Finding Gold: Top Sources for High-Quality Speech Data
What are the Best Sources for High-quality Speech Data?
High-quality speech data has become an indispensable asset. From training robust speech recognition models to improving natural language processing algorithms, the quality and source of speech data can significantly impact the efficacy of these technologies. These solutions are especially important for under-resourced languages such as many found in Africa, Latin America, and other regions with a wide variety of dialects and languages. This short guide aims to explore the best sources for high-quality speech data, addressing common questions that data scientists, AI researchers, machine learning engineers, technology firms, and academic institutions often have.
- What are the characteristics of high-quality speech data?
- Where can we find reliable speech data sources?
- How do we evaluate the quality of speech data?
Whether you’re an experienced data scientist or a tech firm exploring new frontiers, finding the right speech data sources is essential for your project’s success.
Some Important Points Regarding Quality Speech Datasets and Sources
Characteristics of High-Quality Speech Data
High-quality speech data possesses several defining characteristics that ensure its effectiveness for training and developing AI models. Here are the key attributes to look for:
- Accuracy and Clarity: The speech recordings should be clear and free of background noise, ensuring the accuracy of transcriptions.
- Diversity: The dataset should include a variety of accents, dialects, and speaking styles to ensure robustness.
- Contextual Relevance: The content of the speech data should be relevant to the application it will be used for.
- Metadata: Detailed metadata about the recordings, such as speaker demographics and environmental conditions, enhances the dataset’s utility.
- Volume and Scalability: A high volume of data allows for better training of models, and the ability to scale is crucial for ongoing development.
Understanding these characteristics helps in identifying and selecting the best sources for high-quality speech data.
High-quality speech data is paramount for training effective AI and machine learning models, particularly in the realm of speech recognition and natural language processing. The first critical characteristic is accuracy and clarity. Accurate and clear recordings are essential as they form the basis for reliable transcriptions and model training. Clarity in speech recordings implies the absence of background noise and distortions, allowing the AI system to learn from precise, unambiguous data.
For instance, if a dataset is plagued with background noises or muffled sounds, the AI model might learn to interpret these as part of the speech, leading to erroneous outputs. This clarity is particularly important in applications where precision is crucial, such as medical transcriptions or legal documentation.
Another important attribute of high-quality speech data is diversity. A diverse dataset includes a wide range of accents, dialects, and speaking styles. This diversity ensures that the AI model is robust and can generalise well across different speech patterns. For example, a voice assistant trained on a diverse dataset will perform better in understanding and responding to users from different geographical regions with varying accents.
Inadequate diversity in training data can lead to biased models that perform well on certain accents but poorly on others, thereby limiting their usability in global applications. Diversity also extends to the inclusion of various speaking contexts, such as formal, informal, conversational, and scripted speech, enhancing the model’s ability to handle different real-world scenarios.
Contextual relevance is another key factor in determining the quality of speech data. The speech content should be relevant to the specific application it will be used for. For instance, a dataset for training a medical transcription AI should contain speech related to medical terminology and conversations between healthcare professionals and patients. Using irrelevant data can lead to poor model performance and an increased rate of errors.
Additionally, detailed metadata about the recordings, such as speaker demographics, recording environment, and contextual information, enhances the dataset’s utility. Metadata allows for better analysis and understanding of the dataset, facilitating more effective model training and evaluation. Finally, volume and scalability are critical characteristics. A large volume of data is essential for training deep learning models, which require extensive datasets to learn effectively. Scalability ensures that the dataset can be expanded or adapted as new data becomes available, allowing for continuous improvement of the AI models.
Trusted Sources for Speech Data
Several trusted sources provide high-quality speech data, catering to the diverse needs of AI and machine learning projects. Here are some notable ones:
- OpenSLR: This platform offers a variety of public speech datasets, widely used in academic and research settings.
- LibriSpeech: A large-scale corpus derived from audiobooks, providing extensive data for speech recognition tasks.
- Common Voice by Mozilla: A community-driven project that collects and shares diverse speech data, focusing on inclusivity and variety.
- Linguistic Data Consortium (LDC): Provides curated and high-quality speech datasets, commonly used in academia and industry.
- Way With Words Speech Collection: Tailored speech datasets designed to meet specific needs of AI and machine learning projects.
For example, the platform includes datasets like the LibriSpeech corpus, which is derived from audiobooks and provides a rich source of diverse and high-quality speech data.
When sourcing speech data, it is essential to turn to trusted providers that offer high-quality and reliable datasets. One such source is OpenSLR. This platform offers a wide variety of public speech datasets that are extensively used in academic and research settings.
