The Value of Corpus Data in NLP and SRT
Corpus Data is Quickly Advancing The Fields of Natural Language Processing And Speech Recognition Technology
Natural Language Processing (NLP) and Speech Recognition Technology (SRT) are two important fields that are rapidly advancing with the help of corpus data. Corpus data refers to a large and structured set of texts or speech that can be used for linguistic analysis. In this blog post, we will explore the use of corpus data in NLP and SRT, discussing its benefits and limitations, its techniques and tools, and its potential ethical implications.
What is Corpus Data and Why is it Important?
Corpus data refers to a collection of texts or speech that are used for linguistic analysis. These collections can range from small samples to large databases of texts or recordings. They can be created in many different ways, such as collecting data from social media, newspapers, books, or speeches. These collections are then tagged and labelled with specific linguistic features, such as parts of speech or named entities.
Corpus data is important for NLP and SRT applications because it provides a large and structured set of data for training and testing language models. Language models are algorithms that can recognise patterns and structures in language, allowing machines to understand and generate human language. The use of corpus data allows these models to learn from real-world examples and improve their accuracy and performance.
Types of Corpus Data
There are many different types of corpus data that can be used in NLP and SRT applications. Some common types include:
Written corpus data: This type of corpus data includes texts such as books, articles, and web pages.
Spoken corpus data: This type of corpus data includes recordings of speech, such as conversations, interviews, or speeches.
Parallel corpus data: This type of corpus data includes translations of the same text in multiple languages, allowing for machine translation applications.
Domain-specific corpus data: This type of corpus data includes texts or speech from a specific domain, such as legal or medical texts, allowing for domain-specific language models.
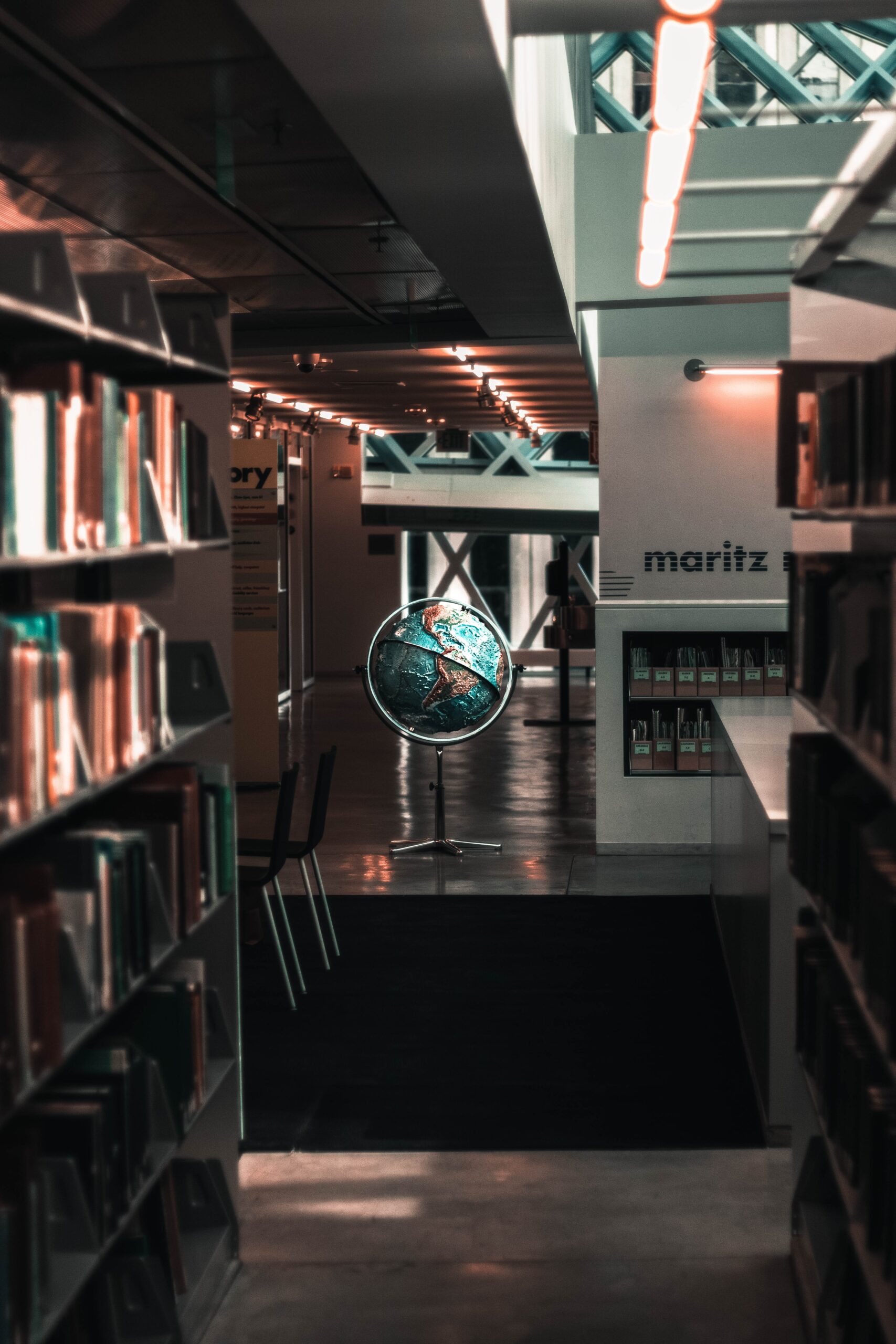
Benefits and Limitations of Using Corpus Data
Benefits
• Allows for the creation of more accurate and reliable NLP and SRT systems, as it provides a large and diverse sample of natural language data to train and test algorithms.
