Identifying Under-resourced African Languages and Taking Action
Which African Languages Have the Least Data Available for Speech Recognition Technologies, Impact, and What to Do?
Speech recognition technology has emerged as a cornerstone of innovation, enabling machines to understand and respond to human speech with increasing accuracy. However, while strides have been made in developing speech recognition technologies for widely spoken languages, numerous African languages remain significantly under-resourced. This discrepancy poses a critical question: Which African languages have the least data available for speech recognition technologies, and why is this the case?
Addressing this question is crucial for data scientists, technology entrepreneurs, software developers, and industries invested in advancing AI capabilities, particularly in regions where these languages are spoken. By identifying languages with scant data, researchers, NGOs, and stakeholders can prioritise efforts to collect and analyse speech data, thereby fostering more inclusive technology that serves diverse linguistic communities.
Key questions to consider regarding African language data scarcity include:
- What factors contribute to the scarcity of data for certain African languages?
- How does this data gap affect the development and implementation of AI technologies in African regions?
- What strategies can be employed to enhance data collection and improve speech recognition capabilities for under-resourced African languages?
Identifying African Languages That Are Under-resourced
Identifying African languages with the least data available for speech recognition technologies involves considering the overall support for these languages in the digital domain, including the availability of digital resources, corpora for training models, and active development efforts. Africa is home to a vast linguistic diversity, with over 2,000 languages spoken across the continent. Many of these languages are underrepresented in digital technologies, including speech recognition, due to a variety of factors such as limited financial resources, low digital literacy rates, and the prioritisation of more widely spoken languages.
Languages with the least data available for speech recognition technologies typically include those with smaller speaker populations, those spoken in more remote areas, or those that have not been a focus of academic or commercial research efforts. Here are some characteristics of languages that might have limited data available:
- Less Documented Languages: Languages that have limited written documentation and resources, making it challenging to develop the necessary corpora for speech recognition technologies.
- Minority Languages: Languages spoken by small communities that might not be recognised or supported at a national or international level.
- Languages without Standardised Orthographies: Languages that lack a standardised writing system, which complicates the development of text-based resources necessary for training speech recognition systems.
- Languages in Remote Areas: Languages spoken in geographically isolated regions may have less exposure to technological development efforts.
- Endangered Languages: Languages that are at risk of falling out of use as their speakers shift to more dominant languages. These languages are less likely to have significant resources devoted to their digitisation.
Examples of African languages that might fall into these categories, and thus potentially have the least data available for speech recognition technologies, include:
- Lesser-known Khoisan languages of Southern Africa, known for their unique click consonants but spoken by very small communities.
- Minority languages in the Niger-Congo family, such as some of the Bantu languages spoken in remote areas.
- Languages of the Sahel spoken by nomadic communities, which might be underrepresented in digital resources.
- Endangered languages in various parts of Africa, such as some of the languages spoken by Pygmy communities in Central Africa.
For precise information about specific languages and the state of speech recognition support for them, detailed research and inquiries within linguistic and technological research communities focusing on African languages would be necessary. This might involve reviewing academic publications, technology reports, and initiatives by organisations working on language preservation and digital inclusion.
Under-resourced African Languages – Impact, Some Actions
Overview of African Languages and Technology
The African continent is home to over 2,000 distinct languages, each with unique linguistic features. The digital representation of these languages, particularly in AI and speech recognition technologies, is uneven, highlighting the need for targeted data collection efforts.
The African continent, with its rich tapestry of over 2,000 distinct languages, presents a unique and complex linguistic landscape. Each of these languages, from the widely spoken Swahili and Hausa to the less known indigenous tongues, carries its own set of phonetic, morphological, and syntactic characteristics that contribute to the diversity of human communication.
Despite this linguistic wealth, the representation of African languages in the digital sphere, especially in areas such as artificial intelligence (AI) and speech recognition technologies, is markedly uneven. This disparity underscores a significant challenge in the technological advancement of the continent: the urgent need for targeted data collection efforts to ensure that AI technologies can understand and interact in these local languages.
