The Difference Between Text Annotators & Data Annotators
Unveiling the Distinctions: Text Annotators vs. Data Annotators in AI Development
What is the difference between a text annotator and a data annotator? Artificial Intelligence (AI) has become an integral part of our lives, transforming the way we interact with technology. Behind every successful AI system lies meticulous data annotation, a process that involves labelling and organising data to train AI models. Within the realm of data annotation, two distinct roles emerge: text annotators and data annotators. In this blog post, we will delve into the key differences between these roles, explore their specific tasks, methodologies, applications, and shed light on the unique challenges associated with text annotation and data annotation.
Text Annotators: The Language Architects
Text annotators play a crucial role in the development of AI systems that process and understand natural language. Their primary responsibility revolves around labelling and structuring textual data. Whether it’s sentiment analysis, named entity recognition, or machine translation, text annotators possess the linguistic prowess and domain expertise required to unravel the intricacies of language.
Tasks and Methodologies
Text annotators work with unstructured textual data, which can include social media posts, customer reviews, scientific articles, or any other form of text. Their tasks include:
Named Entity Recognition (NER): Text annotators identify and classify entities in text, such as names of people, organisations, locations, dates, and other specific terms relevant to the given domain. For example, in a medical context, annotators would recognise and categorise symptoms, medications, and diseases.
Sentiment Analysis: Analysing the sentiment behind a given text is another vital task of text annotators. They label text segments as positive, negative, or neutral, providing valuable data for sentiment analysis models. This information is utilised in applications like customer feedback analysis, brand monitoring, and market research.
Text Categorisation: Text annotators categorise documents or passages into predefined classes or topics. This task aids in content organisation, document retrieval, and filtering. News article classification or email spam filtering are examples where text categorisation plays a crucial role.
Challenges and Applications
Text annotation presents its own set of challenges. Ambiguities, slang, sarcasm, and context-dependent meanings can make the annotation process complex. Furthermore, differences in language usage across regions and cultures require text annotators to have a deep understanding of the target audience.
The applications of text annotation are vast and ever-expanding. Text annotators contribute to the development of chatbots, virtual assistants, language translation systems, content recommendation engines, and much more. Their work empowers AI systems to comprehend and generate human-like text, enhancing user experiences and enabling seamless interactions with technology.