• Enables the development of language models that can understand and generate natural language at a level closer to human proficiency.
• Facilitates the study of language patterns and usage, leading to new discoveries and insights in linguistics and cognitive science.
• Provides a source of authentic language data for linguistic research, including dialectology, sociolinguistics, and language variation studies.
Limitations
• Corpus data may not be representative of all language usage and may contain biases depending on the source of the data.
• It can be time-consuming and costly to collect and process large amounts of corpus data.
• The quality of corpus data can vary, and errors in transcription or annotation can affect the accuracy of NLP and SRT systems.
• The use of corpus data can raise ethical concerns related to data privacy, representation, and bias.
Examples of How Corpus Data is Used
Despite these limitations, corpus data is an essential tool for NLP and SRT applications. Let’s take a look at some specific examples of how corpus data is used in these fields.
Machine Translation: Corpus data is used extensively in machine translation to train algorithms to recognise patterns and translate text from one language to another. The more corpus data available, the more accurate the translation system can become. For example, Google Translate relies on a large corpus of bilingual texts to generate translations.
Sentiment Analysis: Corpus data is also used in sentiment analysis, which involves analysing text to determine the author’s emotional tone. By analysing a large corpus of text, sentiment analysis algorithms can identify patterns and accurately classify text according to sentiment. For example, social media companies use sentiment analysis to monitor customer satisfaction and identify potential issues.
Voice Recognition: Corpus data is used in speech recognition to train algorithms to recognise and transcribe spoken language. By analysing a large corpus of spoken language data, algorithms can identify patterns in speech and improve accuracy. For example, Siri, the voice assistant developed by Apple, relies on a large corpus of recorded speech data to improve accuracy and understand natural language commands.
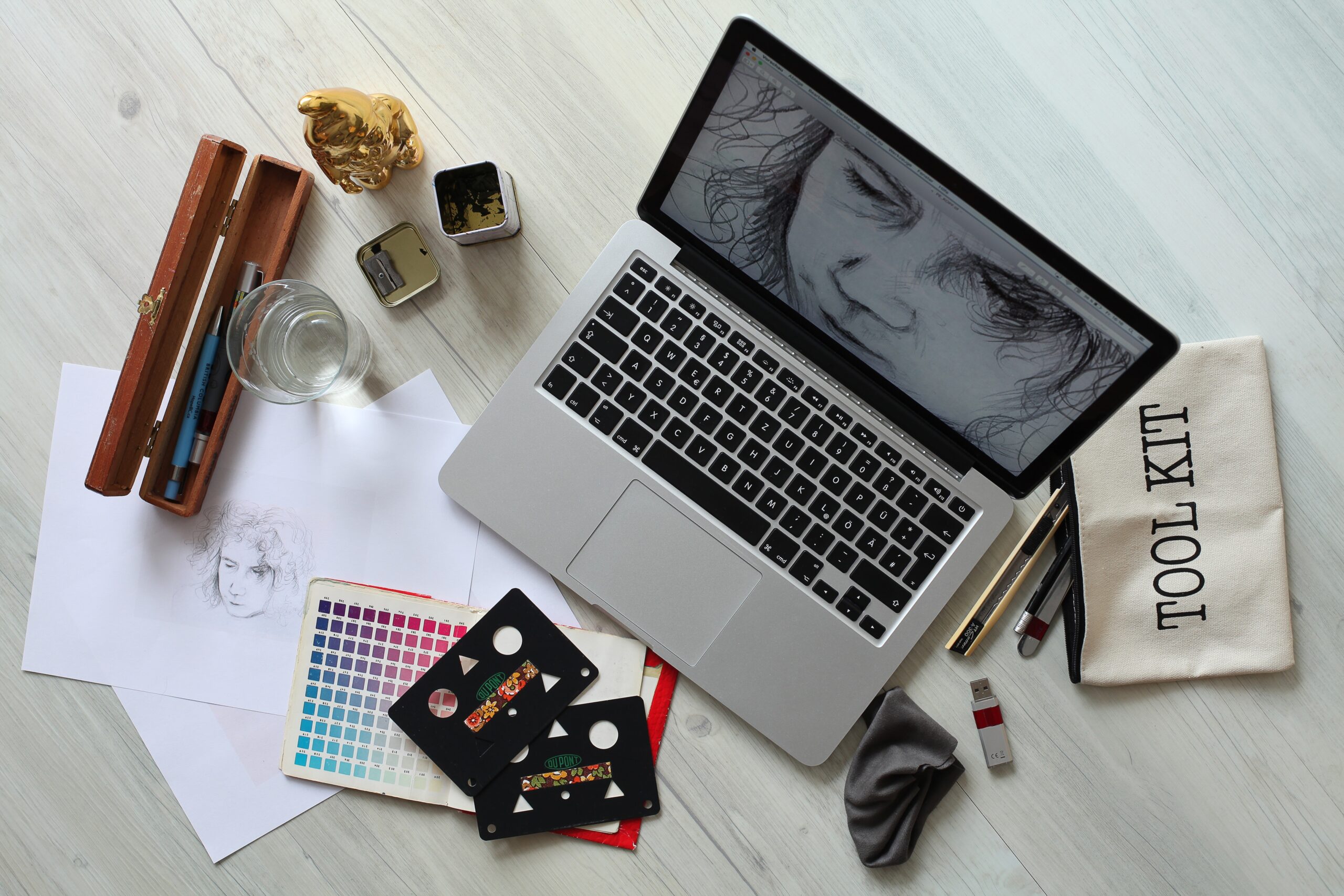
Techniques and Tools for Analysing Corpus Data
Now let’s discuss some of the techniques and tools commonly used to analyse and process corpus data in NLP and SRT.
Frequency Analysis: Frequency analysis involves counting the occurrence of words and phrases in a corpus of text. This technique can help identify common patterns in language usage and provide insights into vocabulary and grammar.
Concordancing: Concordancing involves creating a list of words used in a corpus of text and displaying them in context. This technique can help identify patterns in word usage and provide insights into how words are used in context.
