Machine Learning Models for Speech Recognition in Low-resource African Languages
How are Machine Learning Models Trained for Speech Recognition in Low-resource African Languages?
When it comes to low-resource African languages, the development of machine learning models faces unique challenges. These languages, rich in diversity and cultural significance, often lack the voluminous datasets necessary for traditional model training, presenting a hurdle for data scientists and AI researchers. How can we effectively gather and utilise data for these languages?
What methodologies are proving successful, and what obstacles do developers face in this arena? This article explores the intricacies of training machine learning models for speech recognition in low-resource African languages, offering insights for technology entrepreneurs, software developers, and industries looking to harness AI to improve speech recognition capabilities.
Key Indicators for African Machine Learning Models For Speech Collection
Challenges in Data Collection
Limited availability of annotated speech data. The importance of culturally sensitive and diverse data collection methods.
The journey to harnessing speech recognition capabilities for low-resource African languages begins with the formidable challenge of data collection. Annotated speech data, the lifeblood of machine learning model training, is notoriously scarce for these languages. This scarcity isn’t just a matter of volume but also of diversity and quality. Collecting speech data that accurately represents the myriad dialects, accents, and linguistic nuances of African languages demands a nuanced approach. Moreover, the process of annotation—tagging data with the correct transcriptions or phonetic indicators—is labour-intensive and requires a deep understanding of the language, further complicating the data collection process.
Additionally, the cultural sensitivity and diversity of data collection methods cannot be overstated. Traditional data collection methods often overlook the cultural nuances and social dynamics unique to different African communities. This oversight can lead to datasets that are not only incomplete but also culturally biased, impacting the effectiveness and fairness of the resulting speech recognition models. Culturally sensitive methods involve engaging with local communities in a respectful and inclusive manner, ensuring that data collection accounts for regional dialects, sociolects, and idiolects, and reflects the rich linguistic landscape of the continent. This approach not only improves the quality and diversity of the data collected but also fosters trust and cooperation between technology developers and local communities.
Innovative Data Gathering Techniques
Crowdsourcing through mobile apps and community engagement. Partnerships with local institutions and organisations.