The digital gap in speech recognition technologies for African languages is not just a matter of technological oversight but a reflection of broader issues related to digital inclusion and equity. As the world moves increasingly towards digitalisation, the ability of technologies to cater to the vast array of African languages becomes not only a question of technological capability but also of cultural preservation and accessibility.
The uneven digital representation of African languages highlights a critical area for development, where targeted efforts in data collection and analysis can lead to more inclusive technologies that reflect the continent’s linguistic diversity. This endeavour is crucial for enabling equitable access to digital resources and services, fostering socio-economic development, and preserving cultural heritage in the digital age.
Challenges in Data Collection
Collecting speech data for African languages faces several obstacles, including limited access to technology, diverse dialects, and socio-political factors. These challenges contribute to the scarcity of data essential for building effective speech recognition systems.
Collecting speech data for African languages is fraught with challenges that go beyond the technical aspects of recording and annotation. Limited access to technology in many parts of the continent means that large segments of the population do not have the means to contribute to or benefit from speech data collection initiatives.
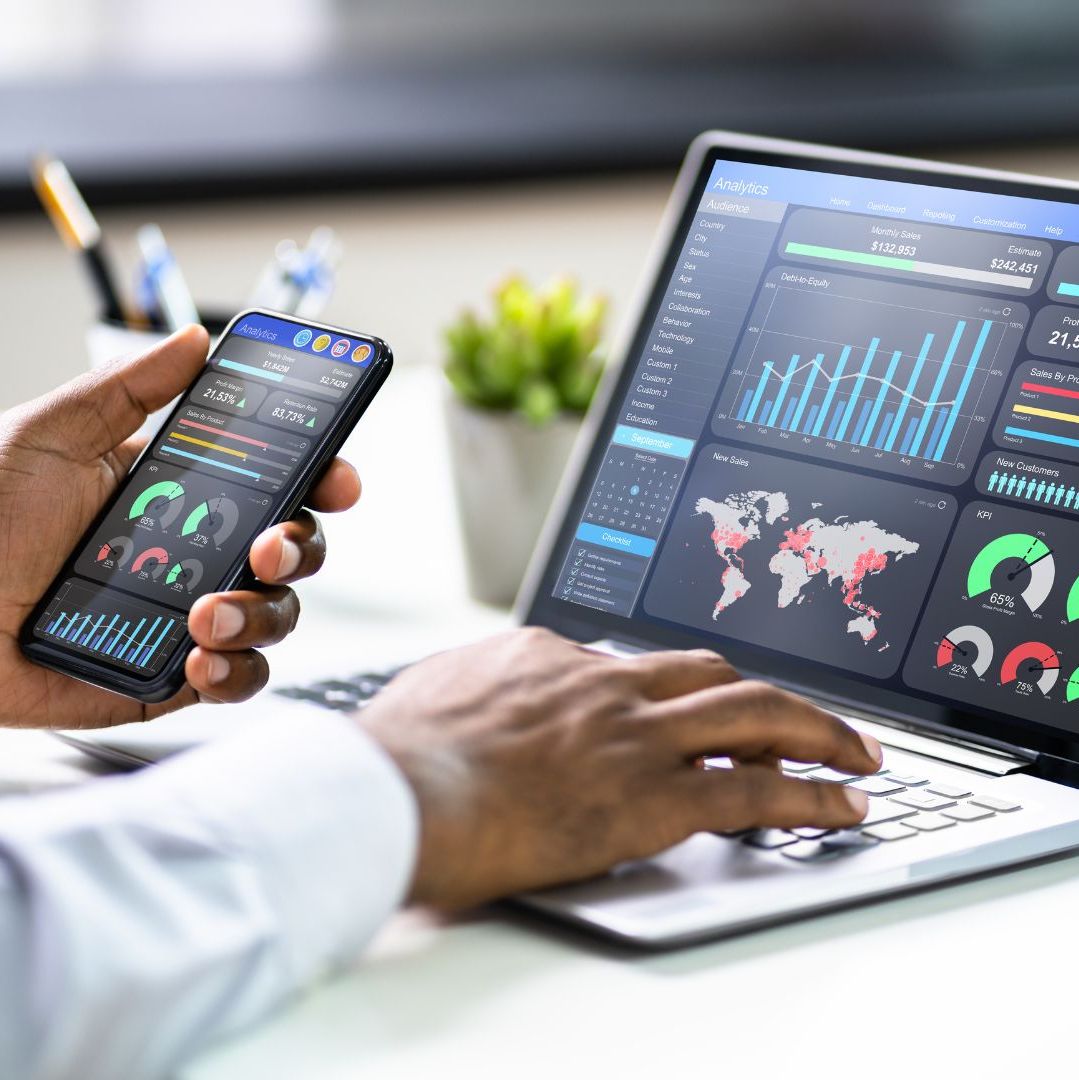
Furthermore, the incredible diversity of dialects within a single language can complicate data collection efforts, as speech recognition systems must be trained on a wide variety of speech patterns to be effective. Additionally, socio-political factors, including instability and resource constraints, can impede the systematic collection of speech data, further exacerbating the scarcity of resources necessary for developing robust speech recognition systems.
