Voice-activated Devices and AI’s Role in Speech Recognition
The Integral Role of AI in Speech Recognition and Voice-Activated Devices and Technologies
In an era dominated by rapid technological advancements, AI has significantly revolutionised the way we interact with machines. Particularly intriguing is the development in AI voice-activated devices and speech recognition technologies. These innovations have not just permeated our daily lives but have also become integral in various professional fields. However, as we marvel at these advancements, critical questions arise: How does AI interpret and respond to spoken language? What implications do these technologies have for professionals across various industries, especially in the realms of translation, data science, and technology development?
In this exploration, we delve into the nuanced relationship between AI and speech recognition, understanding not only their functionalities but also the ethical considerations surrounding data collection and usage. The aim is to provide a comprehensive view of AI’s role in voice-activated devices and technology, catering to a diverse audience including translators, interpreters, data scientists, technology entrepreneurs, software developers, educationalists, and industries leveraging AI to enhance communication and language translation technologies.
AI In Voice-Activated Devices And Speech Recognition Technologies
Understanding AI in Voice Recognition
AI voice-activated devices use sophisticated algorithms to interpret human speech, turning voice commands into actionable responses. This process involves acoustic and linguistic modelling to understand speech patterns and language nuances.
The realm of AI in voice recognition is a fascinating intersection of technology and linguistics, where sophisticated algorithms work tirelessly to decode the intricacies of human speech. This process, at its core, involves two critical aspects: acoustic modelling and linguistic modelling. Acoustic modelling delves into the realm of sounds, where AI systems analyse the various acoustic signals that constitute speech. This involves understanding the nuances in tone, pitch, and rhythm that are unique to each individual’s voice.
On the other hand, linguistic modelling deals with the structure and meaning of language. Here, AI systems must not only recognise words but also comprehend grammar, syntax, and the semantics behind spoken phrases. This dual approach enables AI voice-activated devices to transform mere sound waves into comprehensible, actionable information.
However, the challenge in understanding human speech doesn’t just lie in the complexity of language itself but also in its dynamic nature. Colloquialisms, dialects, and evolving linguistic trends pose continuous challenges for AI systems. Moreover, human speech is often imperfect – filled with hesitations, interruptions, and non-standard utterances, further complicating the recognition process.
AI systems, therefore, must be trained on diverse and extensive datasets to capture this variability and improve their interpretative accuracy. The goal is not just to understand words as isolated entities but to grasp the intent and context behind them, making the interaction as seamless and intuitive as speaking to another human.
Evolution of Speech Recognition
From rudimentary voice command systems to advanced neural network-based models, speech recognition has evolved significantly. Today’s AI systems can understand context, accents, and even emotional cues in speech.
Speech recognition technology has undergone a transformative journey, evolving from simplistic voice command systems to today’s advanced neural network-based models. Initially, speech recognition systems were limited, capable of understanding only a restricted set of commands and often requiring user training to adapt to a specific voice. The shift from these rudimentary systems to more sophisticated models parallels advancements in AI and machine learning.
Today, state-of-the-art speech recognition systems utilise deep neural networks, which mimic the neural structures of the human brain, enabling these systems to process and interpret complex language patterns with remarkable accuracy.
A pivotal development in this evolution is the ability of modern systems to understand context, accents, and even emotional cues. This contextual understanding is critical in differentiating between homophones or interpreting phrases that rely on situational context. For instance, the phrase “Let’s eat, Grandma” versus “Let’s eat Grandma” demonstrates how crucial contextual understanding is for accurate interpretation.
Additionally, the incorporation of emotion detection algorithms marks a significant leap, allowing AI systems to recognise not just what is being said, but how it’s being said, opening avenues for more empathetic and responsive interactions. These advancements reflect a shift towards creating AI systems that not only understand human language but also the nuances and subtleties that come with it.