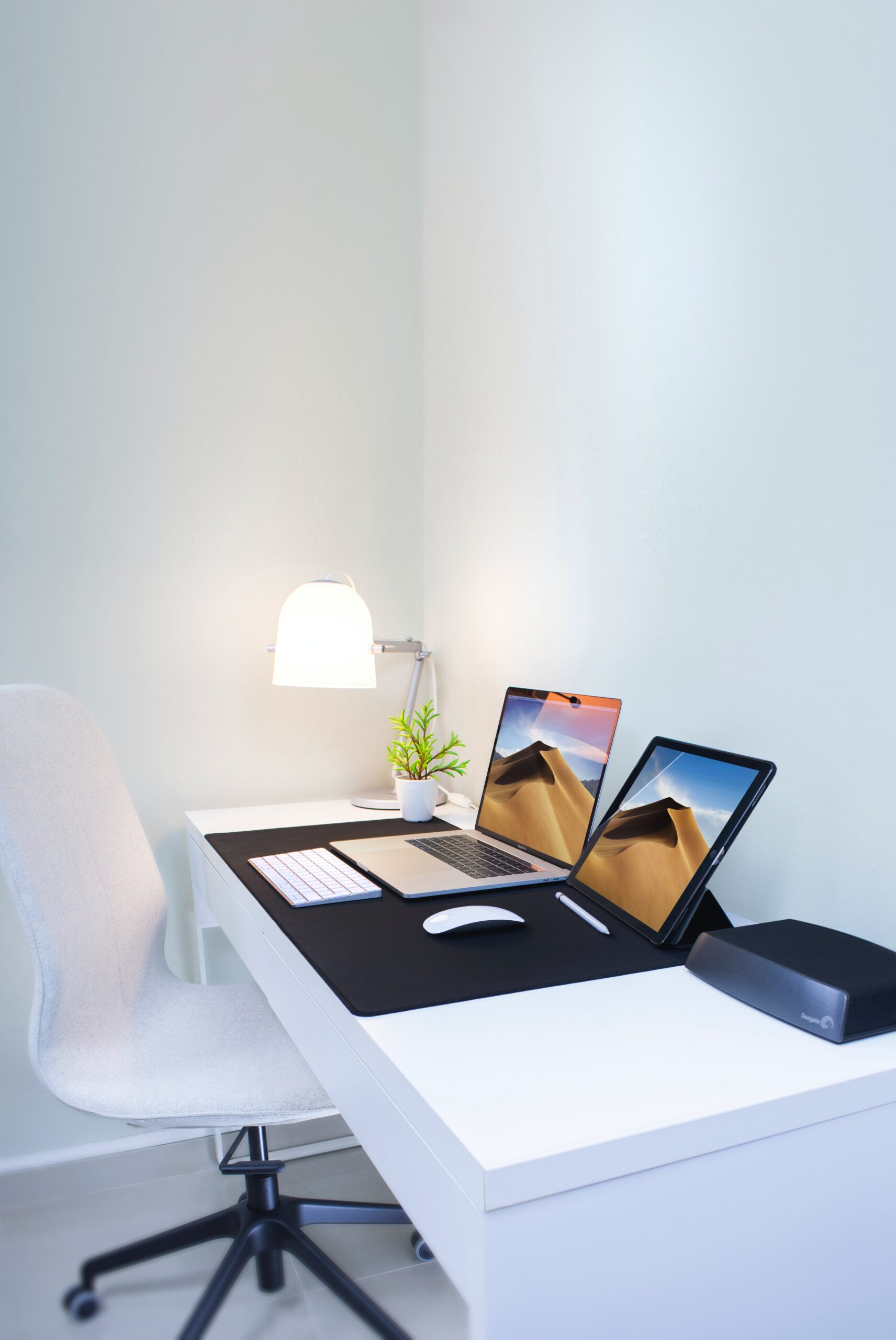
Data Annotators: Mapping the Digital World
Data annotators, also known as general annotators, tackle a broader range of data types beyond textual information. Their role encompasses labelling and organising various forms of data, including images, videos, audio files, and sensor data. Their work ensures that AI models can interpret and make informed decisions based on diverse types of information.
Tasks and Methodologies
Data annotators deal with structured and unstructured data, often employing specialised tools and annotation software. Their tasks include:
Image Annotation: Data annotators mark and classify objects, regions, and attributes within images. This process helps computer vision models recognise and understand visual elements accurately. Object detection, image segmentation, and facial recognition are examples where image annotation is indispensable.
Video Annotation: Videos are dissected frame-by-frame by data annotators to label and track objects, actions, and events. Annotated video data facilitates the training of AI models for tasks like activity recognition, object tracking, and video summarisation.
Audio Annotation: Data annotators transcribe and label speech in audio recordings, enabling AI models to convert spoken language into written text, perform speech recognition, and extract meaningful insights. Applications include voice assistants, transcription services, and speaker identification.
Challenges and Applications
Data annotation presents unique challenges due to the complexity and diversity of data types involved. Subjectivity, variability, and the need for meticulous precision can pose difficulties for data annotators. Interpreting ambiguous images, handling large-scale datasets, and maintaining consistency across annotations are common hurdles they face.
The applications of data annotation span across various industries. Self-driving cars rely on annotated sensor data to navigate and detect objects accurately. E-commerce platforms utilise annotated product images to improve visual search and recommendation systems. Medical imaging annotation aids in the diagnosis of diseases, and social media platforms rely on content moderation annotation to maintain a safe and inclusive online environment.
Collaboration and Synergy
While text annotators and data annotators have distinct roles, their collaboration is often necessary for comprehensive AI development. Text data often accompanies other forms of data, such as images, in real-world applications. For instance, an autonomous vehicle AI system might use both image annotation and text annotation to understand road signs and traffic rules simultaneously.
Furthermore, advancements in AI, such as multimodal learning, are blurring the lines between text and data annotation. Multimodal models leverage the synergy between different types of data, enabling systems to analyse and understand text, images, and other modalities collectively. This requires close collaboration between text annotators and data annotators to create high-quality, multimodal datasets.