In the quest to overcome the hurdles of data scarcity and diversity for low-resource African languages, innovative data gathering techniques have emerged as a beacon of hope. Crowdsourcing, facilitated by mobile apps and community engagement, presents a scalable and cost-effective method for data collection. Mobile apps, with their wide reach and accessibility, offer a direct channel to engage speakers of various African languages, inviting them to contribute their voices to a growing database.
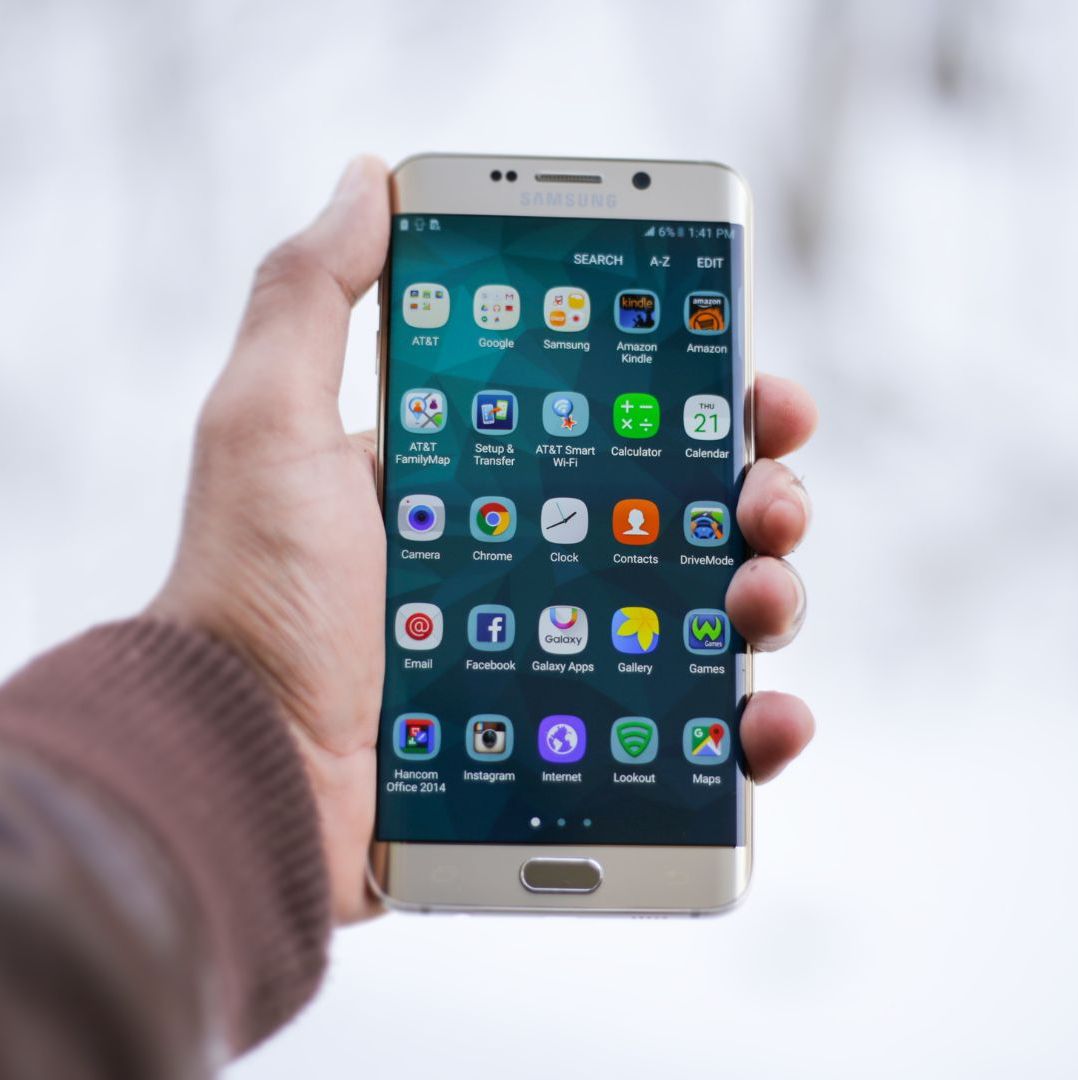
This method democratises the data collection process, allowing for a more representative and inclusive dataset that captures a wide range of dialects, accents, and linguistic nuances. Moreover, community engagement initiatives, such as local workshops or collaborations with educational institutions, further enrich the data pool by incorporating the voices of those who may not have access to technology.
Partnerships with local institutions and organisations play a critical role in this innovative approach to data collection. By collaborating with universities, language research centres, and cultural institutions, AI researchers can tap into existing networks of linguistic experts and communities eager to see their languages represented in the digital age.
These partnerships not only provide access to valuable linguistic resources and expertise but also ensure that data collection efforts are aligned with the needs and values of the communities they aim to serve. Furthermore, such collaborations can foster a sense of ownership and pride among community members, encouraging their active participation in the development of technologies that recognise and value their languages and cultures.
Transfer Learning and Its Benefits
Leveraging data from linguistically similar languages. Reducing the need for large datasets.
Transfer learning has emerged as a powerful tool in the training of machine learning models for speech recognition in low-resource African languages, offering a path to surmount the obstacles of limited data availability. By leveraging the linguistic similarities between well-documented languages and those considered low-resource, transfer learning allows developers to bootstrap new models with pre-existing knowledge. This approach significantly reduces the need for extensive datasets specific to each language, accelerating the development process and making it more feasible to include a broader range of languages in speech recognition technologies.
The benefits of transfer learning extend beyond mere efficiency and practicality. It embodies a more inclusive and equitable approach to AI development, where linguistic diversity is acknowledged and celebrated. By transferring knowledge from linguistically similar languages, researchers can create more robust models that better capture the unique characteristics of each language, including its phonetics, syntax, and semantic nuances.