OpenSLR’s datasets are particularly valued for their diversity and the comprehensive documentation that accompanies them, making them a go-to resource for researchers and developers seeking robust training data. For example, the platform includes datasets like the LibriSpeech corpus, which is derived from audiobooks and provides a rich source of diverse and high-quality speech data.
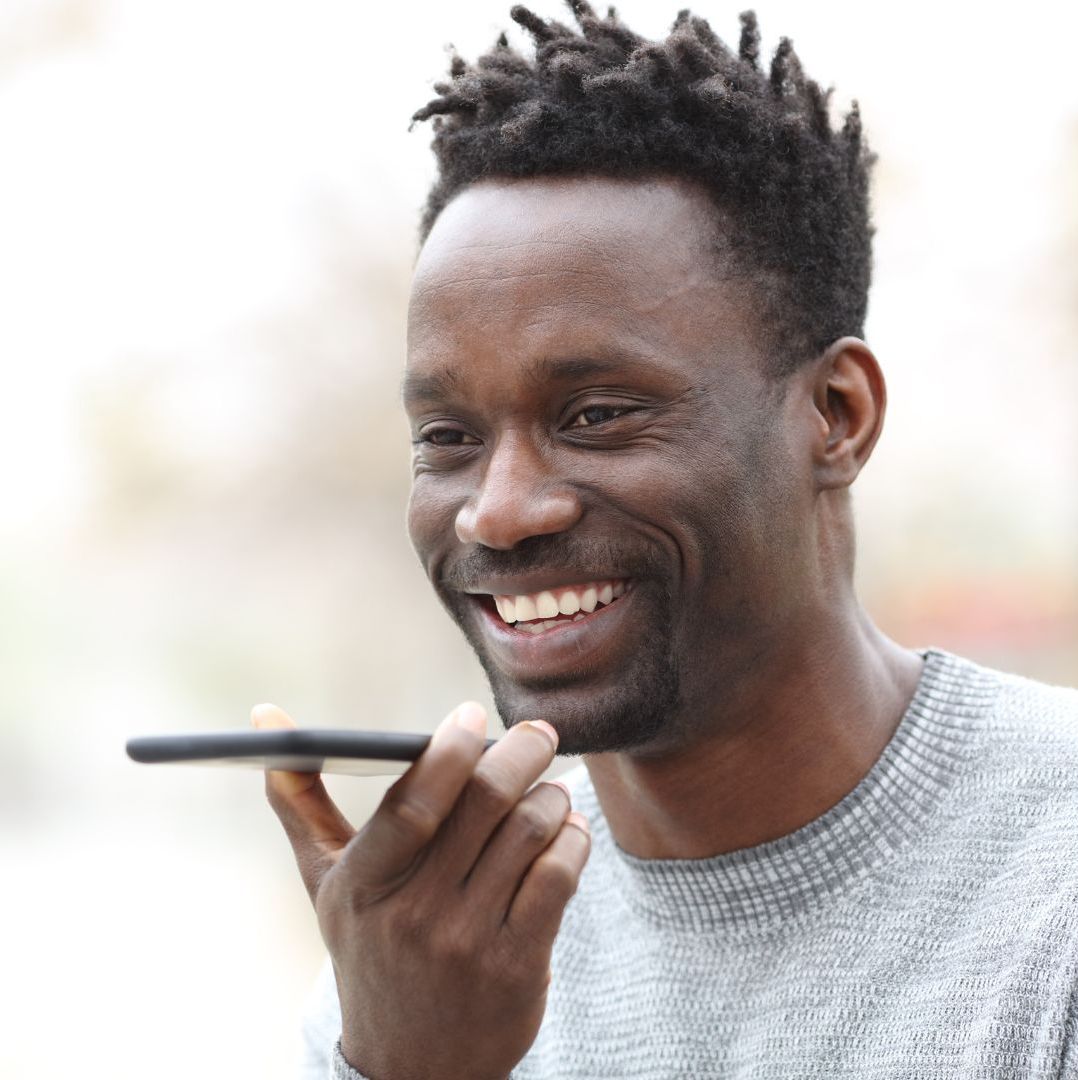
LibriSpeech is another notable source, renowned for its extensive and meticulously curated dataset. Derived from audiobooks, LibriSpeech provides a large-scale corpus of read speech, offering a diverse array of accents and speaking styles. This dataset is especially useful for training speech recognition models as it contains a wealth of transcribed data that can be used to fine-tune algorithms. The quality and size of the LibriSpeech dataset make it a benchmark resource in the field of speech recognition research and development.
