How is Speech Data Used in Natural Language Processing?
How To Effectively Use Speech Data to Improve NLP Technologies
Natural language processing (NLP) stands out as a cornerstone technology that bridges human communication and machine understanding. In NLP, a critical component emerges: speech data. The role of speech data in NLP is multifaceted, serving as the backbone for various applications that require machines to interpret, analyse, and generate human language in a meaningful way. From speech-to-text transcription services to sentiment analysis and language translation, the integration of speech data into NLP tasks is transforming how machines understand and interact with us.
For data scientists, technology entrepreneurs, software developers, and industries leveraging AI to enhance machine learning capabilities, it’s essential to ask: How can we effectively use speech data to improve NLP technologies? What challenges and opportunities does speech data present in training AI models? And how can speech data be optimised for applications in data analytics and speech recognition solutions?
Important Aspects of Natural language Processing (NLP)
The Fundamentals of Speech Data in NLP
Explore the basics of speech data, including its collection, processing, and significance in training NLP models.
The essence of speech data in natural language processing (NLP) encompasses more than just recording and transcribing human speech. It involves a complex process of capturing, processing, and analysing the spoken word to train AI and machine learning models to understand and generate human language effectively.
The collection of speech data is the first critical step, where diverse linguistic patterns, accents, and dialects are gathered from various demographics to ensure the comprehensiveness and inclusivity of the data. This diversity is paramount, as it enables the development of NLP models that can accurately interpret and respond to a wide range of human speech nuances.
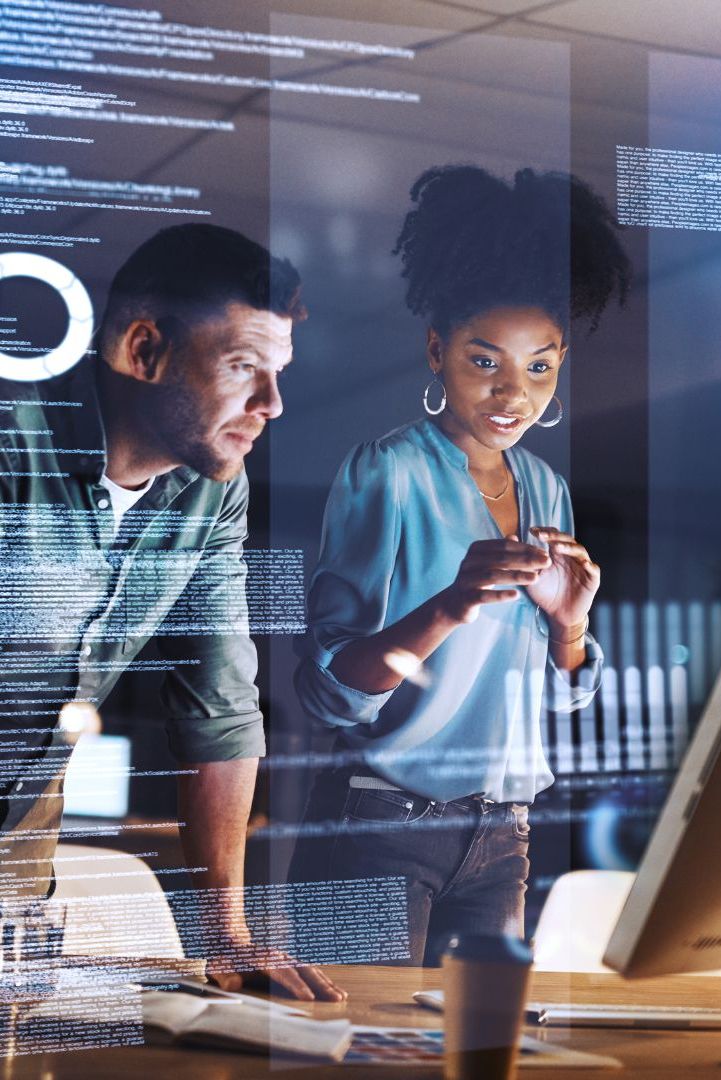
Processing this collected data involves several stages, including segmentation, noise reduction, and normalisation, to prepare it for analysis and training purposes. This processed speech data then plays a significant role in training NLP models by providing real-world examples of how language is used in different contexts. These models learn to recognise speech patterns, understand context, and even predict future linguistic trends, making speech data indispensable in the realm of NLP. The significance of high-quality speech data cannot be overstated, as it directly impacts the accuracy, reliability, and effectiveness of NLP applications, from voice-activated assistants to customer service chatbots.