This method not only enhances the accuracy and reliability of speech recognition models but also paves the way for more nuanced and culturally sensitive AI applications. As a result, transfer learning not only addresses the technical challenges of machine learning model training but also contributes to the preservation and promotion of linguistic diversity on the African continent.
Synthetic Data Generation
Using text-to-speech technology to augment datasets. The role of synthetic voices in training models.
The generation of synthetic data through text-to-speech (TTS) technology offers a novel solution to the challenge of data scarcity in training speech recognition models for low-resource African languages. This approach involves creating artificial speech samples from textual data, which can then be used to augment existing datasets. Synthetic data generation enables developers to simulate a wide range of linguistic features, including different dialects and accents, providing a more comprehensive training ground for machine learning models. Furthermore, this technique allows for the creation of data that specifically targets gaps or weaknesses in the model, improving its overall performance and robustness.
The role of synthetic voices in training models is twofold. Firstly, they provide a valuable resource for testing and refining the models’ ability to recognise and understand diverse speech patterns. This is particularly important for languages with significant regional variation, where access to authentic speech samples may be limited. Secondly, synthetic voices can aid in the development of more inclusive and accessible technologies.
By ensuring that speech recognition systems are trained on a diverse set of voices, developers can create more equitable AI tools that serve the needs of a wider range of users. However, the use of synthetic data is not without its challenges. Ensuring the naturalness and authenticity of synthetic voices is crucial for the effectiveness of the training process, requiring ongoing innovation and refinement in TTS technologies.
Importance of Dialect and Accent Recognition
Tailoring models to understand regional variations. Enhancing model accuracy through diverse speech samples.
Recognising the importance of dialect and accent recognition in speech recognition systems is critical for developing technologies that accurately serve the diverse African continent. Dialects and accents are not mere variations in speech; they are integral to the identity and culture of the speakers. Traditional speech recognition models often struggle with these variations, leading to inaccuracies and biases in voice-driven technologies.
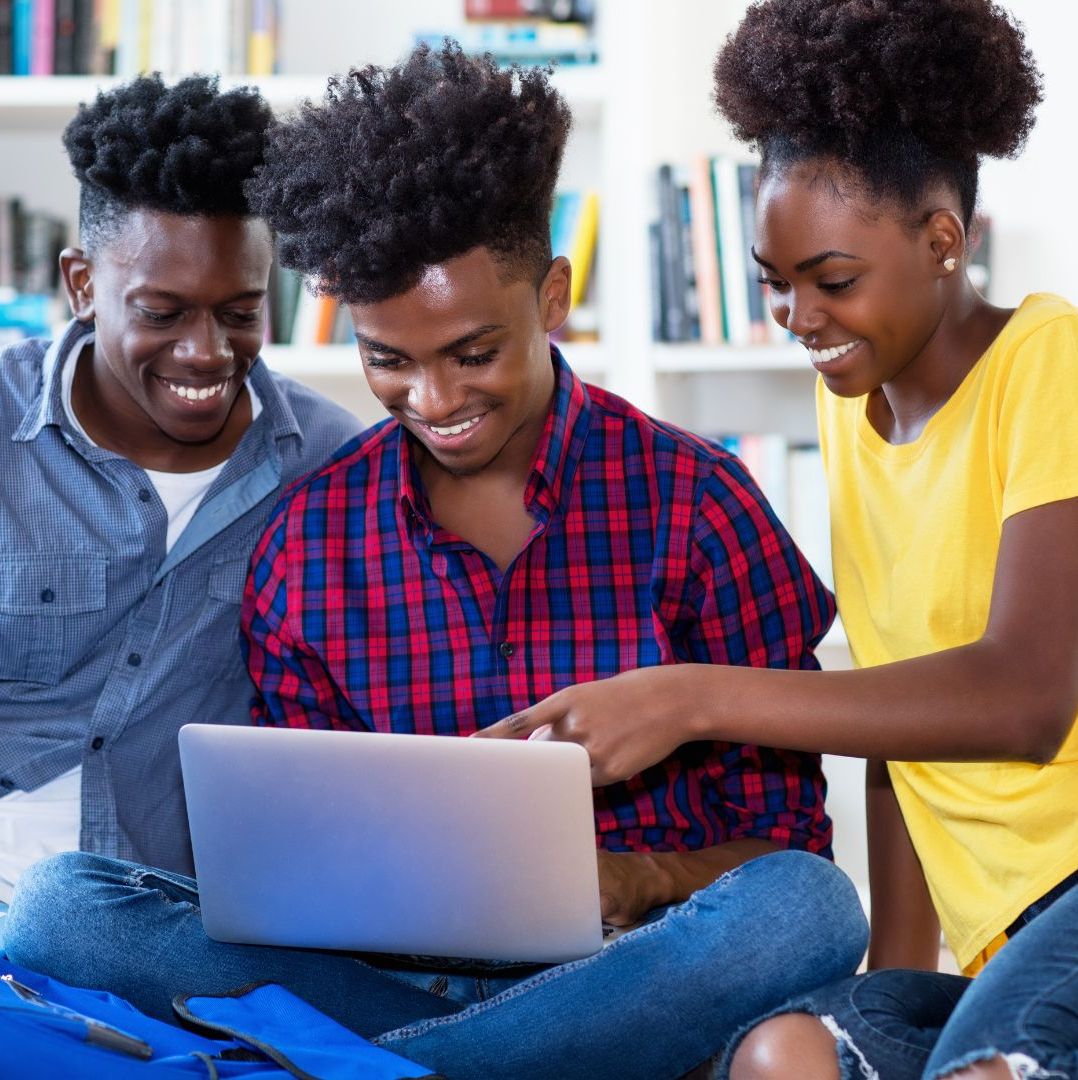
Tailoring models to understand these regional variations is not just a technical challenge but a commitment to inclusivity and fairness. By incorporating diverse speech samples into the training process, developers can enhance the models’ ability to accurately interpret and respond to a wide range of speech patterns, thereby improving user experience and accessibility.