Common Voice by Mozilla is a community-driven project that stands out for its focus on inclusivity and variety. This project collects and shares diverse speech data contributed by volunteers worldwide. Common Voice aims to create datasets that reflect the linguistic diversity of the global population, making it an excellent resource for training models that need to understand and respond to a wide range of accents and dialects. The community-driven nature of Common Voice ensures continuous updates and expansions to the dataset, enhancing its relevance and utility for various applications.
The Linguistic Data Consortium (LDC) is another highly reputable source, providing curated and high-quality speech datasets commonly used in both academia and industry. The LDC’s datasets are meticulously compiled and come with extensive documentation, making them reliable for various research and development purposes.
Finally, Way With Words Speech Collection offers tailored speech datasets designed to meet the specific needs of AI and machine learning projects. This provider ensures that the datasets are high quality and contextually relevant, making them ideal for specialised applications. These trusted sources are well-regarded for their reliability and the quality of data they provide, making them essential resources for any AI or machine learning project involving speech data.
Comparing Public vs. Private Speech Data Sources
When sourcing speech data, one must consider whether to use public or private datasets. Both have their advantages and drawbacks.
Public Speech Data Sources:
- Pros: Easily accessible, often free, and come with extensive documentation and community support.
- Cons: May lack diversity and contextual relevance specific to your application.
Private Speech Data Sources:
- Pros: Can be tailored to specific needs, ensuring higher relevance and quality.
- Cons: Can be expensive and may come with licensing restrictions.
Understanding the trade-offs between public and private sources helps in making an informed decision based on project requirements and budget.
When sourcing speech data, one of the primary considerations is whether to use public or private datasets. Both options have distinct advantages and potential drawbacks that must be weighed carefully based on project requirements and budget. Public speech data sources are easily accessible and often free, making them a cost-effective option for many projects. They come with extensive documentation and community support, which can be invaluable for researchers and developers.
For instance, platforms like OpenSLR and Common Voice by Mozilla provide publicly available datasets that are widely used in academic and research settings. These datasets are well-documented, allowing users to understand their composition and potential limitations fully.
However, public speech data sources can have certain limitations. They may lack the diversity and contextual relevance specific to certain applications. For example, a public dataset may not have enough samples of a particular accent or speaking style that is crucial for a specific AI project. Additionally, public datasets might not be updated frequently, potentially leading to outdated data that does not reflect current linguistic trends or speech patterns. In such cases, relying solely on public sources might limit the effectiveness and accuracy of the resulting AI models.
On the other hand, private speech data sources offer several advantages, particularly in terms of customisation and relevance. Private datasets can be tailored to specific needs, ensuring higher relevance and quality. This is especially important for specialised applications that require speech data with particular characteristics or contexts. For instance, a medical transcription AI would benefit significantly from a private dataset specifically curated with medical conversations and terminology.
However, private speech data sources can be expensive and may come with licensing restrictions, making them less accessible for smaller projects or organisations with limited budgets. The cost of acquiring private datasets can be a significant investment, but it often pays off in terms of the enhanced accuracy and performance of the AI models trained on them.
In conclusion, the choice between public and private speech data sources depends on several factors, including project requirements, budget, and the need for customisation. Public datasets are a great starting point, offering accessible and well-documented resources for initial model training and research. For more specialised applications or when higher accuracy is required, private datasets provide tailored solutions that can significantly enhance model performance. By understanding the trade-offs between public and private sources, developers and researchers can make informed decisions that best align with their specific needs and constraints.
Case Studies of Effective Speech Data Usage
Examining real-world case studies provides valuable insights into how high-quality speech data can be effectively utilised.
Case Study 1: Google Assistant: Google utilises vast amounts of diverse speech data to train its voice assistant, ensuring it understands and responds accurately to a wide range of accents and dialects.
Case Study 2: DeepMind’s WaveNet: DeepMind’s WaveNet, a deep generative model of raw audio waveforms, leverages high-quality speech data to produce natural-sounding synthetic speech.
Case Study 3: Way With Words’ AI Projects: Way With Words has developed custom speech datasets for various AI projects, demonstrating the importance of tailored data in improving model performance.
Real-world case studies provide invaluable insights into how high-quality speech data can be effectively utilised in various applications. One prominent example is Google Assistant. Google employs vast amounts of diverse speech data to train its voice assistant, ensuring it understands and responds accurately to a wide range of accents and dialects. This diversity in training data allows Google Assistant to provide reliable performance across different regions and languages. By leveraging high-quality, diverse speech datasets, Google has been able to create a robust voice assistant that excels in real-world applications, demonstrating the critical role of comprehensive speech data in AI development.