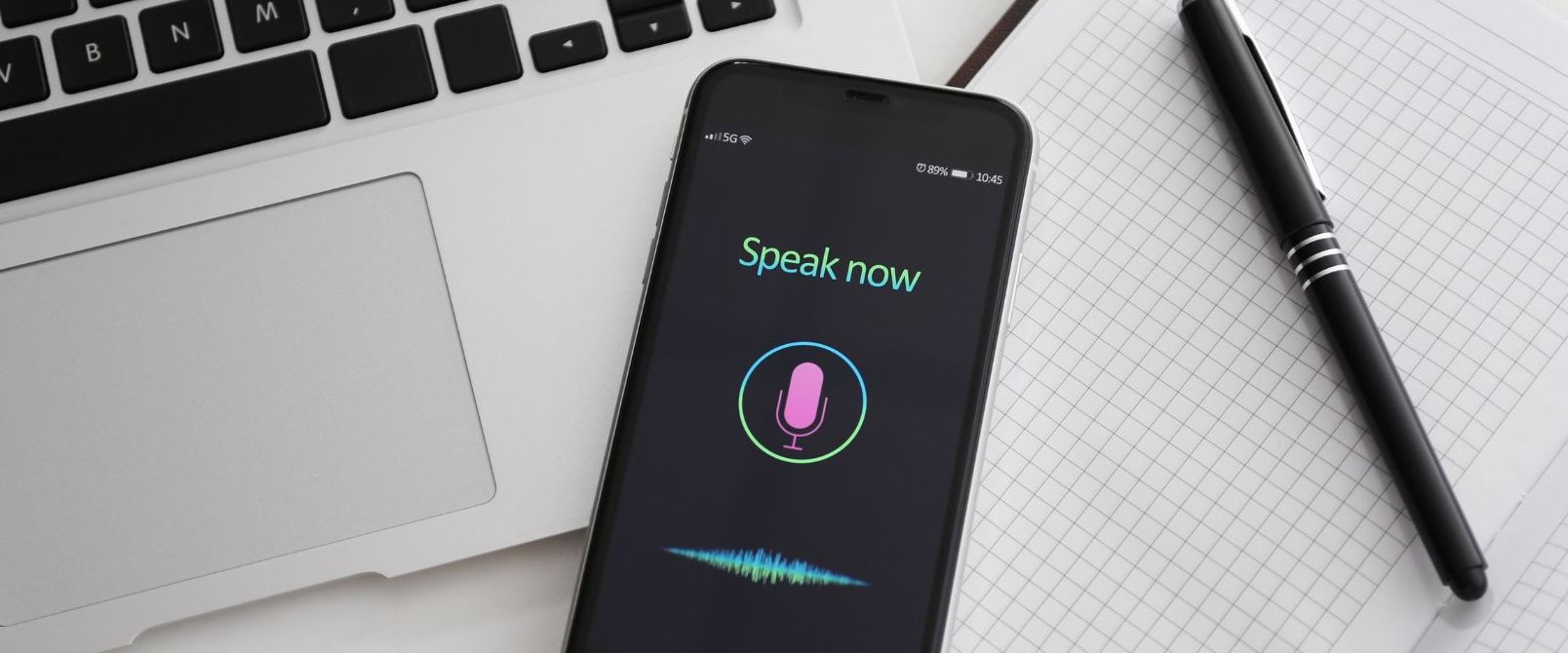
Data Collection and Ethics
Collecting voice data ethically is paramount. It’s essential to ensure that data is gathered with consent and used solely for its intended purpose, respecting privacy and data protection laws.
The ethical collection and use of voice data are critical components in the development of speech recognition technologies. In this digital age, where data is often termed the ‘new oil,’ the way this data is sourced and utilised raises significant ethical considerations.
Collecting voice data ethically involves obtaining explicit consent from individuals, ensuring they are fully aware of how their data will be used. This is not just a legal requirement in many jurisdictions, but also a moral imperative to respect individual privacy and autonomy. Additionally, the data must be used solely for its intended purpose, whether for improving AI algorithms or for specific applications in voice-activated devices.