The enhancement of model accuracy through diverse speech samples extends beyond mere technical improvement; it represents a step towards democratising technology. When speech recognition systems can accurately understand and process speech from all users, regardless of their dialect or accent, it breaks down barriers to technology adoption and empowers individuals with technology that understands them.
This is particularly important in Africa, where the linguistic landscape is incredibly diverse. Successfully tackling the challenge of dialect and accent recognition not only improves the functionality of speech recognition systems but also affirms the value of linguistic diversity, promoting a more inclusive digital future.
Collaborative Efforts and Open Source
The impact of shared resources on accelerating development. Examples of successful community-driven projects.
Collaborative efforts and open-source initiatives represent a fundamental shift in how technology, especially in the field of AI and machine learning, is developed and deployed. The open-source model, predicated on the idea of making software freely available for anyone to use, modify, and distribute, has proven especially beneficial for advancing speech recognition technologies in low-resource African languages. These collaborative efforts have the power to accelerate development by pooling resources, expertise, and data, thus overcoming some of the significant barriers faced by individual researchers or small teams.
By sharing code, algorithms, and datasets, developers can build upon each other’s work, fostering innovation and speeding up the process of refining and deploying sophisticated models. This ecosystem of shared knowledge not only democratises access to technology but also encourages a culture of transparency and cooperation, which is crucial for addressing the complex challenges of language diversity.
One notable example of a successful community-driven project is Mozilla’s Common Voice, an initiative aimed at creating publicly available voice datasets for use in machine learning. By inviting people from around the globe to donate their voices, Common Voice has amassed a vast and diverse collection of speech data, including for several African languages.
This approach not only enriches the available data for underrepresented languages but also exemplifies the potential of collaborative efforts to make significant strides in technology development. Such projects underscore the importance of collective action in the AI field, where the sharing of resources and expertise can lead to breakthroughs that might not be possible through isolated efforts. As these collaborative projects continue to grow, they pave the way for more inclusive and effective speech recognition technologies that better serve the needs of diverse populations.
