Can AI Improve Speech Recognition In Multilingual African Contexts?
How Can Artificial Intelligence be Leveraged to Improve Speech Recognition in Multilingual African Contexts?
One of the most promising and challenging areas of AI application is speech recognition, particularly within the rich tapestry of multilingual African contexts. With over 2,000 languages spoken across the continent, Africa presents a unique set of challenges and opportunities for AI-driven speech recognition technologies. The question then arises: How can AI be leveraged to navigate and embrace the linguistic diversity of the African continent to improve speech recognition technologies?
The complexity of African languages, coupled with their various dialects and sociolects, requires innovative approaches to data collection, model training, and algorithm development. The task is not only to recognise speech accurately but also to understand the nuances and variations inherent in each language and dialect. This calls for a multi-faceted strategy involving extensive data collection, deep learning techniques, and community engagement to ensure inclusivity and accuracy.
For AI researchers, developers, and technology entrepreneurs, addressing these challenges involves asking several key questions: How can we collect and curate diverse speech datasets that reflect the rich linguistic diversity of the continent? What machine learning models and algorithms are best suited to handle the multilingual and dialectal complexities of African languages? How can we ensure that these technologies are accessible and beneficial to all Africans, including those in remote or underserved communities?
By exploring these questions, we embark on a journey to unlock the potential of AI in transforming speech recognition across Africa. This not only advances technological innovation but also fosters social inclusion, economic development, and cultural preservation.
Thoughts On Collecting African Language Data
Data Collection and Curation Challenges
Collecting and curating datasets for African languages requires innovative approaches to capture the diverse linguistic landscape accurately. Solutions include crowdsourced data collection and partnerships with local communities and linguists.
The task of collecting and curating datasets for the multitude of African languages presents a unique set of challenges, necessitating innovative and inclusive approaches to accurately capture the continent’s diverse linguistic landscape. Traditional methods of data collection often fall short in the face of Africa’s linguistic diversity, which includes not only a vast number of languages but also a wide range of dialects and sociolects within those languages.
To overcome these hurdles, a combination of crowdsourced data collection and collaborations with local communities and linguists has emerged as a powerful strategy. Crowdsourcing allows for the gathering of linguistic data from a broad spectrum of speakers, ensuring a more representative and comprehensive dataset. Meanwhile, partnerships with local experts and linguists ensure the accuracy and relevance of the data collected, providing invaluable insights into the nuances of each language and dialect.
Furthermore, the process of curating these datasets involves not only the meticulous organisation and labelling of data but also the ethical considerations of consent and privacy. The involvement of local communities in the curation process not only helps in building trust and ensuring the respectful use of linguistic data but also empowers these communities by recognising and valuing their languages and dialects.
This approach not only enhances the quality and inclusivity of the datasets but also fosters a sense of ownership and pride among the contributors. As AI and speech recognition technologies continue to evolve, the need for diverse and accurately curated linguistic datasets becomes ever more critical, making the innovative methods of data collection and curation in African contexts a model for multilingual data handling worldwide.
Machine Learning Models for Multilingual African Contexts
Developing machine learning models that can handle the complexity of African languages involves leveraging advanced neural network architectures and transfer learning techniques to achieve higher accuracy levels.
Developing machine learning models capable of understanding and processing the complexity of African languages is a formidable task that requires leveraging the latest advancements in neural network architectures and transfer learning techniques.