However, ethical data collection extends beyond mere consent. It involves addressing issues of bias and representation in datasets. AI systems trained on limited or biased data can develop skewed understandings, leading to inaccuracies, especially in recognising diverse accents, dialects, or speech patterns. Therefore, it is essential to source data from a broad demographic spectrum, ensuring inclusivity and fairness in AI development.
Moreover, with increasing awareness of data privacy, transparent data handling practices become crucial in maintaining public trust. Companies and researchers must be clear about data usage, storage, and protection measures to safeguard against unauthorised access or misuse. In essence, ethical data collection is not just about adhering to regulations; it’s about fostering responsible AI development that respects individual rights and societal values.
Role of Machine Learning
Machine learning algorithms are crucial in improving speech recognition accuracy. By analysing vast datasets, these algorithms learn and adapt to various speech patterns and languages.
Machine learning (ML) plays a pivotal role in enhancing the accuracy and efficiency of speech recognition systems. At the heart of ML algorithms lies the ability to learn from data – to identify patterns, make predictions, and improve over time. This learning process is crucial in speech recognition, where the goal is to accurately interpret a vast array of human speech variations. ML algorithms, particularly deep learning models, are adept at processing large datasets, extracting features, and recognising complex patterns that define different languages, accents, and speech nuances.
The continuous improvement of speech recognition accuracy hinges on the volume and quality of data these algorithms are trained on. Diverse datasets that include various languages, dialects, and speech styles enable ML models to generalise better and reduce bias in recognition. This training process also involves continual refinement of algorithms to deal with challenges such as background noise, speech overlaps, and varying speech speeds.
Moreover, ML algorithms are not static; they are constantly updated and retrained to adapt to new linguistic trends and speech patterns. This dynamism is crucial in maintaining the relevance and effectiveness of speech recognition systems in a world where language is ever-evolving. The integration of ML in speech recognition is a testament to how AI can learn and adapt in ways that parallel human learning, albeit at a scale and speed unattainable by humans alone.
Language Diversity and AI
AI’s ability to handle multiple languages and dialects is vital. This includes training AI systems with diverse linguistic data to enhance their cross-cultural applicability.
The ability of AI to handle multiple languages and dialects is not just a technical achievement but a step towards cultural inclusivity and global connectivity. Language diversity in AI involves training systems to recognise and process a wide array of languages, each with its unique syntactic, phonetic, and semantic characteristics. This diversity is crucial, especially considering that there are over 7,000 languages spoken worldwide, each representing a rich cultural and linguistic heritage. For AI to be truly global, it must be capable of understanding this linguistic plurality.
Training AI systems in language diversity involves a comprehensive approach – from sourcing linguistically diverse datasets to implementing algorithms capable of learning different language structures. This includes not only major world languages but also regional dialects and less commonly spoken languages. The challenge here is not just in the linguistic complexity but also in the scarcity of available data for certain languages.
Hence, efforts are made to create balanced datasets that represent the broad spectrum of global languages. The impact of such inclusivity is profound, especially in applications like real-time translation, which can bridge communication gaps and foster understanding across different cultures.
Furthermore, language-diverse AI systems can play a pivotal role in preserving languages, especially those that are endangered, by providing a digital platform for their use and study. In essence, embracing language diversity in AI is about creating technologies that reflect and respect the rich tapestry of human languages and cultures.
Voice-Activated Devices in Everyday Life
From smartphones to smart homes, voice-activated devices have become ubiquitous, aiding in various tasks and providing personalised experiences through AI.
Voice-activated devices have seamlessly integrated into the fabric of everyday life, transforming how we interact with technology. From smartphones that respond to voice commands to smart homes that can be controlled with a simple spoken word, these voice-activated devices offer convenience and enhanced user experience.