Collocation Analysis: Collocation analysis involves identifying and analysing pairs or groups of words that tend to occur together frequently. This technique can help identify patterns in language usage and provide insights into how words are used together in context.
Ethical Implications of Using Corpus Data
It’s important to consider the potential ethical implications of using corpus data in NLP and SRT. The use of corpus data can raise ethical concerns related to data privacy, representation, and bias.
Data Privacy
One of the most significant ethical concerns related to corpus data is data privacy. Corpus data is often collected from public sources, including social media, and individuals may not be aware that their data is being used. This raises concerns about informed consent and the right to privacy. Additionally, the data may be sensitive or personal, and its use without proper consent can be a violation of individuals’ rights.
Representation
Another ethical concern related to corpus data is representation. Corpus data is often biased towards dominant groups, such as white, male, or English-speaking individuals. This can lead to bias in NLP and SRT algorithms, which can perpetuate existing social inequalities. For example, voice recognition algorithms may struggle to recognise accents or dialects that are not represented in the corpus data, leading to discrimination against non-dominant groups.
Bias
Bias is another ethical concern related to corpus data. Corpus data can be biased towards certain topics or perspectives, leading to algorithms that reflect those biases. For example, sentiment analysis algorithms may be biased towards positive sentiment, as the corpus data used to train them may contain more positive than negative sentiment. This can lead to inaccurate analysis and biased results.
Best Practices
To address these ethical concerns, best practices must be followed when using corpus data in NLP and SRT. These include:
• Informed Consent: Individuals should be informed that their data may be used and given the option to opt-out.
• Data Protection: Corpus data should be anonymised and kept secure to protect individuals’ privacy.
• Diversity: Corpus data should be diverse and representative of all groups to prevent bias in algorithms.
• Transparency: The process of collecting and using corpus data should be transparent, and the algorithms should be open to scrutiny to identify and correct any biases.
Corpus data is an essential tool for NLP and SRT applications, providing a large and diverse sample of natural language data to train and test algorithms. While there are limitations and potential ethical concerns associated with the use of corpus data, the benefits of using this data outweigh the risks.
Are you looking to expand and develop your NLP and SRT applications? We offer custom datasets as well as off the shelf datasets for you! Contact us today to find out how we can help you.
Additional Services
About Captioning
Perfectly synched 99%+ accurate closed captions for broadcast-quality video.
Machine Transcription Polishing
For users of machine transcription that require polished machine transcripts.