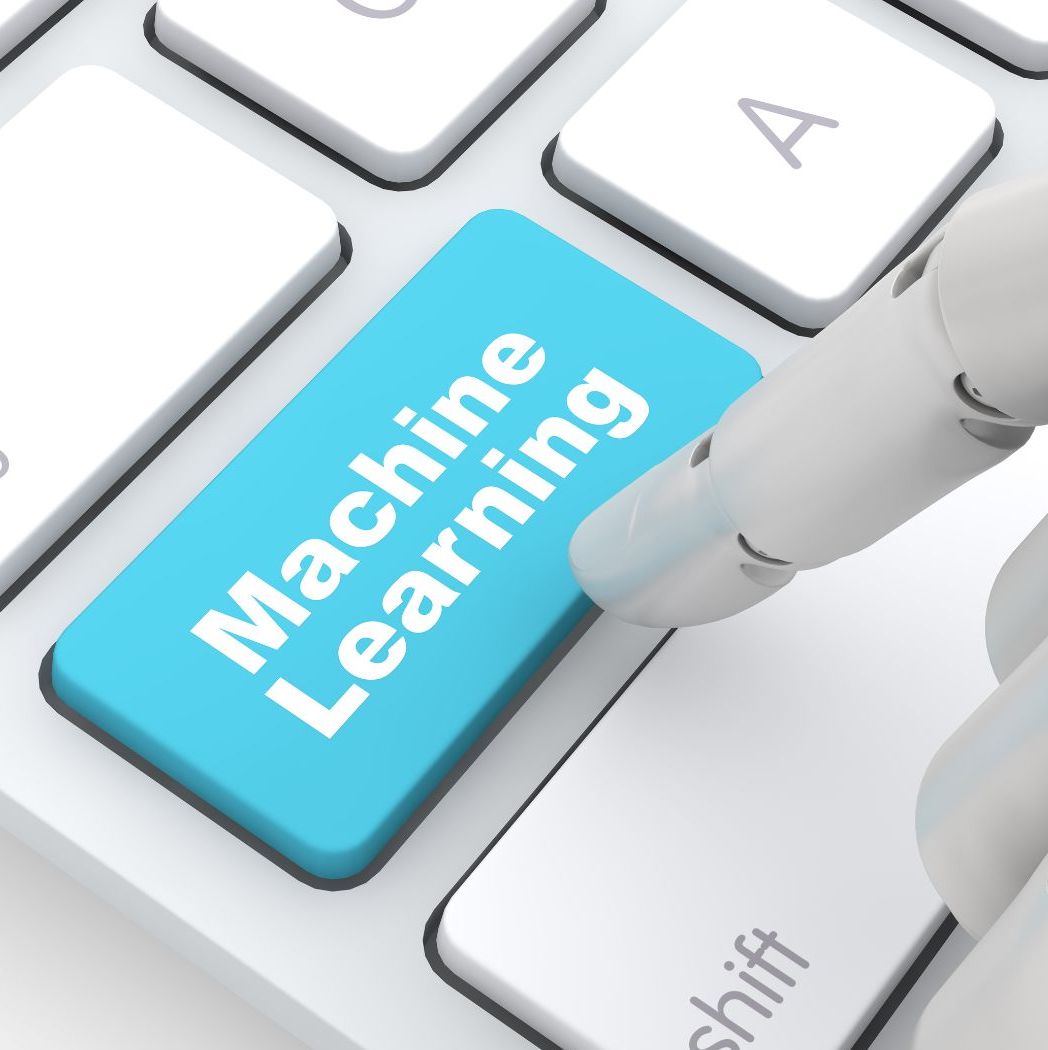
The intrinsic variability and nuanced differences between languages necessitate models that can generalise well across languages while being sensitive to the specificities of each. Advanced neural networks, particularly those based on deep learning, have shown significant promise in this regard, thanks to their ability to learn complex patterns and relationships within large datasets. These models, when trained on the diverse datasets representative of Africa’s linguistic landscape, can achieve remarkable levels of accuracy in speech recognition.
Transfer learning, on the other hand, offers a pragmatic approach to model development by allowing the reuse of pre-trained models on new languages with relatively minimal additional training. This technique is particularly valuable in contexts where data may be scarce or difficult to collect, as is often the case with many African languages.
By leveraging the knowledge gained from one language to aid in the understanding of another, transfer learning can significantly reduce the resources and time required to develop effective speech recognition models for multiple languages. This not only accelerates the deployment of speech recognition technologies across different linguistic contexts but also ensures that these technologies can be more easily adapted and refined as new data becomes available.
Dialect Recognition and Processing
Dialectal variations present a significant challenge for speech recognition. Techniques such as dialect identification and adaptive models can improve recognition accuracy across different dialects.
Dialectal variations pose one of the most significant challenges for speech recognition technologies, particularly in the African context where many languages boast a plethora of dialects. Traditional speech recognition models often struggle with dialectal nuances, leading to decreased accuracy and effectiveness. To address this issue, cutting-edge techniques such as dialect identification and adaptive models have been developed.
Dialect identification involves training models to recognise and differentiate between various dialects of the same language, allowing for more accurate processing of speech inputs. Once identified, adaptive models can adjust their processing parameters to better match the specific characteristics of the detected dialect, significantly improving recognition accuracy.