This integration represents a significant shift from traditional interaction modes, where physical touch or text input was necessary, to a more natural and intuitive voice-based interface. The applications are vast – from setting alarms, playing music, or getting news updates on a smart speaker to controlling lights, thermostats, and security systems in a smart home environment.
The proliferation of these devices has implications beyond mere convenience. For individuals with physical disabilities or limitations, voice-activated devices and technology can be a game-changer, providing accessibility and independence. In educational contexts, these voice-activated devices can facilitate interactive learning experiences, responding to students’ queries and aiding in language learning. In the business realm, voice assistants can streamline operations, handle customer inquiries, and even assist in data analysis.
However, as these devices become more integrated into daily life, concerns around privacy and data security emerge. Ensuring these devices are secure and that user data is protected is crucial in maintaining trust and ensuring the responsible use of voice-activated devices and technology.
Impact on Translation and Interpretation
AI has revolutionised translation and interpretation, offering real-time, accurate language conversion, which is invaluable for global communication.
The impact of AI on translation and interpretation is profound, marking a significant evolution in how we overcome language barriers. AI-powered translation tools offer real-time, accurate language conversion, a feature invaluable in a globalised world where communication across language divides is commonplace. This technology has revolutionised various sectors – from international business and diplomacy to tourism and education.
In business, for instance, AI-assisted translation enables companies to operate across borders, communicate with global partners, and reach diverse customer bases. For educationalists and researchers, it bridges linguistic gaps, allowing access to a broader range of knowledge and collaborative opportunities.
However, the role of AI in translation extends beyond mere word-for-word conversion. Advanced AI systems are now capable of understanding idiomatic expressions, cultural references, and contextual nuances, ensuring translations are not just accurate but also contextually relevant. This is particularly important in interpretation, where the subtleties of spoken language, including tone and inflection, carry significant meaning.
Moreover, AI systems are continuously learning and improving, leveraging vast datasets and user feedback to refine their translations. Despite these advancements, human translators and interpreters remain essential, especially for complex texts, legal documents, or nuanced cultural contexts where human judgment and understanding are irreplaceable. AI in translation and interpretation, therefore, is best viewed as a complement to human expertise, expanding capabilities and reach, but not replacing the nuanced understanding that only humans can provide.
