Why Are Annotations Important
Why Are Annotations Important: Enhancing SRT and NLP Models and Beyond
You may find yourself wondering why are annotations important for SRT and NLP models. Annotations play a crucial role in various industries, providing valuable context and enhancing the performance of technologies such as Speech Recognition Technology (SRT) and Natural Language Processing (NLP) models. These annotations, whether in the form of labelled data, metadata, or semantic markers, contribute to improving accuracy, training efficiency, and overall understanding. In this blog post, we will delve into the technical aspects of annotations and explore their significance in the development of SRT and NLP models.
Understanding Annotations
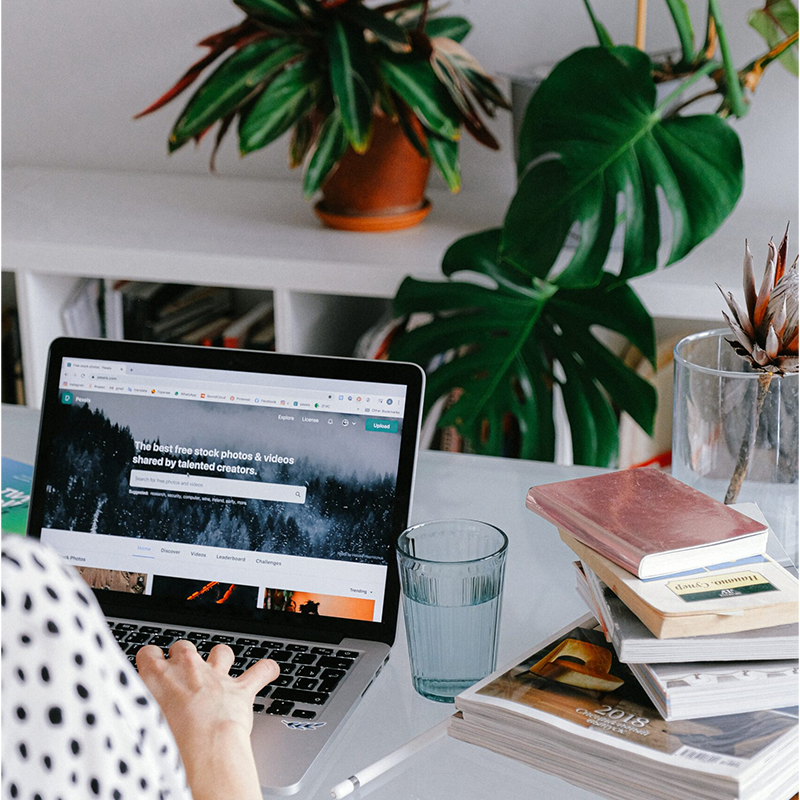
Enhancing Speech Recognition Technology (SRT) with Annotations
SRT systems convert spoken language into written text, revolutionising voice-controlled applications, transcription services, and more. However, training accurate and reliable SRT models requires vast amounts of annotated speech data. Here’s how annotations contribute to SRT development:
Phonetic Annotations: Phonemes represent the smallest units of sound in a language. Annotating speech data with phonetic information aids in training SRT models to recognise and differentiate between different sounds accurately, especially in multilingual applications.
Language-Specific Annotations: Different languages have unique linguistic characteristics. Annotations provide language-specific information such as accents, dialects, intonation patterns, and speech quirks, helping SRT models adapt to diverse linguistic contexts.
Contextual Annotations: Annotations that capture context, such as speaker identification, punctuation, and disfluencies, enhance the accuracy and fluency of SRT models. This enables the recognition of speaker turns, pauses, and other linguistic nuances, resulting in more accurate transcriptions.
Leveraging Annotations for Natural Language Processing (NLP) Models
NLP models focus on understanding, interpreting, and generating human language. Annotations are instrumental in training NLP models and improving their capabilities. Some key aspects where annotations significantly impact NLP models include:
Named Entity Recognition (NER): NER involves identifying and classifying named entities in text, such as names, locations, organisations, and more. Annotations provide labelled examples, enabling NLP models to learn the patterns and context associated with different entity types, improving extraction accuracy.
Sentiment Analysis: Annotations that label text with sentiment polarity (positive, negative, or neutral) facilitate the training of sentiment analysis models. These annotations help NLP models understand subjective opinions and emotions expressed in text, supporting applications like social media monitoring, customer feedback analysis, and brand sentiment analysis.
Semantic Role Labelling (SRL): SRL focuses on identifying the roles of words or phrases in a sentence, such as the subject, object, or predicate. Annotated data with semantic roles helps NLP models comprehend the relationships and dependencies within sentences, enhancing their understanding and generating more accurate outputs.
Beyond SRT and NLP: Annotations in Various Industries
Annotations extend beyond SRT and NLP, playing a pivotal role in numerous industries:
Healthcare: Medical annotations aid in analysing electronic health records, medical imaging, and clinical data, enabling accurate diagnosis, treatment planning, and medical research.
Autonomous Vehicles: Annotations help train machine learning models for object detection, lane recognition, and traffic sign recognition, enabling safe and efficient autonomous driving.
E-commerce: Product annotations, such as categorisation, attributes, and user reviews, improve search relevancy, personalised recommendations, and customer experience.
Content Moderation: Annotations assist in flagging and moderating content in online platforms, ensuring compliance with community guidelines, and identifying inappropriate or harmful content.