The development and implementation of these techniques require a deep understanding of the linguistic features that distinguish one dialect from another, underscoring the importance of interdisciplinary collaboration in this field. Linguists and dialectologists play a crucial role in identifying these distinguishing features, while data scientists and AI experts work to incorporate this knowledge into the models.
This collaborative approach not only enhances the technical capabilities of speech recognition technologies but also ensures that they are more inclusive and representative of the linguistic diversity found within and across African countries. By embracing the complexity of dialectal variations, AI can move closer to providing speech recognition solutions that are truly accessible and effective for all users, regardless of their linguistic background.
Technological Accessibility and Inclusion
Ensuring the accessibility of speech recognition technologies in multilingual African contexts means creating affordable, low-resource solutions that can be deployed on a wide range of devices and internet speeds.
Ensuring the accessibility and inclusivity of speech recognition technologies in multilingual African contexts is a multifaceted challenge that extends beyond the mere technical aspects of AI development. It involves creating affordable, low-resource solutions that can be deployed across a wide range of devices and internet speeds, making these technologies available to the broadest possible audience.
This necessitates not only the development of lightweight models that require minimal computational resources but also the creation of user-friendly interfaces that can accommodate various levels of digital literacy. Additionally, the deployment strategies must consider the infrastructural limitations present in many African communities, such as limited internet access and electricity.
The drive towards technological accessibility and inclusion also demands a concerted effort to understand and address the specific needs and preferences of different user groups. This involves engaging with potential users from a variety of backgrounds to gather insights into how speech recognition technologies can be tailored to better serve their needs.
For instance, applications in local languages for agriculture, education, and healthcare can have a profound impact on communities by providing valuable information and services in an accessible format. By prioritising the development of technologies that are not only linguistically inclusive but also accessible to users with varying levels of technological access and expertise, the field of AI can contribute significantly to bridging the digital divide in Africa and beyond.
Language Preservation and Cultural Representation
AI-driven speech recognition can play a crucial role in preserving endangered languages and dialects by creating digital records and facilitating language learning and documentation.
AI-driven speech recognition technologies offer an unprecedented opportunity for language preservation and cultural representation, particularly for the many endangered languages and dialects across Africa. By creating digital records of these languages, AI can help to document and preserve linguistic diversity that might otherwise be lost.
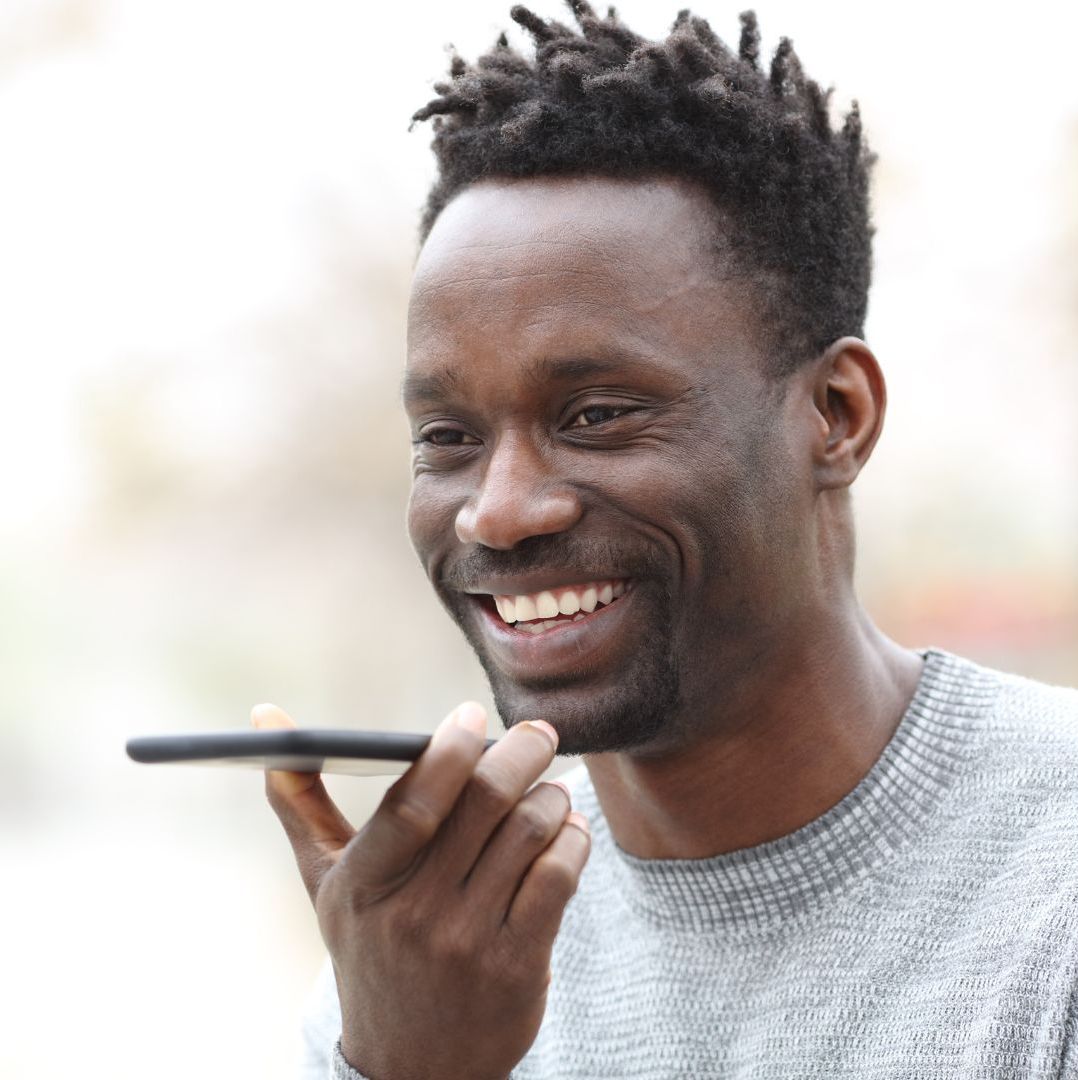
This not only serves as a resource for future generations but also supports ongoing efforts in language revitalisation and education. Digital records can facilitate language learning and documentation, providing a valuable tool for linguists, educators, and communities themselves to engage with and sustain their linguistic heritage.