Speech-to-Text Transcription Techniques
Discuss the technologies behind speech-to-text conversion and its importance in making digital content accessible.
Speech-to-text transcription is a cornerstone of making digital content accessible and interactive. This technology converts spoken language into written text, enabling a myriad of applications, from real-time captioning for the hearing impaired to voice-driven command interfaces.
The technology behind speech-to-text transcription has evolved significantly, with advanced algorithms and deep learning models now capable of understanding and transcribing speech with remarkable accuracy. These models are trained on vast datasets of speech data, learning to discern words and phrases amidst various accents, speech rates, and background noises.
The importance of speech-to-text technology extends beyond accessibility, serving as a foundation for further NLP tasks such as sentiment analysis, language translation, and even AI-driven content creation. By transforming spoken language into text, it opens up new possibilities for analysing and utilising language data, making it a pivotal technology in bridging the gap between human speech and digital information. As the demand for more intuitive and natural user interfaces grows, the role of speech-to-text transcription in enabling seamless human-computer interactions becomes increasingly significant.
Sentiment Analysis Using Speech Data
How speech data is analysed for emotional content to improve customer service and product feedback.
Sentiment analysis of speech data is a powerful tool for gauging emotions, attitudes, and opinions expressed in spoken language. This aspect of NLP allows businesses and organisations to extract valuable insights from customer interactions, feedback, and social media conversations. By analysing the tone, pace, and inflections in speech, AI models can identify and categorise the sentiment behind words, offering a more nuanced understanding of customer sentiment than text-based analysis alone. This emotional intelligence is crucial for improving customer service, tailoring marketing strategies, and enhancing product development based on real feedback.
The complexity of sentiment analysis lies in interpreting the subtle cues in speech that convey emotions, such as sarcasm, excitement, or frustration. Advanced NLP models trained on diverse speech datasets can discern these nuances, transforming raw speech data into actionable insights. For industries ranging from retail to healthcare, this means being able to respond to customer needs more effectively and personalise interactions, thereby fostering stronger customer relationships and improving overall satisfaction.
Language Translation and Speech Data
The role of speech data in breaking down language barriers through real-time translation services.
Language translation powered by speech data is breaking down global communication barriers, enabling real-time, cross-linguistic interactions that were once the realm of science fiction. This NLP application leverages speech data to train models capable of understanding and translating spoken language on the fly, facilitating seamless conversations between speakers of different languages. The complexity of this task cannot be understated, as it requires not only the translation of words and phrases but also the preservation of context, cultural nuances, and the speaker’s intent.
The role of speech data in this transformative technology is pivotal. By training on diverse datasets encompassing a wide range of languages, dialects, and accents, AI models are becoming increasingly proficient at delivering accurate and contextually relevant translations. This progress opens up new opportunities for international business, travel, and cross-cultural exchange, making language translation one of the most impactful applications of speech data in NLP. As these models continue to improve, the dream of removing language barriers altogether becomes more tangible, underscoring the transformative potential of integrating speech data into NLP.
The Challenge of Accent and Dialect in Speech Recognition
Addressing the complexity of various accents and dialects in creating universal speech recognition systems.
The diversity of human language, with its myriad accents and dialects, presents a significant challenge to developing universal speech recognition systems. These systems must not only recognise words and phrases but also understand them across different linguistic variations.
This challenge is met by collecting and processing speech data from a wide demographic, training AI models to discern subtle differences in pronunciation and usage. The goal is to create speech recognition systems that are as inclusive and accessible as possible, capable of serving users from all linguistic backgrounds without bias.