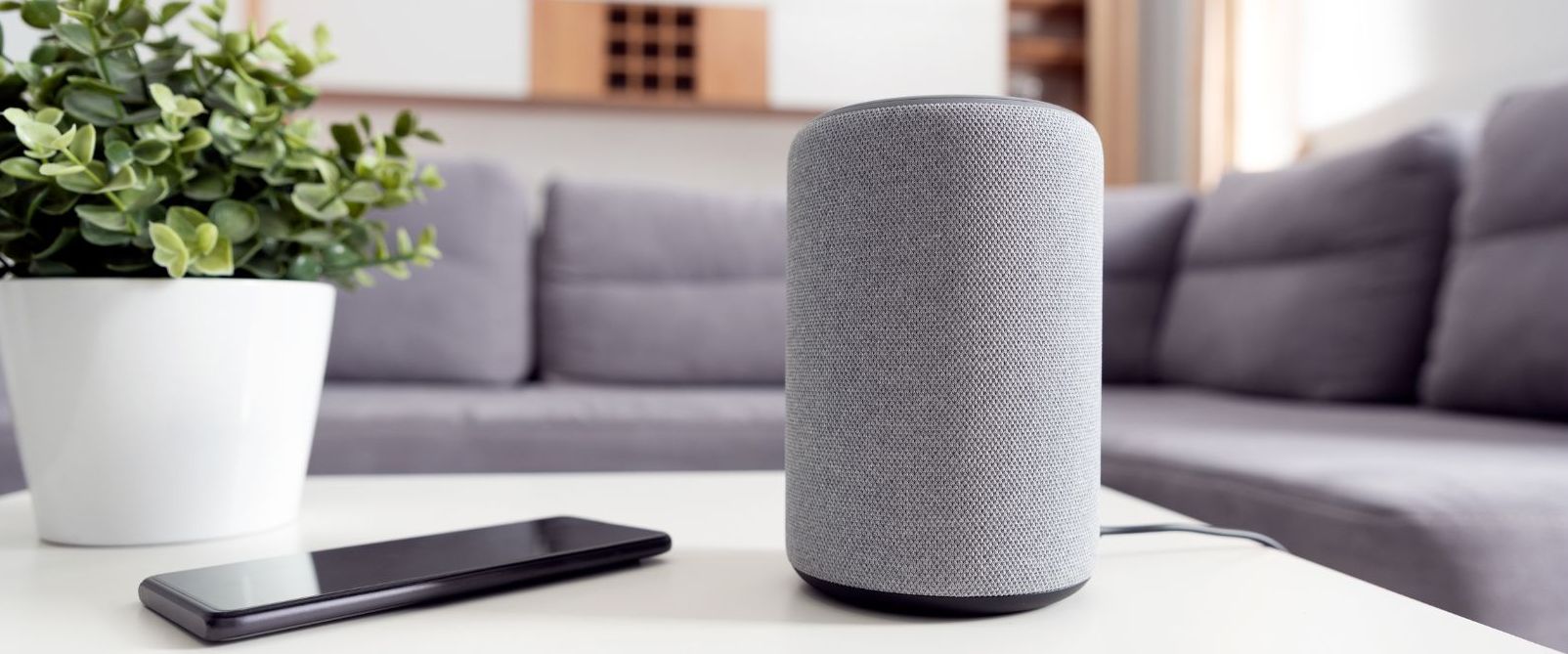
Challenges and Limitations
Despite advancements, challenges persist, such as dealing with complex language structures, accents, and contextual understanding.
Despite significant advancements, the field of speech recognition and AI-powered voice technologies faces several challenges and limitations. One major challenge is dealing with complex language structures and the subtleties of human speech. Languages are not just a collection of rules and vocabulary but are dynamic, evolving, and deeply embedded with cultural nuances. AI systems often struggle with understanding sarcasm, humour, idiomatic expressions, and regional dialects. These subtleties are not just linguistic challenges but also cultural, requiring a level of understanding that goes beyond algorithms and datasets.
Another major challenge is accent recognition. People from different regions or those speaking a second language often have distinct accents, which can pose recognition difficulties for AI systems. While strides have been made in this area, there is still a long way to go in ensuring that speech recognition technology is inclusive and accessible to all, regardless of accent or dialect. Additionally, contextual understanding remains a hurdle. Human speech is often laden with contextual cues, which can drastically alter the meaning of a phrase or word. AI systems must be able to understand not just the speech but the context in which it is spoken, a task that is easier said than done.
These challenges are compounded by technical limitations such as background noise, overlapping speech, and varying speech speeds, which can all impact recognition accuracy. Furthermore, the ethical implications of AI in voice recognition, particularly around privacy and data security, are ongoing concerns. As we continue to integrate these technologies into our daily lives, addressing these challenges becomes not just a technical necessity but also a societal imperative.
Future Trends in Speech Recognition
The future looks promising with advancements like emotion recognition and multilingual support, enhancing the interaction between humans and machines.
The future of speech recognition technology holds exciting prospects, with advancements poised to further enhance human-machine interaction. One promising trend is the integration of emotion recognition. AI systems are being developed to not only understand what is being said but also how it is said, recognising emotional cues such as stress, happiness, or anger. This capability could revolutionise customer service, mental health applications, and many other fields by enabling more empathetic and responsive interactions.
Another significant trend is the push towards multilingual support and cross-lingual understanding. As AI systems become more adept at handling multiple languages, the potential for global communication and information exchange increases dramatically. This includes not only major world languages but also regional dialects and minority languages, fostering inclusivity and preserving linguistic diversity.