Moreover, the inclusion of diverse languages and dialects in speech recognition technologies plays a crucial role in cultural representation. It acknowledges and validates the importance of linguistic diversity, empowering speakers of minority languages by providing them with technology that recognises and understands their speech.
This can have far-reaching implications for social inclusion, allowing for greater participation in the digital economy and access to digital services in one’s native language. By prioritising language preservation and cultural representation, AI developers can ensure that speech recognition technologies not only advance technological innovation but also contribute to the preservation of cultural identity and diversity.
Ethical Considerations and Bias Mitigation
Addressing ethical considerations involves ensuring data privacy, consent, and the mitigation of biases in speech recognition models to prevent discrimination against certain languages or dialects.
The development and deployment of speech recognition technologies in multilingual African contexts raise important ethical considerations, particularly regarding data privacy, consent, and the mitigation of biases. Ensuring the ethical use of linguistic data involves transparent data collection practices, where individuals are fully informed about how their data will be used and are given the option to consent or opt-out.
This is especially crucial in communities where digital literacy may be limited, requiring efforts to ensure that consent processes are accessible and understandable to all participants. Additionally, the protection of personal and linguistic data is paramount, necessitating robust security measures to prevent unauthorised access and ensure data integrity.
Bias mitigation is another critical ethical consideration, as biases in speech recognition models can lead to discriminatory outcomes against certain languages or dialects. This requires a deliberate and ongoing effort to ensure that datasets are diverse and representative, and that models are regularly evaluated and updated to address any biases that may arise.
Involving a diverse group of individuals in the development and testing processes can help identify and correct these biases, ensuring that speech recognition technologies are equitable and inclusive. By prioritising ethical considerations and bias mitigation, developers can build trust in AI technologies and ensure that they serve the needs and interests of all users, regardless of their linguistic background.
Community Engagement and Co-Creation
Engaging with local communities in the development of speech recognition technologies ensures that these tools are culturally sensitive, linguistically accurate, and aligned with users’ needs.
Community engagement and co-creation are essential strategies for developing speech recognition technologies that are culturally sensitive, linguistically accurate, and aligned with users’ needs. By involving local communities in the development process, developers can gain invaluable insights into the linguistic nuances, cultural contexts, and practical needs that should inform the design and functionality of these technologies. This collaborative approach not only enhances the relevance and usability of speech recognition tools but also fosters a sense of ownership and acceptance among community members, increasing the likelihood of successful adoption and use.