Another notable case study is DeepMind’s WaveNet. WaveNet, developed by DeepMind, is a deep generative model of raw audio waveforms. This model leverages high-quality speech data to produce natural-sounding synthetic speech. The success of WaveNet is a testament to the importance of high-quality and diverse speech data in generating realistic audio outputs. DeepMind’s approach involved using extensive datasets that captured various accents, speaking styles, and environmental conditions. This comprehensive dataset enabled WaveNet to generate speech that is remarkably close to human speech in terms of naturalness and clarity, highlighting the impact of quality data on the effectiveness of AI models.
Way With Words’ AI Projects also provide compelling examples of effective speech data usage. Way With Words has developed custom speech datasets for various AI projects, emphasising the importance of tailored data in improving model performance.
For instance, in projects aimed at developing speech recognition systems for specific industries, Way With Words has curated datasets that include industry-specific terminology and contextual speech patterns. This targeted approach ensures that the AI models trained on these datasets perform exceptionally well in their intended applications. The success of these projects underscores the value of customised, high-quality speech data in achieving superior AI performance.
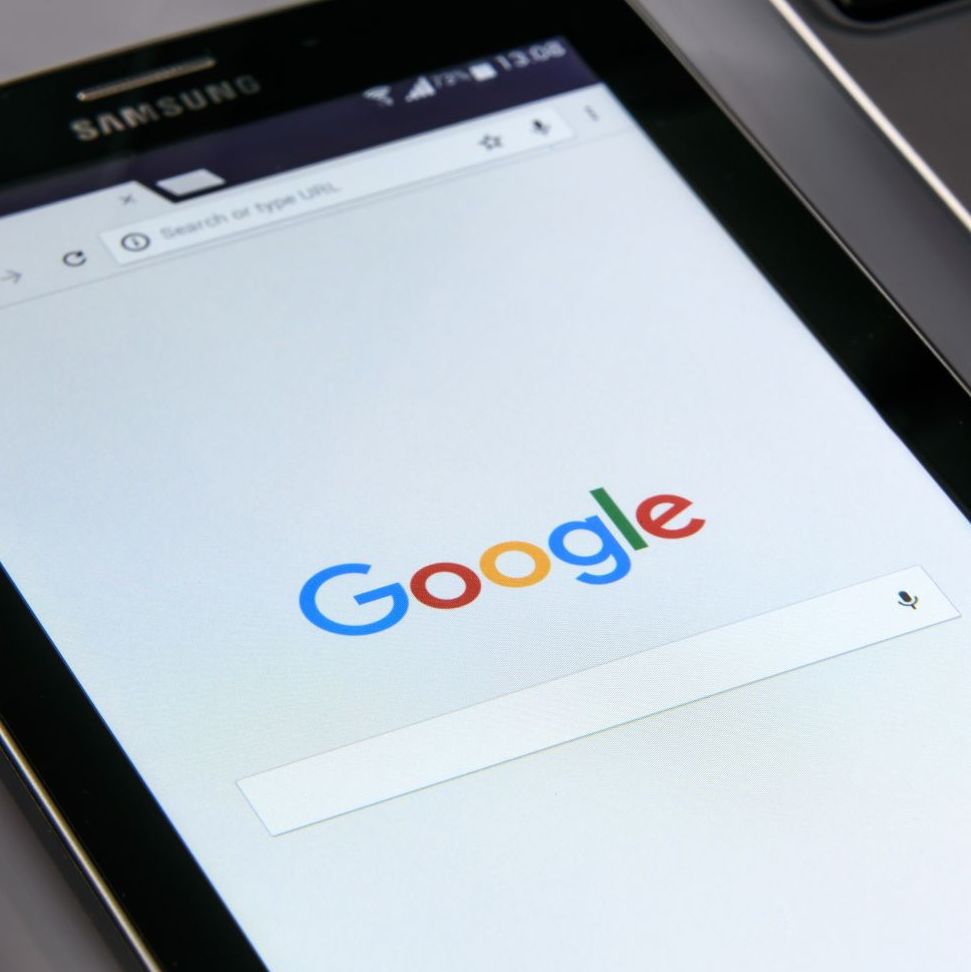
These case studies highlight the critical role of high-quality speech data in developing effective and accurate AI applications. They demonstrate that investing in diverse, well-curated datasets pays off significantly in terms of model performance and user satisfaction.