Advancements in natural language understanding (NLU) are also on the horizon, enabling AI systems to grasp more complex language structures and contextual subtleties. This includes understanding indirect requests, sarcasm, and nuanced language variations. Furthermore, the integration of AI in speech recognition with other emerging technologies, such as augmented reality (AR) and virtual reality (VR), opens new avenues for immersive and interactive experiences.
However, these advancements are not without their challenges. Ensuring that these future technologies are developed ethically and responsibly, with a focus on privacy, security, and inclusivity, remains a critical consideration. As we move towards an increasingly AI-integrated future, balancing innovation with ethical responsibility will be key.
Ethical AI Development
The development of AI in speech recognition must be aligned with ethical standards, focusing on unbiased, transparent, and responsible AI systems.
The ethical development of AI in speech recognition is a crucial concern, as it impacts not just the effectiveness of the technology but also its societal acceptance and trustworthiness. Ethical AI development encompasses several key aspects, including fairness, transparency, accountability, and privacy. One of the primary concerns is the mitigation of bias in AI systems. Biases in training data can lead to skewed AI models that perpetuate stereotypes or discriminate against certain groups. It is, therefore, essential to ensure that datasets are diverse and representative of the global population.
Transparency in AI development is also vital. Users should be aware of how AI systems operate, the data they use, and the decision-making processes behind them. This transparency builds trust and allows users to understand and interact with AI systems more effectively. Additionally, accountability in AI development is crucial, especially in cases where AI systems may make errors or cause harm. Clear guidelines and mechanisms should be in place to address any issues that arise, ensuring that AI systems are not just advanced but also responsible.
Privacy is another critical aspect of ethical AI development. With voice-activated devices and speech recognition technologies becoming more pervasive, safeguarding user data and ensuring it is used ethically and responsibly is paramount. This involves not only secure data handling practices but also user control over their data and its usage. In sum, ethical AI development in speech recognition is not just a technical endeavour but a commitment to creating technology that respects and enhances human values and rights.
Key Tips To Ensure Quality Voice-Activated AI Tech
- Ensure ethical collection and use of speech data.
- Recognise the importance of machine learning in enhancing speech recognition accuracy.
- Understand the significance of language diversity in training AI systems.
- Be aware of the current limitations and challenges in AI-powered speech recognition.
- Anticipate future trends and innovations in the field.
Way With Words excels in providing customised and ethically sourced data collections for speech and other AI applications. Our services enhance AI language and speech development, ensuring quality and diversity in data.
In conclusion, AI’s role in speech recognition and voice-activated devices and technologies is monumental. It has not only facilitated easier human-machine interaction but also opened doors for advancements in various professional fields. However, with great power comes great responsibility. The ethical collection and use of data, understanding the nuances of language diversity, and overcoming current limitations are pivotal challenges that need addressing. As we continue to innovate, it is imperative to align these technologies with ethical standards, ensuring they serve humanity positively.
More Resources on AI Voice-activated Devices
Speech Dataset Creation: Way With Words Speech Collection. “We create speech datasets including transcripts for machine learning purposes. Our service is used for technologies looking to create or improve existing automatic speech recognition models (ASR) using natural language processing (NLP) for select languages and various domains.”
Transcript Polishing Services: Way With Words Machine Transcription Polishing. “We polish machine transcripts for clients across a number of different technologies. Our machine transcription polishing (MTP) service is used for a variety of AI and machine learning purposes. User applications include machine learning models that use speech-to-text for artificial intelligence research, FinTech/InsurTech, SaaS/Cloud Services, Call Centre Software and Voice Analytic services for the customer journey.”
What is speech recognition? Speech recognition, also known as automatic speech recognition (ASR), computer speech recognition, or speech-to-text, is a capability which enables a program to process human speech into a written format.