Ethical Considerations and Privacy
Ensuring consent and protecting speaker identities. Addressing biases in speech recognition systems.
Ethical considerations and privacy concerns are at the forefront of developing AI technologies, particularly in the realm of speech recognition. Ensuring consent and protecting speaker identities are critical challenges that developers must navigate to maintain trust and integrity in their projects. In the context of low-resource African languages, where cultural sensitivities and the risk of exploitation are significant concerns, these issues become even more pronounced. Ethical data collection practices must be established, ensuring that participants are fully informed about how their data will be used and that their participation is voluntary and confidential.
This commitment to ethical standards not only protects individuals but also builds community trust, which is essential for the successful collection and use of speech data. Moreover, addressing biases in speech recognition systems is another ethical imperative. Historical data collection and model training practices have often overlooked minority languages and dialects, leading to systems that perform poorly for speakers of these languages. Developers must actively work to identify and mitigate these biases, ensuring that speech recognition technologies offer equitable access and benefits to all users.
Privacy concerns, particularly regarding the collection and use of speech data, are increasingly prominent in the public discourse. With the advent of AI technologies capable of processing and interpreting human speech, the potential for misuse of sensitive information has become a significant concern. Developers must employ robust data protection measures, anonymise personal information, and ensure that data storage and processing practices comply with local and international privacy laws.
Additionally, the development of speech recognition models should involve ongoing dialogue with communities and stakeholders to address privacy concerns proactively. By prioritising ethical considerations and privacy, developers can create speech recognition technologies that are not only more accurate and inclusive but also respectful of the rights and dignity of all individuals.
Technological Infrastructure and Accessibility
The role of mobile technology in data collection and model deployment. Challenges related to internet connectivity and digital literacy.
The role of mobile technology in data collection and model deployment for speech recognition in low-resource African languages is both transformative and challenging. Mobile devices, with their widespread adoption across Africa, offer a unique platform for engaging communities in data collection efforts and deploying speech recognition technologies directly to end-users. This accessibility has the potential to dramatically increase the volume and diversity of speech data available for training models, as well as to democratise access to AI-driven applications.
Mobile technology enables individuals in even the most remote areas to contribute their voices to datasets and to benefit from advances in speech recognition, thus bridging the digital divide. However, challenges related to internet connectivity and digital literacy remain significant barriers. In many regions, unreliable or expensive internet access limits the ability of individuals to participate in data collection initiatives or to access speech recognition services. Additionally, varying levels of digital literacy can affect the usability and adoption of these technologies, necessitating user-friendly design and educational outreach to ensure broad accessibility.
To overcome these obstacles, developers and researchers must innovate in the ways they collect data and deploy technologies. For instance, offline functionality and data compression techniques can make apps more accessible in areas with limited internet connectivity. Similarly, incorporating intuitive interfaces and leveraging local languages and symbols can improve digital literacy barriers.
Furthermore, partnerships with local telecommunications companies and educational institutions can facilitate greater access and awareness. By addressing these infrastructure and accessibility challenges, the potential of mobile technology to revolutionise speech recognition for African languages can be fully realised, making these powerful tools available to a broader segment of the population.
Machine Learning Techniques and Algorithms
Overview of models and algorithms best suited for low-resource languages. Advances in neural network architectures and unsupervised learning.
The advancement of machine learning techniques and algorithms has been pivotal in the development of speech recognition models for low-resource languages, including African dialects. Neural network architectures, particularly deep learning models, have shown remarkable success in understanding and processing natural language.