Language Translation: Annotated parallel corpora, where texts in different languages are aligned, serve as valuable resources for training machine translation models, enabling accurate and fluent translations
Financial Analysis: Annotations on financial data, such as labelling transactions, categorising expenses, and identifying fraud patterns, support automated financial analysis, risk assessment, and fraud detection.
Virtual Assistants and Chatbots: Annotations contribute to the training of conversational agents, helping them understand user intents, generate appropriate responses, and provide personalised assistance.
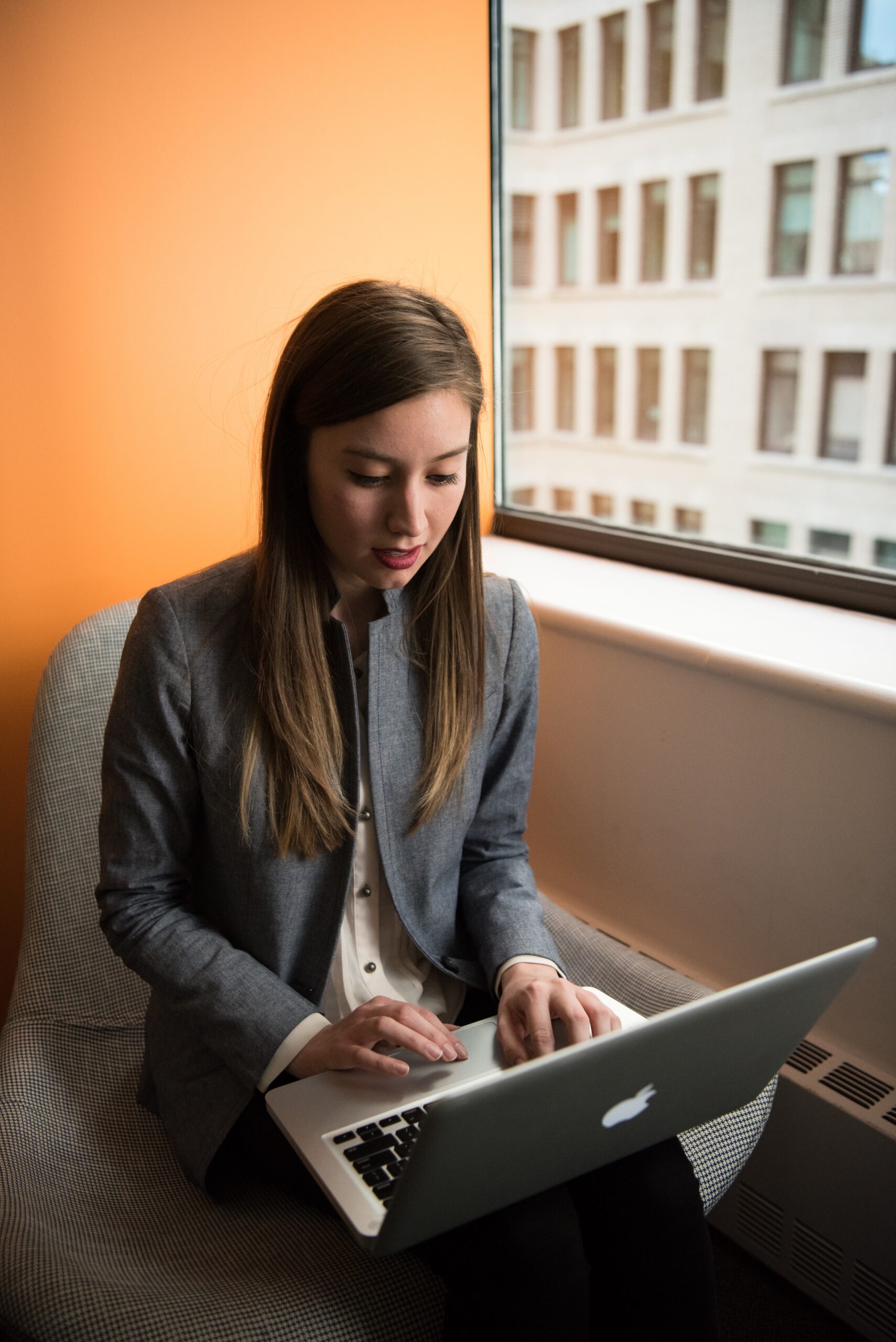
Annotations play a vital role in enhancing SRT and NLP models, enabling accurate speech recognition, language understanding, and natural language generation. They provide context, structure, and labelled data, facilitating effective training and improving performance. However, the significance of annotations extends far beyond SRT and NLP, finding applications in healthcare, autonomous vehicles, e-commerce, content moderation, language translation, financial analysis, and more. As technology advances, the need for high-quality annotations becomes increasingly critical for developing robust and intelligent systems across various industries. By recognising the importance of annotations and investing in their creation, organisations can unlock the full potential of data-driven technologies and drive innovation in the digital age.
With a 21-year track record of excellence, we are considered a trusted partner by many blue-chip companies across a wide range of industries. At this stage of your business, it may be worth your while to invest in a human transcription service that has a Way With Words.
Additional Services
About Captioning
Perfectly synched 99%+ accurate closed captions for broadcast-quality video.
Machine Transcription Polishing
For users of machine transcription that require polished machine transcripts.