These challenges underscore the need for innovative approaches to data collection that account for the unique socio-economic and political landscapes of African countries. Overcoming obstacles to data collection requires not only technological innovation but also a deep understanding of local contexts and the development of strategies that are sensitive to the needs and limitations of communities. Addressing these issues is essential for building effective speech recognition systems that can serve the diverse linguistic needs of the continent and for ensuring that the benefits of AI technologies are accessible to all.
Impact of Under-Resourced Languages on AI Development
The lack of data for under-resourced African languages hinders the creation of inclusive AI technologies, potentially exacerbating digital divides and limiting access to information and services for speakers of these languages.
The scarcity of data for under-resourced African languages has a profound impact on the development and deployment of AI technologies on the continent. This data gap not only hinders the creation of speech recognition systems capable of understanding these languages but also exacerbates existing digital divides, limiting access to information, services, and opportunities for speakers of these languages. The consequence is a technological ecosystem that is less inclusive and equitable, where the benefits of AI are not evenly distributed across linguistic groups. This situation underscores the importance of addressing the data scarcity issue as a matter of both technological advancement and social equity.
Moreover, the lack of linguistic data for many African languages means that these communities are often left out of the conversation around AI and technology development. This exclusion can lead to the loss of valuable cultural and linguistic knowledge, as well as missed opportunities for innovation that draws on the rich linguistic heritage of the continent. By prioritising the collection and analysis of speech data for under-resourced languages, stakeholders can ensure that AI technologies are more inclusive, reflective of the continent’s diversity, and capable of serving the needs of all its people.
Strategies for Data Collection
Innovative approaches to data collection, such as community-driven initiatives and partnerships with local institutions, can play a pivotal role in gathering speech data for under-represented languages.
Developing effective strategies for the collection of speech data in under-represented African languages requires innovative approaches that leverage community-driven initiatives and partnerships with local institutions. Engaging with communities at the grassroots level can facilitate the collection of diverse speech samples, ensuring that the data reflects the rich linguistic diversity within and across African languages.
Such community-driven approaches not only aid in data collection but also help in building trust and ensuring that the process respects the cultural and linguistic heritage of the communities involved. Additionally, partnerships with local educational institutions, NGOs, and government bodies can provide the necessary infrastructure and logistical support for large-scale data collection efforts.
These strategies must be underpinned by a commitment to inclusivity and accessibility, ensuring that the benefits of speech recognition technologies are equitably distributed. By incorporating local knowledge and expertise in the data collection process, stakeholders can overcome some of the challenges associated with linguistic diversity and limited technological infrastructure. Moreover, innovative use of mobile technology, which has widespread penetration even in remote areas of Africa, can be a powerful tool in gathering speech data, thereby helping to bridge the digital divide and bring the voices of under-represented communities into the forefront of AI development.
The Role of NGOs and Researchers
NGOs and researchers are critical in identifying data gaps and mobilising resources to support data collection and analysis for under-resourced African languages, thereby facilitating the development of more inclusive speech recognition technologies.