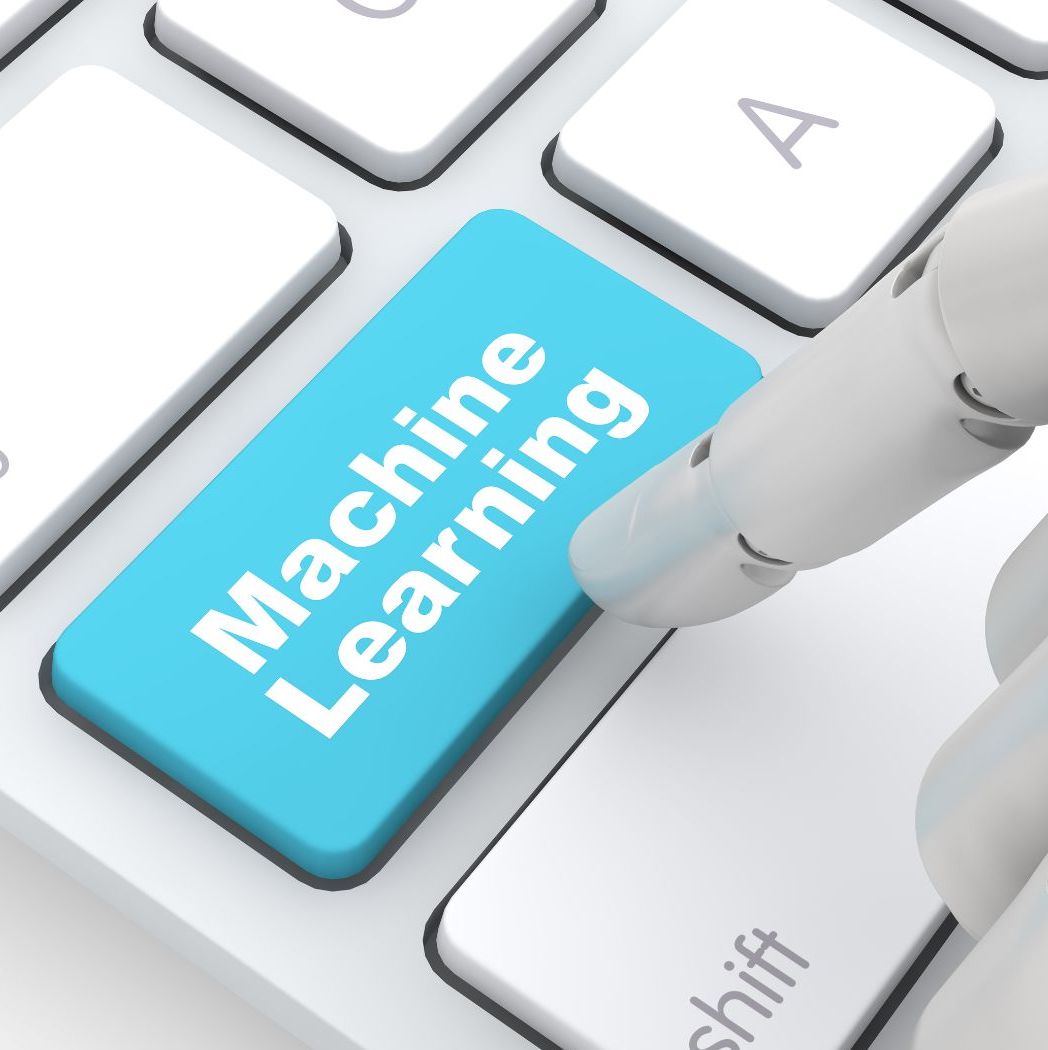
For languages with limited datasets, the choice of models and algorithms becomes crucial. Techniques such as unsupervised learning, which does not require labelled data, and semi-supervised learning, which uses a small amount of labelled data alongside a larger amount of unlabelled data, are particularly relevant. These approaches can leverage the intrinsic structures of language to learn from minimal data, making them well-suited for low-resource language processing.
Additionally, advances in neural network architectures, like recurrent neural networks (RNNs) and convolutional neural networks (CNNs), have been adapted to better handle the sequential and hierarchical nature of language, improving model performance even with limited training data.
Transfer learning, as previously mentioned, has also become a cornerstone technique, allowing models trained on high-resource languages to be adapted for use with low-resource languages. This method reduces the need for extensive annotated datasets by transferring knowledge from one language to another, leveraging linguistic similarities.