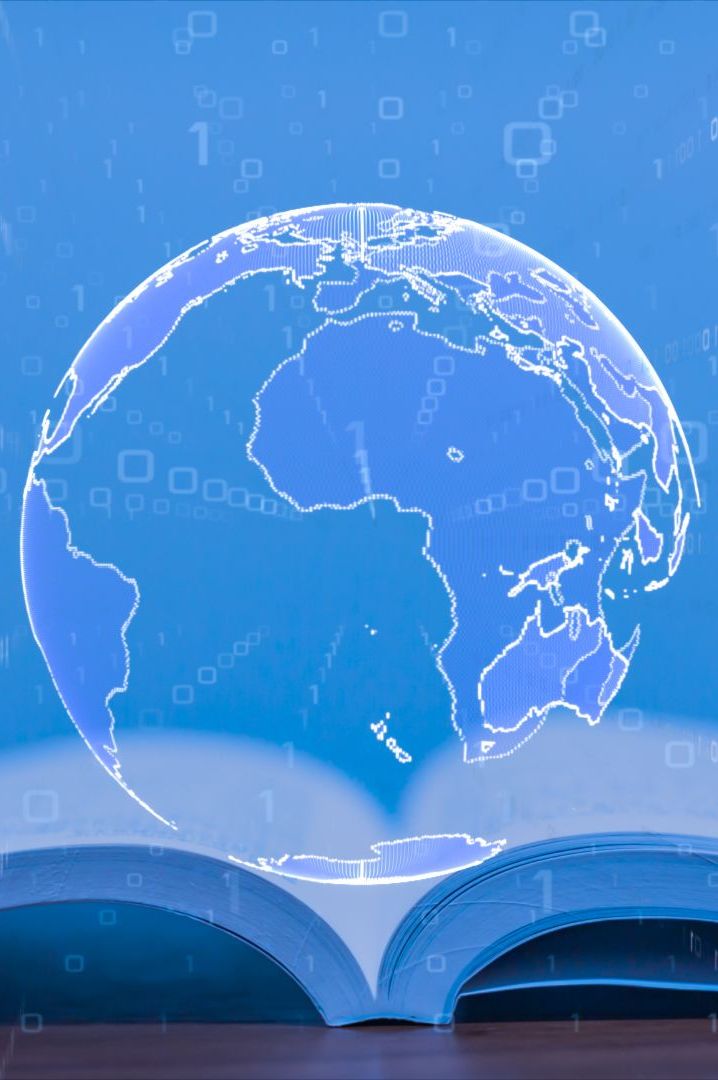
Addressing the complexity of accents and dialects in speech recognition is not just a technical challenge but also a matter of fairness and accessibility. By ensuring that NLP models are exposed to a broad spectrum of speech patterns, developers can mitigate the risk of bias and enhance the system’s ability to accurately interpret and respond to diverse users. This inclusivity is crucial for applications ranging from voice-activated assistants to automated transcription services, where the ability to understand varied speech patterns directly impacts user experience and satisfaction.
Noise Reduction and Quality Improvement in Speech Data
Techniques for cleaning speech data to enhance the accuracy of NLP applications.
Enhancing the accuracy of NLP applications begins with the quality of speech data, where noise reduction plays a critical role. Background noise, varying recording qualities, and other auditory interferences can significantly impact the clarity of speech data, challenging the training and performance of AI models. Employing advanced noise reduction techniques, such as spectral subtraction and machine learning algorithms, helps isolate the speech signal from unwanted noise, resulting in cleaner data for analysis and model training.
Improving the quality of speech data not only aids in the accuracy of transcription and recognition tasks but also enhances the performance of more complex NLP applications, such as sentiment analysis and language translation. High-quality speech data ensures that AI models can focus on linguistic patterns rather than being misled by extraneous sounds, leading to more reliable and effective NLP solutions. As technology advances, the continuous improvement of speech data quality remains a pivotal focus, driving the development of more sophisticated and accurate AI-driven applications.
Ethical Considerations in Speech Data Collection
Discuss the importance of privacy and consent in collecting and using speech data.