Non-governmental organisations (NGOs) and researchers play a pivotal role in bridging the data gap for under-resourced African languages. By identifying linguistic communities that are underrepresented in digital platforms and mobilising resources for data collection, these entities can drive the development of more inclusive speech recognition technologies.
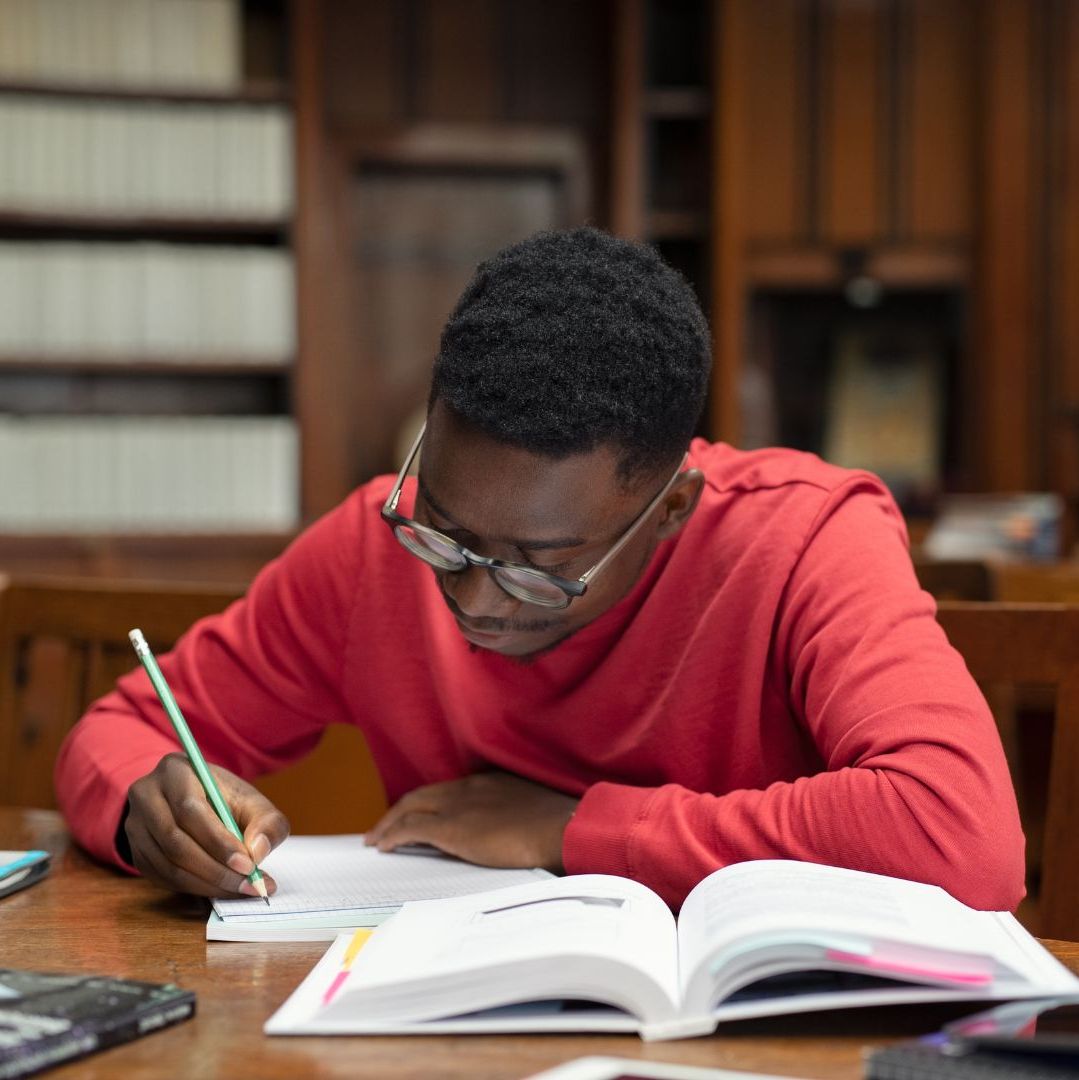
Their work is crucial in highlighting the importance of linguistic diversity in the technological landscape and in advocating for the allocation of resources towards the collection and analysis of speech data in African languages. Through collaborative research projects, capacity-building initiatives, and advocacy, NGOs and researchers can help to raise awareness of the importance of linguistic data for AI development and encourage the adoption of best practices in data collection and analysis.