The continuous improvement of these algorithms, coupled with innovations in unsupervised and transfer learning, is breaking down barriers to developing effective speech recognition systems for low-resource languages. As researchers refine these techniques and develop new algorithms, the potential for creating inclusive, efficient, and accurate speech recognition models for every language spoken across the African continent becomes increasingly tangible.
Case Studies and Success Stories
Highlighting projects and organisations making strides in this field. Lessons learned and best practices.
Highlighting case studies and success stories provides concrete examples of progress in speech recognition for low-resource African languages, offering valuable lessons and best practices. One notable success story is the Masakhane project, a pan-African effort to build natural language processing (NLP) models for African languages.
By bringing together researchers, developers, and language experts from across the continent, Masakhane has fostered a collaborative environment that has led to significant advancements in NLP and speech recognition for dozens of African languages. This community-driven approach emphasises the importance of inclusivity, collaboration, and open access to data and models, principles that are crucial for success in this field.
Another inspiring example is the work done by AI4D Africa, which aims to promote AI development that benefits African societies. Through their support of research and development projects, AI4D Africa has facilitated the creation of speech recognition models and datasets for several African languages, demonstrating the potential of targeted investment and support for innovation in this area.
These case studies exemplify how collaborative efforts, community engagement, and targeted support can overcome the challenges of data scarcity, linguistic diversity, and technological barriers. They serve as a beacon of hope and a blueprint for future projects aiming to unlock the full potential of speech recognition technologies for all African languages.
Key ML African Language Collection Tips
-
Prioritise the collection of diverse and culturally relevant speech data.
-
Explore innovative data collection methods, including crowdsourcing and synthetic data generation.
-
Utilise transfer learning to make the most of available data.
-
Ensure models are trained to recognise dialects and accents.
-
Leverage collaborative and open-source efforts to enhance model development.
-
Address ethical considerations and privacy concerns from the outset.
-
Way With Words provides highly customised and appropriate speech data collections for African languages, aiding technologies in creating or improving ASR models using NLP for various domains.
Training machine learning models for speech recognition in low-resource African languages presents a unique set of challenges but also opens up a world of opportunities. The key to success lies in innovative data collection, leveraging existing resources through transfer learning, and ensuring models are inclusive of the rich diversity found within African dialects and accents. Collaboration, both locally and globally, alongside a strong ethical framework, can accelerate progress in this field.
For technology entrepreneurs, software developers, and industries looking to advance their machine learning capabilities, understanding these intricacies is crucial. As we move forward, the efforts of companies like Way With Words, with their specialised services in speech data collection and transcription polishing for African languages, become invaluable. Embracing these challenges and opportunities can lead to the development of more inclusive, effective, and accessible speech recognition technologies that truly represent the diversity of human language.
African Speech Data Machine Learning Resources
African Language Speech Collection Solution: “We create custom speech datasets for African languages including transcripts for machine learning purposes. Our service is used for technologies looking to create or improve existing automatic speech recognition models (ASR) using natural language processing (NLP) for select African languages and various domains.”
Machine Transcription Polishing of Captured Speech Data: “We polish machine transcripts for clients across a number of different technologies. Our machine transcription polishing (MTP) service is used for a variety of AI and machine learning purposes that are intended to be applied in various African languages. User applications include machine learning models that use speech-to-text for artificial intelligence research, FinTech/InsurTech, SaaS/Cloud Services, Call Centre Software and Voice Analytic services for the customer journey.”
Speech recognition datasets for low-resource Congolese languages: Advanced Automatic Speech Recognition (ASR) models have improved handling of languages with fewer resources, thanks to more available data and transfer learning benefits. Yet, few languages have enough resources to make full use of these advantages. Benchmark datasets are essential in such situations. This article presents two new benchmarks for under-resourced languages in the Democratic Republic of the Congo: the Lingala Read Speech Corpus, featuring 4 hours of labelled audio, and the Congolese Speech Radio Corpus, with 741 hours of unlabelled audio across four key languages of the area.