By examining these real-world examples, researchers and developers can gain a deeper understanding of how to leverage high-quality speech data to enhance their AI projects. Whether it’s training a voice assistant to handle diverse accents or generating natural-sounding synthetic speech, the quality and relevance of the speech data used play a pivotal role in the success of these technologies.
Guidelines for Evaluating Speech Data Quality
Evaluating the quality of speech data involves several critical steps:
- Source Credibility: Ensure the data source is reputable and has a track record of providing high-quality datasets.
- Dataset Documentation: Comprehensive documentation is essential for understanding the dataset’s composition and limitations.
- Sample Testing: Conducting preliminary tests on a sample of the data helps identify any potential issues.
- Relevance to Application: The data should closely match the real-world scenarios in which the AI system will operate.
- Continuous Monitoring: Regularly review and update the dataset to maintain its relevance and quality.
Evaluating the quality of speech data is a critical step in ensuring the success of AI and machine learning projects. One of the first steps in this evaluation process is assessing the credibility of the data source. Ensuring that the source is reputable and has a proven track record of providing high-quality datasets is essential. For instance, datasets from established institutions like the Linguistic Data Consortium (LDC) or well-known platforms like OpenSLR are often more reliable. These sources typically provide comprehensive documentation and detailed descriptions of their datasets, which help users understand the data’s composition and any potential limitations.
Dataset documentation is another crucial aspect of evaluating speech data quality. Comprehensive documentation includes detailed information about the dataset, such as the number of recordings, the demographics of the speakers, the recording conditions, and any preprocessing steps that have been applied. This information is vital for understanding the context and potential biases in the data. Well-documented datasets allow researchers to make informed decisions about the suitability of the data for their specific applications. For example, a dataset with detailed metadata about speaker accents and environmental conditions can help in training more robust and contextually aware AI models.
Sample testing is a practical approach to evaluate the quality of speech data. Conducting preliminary tests on a sample of the data can help identify any issues related to clarity, accuracy, or diversity. For instance, listening to a subset of recordings can reveal background noise or speech distortions that might affect model training. Additionally, testing the data with existing models can provide insights into its effectiveness and any potential biases. This step is particularly important for identifying issues that might not be apparent from the documentation alone.
Relevance to the intended application is another critical factor in evaluating speech data quality. The data should closely match the real-world scenarios in which the AI system will operate. For example, a dataset for a voice assistant should include diverse speech patterns, accents, and contextual scenarios that the assistant is likely to encounter.
Ensuring the data’s relevance helps in training models that perform well in practical applications, reducing the likelihood of errors and improving user satisfaction. Continuous monitoring and updating of the dataset are also essential to maintain its relevance and quality over time. As new speech patterns and accents emerge, updating the dataset ensures that the AI models remain accurate and effective.
In conclusion, evaluating the quality of speech data involves several critical steps, including assessing the source credibility, reviewing documentation, conducting sample testing, ensuring relevance, and continuous monitoring. By following these guidelines, researchers and developers can ensure that the speech data they choose is of the highest quality and suitable for their specific needs. High-quality speech data forms the foundation for successful AI and machine learning projects, enabling the development of accurate and reliable speech recognition and natural language processing systems.
Key Tips For Ensuring High-Quality Speech Data
- Diversify Your Data: Ensure your dataset includes various accents, dialects, and speaking styles.
- Verify Metadata: Detailed metadata enhances the dataset’s utility.
- Balance Public and Private Sources: Use a mix of both to get the best of accessibility and relevance.
- Continuous Evaluation: Regularly assess and update your datasets.
- Leverage Community Resources: Utilise community-driven projects like Common Voice for diverse data.
High-quality speech data is a cornerstone for successful AI and machine learning projects. By understanding the characteristics of good speech data, utilising trusted sources, and carefully evaluating the quality, you can ensure that your AI systems perform effectively and accurately. Whether you opt for public datasets like LibriSpeech or tailor-made collections from Way With Words, the key is to choose data that is diverse, well-documented, and relevant to your application. With continuous evaluation and a balanced approach, finding the gold in speech data becomes a more achievable task.
Further Speech Data Sources
Wikipedia: Dataset – This article explains what datasets are, including their importance, types, and how they are used in various applications, helping readers understand the context of speech datasets.
Way With Words: Speech Collection – Way With Words offers access to high-quality speech datasets, tailored to meet the specific needs of AI and machine learning projects. These datasets ensure the accuracy and reliability required for developing sophisticated speech recognition systems