The collection and use of speech data are fraught with ethical considerations, emphasising the need for transparency, consent, and privacy. As speech data can contain sensitive personal information, it’s imperative that entities collecting this data adhere to strict ethical guidelines and legal requirements. This includes obtaining explicit consent from individuals, anonymising data to protect identities, and ensuring that the data collection process is transparent and respectful of individual rights. Ethical speech data collection is not just a legal obligation but also a trust-building measure, crucial for maintaining public confidence in AI technologies.
Moreover, ethical considerations extend to the fairness and bias in AI models trained on speech data. Ensuring that speech datasets are diverse and representative of various demographics is essential to prevent biases that could lead to unequal treatment or discrimination. Ethical NLP practices demand a commitment to inclusivity, fairness, and respect for privacy, guiding the development of technologies that are not only advanced but also equitable and respectful of individual rights.
Custom Speech Datasets for Niche Applications
The value of tailored speech datasets in training specialised NLP models.
The creation of custom speech datasets tailored to specific industries or applications is proving invaluable in training specialised NLP models. These bespoke datasets are designed to reflect the unique linguistic characteristics, terminology, and speech patterns relevant to a particular field, such as medical, legal, or customer service industries.
By focusing on the specific needs and challenges of these domains, custom speech datasets enable the development of highly accurate and efficient NLP solutions that can understand and process domain-specific language with precision. The value of these tailored datasets lies in their ability to address the nuanced requirements of niche applications, enhancing the functionality and reliability of NLP technologies in specialised contexts.
For instance, in healthcare, a custom speech dataset can include medical terminology and patient interactions, facilitating the development of AI tools that assist with documentation, diagnosis, and patient care. This targeted approach ensures that NLP models are not just broadly competent but exceptionally proficient in specific domains, driving innovation and efficiency in industries where accuracy and domain knowledge are paramount.
Advancements in AI and Machine Learning for Speech Analysis
Explore the latest breakthroughs in AI that are enhancing speech data processing and analysis.
Recent breakthroughs in AI and machine learning are significantly enhancing the processing and analysis of speech data, pushing the boundaries of what’s possible in NLP. These advancements include the development of deep learning models that can more accurately recognise and interpret complex speech patterns, understand context, and even detect emotions and subtleties in tone.
Such progress is not only improving the accuracy of speech recognition and transcription but also enabling more sophisticated applications like real-time sentiment analysis and automated conversational agents.
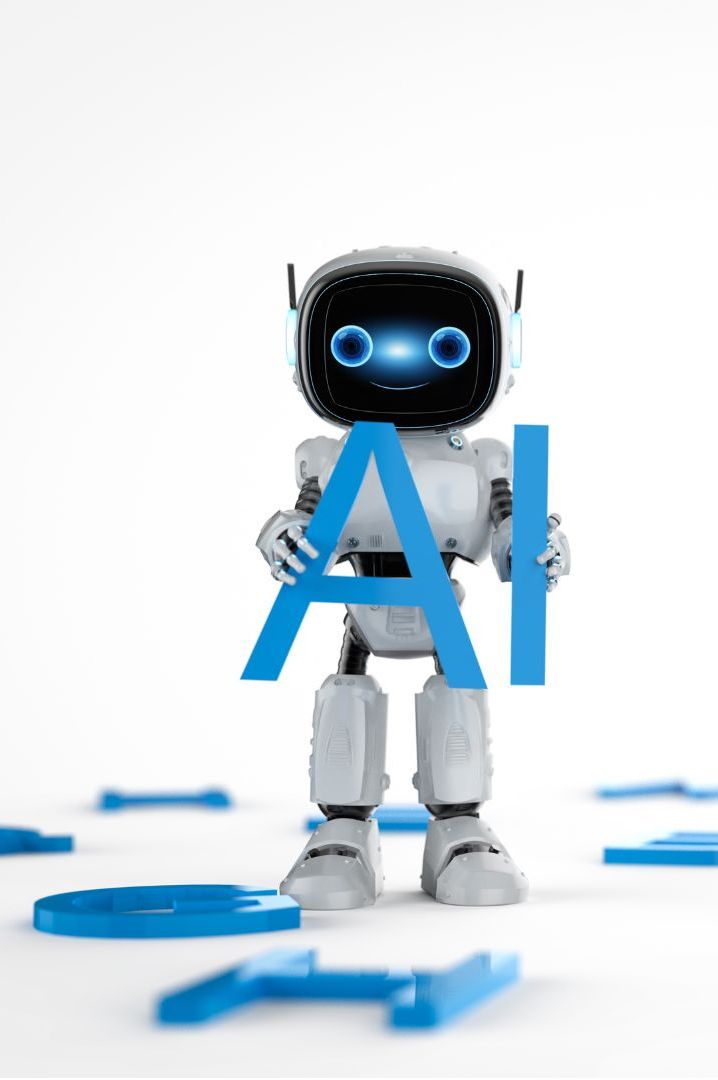
These technological advancements are driven by the increasing availability of large, diverse speech datasets and improvements in computational power and algorithms. As AI models become more refined and capable of handling the intricacies of human speech, the potential applications of speech data in NLP expand, offering more natural and intuitive ways for humans to interact with technology. The ongoing innovation in AI and machine learning for speech analysis promises to further bridge the gap between human communication and machine understanding, making AI-driven interactions more seamless and effective.