Furthermore, NGOs and researchers are uniquely positioned to facilitate partnerships between different stakeholders, including local communities, technology companies, and government agencies. These collaborations can leverage the strengths of each partner to address the challenges of data collection and ensure that the development of AI technologies is grounded in an understanding of local needs and contexts. By fostering a multidisciplinary approach to data collection, NGOs and researchers can help to ensure that speech recognition technologies are developed in a way that is respectful of cultural and linguistic diversity, ethically sound, and socially beneficial.
Technological Solutions for Data Analysis
Advanced machine learning algorithms and natural language processing techniques are essential tools for analysing speech data, enabling the development of speech recognition models that can accommodate the linguistic complexity of African languages.
Advanced machine learning algorithms and natural language processing (NLP) techniques are at the heart of developing speech recognition models that can navigate the linguistic complexity of African languages. These technologies offer powerful tools for analysing vast amounts of speech data, identifying patterns, and learning the nuances of different languages and dialects.
The application of these technologies in the analysis of speech data for under-resourced African languages is critical for building effective and accurate speech recognition systems. By leveraging the latest advancements in AI and machine learning, researchers can develop models that are capable of understanding and processing speech in a wide range of African languages, thereby making technology more accessible and inclusive.
However, the effective application of these technological solutions requires a careful consideration of the linguistic diversity and complexity of African languages. This entails not only the collection of large and diverse datasets but also the development of algorithms that are specifically tailored to the linguistic features of these languages. Such efforts must be guided by a deep understanding of the phonetic, morphological, and syntactic properties of African languages, as well as the cultural contexts in which they are spoken.
Through a combination of technological innovation and linguistic expertise, it is possible to overcome the challenges associated with speech data analysis for under-resourced languages and pave the way for the development of speech recognition technologies that truly serve the needs of African communities.
Case Studies of Successful Data Collection Initiatives
Highlighting successful initiatives that have managed to collect and utilise speech data for under-resourced African languages can provide valuable insights and best practices for similar efforts.
Highlighting successful initiatives that have managed to collect and utilise speech data for under-resourced African languages can provide valuable insights and best practices for similar efforts. These case studies exemplify how innovative approaches to data collection, coupled with strong community engagement and partnerships, can overcome the challenges associated with linguistic diversity and limited technological infrastructure.
For instance, projects that leverage mobile technology to gather speech samples from remote or linguistically diverse communities demonstrate the potential of technology to bridge the digital divide and facilitate the inclusion of under-represented languages in digital platforms.
Such initiatives often rely on a collaborative approach, bringing together NGOs, local communities, researchers, and technology companies to work towards a common goal. By sharing the lessons learned from these successful projects, stakeholders can replicate and scale up effective strategies for speech data collection across the continent. These case studies not only serve as a testament to the feasibility of collecting speech data for under-resourced languages but also highlight the importance of community involvement, technological innovation, and cross-sector partnerships in the development of inclusive speech recognition technologies.
The Importance of Ethical Considerations
Ethical considerations, including data privacy and consent, are paramount in the collection and use of speech data, ensuring that such efforts respect the rights and cultures of language communities.
In the collection and use of speech data, ethical considerations are paramount to ensure that the rights and cultures of language communities are respected. This includes obtaining informed consent from participants, ensuring data privacy, and being transparent about how the data will be used. Ethical data collection practices are essential for building trust with communities and for the sustainable development of speech recognition technologies that are culturally sensitive and inclusive. Moreover, ethical considerations extend to the development and deployment of these technologies, ensuring that they do not reinforce existing inequalities or biases but rather contribute to the empowerment of linguistic communities.
The adherence to ethical principles in the collection and analysis of speech data also involves a commitment to the fair and equitable distribution of the benefits derived from AI technologies. This means that the development of speech recognition systems should not only aim to include under-resourced languages but also to ensure that these technologies are accessible to the speakers of these languages. By prioritising ethical considerations in every stage of the data collection and technology development process, stakeholders can contribute to the creation of a digital ecosystem that respects linguistic diversity and promotes social equity.