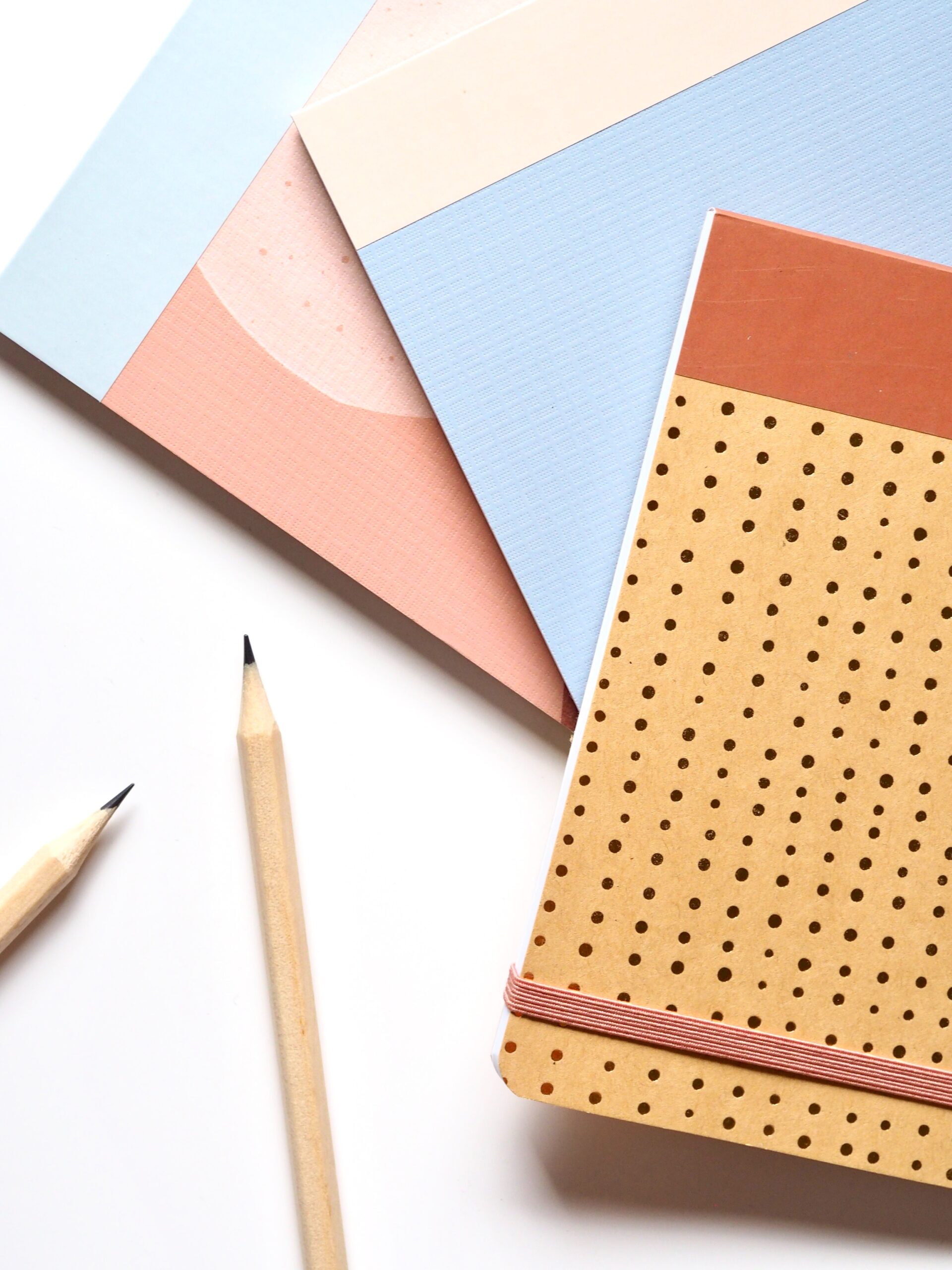
Text annotators and data annotators play vital roles in AI development, each with its own set of tasks, methodologies, challenges, and applications. Text annotators specialise in processing and structuring textual data, providing the necessary linguistic insights for language-related AI tasks. On the other hand, data annotators handle a wide range of data types, labelling and organising information to enable AI models to interpret and make informed decisions.
Their collaboration is often essential, especially in projects involving multiple data modalities. The seamless integration of text and data annotation is crucial for developing robust AI systems capable of understanding and processing diverse forms of information.
As AI continues to evolve, the demand for skilled text annotators and data annotators will only grow. Their expertise and meticulous work lay the foundation for AI systems that can understand, interpret, and interact with humans in meaningful ways. By recognising and appreciating the distinctions between these roles, we can harness their collective power and unlock the true potential of AI in our increasingly intelligent world.
With a 21-year track record of excellence, we are considered a trusted partner by many blue-chip companies across a wide range of industries. At this stage of your business, it may be worth your while to invest in a human transcription service that has a Way With Words.
Additional Services
About Captioning
Perfectly synched 99%+ accurate closed captions for broadcast-quality video.
Machine Transcription Polishing
For users of machine transcription that require polished machine transcripts.