised healthcare. Beyond documenting symptoms, systems will use patient speech to predict health events, track chronic condition progression, and suggest tailored interventions.
Emerging research is looking at integrating speech analysis into wearables and smart home devices to provide round-the-clock monitoring. For instance, a smart speaker could detect signs of respiratory distress or stroke in real time and notify emergency services.
In hospital settings, integrated voice systems may help manage post-operative care, medication reminders, and pain assessments through verbal check-ins. This human-like interface can improve compliance and reduce readmissions.
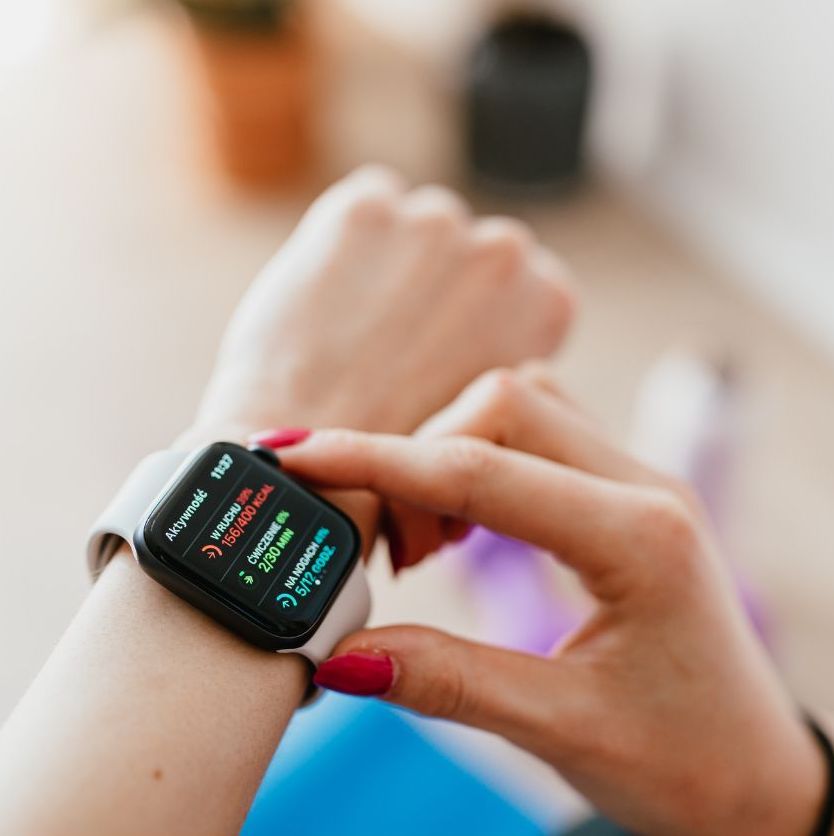
Ultimately, the convergence of voice technology, predictive analytics, and patient-centred design may herald a time of proactive and intuitive healthcare delivery.
Key Tips for Using Speech Data in Healthcare
- Start with Clear Use Cases: Identify specific functions—clinical, administrative, or operational—where speech data can offer tangible improvements.
- Ensure Regulatory Compliance: Stay aligned with national and international privacy laws by incorporating legal reviews in every implementation phase.
- Invest in Domain-Specific Models: Use speech systems that are trained specifically on medical data to improve transcription and interpretation accuracy.
- Collaborate Across Disciplines: Involve clinicians, IT professionals, legal advisors, and patient advocates in planning and deployment.
- Evaluate and Iterate: Regularly test systems in live environments, gather feedback, and refine based on evolving needs.
Speech data is emerging as a powerful enabler of change across the healthcare sector. Its value lies not just in speeding up documentation or automating routine tasks, but in offering new pathways to understand, diagnose, and support patients. From detecting early signs of neurological decline to enabling multilingual care, the applications are diverse and rapidly maturing.
However, reaping the benefits of speech data requires a strategic approach. Healthcare leaders must invest in secure, accurate, and ethically sound solutions. This includes training staff, securing informed consent, and continuously evaluating the systems in use.
The potential for speech data to drive personalised medicine is perhaps its most compelling promise. In a time when patient expectations and systemic pressures are rising, the ability to communicate clearly, respond intuitively, and act pre-emptively could make speech data not just a tool—but a vital ally.
Further Speech Data Resources
Wikipedia: Healthcare – This article provides an overview of healthcare systems and technologies, essential for understanding the applications of speech data in healthcare.
Way With Words: Speech Collection – Way With Words supports healthcare applications with specialised speech data solutions, facilitating medical diagnostics and patient care. Their expertise enhances efficiency and accuracy in healthcare AI, improving outcomes for providers and patients alike.