Future Directions for Research and Development
Identifying emerging trends and opportunities for research and development can guide stakeholders in addressing the data scarcity for African languages and enhancing speech recognition technologies.
Identifying emerging trends and opportunities for research and development is crucial for addressing the data scarcity for African languages and enhancing speech recognition technologies. This includes exploring new methodologies for data collection, such as crowd-sourcing and the use of gamification, to engage wider communities in the process.
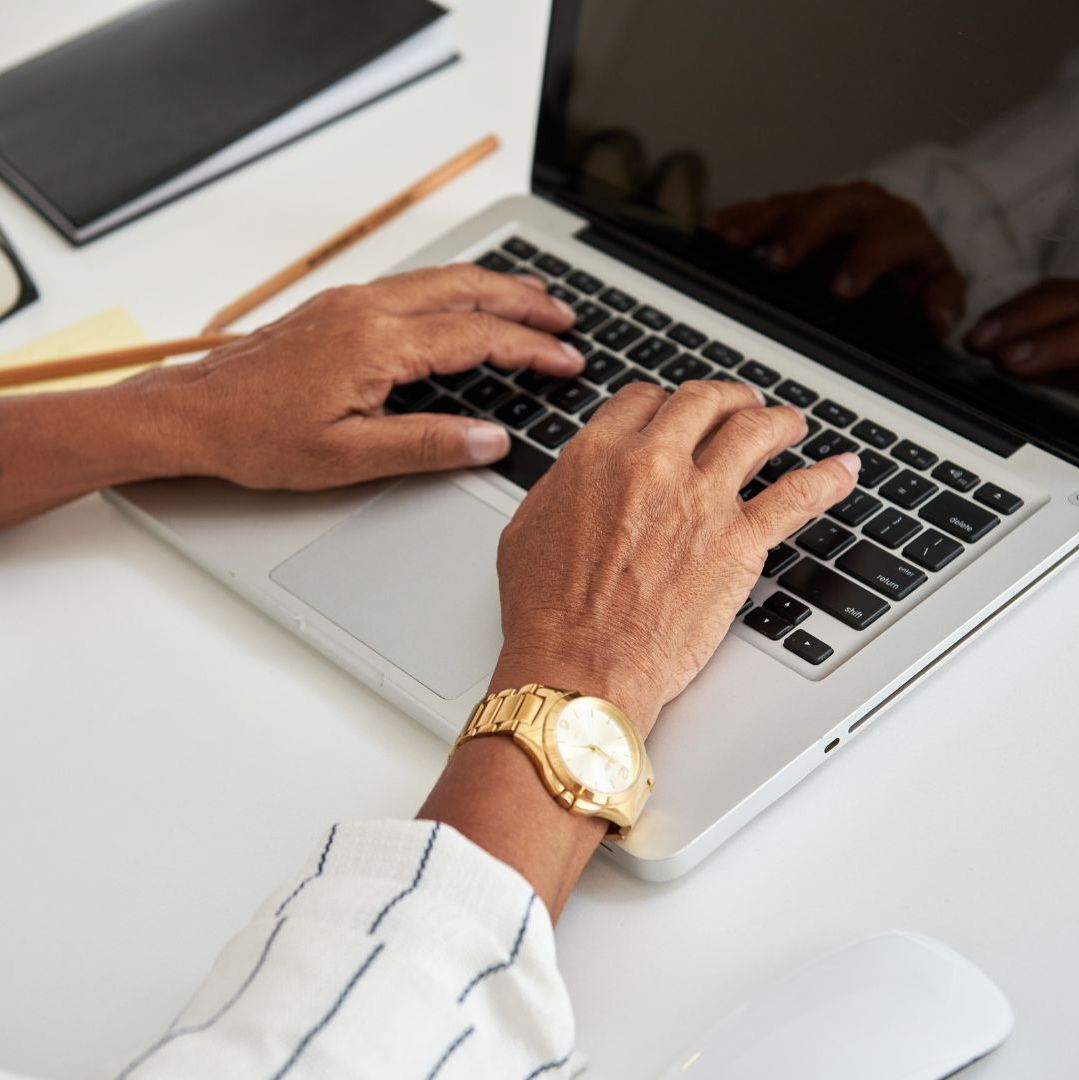
Additionally, advancements in AI and machine learning offer promising avenues for improving the accuracy and efficiency of speech recognition models, even with limited data. Research into transfer learning and unsupervised learning techniques, for example, could enable the development of models that can learn from related languages or dialects, thereby reducing the amount of data needed for effective speech recognition.