Integrating Speech Data with Other Data Types for Comprehensive Analysis
The synergy between speech, text, and other data forms in creating robust AI models.
The integration of speech data with other types of data, such as text, images, and biometric signals, is creating a more holistic approach to NLP, enabling comprehensive analysis and richer insights. This multimodal integration allows AI models to interpret human communication in a more nuanced manner, considering not just what is said but also how it is said, in conjunction with other contextual information.
For example, combining speech data with facial expression analysis can enhance emotion recognition systems, while merging speech and text data can improve the accuracy of language translation services.
This synergy between different data types enriches the AI’s understanding of human interactions, leading to more accurate, responsive, and empathetic AI systems. By leveraging the strengths of each data type, NLP technologies can achieve a deeper understanding of language and communication, opening up new possibilities for AI applications that are more aligned with human ways of interacting. The future of NLP lies in this multidimensional analysis, where the integration of diverse data types continues to enhance the capabilities and effectiveness of AI technologies.
Key Tips For Natural Language Processing (NLP)
- Quality over Quantity: Prioritise high-quality, diverse speech datasets for training NLP models.
- Privacy and Ethics: Always ensure speech data collection complies with privacy laws and ethical standards.
- Customisation is Key: Utilise custom speech datasets to address specific challenges and improve model performance.
- Continuous Improvement: Regularly update and refine speech datasets and models to adapt to new linguistic patterns.
Way With Words provides highly customised and appropriate data collections for speech and other use cases, crucial for technologies where AI language and speech are key developments.
Their services include:
- Creating speech datasets including transcripts for machine learning purposes, crucial for technologies aiming to create or improve existing automatic speech recognition models using NLP.
- Polishing machine transcripts across various technologies, enhancing machine learning models that use speech-to-text for AI research and various applications.
The integration of speech data into NLP tasks is a dynamic and challenging activity, offering immense potential to enhance how machines understand and interact with human language. As we’ve explored, speech data is vital in various NLP applications, from transcription and sentiment analysis to language translation. However, the effective use of speech data requires attention to quality, ethical collection practices, and ongoing customisation to meet specific needs.
With services like Way With Words, companies have access to specialised resources to collect and refine speech data, ensuring their AI and machine learning projects are not only innovative but also relevant and ethical. The key piece of advice for leveraging speech data in NLP is to remain adaptable, continuously seeking to improve data quality and model accuracy to keep pace with the evolving landscape of human-machine communication.
NLP Resources
Way With Words Speech Collection: We create speech datasets including transcripts for machine learning purposes. Our service is used for technologies looking to create or improve existing automatic speech recognition models (ASR) using natural language processing (NLP) for select languages and various domains.
Machine Transcription Polishing Service: We polish machine transcripts for clients across a number of different technologies. Our machine transcription polishing (MTP) service is used for a variety of AI and machine learning purposes. User applications include machine learning models that use speech-to-text for artificial intelligence research, FinTech/InsurTech, SaaS/Cloud Services, Call Centre Software and Voice Analytic services for the customer journey.
Tableu: 10 Great Natural Language Processing (NLP) Blogs to follow.