Co-creation workshops, participatory design sessions, and continuous feedback mechanisms are effective ways to engage communities in the development process. These activities provide platforms for users to express their preferences, concerns, and ideas, directly influencing the direction and outcomes of technology development projects.
Moreover, community engagement and co-creation can also facilitate capacity building, as community members acquire new skills and knowledge related to technology development and application. This not only contributes to the immediate goals of creating effective speech recognition solutions but also empowers communities, enhancing their ability to participate in and benefit from technological advancements.
Interdisciplinary Collaboration
Collaboration between technologists, linguists, anthropologists, and local communities is essential to address the multifaceted challenges of multilingual speech recognition in Africa.
Addressing the multifaceted challenges of multilingual speech recognition in Africa requires interdisciplinary collaboration, bringing together experts from technology, linguistics, anthropology, and beyond. This collaborative approach enables a holistic understanding of the linguistic, cultural, and technical aspects involved in developing effective speech recognition technologies.
Technologists and AI developers bring expertise in machine learning models and algorithm development, while linguists and anthropologists provide insights into the linguistic structures, dialectal variations, and cultural contexts of African languages. Together, these diverse perspectives can inform the development of speech recognition models that are not only technically sophisticated but also culturally and linguistically nuanced.
Interdisciplinary collaboration also fosters innovation, as ideas and methods from different fields intersect and inspire new approaches to complex problems. For example, anthropological insights into communication practices and social norms can inform the design of user interfaces and interaction models, making them more intuitive and accessible for users from diverse cultural backgrounds.
Similarly, linguistic research can guide the development of algorithms that better capture the subtleties of language, improving the accuracy and effectiveness of speech recognition technologies. By embracing interdisciplinary collaboration, the field of AI can achieve breakthroughs that are not only technologically advanced but also deeply rooted in the social and cultural fabric of African societies.
Scaling Solutions Across Languages
Strategies for scaling speech recognition solutions involve modular architectures and language-agnostic models that can be easily adapted to new languages and dialects.
Developing speech recognition solutions that can be scaled across the diverse linguistic landscape of Africa presents both a challenge and an opportunity. Strategies for scaling involve creating modular architectures and language-agnostic models that can be easily adapted to new languages and dialects with minimal additional development effort.

This requires a foundational understanding of the commonalities and differences among languages and the ability to abstract these into models that can accommodate linguistic diversity. Modular architectures allow for components of the speech recognition system to be updated or replaced as needed, facilitating the incorporation of new languages or dialects without having to redesign the entire system.
Language-agnostic models, on the other hand, are designed to learn universal linguistic features that transcend individual languages, making them capable of processing speech from a wide range of languages with relatively little customisation. This approach leverages the power of machine learning to create flexible and adaptable speech recognition technologies that can grow and evolve alongside linguistic research and data collection efforts.
By focusing on scalability, developers can ensure that speech recognition technologies remain accessible and relevant to a wide audience, supporting linguistic diversity and inclusivity. This not only broadens the impact of these technologies but also paves the way for their application in a variety of contexts, from education and healthcare to commerce and entertainment, enriching the lives of people across Africa and beyond.
Innovative Applications and Use Cases
Exploring innovative applications of speech recognition in healthcare, education, finance, and agriculture can drive social and economic benefits across the continent.
Scaling speech recognition solutions across the diverse linguistic landscape of Africa requires innovative approaches that can accommodate the continent’s vast array of languages and dialects. Modular architectures and language-agnostic models stand out as effective strategies for achieving this scalability. Modular architectures, in particular, allow for the flexible design of speech recognition systems wherein various components can be independently developed, tested, and integrated.
This modularity is crucial for adapting to new languages because it enables specific components, such as language models or acoustic models, to be updated or replaced without the need for overhauling the entire system. This approach not only accelerates the process of adapting to new languages but also facilitates continuous improvement as better models or data become available.
Language-agnostic models take scalability a step further by focusing on the development of universal models that are not tied to any specific language. These models leverage the underlying similarities across languages, such as phonetic patterns or grammatical structures, to create a foundation that is broadly applicable. By focusing on these universal aspects of language, developers can create systems that require minimal adjustments to work with a new language or dialect.
This approach is particularly powerful in the African context, where many languages may have limited datasets available. Language-agnostic models can be initially trained on languages with ample data and then fine-tuned with smaller datasets from less-represented languages, significantly reducing the barriers to entry for developing speech recognition capabilities in these languages.