Moreover, the integration of speech recognition technologies with other forms of AI, such as machine translation and natural language understanding, can open up new possibilities for multilingual communication and access to information. By focusing on the development of holistic AI systems that can understand, translate, and respond in a wide range of African languages, researchers and developers can significantly enhance the inclusivity and utility of technology on the continent. The future of AI development in Africa lies in embracing the linguistic diversity of the continent as a source of innovation and strength, rather than as a barrier to technological advancement.
Collaboration and Funding Opportunities
Collaboration between governments, international organisations, and the private sector is essential for securing the funding and support needed to advance speech recognition technologies for under-resourced African languages.
Collaboration between governments, international organisations, and the private sector is essential for securing the funding and support needed to advance speech recognition technologies for under-resourced African languages. These partnerships can mobilise the necessary resources for large-scale data collection initiatives and support the development of AI technologies that are inclusive of linguistic diversity. Government policies that promote technological innovation and linguistic inclusivity can also play a crucial role in encouraging private sector investment in the development of speech recognition technologies for African languages.
Furthermore, international organisations and funding bodies can provide critical support for research and development projects that aim to address the data scarcity for under-resourced languages. By prioritising funding for initiatives that promote linguistic diversity and digital inclusion, these organisations can help to ensure that the benefits of AI technologies are accessible to all. Collaboration across sectors and disciplines is key to overcoming the challenges associated with speech recognition for African languages and to harnessing the full potential of AI for social and economic development on the continent.
Collecting Under-resourced African Language Data – Key Tips
- Focus on innovative and ethical data collection methods to build speech datasets for under-resourced African languages.
- Leverage partnerships with local communities and institutions to facilitate data collection and ensure cultural and linguistic accuracy.
- Utilise advanced machine learning and natural language processing techniques to analyse and improve speech recognition models for African languages.
Way With Words provides highly customised speech data collections for African languages, aiding technologies in creating or improving existing ASR models using NLP for select African languages across various domains.
The development of speech recognition technologies for African languages presents a unique set of challenges and opportunities. By identifying languages with the least data available, stakeholders can prioritise efforts to bridge these gaps, thereby fostering the creation of more inclusive and accessible AI technologies. Success in this regard requires a collaborative approach, involving researchers, NGOs, technology entrepreneurs, and local communities, to ensure that speech recognition technologies can serve the diverse linguistic landscape of Africa.
The key piece of advice for advancing in this field is to embrace innovation, collaboration, and ethical considerations in all data collection and analysis efforts, ensuring that the development of AI technologies respects and uplifts the voices of all language communities.
Under-resourced African Languages Resources
African Language Speech Collection Solution: Way With Words -We create custom speech datasets for African languages including transcripts for machine learning purposes. Our service is used for technologies looking to create or improve existing automatic speech recognition models (ASR) using natural language processing (NLP) for select African languages and various domains.
Machine Transcription Polishing of Captured Speech Data: Way With Words -We polish machine transcripts for clients across a number of different technologies. Our machine transcription polishing (MTP) service is used for a variety of AI and machine learning purposes that are intended to be applied in various African languages. User applications include machine learning models that use speech-to-text for artificial intelligence research, FinTech/InsurTech, SaaS/Cloud Services, Call Centre Software, and Voice Analytic services for the customer journey.
Multilingual Speech Recognition Initiative for African Languages: This paper summarises a speech recognition initiative for African languages. More precisely, we propose innovative approaches that address the low-resource property of these languages.