The challenge of scaling speech recognition technologies across languages also presents an opportunity to innovate in the way we approach language data collection and model training. For instance, unsupervised learning techniques and semi-supervised learning can play pivotal roles in leveraging unlabelled data, which is abundant for many African languages.
These techniques can extract valuable insights from unlabelled audio recordings, gradually improving the model’s understanding of a new language’s phonetic and syntactic characteristics without the need for extensive manually annotated datasets. Furthermore, the use of transfer learning, where a model trained on one task is adapted for another, can facilitate the rapid deployment of speech recognition technologies across languages with similar characteristics, thereby streamlining the scaling process.
Moreover, the implementation of scalable speech recognition solutions necessitates a collaborative and inclusive approach that involves linguists, technologists, and local communities. This collaboration ensures that the development of language models takes into account not just the linguistic aspects but also the cultural and contextual nuances that are essential for accurate speech recognition.
Engaging with local communities not only aids in the collection of diverse and representative data but also ensures that the technologies developed are aligned with the needs and preferences of the end-users. This user-centric approach to technology development is crucial for the successful adoption and utilisation of speech recognition technologies in various applications, from education and healthcare to business and entertainment.
In conclusion, scaling speech recognition solutions across the diverse languages of Africa requires a multifaceted approach that embraces technological innovation, collaborative development, and user-centric design. Modular architectures and language-agnostic models offer promising pathways toward achieving this goal, enabling the rapid adaptation of technologies to new languages and dialects.
By leveraging these strategies in conjunction with advanced machine learning techniques and inclusive development practices, we can create speech recognition technologies that are not only linguistically comprehensive but also culturally sensitive and broadly accessible. This effort not only advances the field of artificial intelligence but also plays a critical role in bridging linguistic divides, fostering inclusivity, and empowering communities across Africa and beyond.
Key Tips For Collecting For Multilingual Speech Recognition For Africa
- Focus on comprehensive data collection that reflects the linguistic diversity of the continent.
- Leverage advanced AI and machine learning techniques to handle multilingual and dialectal complexities.
- Prioritise accessibility and inclusivity to ensure technologies benefit all Africans.
- Engage with local communities and experts in the development process to ensure cultural sensitivity and accuracy.
- Mitigate biases and address ethical considerations to foster trust and adoption.
- Explore interdisciplinary collaborations to tackle the multifaceted challenges of speech recognition.
- Investigate innovative applications that can drive social and economic benefits.
Way With Words provides highly customised and appropriate speech data collections for African languages, addressing key challenges in developing accurate and inclusive speech recognition technologies.
Leveraging artificial intelligence to improve speech recognition in multilingual African contexts presents a unique set of challenges and opportunities. It requires a holistic approach that encompasses data collection, machine learning, ethical considerations, and community engagement. By addressing these areas, we can develop speech recognition technologies that are not only technologically advanced but also culturally sensitive and inclusive.
The journey towards achieving effective multilingual speech recognition in Africa is complex, requiring the collaboration of AI researchers, developers, linguists, and local communities. It is an endeavour that goes beyond mere technological innovation, touching on issues of language preservation, cultural representation, and social inclusion.
A key piece of advice for those embarking on this journey is to maintain a focus on inclusivity and accessibility. By ensuring that speech recognition technologies are accessible to all, regardless of linguistic background or geographical location, we can unlock the full potential of AI to serve the diverse needs of the African continent. The path to improving speech recognition in multilingual African contexts is paved with challenges but also brimming with possibilities. By leveraging AI in thoughtful, inclusive, and innovative ways, we can create technologies that not only understand the myriad of languages spoken across Africa but also empower its people in unprecedented ways.
ASR Resources
African Language Speech Collection Solution: “We create custom speech datasets for African languages, including transcripts for machine learning purposes. Our service is used for technologies looking to create or improve existing automatic speech recognition models (ASR) using natural language processing (NLP) for select African languages and various domains.”
Machine Transcription Polishing of Captured Speech Data: “We polish machine transcripts for clients across a number of different technologies. Our machine transcription polishing (MTP) service is used for a variety of AI and machine learning purposes that are intended to be applied in various African languages.”
Forum on Artificial Intelligence in Africa (UNESCO): Knowledge, and Artificial intelligence in Africa.